What is Data Quality | Management, Tools, Dimensions and Best Practices [Updated 2024]
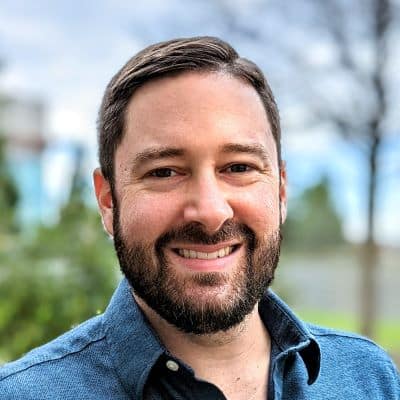
![Header image for Data Quality - What, Why, How, 10 Best Practices & More [2023]](https://profisee.com/wp-content/uploads/2023/08/Skyscraper-DataQuality_Social_1200x628.jpg)
Updated: May 17, 2024
According to a recent Gartner article, 84 percent of customer service and service support leaders cited customer data and analytics as “very or extremely important” for achieving their organizational goals in 2023. As data is becoming a core part of every business operation, the quality of the data that is gathered, stored and consumed during business processes will determine the success achieved in doing business now and in the foreseeable future.
Download your copy of the comprehensive guide to keep in your back pocket. Or if you are ready to learn more, continue reading below.
October
Start reading!
What is data quality?
Treat data as the foundation for a hierarchy where data is at the bottom level. On top of data, you have information, being data in context. Further up, we have knowledge seen as actionable information and on the top level, wisdom as the applied knowledge.
If you have poor data quality, your information’s credibility suffers. With bad information quality, you lack actionable knowledge in business operations and are unable to apply that knowledge or do it incorrectly resulting in risky business outcomes.
There are several definitions of data quality. The two predominant ones are:
- Data is of high quality if it is fit for its intended use or purpose.
- Data is of high quality if it correctly represents the real-world construct it describes.
These two possible definitions may contradict each other. If, for example, a customer master data record is fit for issuing an invoice for receiving payment, it may be fit for that purpose. But if that same customer master data record is also incomplete or incorrect for customer service, because the data incorrectly describes the who, what and where of the real-world entity of having the customer role in that business operation, we have a business problem.
Master data must be suitable for multiple purposes. You can achieve that by ensuring real-world alignment. On the other hand, it might not be profitable and proportionate to strive for the perfect real-world alignment in order to have data fit for the intended purpose within the business objective where a data quality initiative is funded. In practice, it is about striking a balance between these two definitions.
One of the biggest contributing factors for data inaccuracy is simply human error. Avoiding or eventually correcting low quality data caused by human errors requires a comprehensive effort with the right mix of remedies concerning people, processes and technology.
Other top reasons for data inaccuracies are a lack of communication between departments and inadequate data strategy. Solving such issues calls for passionate top-level management involvement.
The Importance of data quality
Usually, it is not hard to get everyone in a business, including the top-level management, to agree that having good data quality is good for business. In the current era of digital transformation, the support for focusing on data quality has improved.
However, when it comes to the essential questions about who is responsible for data quality, who must do something about it and who will fund the necessary activities, there remains a challenge.
Data quality resembles human health. Accurately testing how any one element of our diet and exercising may affect our health is fiendishly difficult. In the same way, accurately testing how any one element of our data may affect our business is difficult as well.
Nevertheless, numerous experiences tell us that bad data quality is not very healthy for business.
classic examples are:
In marketing you overspend, and annoy your prospects, by sending the same material more than once to the same person – with the name and address spelled a bit different. The problem here is duplicates within the same database and across several internal and external sources.
- In online sales, you cannot present sufficient product data to support a self-service buying decision. The issues here are the completeness of product data within your databases and how product data is syndicated between trading partners.
- In the supply chain, you cannot automate processes based on reliable location information. The challenges here are using the same standards and having the necessary precision within the location data.
- In financial reporting, you receive different answers for the same question. This is due to inconsistent data, varying data freshness and unclear data definitions.
On a corporate level, data quality issues have a drastic impact on meeting core business objectives, as:
- Inability to promptly react to new market opportunities thus hindering profit and growth achievements. Often this is due to not being ready for repurposing existing data that were only fit for yesterday’s requirements.
- Obstacles in implementing cost reduction programs, as the data that must support the ongoing business processes needs too much manual inspection and correction. Automation will only work on complete and consistent data.
- Shortcomings in meeting increasing compliance requirements. These requirements span from privacy and data protection regulations as GDPR, health and safety requirements in various industries to financial restrictions, requirements and guidelines. Better data quality is most times a must in order to meet those compliance objectives.
- Difficulties in exploiting predictive analysis on corporate data assets resulting in more risk than necessary when making both short-term and long-term decisions. These challenges stem from issues around the duplication of data, data incompleteness, data inconsistency and data inaccuracy.
How to Improve Data Quality and Build a Data Quality Strategy
Improving data quality and creating a data quality strategy takes a balanced of encompassing people, processes and technology as well as a good portion of top-level management involvement.
Data Quality Dimensions
When improving data quality, the aim is to measure and improve a range of data quality dimensions.
Uniqueness
Uniqueness is a critical data quality dimension, especially for customer master data. Duplicates — where two or more database rows describe the same real-world entity — are a common issue. To address this, implement measures such as intercepting duplicates during the onboarding process and conducting bulk deduplication of existing records. Use tools and algorithms designed for duplicate detection and resolution to maintain clean data.
Completeness
With product master data, completeness is often a significant challenge. Different product categories require different completeness criteria. Establish clear data collection standards and guidelines for each product category. Regularly audit and update data to ensure it meets these standards. Utilize automated systems to flag incomplete data entries and prompt immediate corrections.
Consistency
Consistency is crucial when dealing with location master data due to global variations in postal address formats. Implement standardized address formats and validation checks across your database. Use location intelligence tools like Melissa or Dun & Bradstreet to harmonize addresses and ensure consistency. Regularly review and update your data to account for changes in postal codes and address formats.
Precision
Precision in location and customer data is vital, as different use cases require varying levels of detail. Define the necessary precision for each use case—whether a postal address or a geographic position. Use geocoding services to enhance the precision of your location data. Regularly assess and adjust the precision levels based on evolving business needs.
Conformity
Conformity in product data, such as unit measurements, can be challenging due to regional differences. Establish a unified measurement system and convert units where necessary. For example, standardize length measurements to either inches or centimeters based on your target market. Provide clear guidelines for data entry to ensure conformity across all records.
Timeliness
Timeliness — having data available when needed — is a fundamental data quality dimension. Implement real-time data synchronization and updates to ensure timely availability. Use data monitoring tools to track the timeliness of your data and identify any delays promptly. Establish protocols for regular data refreshes to maintain up-to-date information.
Accuracy, Validity and Integrity
Accuracy involves ensuring data aligns with real-world values or verifiable sources. Regularly verify data against external sources and correct any discrepancies. Validity ensures data meets specified business requirements. Use validation rules and automated checks to enforce data validity. Integrity involves maintaining consistent relationships between entities and attributes. Implement relational database management systems to uphold data integrity and perform regular integrity checks.
By applying these actionable insights, you can effectively measure and improve your data quality against these essential dimensions.
Data Quality Management
In data quality management, the goal is to exploit a balanced set of remedies in order to prevent future data quality issues and to cleanse (or ultimately purge) data that does not meet the data quality Key Performance Indicators (KPIs) needed to achieve the established business objectives.
The data quality KPIs will typically be measured on the core business data assets within the data quality dimensions as data uniqueness, completeness, consistency, conformity, precision, relevance, timeliness, accuracy, validity and integrity.
The data quality KPIs must relate to the KPIs used to measure the business performance in general.
The remedies used to prevent data quality issues and eventual data cleansing includes these disciplines:
- Data Governance
- Data Profiling
- Data Matching
- Data Quality Reporting
- Master Data Management (MDM)
- Customer Data Integration (CDI)
- Product Information Management (PIM)
- Digital Asset Management (DAM)
Data Governance
A data governance framework must lay out the data policies and data standards that set the bar for what data quality KPIs are needed and which data elements should be addressed. This includes what business rules must be adhered to and underpinned by data quality measures.
Furthermore, the data governance framework must encompass the organizational structures needed to achieve the required level of data quality. This includes a data governance committee or similar, roles as data owners, data stewards, data custodians or similar in balance with what makes sense in a given organization.
A business glossary is another valuable outcome of data governance used in data quality management. The business glossary is a primer to establish the metadata used to achieve common data definitions within an organization and eventually in the business ecosystem where the organization operates.
Data Profiling
It is essential that the people who are responsible for data quality and those who are tasked with preventing data quality issues and data cleansing have a deep understanding of the information at hand.
Data profiling is a method, often supported by dedicated technology, used to understand the data assets involved in data quality management. These data assets are often populated by different people operating under varying business rules and gathered for bespoke business objectives.
In data profiling, the frequency and distribution of data values are counted on relevant structural levels. Data profiling can also be used to discover the keys that relate to data entities across different databases and to the degree that this is not already done within the single databases.
Data profiling can directly measure data integrity and be used as input to set up the measurement of other data quality dimensions.
Data Matching
When it comes to real-world alignment, using exact keys in databases is not enough.
The classic example is how we spell the name of a person differently due to misunderstandings, typos, use of nicknames and more. With company names, the issues just pile up with funny mnemonics and the inclusion of legal forms. When we place these persons and organizations at locations using a postal address, the ways of writing that has numerous outcomes too.
Data matching is a technology based on match codes, for example, Soundex , fuzzy logic and increasingly also machine learning used to determine if two or more data records are describing the same real-world entity (typically a person, a household or an organization).
This method can be used in deduplicating a single database and finding matching entities across several data sources.
Oftentimes, data matching is based on data parsing where names, addresses and other data elements are split into discrete data elements. For example, an envelope type address is split into building name, unit, house number, street, postal code, city, state/province and country. This may be supplemented by data standardization using the same value for street – Str and St.
Data Quality Reporting and Monitoring
The findings from data profiling can be used as input to measure data quality KPIs based on the data quality dimensions relevant to a given organization. The findings from data matching are especially useful for measuring data uniqueness.
In addition to that, it is helpful to operate a data quality issue log, where known data quality issues are documented, and the preventive and data cleansing activities are followed up.
Organizations focusing on data quality find it useful to operate a data quality dashboard highlighting the data quality KPIs and the trend in their measurements as well as the trend in issues going through the data quality issue log.
Master Data Management (MDM)
The most difficult data quality issues are related to master data as party master data (customer roles, supplier roles, employee roles and more), product master data and location master data.
Preventing data quality issues in a sustainable way and not being forced to launch data cleansing activities over and again will, for most organizations, mean that a master data management (MDM) framework must be in place.
MDM and Data Quality Management (DQM) are tightly coupled disciplines. MDM and DQM will be a part of the same data governance framework and share the same roles as data owners, data stewards and data custodians. Data profiling activities will most often occur with master data assets. When doing data matching, the results must be kept in master data assets controlling the merged and purged records and the survivorship of data attributes relating to those records.
Customer Data Integration (CDI)
Customer master data is sourced in many organizations from a range of applications. These are self-service registration sites, Customer Relationship Management (CRM) applications, ERP applications, customer service applications and many more.
Besides setting up the technical platform for compiling the customer master data from these sources into one source of truth, there is a huge effort in ensuring the data quality of that source of truth. This involves data matching and a sustainable way of ensuring the right data completeness, the best data consistency and adequate data accuracy.
Product Information Management (PIM)
As a product manufacturer, you need to align your internal data quality KPIs with those of your distributors and merchants in order to allow your products to be chosen by end customers wherever they have a touchpoint in the supply chain. This must be done by ensuring the data completeness and other data quality dimensions within the product data syndication processes.
As a merchant, you will collect product information from many suppliers with each having their data quality KPIs – or not having that yet. Merchants must work closely with their suppliers and strive to maintain a uniform way of receiving product data of the best quality according to the data quality KPIs on the merchant side.
Digital Asset Management (DAM)
Digital assets are images, text documents, videos and other files often used in conjunction with product data. In the data quality lens, the challenges for this kind of data are around correct relevant tagging (metadata) as well as the quality of the assets. For example, if a product image only shows the product clearly and not much else.
Top 10 Data Quality Best Practices
The following we will be based on the reasoning provided above in this post, list a collection of 10 highly important data quality best practices.
These are:
Ensuring top-level management involvement.
Quite a lot of data quality issues are only solved by having a cross-departmental view.
Managing data quality activities as a part of a data governance framework.
This framework should set the data policies, data standards, the roles needed and provide a business glossary.
Occupying roles as data owners and data stewards from the business side of the organization and occupy data custodian roles from business or IT where it makes the most sense.
Using a business glossary as the foundation for metadata management.
Metadata is data about data and metadata management must be used to have common data definitions linking those to current and future business applications.
Operating a data quality issue log with an entry for each issue with information about the assigned data owner and the involved data steward(s), the impact of the issue, the resolution and the timing of the necessary proceedings.
For each data quality issue raised, start with a root cause analysis.
The data quality problems will only go away if the solution addresses the root cause.
When finding solutions, striving to implement processes and technology that prevent the issues from occurring as close to the data onboarding point as possible rather than relying on downstream data cleansing.
Defining data quality KPIs that are linked to the general KPIs for business performance.
Data quality KPIs, sometimes also called Data Quality Indicators (DQIs), can be related to data quality dimensions for example data uniqueness, data completeness and data consistency.
Using anecdotes about data quality train wrecks to get awareness around the importance of data quality.
However, also using fact-based impact and risk analysis to justify the solutions and the needed funding.
Nowadays, a lot of data is already digitalized. Therefore, avoid inputting data where possible.
Instead, try to find cost-effective solutions for data onboarding utilizing third-party data sources for publicly available data. For example, with locations in general and names, addresses and IDs for companies and some cases individual persons. For product data, utilize second-party data from trading partners where possible.
Data Quality Tools and Platforms
Data quality tools play a crucial role in today’s data-driven world, helping organizations ensure that their data is accurate, reliable, and consistent. These tools help businesses address common data quality issues, such as duplicate records, missing information, and inconsistent formatting, which can significantly impact decision-making and operational efficiency.
One of the primary reasons data quality tools are vital is that they enable organizations to trust their data. Accurate and reliable data is fundamental for making informed decisions, reducing errors, and avoiding costly mistakes. Data quality tools help organizations identify and rectify data inaccuracies, ensuring that their data remains a valuable asset rather than a liability. Data quality tools also aid in complying with data regulations, such as GDPR and CCPA, which require organizations to manage and protect personal information responsibly.
The importance of data quality tools, such as those offered by Profisee, cannot be overstated in today’s data-centric business landscape. These tools ensure that organizations can rely on their data for critical decisions, maintain operational efficiency, and stay compliant with data regulations. With the ability to automate data cleaning and ensure data consistency, data quality tools provide a strong foundation for organizations to harness the true power of their data, unlocking new opportunities and maintaining a competitive edge in the market.
Types of Data Quality Tools
Data quality tools encompass a variety of functionalities and capabilities to ensure that data is accurate, consistent, reliable, and compliant with relevant standards and regulations. Broadly, these tools can be categorized into several types or categories, including:
Data Profiling Tools
These tools analyze and assess the quality of data by examining its structure, content, and relationships. They help identify anomalies, outliers, and patterns within the data, giving organizations insights into the overall health of their data.
Data Cleansing Tools
Data cleansing tools focus on correcting or removing errors and inconsistencies within the data. They can automatically standardize formats, eliminate duplicate records, and address missing or inaccurate data values, improving data accuracy and completeness.
Data Matching and Deduplication Tools
These tools are specifically designed to identify and eliminate duplicate or redundant records within datasets. They use various algorithms and techniques to detect similar or identical data entries, helping maintain data integrity.
Data Validation Tools
Data validation tools check data against predefined rules and criteria to ensure it meets specific standards and validation requirements. These tools help organizations maintain data quality by flagging data that does not conform to set guidelines.
Data Enrichment Tools
Data enrichment tools augment existing data with additional information from external sources, such as public databases or third-party providers. This process can enhance the completeness and accuracy of data, making it more valuable for analysis and decision-making.
Data Governance and Data Quality Management Tools
These tools provide a framework and set of processes to establish data governance policies, data quality rules, and data stewardship practices within an organization. They help define roles and responsibilities for managing data quality and compliance.
Data Monitoring and Profiling Tools
Data monitoring tools continuously track and analyze data as it enters and exits the organization. They can trigger alerts and notifications when data quality issues are detected, allowing for real-time data quality management.
Master Data Management (MDM) Tools
MDM tools focus on creating and managing a single, authoritative source of master data across an organization. They help maintain consistency and accuracy in critical data elements like customer information, product data, and employee records.
Data Quality Reporting and Dashboards
These tools offer visualization and reporting capabilities to help organizations monitor and communicate the state of data quality. They provide insights into data quality metrics, trends, and areas that require attention.
Data Quality Assessment and Profiling Tools
These tools are designed to assess data quality on a periodic or ad-hoc basis, helping organizations evaluate their data quality, identify issues, and prioritize data improvement efforts.
Each of these types of data quality tools plays a crucial role in ensuring that data is fit for its intended purpose, maintaining data integrity, and supporting informed decision-making within an organization. The choice of which tools to use depends on the specific data quality challenges and objectives of the organization.
Data Quality Resources
There are many data quality resources available where you can learn more.
Below is a list of some of the useful resources for framing a data quality strategy and addressing specific data quality issues:
Larry P. English is the father of data and information quality management. His thoughts are still available here: https://www.information-management.com/author/larry-english-im30029
Thomas C. Redman, aka the Data Doc, writes about data quality and data in general on Howard Business Review. His articles are found here: https://hbr.org/search?term=thomas%20c.%20redman
David Loshin has made a book with the title The Practitioners’ Guide to Data Quality Improvement https://dataqualitybook.com/?page_id=2
Gartner, the analyst firm, has a glossary with definitions of data quality terms here: https://www.gartner.com/it-glossary/?s=data+quality
Massachusetts Institute of Technology (MIT) has a Total Data Management Program (TDQM) https://web.mit.edu/tdqm/www/index.shtml
Knowledgent, a part of Accenture, provides a white paper on Data Quality Management here: https://knowledgent.com/whitepaper/building-successful-data-quality-management-program/
Deloitte has published a case study called data quality driven, customer insights enabled: https://www2.deloitte.com/us/en/pages/deloitte-analytics/articles/data-quality-driven-customer-insights-enabled.html
An article on bi-survey examines why data quality is essential in Business Intelligence https://bi-survey.com/data-quality-master-data-management
The University of Leipzig has a page on data matching in big data environments (they call it dedoop) https://dbs.uni-leipzig.de/dedoop
A Toolbox article by Steve Jones goes through How to Achieve Quality Data in a Big Data context https://it.toolbox.com/blogs/stevejones/how-to-achieve-quality-data-111618
An Information Week article points to 8 Ways To Ensure Data Quality https://www.informationweek.com/big-data/big-data-analytics/8-ways-to-ensure-data-quality/d/d-id/1322239?image_number=1
Data Quality Pro is a site, manged by Dylan Jones, with a lot of information about data quality: https://www.dataqualitypro.com/
Obsessive-Compulsive Data Quality (OCDQ) by Jim Harris is an inspiring blog about data quality and its related disciplines https://www.ocdqblog.com/
Nicola Askham runs a blog about data governance: https://www.nicolaaskham.com/blog One of the posts in this blog is about what to include in a data quality issue log: https://www.nicolaaskham.com/blog/2018-21-02what-do-you-include-in-data-quality-issue-log
Henrik Liliendahl have a long-time running blog with over 1,000 blog posts about data quality and Master Data Management: https://liliendahl.com/
A blog called Viqtor Davis Data Craftmanship provides some useful insights on data management: https://www.viqtordavis.com/blog/
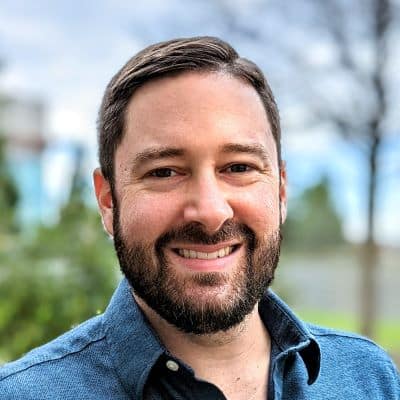
Benjamin Bourgeois
Ben Bourgeois is the Director of Digital Marketing at Profisee, where he works with product, engineering and pre-sales teams to develop useful content and resources for prospective and current customers. He has experience managing content teams across the B2B SaaS, healthcare, specialty chemical and manufacturing industries.