Table of Contents
- Data Governance vs. Data Quality: What's the Difference?
- How Data Governance and Data Quality Overlap
- Why Data Governance and Quality Are Important
- Examples of Data Governance
- Examples of Data Quality
- Integrating Data Quality Into Governance
- Using Data Governance to Improve Data Quality
- The Importance of Data Quality in Governance
- Data Quality Dimensions in Governance
- Ensuring Data Quality in Governance
- How Profisee MDM Can Help with Data Governance and Quality
- The Essential Role of Governance & MDM in Microsoft Fabric
Data governance and data quality are two areas of data management and data science that get a lot of attention — and for good reason. Both are important terms for team members of any data organization to know — from the data steward to the chief data officer (CDO) — as they’re crucial not only for business intelligence (BI) and data analytics but also for important issues like data privacy and data integrity.
In this article, we’ll explore the relationship between data governance and data quality to help you understand how they differ, where they overlap, why they’re so important, how you can incorporate them into your data strategy and more.
Data Governance vs. Data Quality: What’s the Difference?
While data governance and data quality are related, they are distinct concepts and practices. Here’s a quick overview of how they differ:
Data Governance | Data Quality | |
---|---|---|
Definition | A set of principles and practices that ensure high data quality through the complete lifecycle of your data |
|
Dimensions | Focuses on policy, strategy, roles, metrics, methodologies | Focuses on accuracy, completeness, consistency, uniqueness, validity, timeliness |
Process | Ongoing program with defined framework and processes | Result of processes and controls, though the processes evolve over time as new data sources, data domains and other variables are introduced |
Outcome | Enables data quality for establishing trust in data | Provides confidence in using data in downstream systems like BI, analytics and artificial intelligence (AI) in addition to helping maintain regulatory compliance and protect data privacy |
Data governance provides the high-level strategy and oversight needed to manage data, while data quality looks at the more tactical metrics and dimensions for ensuring data is fit for purpose.
Both work together, with governance enabling and monitoring quality — but they have different focuses in ensuring organizational data meets business needs.
How Data Governance and Data Quality Overlap
Data governance and data quality have important overlaps in how they help organizations manage their data. While their focuses differ, both practices aim to assess, define and manage data in several ways:
- Data governance establishes roles, policies, standards and metrics to oversee data. These policies help define what quality data looks like for the organization.
- Data quality management assesses data against benchmarks to identify quality issues. It also puts processes in place to improve and maintain quality over time.
- They both aim to make data “fit for purpose,” ensuring it meets the organization’s needs for operations, analytics, reporting and more. High-quality data that aligns with governance enables these business activities.
- Governance and quality management both require understanding how data is used across the business. This knowledge helps define appropriate governance policies and data quality dimensions to meet different requirements.
- Assessing data risks is another shared concern. Governance oversees risk identification and mitigation while quality management aims to reduce risks like inaccuracies, inconsistencies and inaccessibility.
- Both practices also seek to improve trust and reliability in data. Governance policies provide accountability, while consistent quality gives people confidence that the data is dependable.
Data governance and quality management work hand-in-hand to regulate, assess and enhance data for business value. When coordinated, they provide a robust structure for organizations to effectively oversee and optimize their data.
Why Data Governance and Quality Are Important
High-quality data and proper governance practices are essential for any successful data management strategy. There are several key reasons why organizations should prioritize improving data quality through governance.
1. Better Decision-Making
Data-driven decision-making relies on having accurate and reliable data. Low-quality data leads to poor analytic insights and flawed business decisions. With strong governance policies that improve quality, companies can trust their data to drive strategic choices.
2. Increased Efficiency
When data is error-free and consistent, analysts spend less time cleaning and prepping data. This enables them to focus on extracting insights. Good data quality also means less re-work caused by incorrect data. Higher-quality data results in greater productivity and efficiency.
3. Regulatory Compliance
Many regulations like GDPR require companies to have accurate customer data. Poor data quality can lead to compliance issues and penalties. Data governance helps monitor and enforce policies to fulfill regulatory needs and avoid fines. Documented data quality and governance procedures also demonstrate compliance readiness.
Examples of Data Governance
Data governance establishes the processes, policies, guidelines, roles and responsibilities for managing data within an organization. Here are some examples of key data governance activities:
- Policies: Developing organization-wide data policies that specify how data should be managed, including data security, privacy, retention and data-access policies. Policies provide guidelines for making decisions about enterprise data.
- Guidelines: Creating data standards, procedures and guidelines for how data should be collected, stored, integrated, transformed, archived, accessed and used. This includes guidelines for metadata, data models, data quality, master data management (MDM), taxonomies and more.
- Roles and responsibilities: Defining data governance roles and responsibilities, such as a data governance council, data stewards, data owner and data stewards to clarify accountability across business and IT.
- Data lifecycle management: Applying governance to key data lifecycle stages from creation and acquisition to disposal. This includes retention schedules, archival policies and procedures for defunct data.
- Data issue escalation: Having clear procedures for reporting data issues, gaps or conflicts and escalating them to the attention of data stewards and data governance committees.
- Compliance management: Ensuring data management complies with regulations, laws and organizational policies through audits, checks and controls.
- Change management: Managing changes in data, systems, policies and processes through a structured change control process.
- Risk management: Identifying, prioritizing and controlling data risks such as data breaches, lack of quality, conflicts or over-exposure.
Examples of Data Quality
In many cases, poor data quality is a product of human error. This could be as simple as someone making a typo when entering data into a source system, or it could be a data stewardship mistake, like accidentally assigning the wrong access permissions to a data set or record.
A simple example of this would be misspelling a customer’s name. This might not seem like that much of a problem at first, but if the same customer’s data gets entered into the system again because the existing record couldn’t be found, you now have a data duplication — and therefore a data quality — problem.
Having two customers in your system with the same address but slightly different name spellings could result in sending two shipments of an order to the same person. Likewise, if someone contacts your company to unsubscribe from direct-mail advertising, they might continue receiving mail because a duplicate record in your system went unnoticed due to the typo.
Let’s also consider an example for location data. If one system records location data of a shipment one way and another system records it in a different format or at different time intervals, you might not be able to automate certain supply chain processes because the location data is unreliable.
A focus on data quality can prevent issues like these by making sure records satisfy the right requirements so that you can use data the way you need to.
Integrating Data Quality Into Governance
Effective data governance requires integrating data quality practices throughout policies, processes and metrics. Here are some tips:
- Update policies: Make data quality an explicit part of data governance policies. Define quality expectations, standards and accountabilities.
- Build into processes: Integrate data quality checks and monitoring into key processes like data entry, migration, integration and analysis.
- Prioritize critical data: Focus governance processes on mission-critical data first, including broadly shared master data. Identify high-impact data and master data sources.
- Monitor with metrics: Track quality KPIs like accuracy, completeness, conformity and consistency rates while measuring against thresholds.
- Automate where possible: Automated data quality rules and checks enable scalable, consistent quality.
- Create data quality feedback loops: Collect input on quality issues from data consumers to drive continuous improvement.
- Communicate policies and metrics: Share data quality goals, policies, processes and metrics to align stakeholders.
- Make roles and ownership clear: Define data quality responsibilities across teams like IT, compliance and analytics.
- Train staff: Educate all personnel who impact data quality on governance policies and procedures.
Integrating data quality into formal governance frameworks, policies and procedures ensures it receives appropriate focus as a priority. Consistent processes and measurement enable sustainable quality improvements over time.
Using Data Governance to Improve Data Quality
Data governance provides the foundation and structure for improving data quality through standards, auditing and issue tracking. By instituting data governance policies, organizations can define rules and standards for how data should be managed at each stage of its lifecycle.
For example, an organization may create data entry standards that outline the required fields, formats and business rules for capturing high quality data from the source. Data stewards can audit ongoing data collection processes to ensure they adhere to these standards. Any issues identified can be logged and tracked through data governance issue-tracking tools.
Regular data quality assessments and auditing procedures should be incorporated into formal governance processes. As problems emerge, they can be addressed at the root cause through updated policies and standards. Data stewards have an ongoing responsibility to monitor compliance, analyze where quality is breaking down and recommend improvements.
In addition to reactive methods like audits and issue logs, data governance facilitates a proactive approach to continuous data quality improvement. This includes guidelines for data monitoring, profiling, cleansing and anomaly detection. Data quality KPIs can be defined and measured over time. All of these proactive methods are enabled through the systematic processes outlined by data governance policies and committees.
With a data governance foundation in place, organizations can build a culture focused on defining, measuring and improving data quality. Standards provide clear expectations, while governance committees ensure accountability across departments and roles. This allows organizations to scale their data quality initiatives and sustain reliable, high-quality data in the long run.
The Importance of Data Quality in Governance
High quality data is crucial for effective data governance. After all, it’s very difficult to govern data properly if that data is incomplete, inaccurate, inconsistent or otherwise flawed. Quality data enables good governance, while low quality data undermines governance efforts.
Some key reasons why data quality is so important for governance include:
- Trust: Users will not trust data they perceive as poor quality. This erodes confidence in governance policies.
- Integrity: Governance aims to ensure integrity in how data is managed. But this is meaningless if the data itself lacks integrity due to quality issues.
- Compliance: Many governance regulations require high quality data. Weak data makes compliance difficult.
- Analytics: Analytics, reporting and dashboards rely on quality data. Governance oversight is undermined by unreliable data.
- Decisions: Business decisions depend on quality data. Governance seeks to enable data-driven decisions, so quality is critical.
Organizations cannot hope to govern data properly unless they first ensure a solid quality foundation. By prioritizing and improving data quality, the goals of governance become far easier to fulfill.
Data Quality Dimensions in Governance
When implementing data governance, it’s important to consider the key dimensions that comprise data quality. Some of the main dimensions include:
- Accuracy: The data values and metrics must be correct and reliable. Inaccurate data can lead to faulty analysis and poor decision making. Data governance policies should aim to improve accuracy.
- Completeness: Data should have sufficient breadth, depth and scope for the intended purposes. There should be no critical omissions. Completeness ensures information is comprehensive.
- Consistency: Data should be presented in the same format and be compatible across systems. Consistent data is easier to analyze.
- Timeliness: Data should be current and up to date. Stale data has less value for analytics and reporting. Governance principles can ensure timeliness.
- Validity: Data should conform to syntax rules and business logic. Invalid data can cause errors. Validation checks can identify issues.
- Uniqueness: Duplicates and redundant data can skew analysis. Governance policies should aim to maintain uniqueness.
- Usability: Data should be discoverable, understandable and easy to work with for users. Governance involves making data more usable.
By considering these key dimensions of quality, data governance initiatives can significantly improve overall data health and reliability. The governance policies direct how data is managed and used to uphold quality standards.
Ensuring Data Quality in Governance
To ensure high quality data across an organization, data governance programs must incorporate ongoing quality assurance practices. This involves establishing policies, metrics and processes for issue resolution and audits to continually monitor and improve data quality.
Data Governance Policy
Formal data quality policies provide standards that data must meet. Policies typically specify dimensions like accuracy, completeness, consistency, and timeliness. They lay out exact thresholds or targets to achieve for key metrics. Policies also clarify stakeholder responsibilities for measuring, reporting on and correcting quality issues.
Metrics
Quantitative metrics make data quality tangible. Common metrics tracked include:
- Percent of records with incomplete or invalid entries
- Rate of accuracy based on audits or assessments
- Number of data defects reported and resolved
Metrics demonstrate where quality problems exist and whether policies are being followed. They enable data stewards to prioritize efforts.
Issue Resolution
A clear process for logging, prioritizing, investigating and resolving data defects is essential. Often this involves ticketing systems where users can report issues like inaccurate, outdated or incomplete data. Data stewards then triage and assign tickets to subject matter experts to diagnose root causes and correct problems.
Audits
Scheduled data quality audits examine a sample of data against benchmarks to gauge conformance to policies. Both manual inspection by data analysts and automated profiling tools can perform audits. Reviews help uncover where quality is lacking to target remediation. Ongoing auditing provides assurance that governance practices are working.
How Profisee MDM Can Help with Data Governance and Quality
If your organization is working to improve data quality through data governance, using a master data management (MDM) tool like Profisee in tandem with a data governance tool can take you much farther than relying on data governance alone.
While a data governance platform lets you define policies and standards for data quality and identify data quality issues, Profisee MDM gives you the power to enforce those policies and standards, improve missing and incomplete data and share that data with downstream systems and relevant stakeholders.
Profisee supports a bi-directional, native integration with Microsoft Purview for data governance in addition to integrations for data catalog, data glossary and metadata management tools. MDM is by no means a replacement for data governance; rather, the two work best when they work together.
To learn more about why MDM and data governance are better together — especially when it comes to AI readiness — download a copy of our guide, “The Essential Role of Governance & MDM in Microsoft Fabric.”
The Essential Role of Governance & MDM in Microsoft Fabric
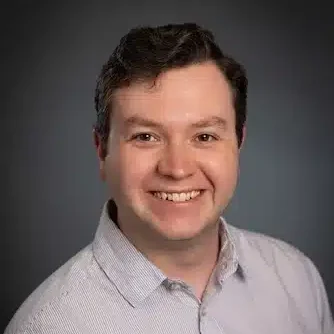
Forrest Brown
Forrest Brown is the Content Marketing Manager at Profisee and has been writing about B2B tech for eight years, spanning software categories like project management, enterprise resource planning (ERP) and now master data management (MDM). When he's not at work, Forrest enjoys playing music, writing and exploring the Atlanta food scene.