Table of Contents
- Combining Similar Data from Multiple Data Sources
- Working with Low-quality, Inconsistently Formatted Data
- Data Consumers Question Analytics, Reports and Recommendations
- Remediating Data Quality Issues with Master Data Management
- Unleash the Power of Your Azure Investment with Natively Integrated Master Data Management
In this four-part blog series, we explore the critical, complementary disciplines of data governance and master data management (MDM). While these principles hold true for any deployment, here we focus on the Azure cloud.
This is Part 1 in the series. Be sure to read Part 2 on the risks poor data quality poses to your cloud investment, Part 3 on how MDM can unleash the power of your governance program and Part 4 on why Microsoft Purview and Profisee MDM are the clear choice for governance and MDM in Azure.
In the seemingly never-ending race to gain a competitive edge in today’s digital world, companies often look to migrate their data and applications to cloud-native environments to scale their existing IT investments, take advantage of enhanced security features and benefit from simplified management and monitoring.
And Microsoft’s Azure cloud platform is an industry leader in helping them build scalable applications in the cloud and access on-premises data centers so they can rest easy knowing their data and applications are securely backed up.
But for an Azure investment to truly deliver business value through comprehensive analytics and insights, it will often combine data from across the organization to provide complete visibility to both IT and business users.
When it does, it is an ideal digital transformation vehicle that brings together data and infrastructure with a modern cloud approach. This gives companies tremendous opportunities to ”re-imagine” their business, including use cases such as cross-selling and upselling with machine learning (ML) and artificial intelligence (AI), building customer loyalty programs for digital transformation and strategic sourcing and procurement for manufacturing and supply chain management.
But how do you know you can trust the quality, consistency and accuracy of your business data in Azure? Below are some common challenges organizations face when combining business data in Azure and preparing it for business use.
Combining Similar Data from Multiple Data Sources
Organizations moving their data and applications to the Azure cloud often start with their most business-critical data — what we refer to as master data.
Master data can be considered the “nouns” of your business and can include customers, products, suppliers, locations and more — essentially any core data assets that organizations use across the enterprise.
But companies starting their cloud-migration journey often find they have similar data stored across several disparate source systems and databases.
For example, a large financial institution may have a partial record for a single customer in their Dynamics 365 CRM that contains transaction information and recent marketing campaigns that customers have interacted with — and another record for that same customer in their financial-reporting system with pricing terms, credit scoring and accounting information.
Or a manufacturer may have business data stored across several ERPs due to a half-dozen recent acquisitions and have inconsistent customer IDs, payment terms and transaction history among them.
It is clear how these data, or IT-related, issues can quickly grow into critical business failures.
Perhaps a customer approved for a $10,000 line of credit can successfully purchase $50,000 worth of goods if she orders from different subsidiaries. Or maybe a checking account customer who has opted out of marketing emails will still receive them from the credit card department because they track their CAN-SPAM and GDPR opt-outs in separate systems.
These are just a few examples of how duplicate, inconsistent and incomplete data can inhibit the value of an investment in Azure, causing leaders to doubt the effectiveness of their IT initiatives and potentially reduce investment in future projects.
Working with Low-quality, Inconsistently Formatted Data
Beyond having duplicate records of similar data types, another symptom of data quality issues in Azure is the inability to compare and use data side-by-side because of inconsistent formatting or completeness.
Symptoms of low-quality data include a failure to meet data-quality rules — or perhaps a lack of data-validation rules at all.
Data is of high quality when:
1) It is fit for the intended purpose and use.
2) The data correctly represents the real-world construct that the data describes.
But these rules may contradict one another. For example, a customer record in an ERP system may be sufficient for issuing and receiving payment but otherwise lacks critical information required to provide timely customer service.
This highlights the importance of the customer master record, the comprehensive record of a customer with all the relevant attributes, hierarchical information and lineage required to provide a seamless shopping experience, provide responsive customer service and receive timely payments.
Low-quality data users often complain about constant “data clean-up,” or having to manually match, merge, correct and compare records from multiple systems. Whether manually manipulating data in spreadsheets or consolidating records into a reporting or business intelligence (BI) tool like Power BI, this fails to correct the issue at its core as it is incredibly difficult — or impossible — to maintain over time.
Data Consumers Question Analytics, Reports and Recommendations
The unfortunate result of poor-quality data throughout the organization is that IT and business users alike continually question the analytics, reports and recommendations derived from enterprise data.
Incremental revenue may not be attributed to right product or suite of products. Leaders cannot identify poorly performing sales regions. The marketing team does not know who has opted out of email communications or who has requested a copy of their data under GDPR.
These issues often compound and find their way to business operations and customer interactions.
The supply chain team cannot automate procurement or delivery processes without reliable location information. The marketing team overspends, and annoy theirs prospects, by sending the same material more than once to the same person — with the name and address spelled a bit differently.
End users are unable to run simple reports about the state of the business without continuous data clean-up, much less take advantage of predictive analytics or opportunities identified by the BI team.
This can create a toxic data culture and stymie innovation. When no one can agree on a single source of the truth, collaboration diminishes and organizations suffer.
Complete
Data that is integrated and consolidated into a single, complete version of the truth for decision making.
Current
Data that is timely and current while also being consistent, clean and compliant.
Consistent
Data that is built and validated according to the same business rules irrespective of source system.
Clean
Data that is standardized, verified, matched and deduplicated.
Remediating Data Quality Issues with Master Data Management
Thankfully, companies have a way to remediate their data quality issues at the source and arrive at a single source of truth: master data management (MDM).
MDM serves as a hub for matching and merging records and implementing data quality standards for undeniable trust. For the organization taking advantage of the Azure cloud stack, they need a solution that can be quickly implemented with a native Azure integration and can scale to additional use cases.
Having an effective data governance strategy or dedicated application like Microsoft Purview makes an MDM implementation even more effective, as the work done during the data governance phase significantly informs the master data model.
Comprehensively scanning data sources, identifying lineage information and assigning data sensitivity labels is a helpful step in determining the scale of data quality issues.
While governance can identify data sources containing similar information and specify rules to govern that information, MDM can look at those data sources and identify missing, conflicting, duplicated and outdated information and then correct these problems by uniformly enforcing the data governance rules. This will typically involve matching, merging, validating, and correcting the data. Most often this involves syncing it back to source systems as well as publishing it to downstream systems for consumption.
MDM platforms can connect data from multiple source systems, including ERPs, CRMs, custom applications, cloud applications, legacy apps and more. They also excel in multi-domain use cases like combining householding, product and contract information for risk-based pricing in finance, or coupling provider, patient and treatment data in healthcare.
Unleash the Power of Your Azure Investment with Natively Integrated Master Data Management
Master data management provides a single source of truth for data in Azure and is the best path to ensure data quality because of its multi-source, multi-domain design.
A competitive MDM solution should be fast to implement and show value and be able to scale to any type of data in Azure. It should also natively integrate with the full Azure tech stack in a containerized, Platform as a Service (PaaS) environment.
The right MDM platform gives organizations the quality and trust in data necessary to fulfill business goals in Azure. Simply put, effective master data management can help unleash the power of your data and maximize the value of your Azure investment.
To learn more about how MDM can seamlessly complement your Azure investment, explore the Profisee platform see how it seamlessly integrates into Azure to deliver high-quality, trusted data across the Azure data estate.
For the full story and technical details, download The Complete Guide Data Governance and Master Data Management in Azure.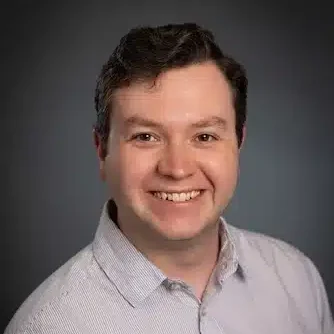
Forrest Brown
Forrest Brown is the Content Marketing Manager at Profisee and has been writing about B2B tech for eight years, spanning software categories like project management, enterprise resource planning (ERP) and now master data management (MDM). When he's not at work, Forrest enjoys playing music, writing and exploring the Atlanta food scene.