Hello CDO Matters Community!
I’m writing this update today from 40,000 feet, somewhere over the western portion of Ontario, Canada as I travel to spend a few days with my family and friends in my hometown of Edmonton, Alberta. I’m knee-deep into my hectic fall travel schedule that includes meetups at Microsoft Technology Centers (MTCs) across the globe, as well as several notable data-centric industry events.
I’ll be talking about the future of data management at these events in addition to how MDM will fit into a data fabric-enabled data ecosystem. Our businesses operate in a world where disruption and digital transformation are the new normal and our ability to scale and adapt the people, processes and technologies supporting our data organizations is more critical than ever.
A year ago, I was a data fabric contrarian. I viewed the idea of a data management ecosystem where data informs its own classification and governance policies as a laudable concept but one that is far too technically complex to execute at scale.
Then along came OpenAI, and in the time it took me to grasp some of the basics of how generative AI works, I was transformed into a data fabric believer. My conviction in the data fabric grew stronger earlier this year with the release of the Microsoft Fabric. I view this as a “V1” release, but it’s still a critical first step in the evolution of this new paradigm.
——————————
What Microsoft is doing in this space is groundbreaking and highly disruptive. At a high level, I view the Microsoft Fabric as a turbo-charged virtualization layer that abstracts the differences between database management systems. In a single query, you can access data from across any number of disparate databases— even across multiple clouds or locations. Microsoft Fabric is currently focused on analytical use cases, but given Microsoft also has a suite of business applications (CRM, ERP, etc.), I think it’s only a matter of time before the fabric paradigm extends into these transactional databases and systems.
What this could ultimately represent is the commoditization of databases, where the differences between various data stores (object-based, file-based, relational, graph, etc.) are all largely abstracted away and become transparent to both users and applications.
If true, we may eventually see a virtual (if not physical) consolidation of the databases supporting both analytics and business applications in the future. Your CRM and your BI tool could conceivably use the exact same virtual dataset. On the backend, the differences between systems could still exist, but on the frontend, those differences would be completely abstracted at an application or user level.
As the cloud has completely abstracted away the complexities of the deployment and management of physical hardware and servers, the fabric will do the same for the physical management of data. Database management will increasingly become commoditized, forcing vendors to extract value at higher levels up the data stack (into areas like data quality and MDM). I suspect that in time there will be Microsoft Fabric and Amazon Fabric and that the differences between the two will become functionally negligible.
In the interim, I believe companies that embrace the fabric will have a significant first-mover advantage – realized largely through: (a) the depth of analytical insights exposed through the activation of metadata that will benefit business stakeholders and (b) increasing levels of automation in data management tasks, especially data governance and quality. We are still very early in this evolution, and the technologies are still largely nascent. But the evolution of the cloud is a perfect analog to what is rapidly unfolding in the world of data.
———————–
In my various (and numerous) conversations with data leaders, it’s clear that artificial intelligence is front of mind for just about everyone. Ever since the launch of ChatGPT in November 2022, there has been a growing focus within the data community on the long list of issues associated with building, managing and governing AI-based or augmented systems.
However, what is also clear is that there is a massive lack of understanding (and an equal level of confusion) within the data community on how these AI technologies work, particularly generative AI. This is problematic, given it’s typical for data science and AI roles to exist within the data function under a CDO – meaning our business customers should naturally expect people within the data world to at least have a working grasp of it.
If somebody in procurement or finance asks a data leader for an overview of how AI works and how it might impact their business, it’s reasonable to assume that data leader should be able to oblige with at least a remedial level of expertise.
Unfortunately, a base level of AI expertise within our data teams is not what I’m seeing, and I think we need to seriously consider having our teams attend some AI bootcamps as quickly as possible. I’m by no means an AI expert, so I am doing my best to try to catch up.
My training regimen for AI includes consuming a ton of AI-centric content on LinkedIn and other channels (from noted AI experts) and reading my friend Bill Schmarzo’s book titled AI and Data Literacy. I discussed this book with Bill in a recent episode of the CDO Matters podcast, which I recommend for anyone trying to become more conversant on all things AI.
If I made only one recommendation to data leaders to improve how others perceive their AI knowledge, it would be to dispense of any suggestions that the existing commercial LLMs (like ChatGPT, Bard, Bing, etc.) can be “changed” or “retrained.” The commercial LLMs have been trained and are highly unlikely to change.
Think of the LLM as a prism or a filter. You pass data into the filter, and other data comes out on the other side of it. You can try to influence how the filter behaves through the information you pass to it (the prompt), but the filter itself does not change — nor does it remember anything from one session to the next.
Other companies are working on developing other models built specifically to support more specific use cases, but building new models is currently a costly and time-consuming exercise. In the future, changing or retraining models will certainly be more commonplace, but for now — at least insofar as the well-known LLMs are concerned — the models do not change.
I hope everyone (at least in the northern half of our planet) had an amazing summer, and I hope to see you at some data event in a city near you soon! Or please join me every month during CDO Matters LIVE, where I lead a discussion with a growing community of thought leaders.
————
Malcolm Hawker
Head of Data Strategy @ Profisee
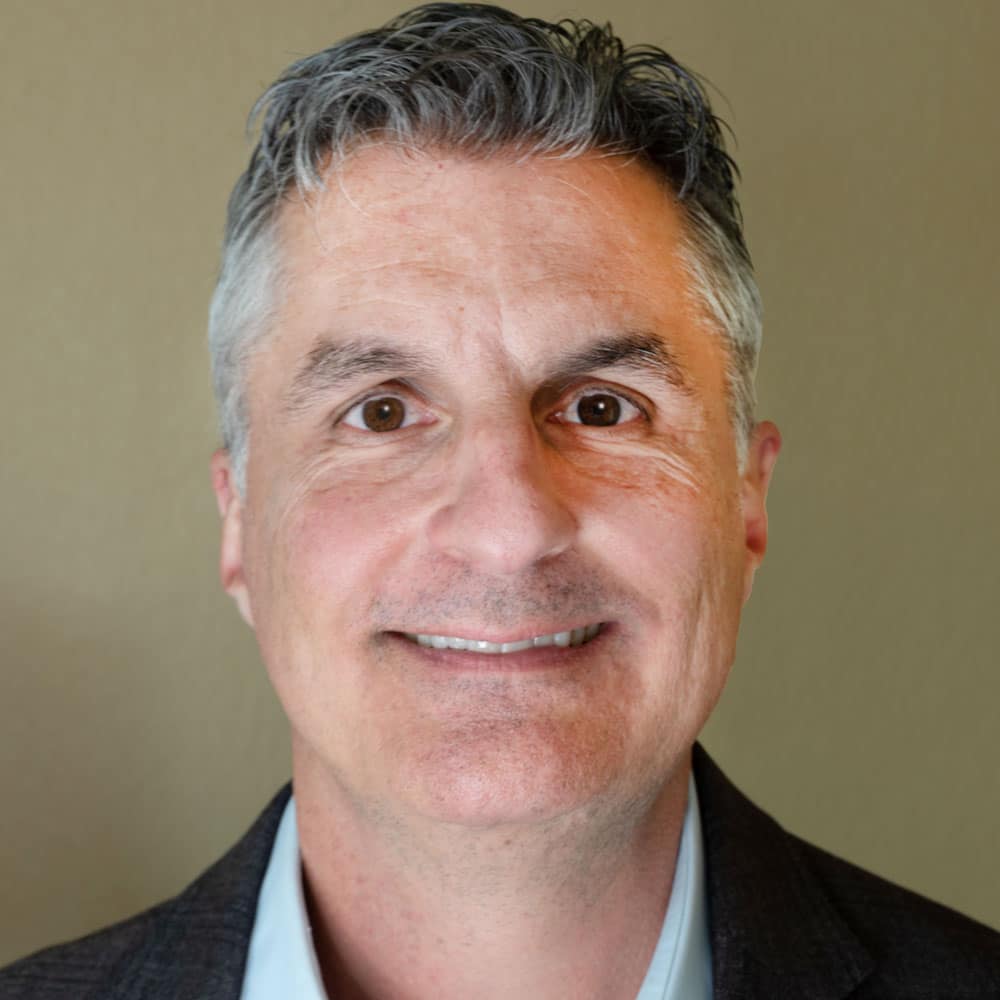
Malcolm Hawker
Malcolm Hawker is a former Gartner analyst and the Head of Data Strategy at Profisee.