Table of Contents
Key Takeaways
Poor data quality compels analysts to spend significant time — often over 40% — on data validation and cleanup before meaningful analysis can begin. This not only delays decision making but also diverts resources from strategic initiatives.
Inaccurate data can lead to errors in product listings and order fulfillment, resulting in customers receiving incorrect items. Such mistakes diminish customer confidence and can lead to loss of business, as dissatisfied customers may turn to competitors.
Unreliable data hampers personalized marketing efforts, leading to irrelevant or mistimed promotions. This not only reduces the effectiveness of marketing campaigns but also risks alienating customers, thereby missing potential sales opportunities.
Bad data is more than an inconvenience. According to Gartner research, organizations that don’t resolve their issues with poor data quality are losing $9.7 million each year.
That adds up to a whopping $3 trillion per year for businesses in the U.S. says Harvard Business Review. This may not be surprising considering how reliant businesses are on their data. Nearly every decision maker including managers, data scientists, and all types of knowledge workers look to reports and analysis derived from company data.
The wrong data applied to an important decision can easily throw off projections that can kill projects and destroy earnings. The IT leader needs to understand how the company’s data is used and take aggressive measures to assure it is accurate and reliable.
The Known Problem
The fact that enterprise data may be unreliable is no surprise to the analysts who struggle with it and have possibly been burned by false assumptions made based on their findings. The estimated costs to enterprises are bad enough but most companies can’t definitively state whether they are performing better than or worse than the general projections.
The IT leader should identify all the data in the enterprise and put the right tools and practices in place to identify, manage, and make it usable and reliable without question. If they don’t already have systems in place to easily manage the discovery and management process, they are tacitly promoting the creation of false assumptions that will have lasting effects on their company. Here are the risks companies face by not taking control of their data.
Wasting Staff Time
Forrester’s report, “Data Performance Management Is Essential To Prove Data’s ROI” concludes, “Nearly one third of analysts spend more than 40 percent of their time vetting and validating their analytics data before it can be used for strategic decision-making.” That’s a lot of time spent before they can even begin their analysis. They are forced to spend so much time because businesses add new applications creating different incompatible data sets over their history.
When it’s time to bring that data together into something cohesive, the first step is to identify and resolve the differences and similarities in the multiple structures. Getting past these roadblocks slows down progress and changes the role of the data scientist to that of a ‘data wrangler’ all of which delays progress and wastes valuable time.
Keeping Customer Respect
Data drives customer interaction and product fulfillment, and bad data can cause missteps that can be difficult to recover from. Online catalogs can contain manually entered product information that customers rely on to make their purchase decisions, and both the customer and the company falsely assume the data is valid. But when the product that arrives at their door doesn’t match what they ordered the fault may be caused by differences between the catalog data and the fulfillment request.
At best the customer can return the item for replacement with the right one but two things happen. First the return process costs the company money reducing the profit on the sale or even creating a loss. Second the customer has lost time in the return process and faith that the company can deliver their next order properly.
Caution: Customers can easily find the same or similar items from other sellers and may never come back because of bad data.
Missing Chances
Marketing relies heavily on customer information in many forms because personalization enhances both the customer experience and the company’s ability to deliver based on each customer’s expectations and buying history. Accurate data can be used to present relevant promotions at the right times to customers eager to hear from them. But unmanaged data that leads to false assumptions can cause delivery of the wrong message, alienating the customer, or it can lead to presenting no message at all, in which case the opportunity for a sale is lost.
The IT leader who understands the issues at stake and takes charge of managing the enterprise data in its totality can make significant positive differences in how the company uses its data and how successful the varied uses of that data affect the success of efforts across multiple business segments. Addressing poor data quality is a “must do” for today’s IT leaders.
Interested in learning more? Download a copy of the Complete Guide to Data Quality below.
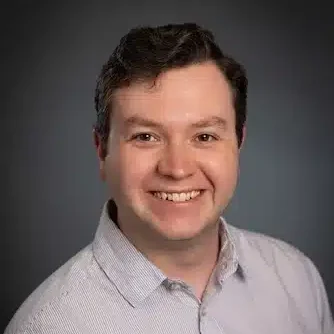
Forrest Brown
Forrest Brown is the Content Marketing Manager at Profisee and has been writing about B2B tech for eight years, spanning software categories like project management, enterprise resource planning (ERP) and now master data management (MDM). When he's not at work, Forrest enjoys playing music, writing and exploring the Atlanta food scene.