Table of Contents
- What Is Enterprise Data Quality?
- What Is Enterprise Data Management?
- What Are the Benefits of Maintaining Data Quality in an Enterprise?
- Who Should Be Responsible for Data Quality in an Enterprise?
- Enterprise Data Quality Methods and Best Practices
- Create a Data Strategy to Improve Enterprise Data Quality
Managing data across the enterprise ensures not just that the right data gets to the right place and the right people at the right time, but also that, when it does arrive, the people and systems who need it can use it for its intended purpose. This is why enterprise data quality is so important — it’s the bedrock upon which a modern, data-driven organization’s operations and analytics are built.
Without high-quality data, it’s very difficult and, in some cases, impossible, to use enterprise data to drive business outcomes like increasing revenue, boosting operational efficiency and deriving trustworthy insights to improve decision making at the highest levels of an organization.
What Is Enterprise Data Quality?
Enterprise data quality is the standard an organization sets for useful and consumable enterprise data — that is, data generated or owned by a business or organization. Quality in this sense not only describes the accuracy of the data itself, but whether that data can be used within company systems to a productive end.
For example, customer data collected by the sales team in Salesforce and by the marketing team in Marketo may describe the same customers, but if the formatting and metadata do not align, the information may be duplicated or unusable for analyzing the customer journey. Usable data is high quality and doesn’t require much further cleansing or reformatting to use.
What Is Enterprise Data Management?
Enterprise data management encompasses the processes, tools and policies defined and practiced by the organization that ensure consumable and useful data is maintained across the enterprise. The policies and procedures teams follow to implement enterprise data management extend across the data lifecycle from production through storage and use to disposal.
A healthcare company with good enterprise data management systems, for example, would define and document the usable parameters for providers within their network into a data governance policy. When data engineers write transfer scripts to save provider information, they use the policy to map data from the organization’s SaaS tools into the data warehouse where those disparate data sets become part of the golden record. When a business user accesses provider data for analysis, they can rest easy knowing the provider information from the golden record is correct and complete.
What Are the Benefits of Maintaining Data Quality in an Enterprise?
Building and maintaining high-quality, usable data across the enterprise pays dividends in business outcomes, with measurable effects for end users and business teams.
Consistency
There’s nothing more frustrating than when analysts from different teams present different findings on seemingly similar data sets. When data governance is in place and good data hygiene is practiced, the sales and marketing customer lists align, providing opportunities for customer lifecycle marketing that send offers and customized information to the right buying stage, rather than relying solely on discounts for revenue growth.
Reliable Forecasting
Reliable forecasts depend entirely on how complete and accurate the data sets are. Supply chain analysts armed with quality data can effectively identify at-risk suppliers based on current location data combined with public weather or political climate information within the company’s business intelligence (BI) tool. Without supplier data in formats designed for BI consumption, supply chain professionals may make similar guesses, but could easily lose sight of emergent conditions.
Assured Compliance
With so much data being created and consumed by companies, people are understandably concerned whether their data will truly be forgotten by those companies upon request. A company that maintains the quality of the personal identifying information (PII) of all its customers can comply with data regulations like GDPR quickly and completely because customer data lives in a single location rather than being spread across data silos.
Increased Efficiency
A company equipped with high quality, consumable data that complies with company data quality standards and data governance policies can quickly connect data sets with business software for use. Without proper maintenance protocols, all analysis, dashboard or forecast projects must account for the time it takes for data professionals to locate, cleanse, format and de-duplicate data prior to build.
Increased Revenue
Trustworthy data ready for use lets leaders act confidently in the face of difficult decisions because they understand the true state of the enterprise. They forecast better, allocating the right resources to the right team at the right time. They have better customer interactions because they understand the customers. All of these and more add up to a more efficient and effective enterprise that serves the needs of its employees and customers.
Who Should Be Responsible for Data Quality in an Enterprise?
When data quality is everyone’s responsibility, it quickly becomes no one’s priority. Enterprise data quality planning requires careful consideration of the needs of the business in line with the scope of individual job descriptions and levels of formal and informal influence across the organization. Consider publishing a roles and responsibilities document like the table below along with the company’s data governance policy.
Organizational Level | Example Titles | Role Responsibilities |
---|---|---|
Leadership | Chief Data Officer, Data Quality Manager | Define data governance policy objectives, ensure policy compliance |
Execution contributors |
|
Align business needs with data availability, define data formats, identify potential issues, provide guidance on workable outcomes |
End users | Business users across the enterprise, leaders of business teams | Define business goals and needs, provide feedback on data quality and usability |
Enterprise Data Quality Methods and Best Practices
Data quality frameworks vary in complexity and usefulness for different industries, so choosing a framework to guide you will require a bit of research. Here are three common frameworks:
- Data Quality Assessment Framework: Focuses on systematically evaluating the quality of data in an organization and identifying areas for improvement.
- Data Quality Scorecard: Uses quantitative metrics to track and report data quality over time, often tied to organizational KPIs.
- Data Quality Maturity Model: Helps organizations assess their current state of data quality and set goals for improvement.
No matter what framework you choose, there are three main steps to follow with a couple of best practices within each to ensure consumable data across the enterprise.
Make a Plan
Just like any project, your team should gather stakeholders, understand business needs and define goals for the project. From there, you’ll want to define the data required for each business need to achieve those goals, and document it. Within your documented plan, you should also draw up some definitions for metadata and define roles for stakeholders.
Use the Right Tools
The right software for your data quality management project should provide as many features as you need without too many extras to weigh it down. Look for software with dashboards, alerts and issues logs to help the team stay on top of reporting. The tool should also work closely with data governance software so you can define policies, standards and KPIs. And finally, the tool should integrate easily with the organization’s current software stack to transfer data via API or webhook.
For example, most master data management (MDM) tools can integrate with other systems to integrate poor quality data, enforce data governance policies and send back clean data for use in downstream systems like analytics or business intelligence (BI). Platforms like Profisee also let you measure and track the quality of your data in addition to defining and managing validation and assignment rules.
Implement Processes
Once the plan is documented, the software is in place and the data begins moving, a successful organization will begin training on and reinforcement of the processes the team uses to determine and sustain data quality. Stakeholders must check for compliance with regulations and the company’s data policies and continue to meet to discuss upgrades and future needs for the organization’s data.
Create a Data Strategy to Improve Enterprise Data Quality
If your enterprise data is of poor quality and not fit for consumption, it might be time to create or revisit your organization’s data strategy. Creating a data strategy is often a key responsibility of the (CDO), and, when done right, it can do wonders to improve data quality and make sure it’s ready for use by the people and downstream systems who need it.
To learn more about how to create a data strategy, read our article on creating a data strategy roadmap by my colleague and Profisee CDO Malcolm Hawker. Malcolm recommends taking a minimally viable product (MVP) approach to crafting data strategies, laying it out in a way that is both accessible and actionable.
Creating a Data Strategy Roadmap: Key Phases & Tips
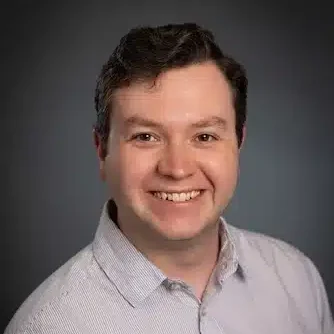
Forrest Brown
Forrest Brown is the Content Marketing Manager at Profisee and has been writing about B2B tech for eight years, spanning software categories like project management, enterprise resource planning (ERP) and now master data management (MDM). When he's not at work, Forrest enjoys playing music, writing and exploring the Atlanta food scene.