CDO Matters LIVE with Malcolm Hawker
Join our monthly event CDO Matters LIVE with Malcolm Hawker to have your burning questions answered by a former Gartner analyst.
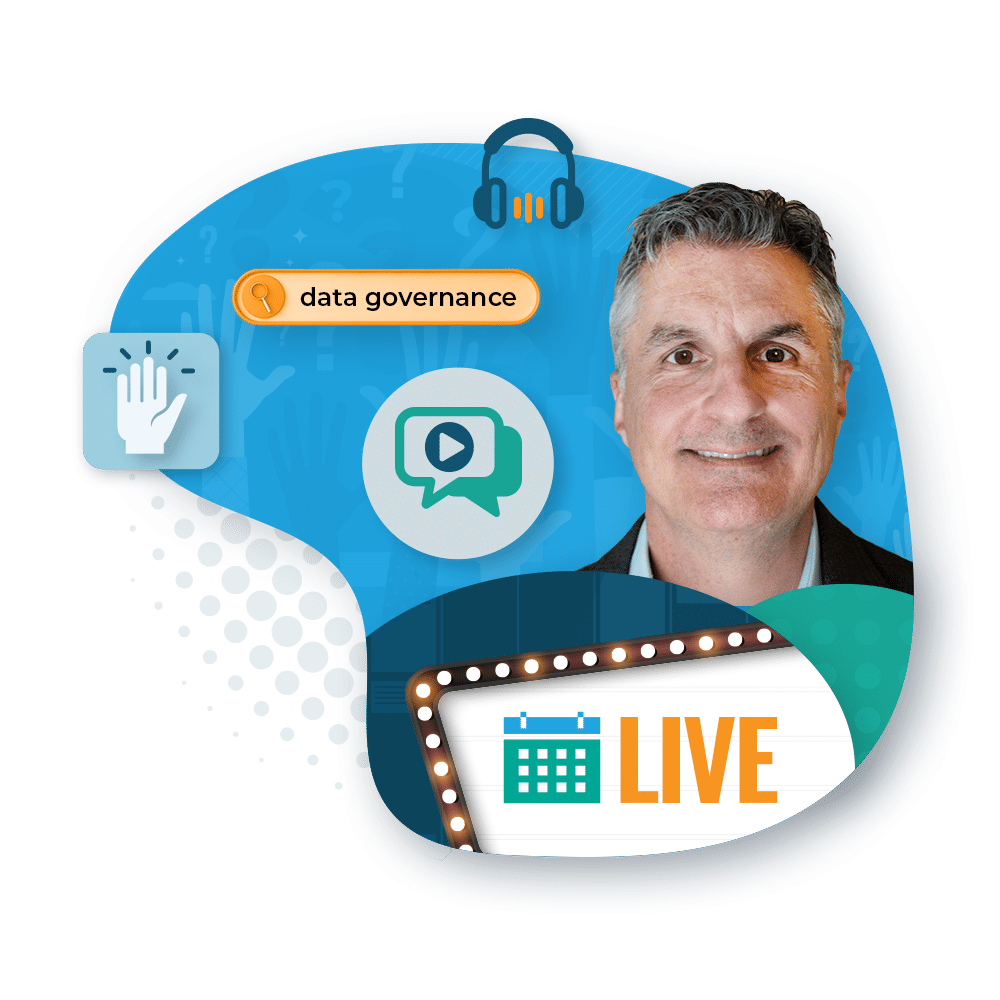
EXPERT ADVICE FOR
YOUR
NEXT DATA PROJECT
When it comes to growing a business, your critical enterprise data is everything. But that doesn’t mean you need a high-priced consultant or analyst to weigh in on your MDM strategy!
What if you could bring your burning data questions to a seasoned professional without the financial burden?
Join us every last Friday of the month at 11 AM ET for CDO Matters LIVE with former Gartner Senior Director Analyst and Profisee Head of Data Strategy Malcolm Hawker.
In each session, Malcolm will answer your top-of-mind inquiries about all things MDM, data governance, data fabrics, business value and more. With thousands of customer consultations under his belt, Malcolm will help you initiate an effective data strategy.
Check out our list of upcoming sessions and register today to start the next step of your digital transformation!
REGISTER BELOW
Good morning, everybody. I should also say good evening or afternoon because we do certainly record these. I’m Malcolm Hawker, the head of data strategy with Proficy Software and your host for the CDO Matters live edition of the podcast. And there he is.
AirPods working?
Yep. AirPods are working.
Yeah. Well, that’s actually better audio quality.
Awesome. Great.
Good stuff. So I was just doing I was just doing my usual, intro. Happy Friday to everybody. We record these on Friday.
Typically, we do these, live versions of CDO matters the last Friday of every month. But because the the holidays are right around the corner, we’re doing it, on on the fifteenth. I’m beyond thrilled, to be joined by Eric Zwiefel, the CDAO of Microsoft for the Americas on today’s episode. Eric, welcome.
Thank you very much for having me. I’m excited to be here.
We’re we’re thrilled that you’re here. We’re gonna have a good conversation over the next, hour.
Eric and I were just talking about, upcoming holidays here in in in the US.
So happy holidays to to everybody. I hope I hope everybody gets to spend some time with family and friends.
What are your big plans? Are you staying are you staying home? Are you traveling?
Yep. Staying home. Just kinda, yeah, being have happy time with the big fam.
Yeah. That’s that’s that’s that that’s awesome. I will be traveling north to my homeland in Canada, Edmonton, Alberta. Hopefully, fingers crossed, the weather holds.
But, apparently, El Nino has been making it rather bearable this year. So so, anyway Yeah. Enough with the frivolities and the weather, Chad. I mean, you can do that with anybody.
Anybody could have be having but but but since I have you here, let’s let’s let’s let’s dive into it. Again, thank you so much, Eric. I think I met you I don’t know where I met you for the first time. Probably CDOIQ two years ago is my guess maybe, but we had a chance to have the dinner.
Where were we? We Boston?
Yeah. Yeah. I think that was Boston. Yep.
Yeah. At at the at the last CDOIQ conference, we had a wonderful dinner in Boston. Had, like, a a a great long chat. I got a chance to know you, which which was fantastic. We we share a lot of similar views. We’ve got a a passion for for data and analytics quite obviously.
Looking forward to picking your brain in a little more detail here today. Welcome to everybody.
Thank you for joining.
Let’s let’s let’s dive into it. Everybody wants to talk about AI, of course.
Yep.
It’s been obviously kind of like the number one topic this this year. I go to a lot of industry events. People are talking about it. One of the things, Eric, I’m I’m hearing a lot of data leaders express concern about the the idea of being let’s just call it AI ready, becoming more AI ready.
In your conversations with your clients, I imagine you’re hearing the same. And if you are, what what do you tell them? What what do you say? How do you get more AI ready?
Oh, great question. For for me, it comes back to the same things that we’ve been talking about for years. Like, you need to govern your data. You need to have good policies. You need to have you know, know who accesses and who can’t access.
MDM is very important. Data quality is very important and so on. You know, we say that data is the fuel that powers AI. Whether you’re training a model or more likely using the rag pattern.
Either way, you need to have, be able to get access to the right data at the right time. And then on top of that is this extra layer of you need to think responsible AI in ways that maybe we haven’t before for many organizations.
And we need to think about how is this going to play out and, work that into our overall governance posture.
So so so you mentioned something there called the rag pattern. Tell tell us a little bit more what that actually means.
Yeah. Absolutely. So I I don’t know the exact. I believe that’s retrieve, augment, and generate is the You’re right. Thank you. And I I I always worry about is it retrieval or retrieve augmentation?
So anyway interchangeably. But yeah.
Yeah. So, essentially, the reg pattern is I don’t need to train a custom large language model.
Instead, the way that I think about it, and this is an overly simplistic view, I use the large language model as almost like a reasoning and language center of the brain.
And when a user asks a question, like, if we take Bing chat, for instance, if I ask what’s a good gift to get a eight year old child, There’s an orchestration layer that will go run a web query, get those results back, and then pass the results and the question back to the LLM to say, you know, look at all of these results and answer this question for Eric on what he should buy his son, and then generates that response. So that’s essentially distilled down that rag pattern is just retrieving data, giving it back to the LLM, and answering the questions that way so you don’t have to have a custom trained model.
So that import that that bit there is is really important, which is the retrieval part, which is getting data to act in essence. What I’m hearing is kind of a fact set, a a known fact set. If if these things are my constraints, if these things are known to be true, then draw a conclusion based off of this. Is that is it do do you agree with what I just said?
Yep. Absolutely. And and we’ve seen instances where that can, reduce you know, we’ve heard a lot about hallucinations with these LOMs.
This can reduce those hallucinations because part of your meta prompt, what you’re instructing the LLM is don’t answer outside of what is in these documents. And so it can start to reduce the hallucinations.
So for those listening, this is where you may have heard you may have heard this concept of something called the vector database Yep. Which is which is can be very effective here. Good friend of mine named, Juan Sequeta is is looking at these graph databases as it to to to help fuel these things as well. So whether it is a snippet of text, whether it’s a URL, whether it’s a vector, whether it’s a graph, What you what you’re basically doing is telling an LLM, giving it constraints, and giving it facts and say and say do something with this. Okay.
Yep. Absolutely.
That’s that’s really, really insightful because one of the ways that I I’m starting to get attracted to the idea I’d love your idea. I’d love to hear your thoughts on this. I’m starting to get attracted to the idea as of the LLM as an operating system.
I mean, I know that’s a really drastic simplification here, but but that’s I’m kinda starting to get attracted to that to the LLMs in the west.
What what do you when you hear that, how do you react?
I, like, absolutely agree with you. I think we’re seeing that with, you know, both OpenAI and then Microsoft, three sixty five Copilot having the idea of add ons. And I think these LLMs become like a new OS, like you’re saying, and these add ons become, like, the new apps.
And it’s, you know, allowing this large LLM to choose which add on to use as it works through that rag pattern. So we’ve seen things like OpenTable announcing add ons and so on.
Yeah. Well, I I think I think what’s interesting here is that you’re you’re kind of, god, I’m showing my age.
I I remember the early days of the Internet, believe it or not.
And I’m seeing a lot of similarities here where there was a time when you had to hand key an IP address into a browser window.
Right? And and and to make sure that you ended up where you wanted to go.
And I and I’m seeing something similar here where it’s like, what we started with is just kind of raw operating system, and and we’re kind of and and it had a chatbot interface, which was great, natural language interface, which was fantastic.
And then data people started to figure out, okay. Well, wait a minute. I’ve got all of this data in is sitting in my world in rows and columns. Right? And and how do I kind of inject that into the flow? Start talking about rag patterns. And now where I see things going, I’d love your perspective on on this.
It is maybe some idea of maybe like a like an app. You use you use the phrase an app. I love that, by the way. But where the app is maybe more like a smart agent that is actually interacting with people, do you like, it looks like Microsoft is is really kind of betting on that. Is is agreed?
Yeah. Absolutely. I think that is the future of kinda technology is moving away from this app to more of this smart assistant.
You may hear Satya talk to, like, the era of copilots or the age of copilots. Yeah. It’s correct. Really, that’s where we see kind of LLMs playing a role right now is as a copilot for us as we are working through.
And a lot of people are excited about that, in terms of I would love a Copilot to help me with my day and and so on. But, you you mentioned the dawn of the Internet, and that’s something I often bring up is that’s that’s where we are at right now. When it comes to AI, it we’re at that stage, the dawn of the Internet. We see this is going to have big implications, And I don’t think it’s hyperbole to say this is going to change every interaction, every user experience that we have.
We just don’t know how yet because we’re at the early stages, the dawn of the Internet.
Right. Well, it’s it’s it’s interesting.
I I I think that is playing out before our our very eyes, and people are starting to answer questions kind of as as as we go.
One of the things that I find I love the Copilot metaphor.
And and one of the the things that not not to be, overly flowery, about Microsoft, although I I can be, because I I think they’re really ahead of the curve when it comes to data infrastructure and and data management in in the cloud, and we’ll talk about that in a in a little bit. But one of the things that I like is that the Copilot metaphor can be applied in so many different applications that we use all day every day.
Yep.
Right? Like, historically, you know, data science, AI, that that happened over there, and it was the really, really smart people that got paid a lot of money to build custom models to that did propensity and did all of this really cool stuff, and don’t get me wrong, and recommend the next song that I heard on my playlist and all that stuff. Really cool. Don’t get me wrong. But it was this kind of this other world. But what I’m seeing now is through these copilots and use of LLMs is that AI anybody can be a data analyst now.
Yeah.
Through and and and I could be using I could just be looking at a Power BI BI dashboard and make that a highly interactive experience, which is, like, incredibly valuable.
So Yeah. Yeah. And I I love the I love the copilot metaphor. So getting back to AI readiness.
Yeah.
What you what you said was, hey. Let’s not forget kind of the basics, the the the blocking and tackling. Right? Let’s not forget security and access. Let’s not forget MDM. Let’s not forget data quality.
I I think that is incredibly relevant when you start talking about these rag patterns. Right? Because if you are telling the LLM, hey. These I know these things to be true, and they’re not.
Yep.
That could be a problem.
Right? Absolutely.
That is the old and tried and true metaphor of of garbage in and and and garbage out. Yep.
Different story if you’re training LLMs, but most people won’t be. I mean, you you you you mentioned that. I mean, like, we’re talking millions and millions and millions to train an an LLM. And and do you see them becoming I I I hate to use the word commoditized, but it seems like they all largely kind of work the same way. Do you agree?
To some extent, yeah. I believe that, we will see that kind of convergence and commoditization of these LLMs.
And I do think there there may be times where some folks or some companies will need to fine tune an LLM, And that won’t be a total train from the beginning, but this is more about how do I help the LLM not memorize facts about my company.
I can have the rag pattern to get the facts. Like you said, this is the fact set.
But more of, can I start to fine tune the LLM so it understands the nomenclature that we use, can help sound like our company, and and just fine tune to be more domain specific in how it interacts with that fact set?
But, yeah, I absolutely think that, for the most part, we will head towards, these LLMs. We’ll be you know, you may fine tune them, but we’re seeing a lot of times where you don’t need to and you’re still getting really good results.
You you raised something there that is very important, which which I don’t think we we talk about enough, which is this distinction between training and fine tuning.
Mhmm.
Right? Train training is, like, go figure out the Internet.
Yep.
Right? Like and and and the one example that I gave because it’s public knowledge because it’s open source is is LAMA.
One of their more simple models is only only seventy billion parameters.
GPD four, I think, at seven seven hundred billion parameters, which is just, like, mind boggling. But Llama had Llama had seventy billion parameters, and they published that it took six thousand GPUs running for twelve weeks straight.
Right? That’s that’s the training process. So you can put you can times that by ten for for for OpenAI and your latest version. Right? And, oh, and by the way, that doing that, the twelve with the six thousand GPUs for twelve weeks straight for for Llama was a two million dollar investment.
So, again, I think you could put a zero on at at the minimum for seven hundred billion parameter model at a minimum.
Yeah. That would be my guess. Yeah.
Then there’s fine tuning, and that’s what you just talked about, which is a which is a different thing, which is, again, loading known facts, generally in a in in the form of a question and an answer into an LLM to help it that to the machine learning part of it to say, okay. This is true and this is true and this is true.
Most people are not gonna be doing the training stuff. They may be doing the fine tuning. I think that’s where do do you see that where things evolving from more kind of domain kind of expertise?
Like, fine tuning to be like a, like, you know, maybe a medical domain or a supply chain domain. Is that kinda how you see fine tuning playing in?
Yes. I absolutely think so. And and, you know, while you were talking, an analogy occurred to me. So this is the first time I’m using it.
So if it doesn’t play out, bear with me. But, you know, training a model is like raising a baby all the way to adulthood. You know? You have to put in a lot of things to get there.
Whereas fine tuning a model is more like, onboarding them into your company. They already know a bunch of stuff, and you’re just teaching them, here’s how we do it here.
Yeah. So I don’t know. Tell me does that analogy play out for you?
I like I like it. I mean, like, onboarding to a a domain or to your company. Right? And then that that’s the intersection. Early on, when when everybody was still kind of learning how these things work, I was hearing from CDOs over and over and over again.
We can’t train, you know, we can’t we our data is in such a bad state that we can’t use it to train an LLM, and you’re never probably gonna need to train an LLM. The fine tuning part, onboarding, I I like that. You could take your internal data.
Well, but here’s the question. Fine tuning generally relies on full like, on text.
Mhmm.
Right? Like like, written text, like verbiage and most information. Well, I guess this isn’t true because there’s a lot of unstructured data floating around out there. But a lot of the good stuff is sitting in rows and columns.
What’s the what’s the what’s the bridge there? Do you see do you see a future where maybe we’re or or kind of by database methodologies change the future where it’s less about rows and columns? Or what’s what’s the bridge to go? Because because all of these the AI is being trained on net on on natural language text. It’s on on on text, and we’re storing in rows and columns.
I I just have this, like, this this synapse fire in my brain. It’s like, oh, wait a minute. Maybe maybe we need to change how the data is being stored. I don’t know.
Does this make any sense? Yeah. Abs absolutely makes sense.
And I I think, two things about that. The first is, again, the fine tuning for me is not about trying to get it to memorize my company facts.
So I don’t need to worry as much about, like, here’s all of the sales data I had since twenty fifteen and make sure that gets fine tuned into FLM. It’s more about the language that we use.
You may fine tune it to say, you know, here are the column names that we use and so on so that it helps the rag pattern be more efficient and get the data faster, and you still rely on the rag pattern to get the up to date facts.
Well, I think this is fine.
We have seen Go ahead.
So my apologies.
I was gonna say, no. No worries. We have seen taking, you know, text based data or excuse me, row and column based data and putting it in, like, a CSV type format, and the LLMs can still do kind of reasoning over that CSV type format. So we can inject the text that way or mark down or however you want to inject it. So there are ways to do it, but, yeah, I just think the the rag pattern is more about, you know, let’s make sure my data estate is in order, and I can get access to those facts quickly. And I may fine tune the model to make it more efficient to get access to those. But I may not need to fine tune it and still be able to do that.
I think this is one of the reasons why maybe graph can be one of the effective ways of of of of implementing these rag patterns because it contains context.
Yeah.
That may not be there in the rows and columns. Right? The context in rows and columns happens in the joins, whereas in a graph, it’s it’s there in the in the relationships between the nodes. Anyway, we’re we’re nerding out.
Let’s let’s pull up between Totally.
Yeah.
Alright. Getting getting this will be probably the last question about AI because I I know people wanna talk. We we just we just had, people asking, hey. Are you gonna talk about the fabric too?
We most certainly are gonna talk about the fabric.
Thank you. Thank you, Marcus, for asking the question. We’ll we’ll we’ll talk about that. And thank you every for everybody for joining. By the way, you can ask questions.
But last question around, AI. There’s a lot of CDOs out there who, by my estimation, by some of the research I’ve done, there’s about I I’m guessing about fifty percent of companies have some sort of data science function. Maybe that’s in a formal kind of CDO organization or maybe with it’s within a line of business.
But there’s a good fifty percent of companies out there that that, to a certain degree, I think you could say we’re caught a little flat footed. Right? Yeah. And and and and are are playing catch up when it comes to to to OpenAI and and Gen l GenAI and and LLMs. What what would you say to those CDOs who who are under pressure from a board of directors to have an AI story to tell? What what would you recommend they do?
Yeah. Absolutely. Reminds me of a discussion I was having with the CDO and asked, you know, what’s the the hardest part for you about kind of the AI this period of AI?
And they said that my board knows it exists.
So yeah. Unlike BI in the past, like, nobody know like, now now they all know?
Oh, it’s They all know it’s there.
They’re expecting a lot from it and maybe not reality from it too. There’s a lot of hype around this.
But I would say for those CDOs that are facing that pressure, there are ways that you can build a kind of, maybe low risk internally facing, AI projects. So you can start to demonstrate progress to your board, show that, you know, we’re experimenting with this. We’re moving forward with this. We’re trying to do that mindfully and responsibly by starting off on, like, a employee q and a chatbot or benefits or something to that effect.
So you could do that with your datasets that, maybe they’re not in the state that you want them to be in yet, but you can still start to show progress to your board.
Meanwhile, looking at those kind of horizon three and horizon two type projects, you can start to lay the foundation and show the board that in order to get there, this is where we wanna go. But we’re going to need to lay some data management foundations or consolidate our data, MDM and so on.
Yeah. I I I love it. So what I heard was build in essence I’m paraphrasing you. Build in essence some form of an AI road map as a part of, I would assume, a broader data strategy.
And if you don’t have Yeah.
You should probably have one.
But build out an AI road map, but start on some of the low hanging fruit. What what I what I heard you say is that there are more than likely, I’m putting words in your mouth, but what I heard you say is that there are more than likely use cases where you can be using AI now.
Agree?
Absolutely. Yes. Yep. Yep. I absolutely agree.
Whether that is a online chat agent, whether that is something else, whether that is a Copilot. I don’t know a lot of software development companies that aren’t using Copilot now. I mean, like, the stuff that GitLab is un GitLab is unbelievable.
But find a way to to leverage that now. And there are, you know, probably use cases that are less is there a way to say this?
Less susceptible, for lack of a better word, to some of the con concerns you may have around AI ethics or hallucinations or anything else. Awesome.
Absolutely. Yep.
Awesome. Where do you okay. I lied. One more AI question.
To the degree that you have magic forward looking glasses, I I like to pull mine out of my back pocket and put on my magic forward looking glasses. Where where do you where do you see things going? Things are moving so fast.
Right? Yeah. I I’m I’ve been blown away by the pace of innovation here. So I know this is a really, really difficult question to answer, but through the lens of kind of some of the classic data management stuff that we that we just talked about, the things you gotta get right, right, to become a little more AI ready, Where do you see things unfolding in the next twelve months?
Do you do you do you do you see I mean, we’ve been talking a lot about data catalogs. Right? Microsoft’s got a solution in Purview. We’ve been talking a lot about governance and ethics.
We’ve been talking about where do you see things kind of unfolding in the next year?
That is a really tough question because you like you said, it’s moving so fast, and we’re finding these nascent capabilities in these LLMs.
And even as we start to add things like vision Oh, wow.
Yeah.
That adds a whole other layer of, like, wow. Where is this heading?
I think you will see more and more when it comes to, like, your core data management capabilities, trying to leverage AI to help out in those areas, maybe in ways that, we haven’t been able to do fully before, but now we can tweak that.
And so you might see things like, data quality tools start to leverage AI more and more to help find out the data quality, find these data quality issues for you. Your data catalog might get more efficient in suggesting, like, here are some business glossary terms that we think you may have missed. Consider adding these.
Or Yeah.
We are inferring from the name of this column that this is the data that is included in that, in the description of that data. So I think we’ll start to see those tools built more and more into those core data management functionality.
Couldn’t couldn’t agree more, you know, to the degree that I that I can put on my forward looking glasses.
You know, I I think you’re absolutely right. I I I can tell you that at Prophecy, we’re certainly looking at doing the exact same thing. How do we integrate some of these capabilities beyond what we’ve already done? And, Rahul, you asked the question about, how does prophecy leverage OpenAI? We we we do in our last release.
We, we released some capabilities to allow for some data quality use cases, and we see more of these unfolding in the future, particularly around administration. Things like data modeling, perhaps, things like in the in the MDM world, entity resolution, suggesting potential matches.
Right? Where in the past, humans were the ones that were making all the decisions about, you know, how do I model a a data? What matters? What’s master data? What’s not master data? What’s data that’s being widely shared versus not? And, frankly, a lot of the answers on the human side were based on assumptions that may not necessarily always be true.
Right. So so I I think data management professionals, if you’re not there already, need to start to warm to the idea that, hey. This AI can actually help us be more AI ready. I know that sounds a a little what what do you mean? Right?
Yeah. The building’s building the building. Yeah. The machine’s building the machines. Absolutely.
It’s a little it’s a little meta, but but but that’s what you I’m paraphrasing you. That’s but that’s kinda what you just said, and I I totally agree. Where where can you leverage some of these tools to accelerate data quality use cases, to accelerate things like understanding, you know, the state of your data ecosystem, what’s mastered, what’s not, how to best match, on and on and on. So love it. It’s great stuff.
And if you do have more questions and if you’re asking them through LinkedIn and we don’t get to them today, I promise I will follow-up, probably later on this afternoon or maybe over the weekend. So if we don’t get to your question, you ask one, I’ll make sure that I do follow-up.
Let’s let’s transition. Let’s talk about one of my favorite topics, the the the I will call it the data fabric. Now I know that Microsoft has a branded version. It’s the Microsoft fabric, and we can draw a distinction here.
I was talking about data fabrics two years ago three years ago when I was a gardener.
Right. And I’ll be honest, Eric. At at the time, I was a little bit of a contrarian because I didn’t think that the technology had really kinda got there yet.
Yeah.
But in the last year and a half, I’ve completely made a a a pivot.
And and now I I see I see the light, and there’s, like, an particularly with the explosion of AI.
How would you explain it take kind of an elevator pitch approach maybe or or or kind of value prop approach. How would you explain it, the let’s just stick with the Microsoft fabric.
How would you explain that to in layman’s terms to somebody? What’s what’s the value there? What is the Microsoft Fabric and why?
Yeah. So for me, Microsoft Fabric starts with a foundation of what we call one lake, which is imagine one drive for your data lakehouse where I can start to have I don’t need to worry about storage accounts or provisioning things. I can just start to have my data put into an area where anyone in my company can access it. And then it standardizes on the, Delta Lake format for storing tabular data.
And we have rewritten our engines like SQL and so on, or the SQL data warehouse to read and write from that, Delta Lake format.
And now we add on top of that the compute, which we’ve made more serverless, where you don’t have to worry about, you know, I want to provision this arc cluster here, and I want to provision this data warehouse here. Instead, I say I have a fabric capacity pool that I can draw from.
And individuals can go in and leverage the different tool sets that they want to. And so you can have someone running Power BI reports against a Delta Lake table at the same time that someone else is running SQL warehousing, at the same time someone else is writing through Spark or streaming it to this table, all happening at the same time against the same dataset than just making it you know, everyone is operating on the same one copy of the data.
And so that’s, for me, the the quick elevator pitch of what it is. So it’s just ease of use. It becomes, just kind of across everything you do. It’s easy to use, and I can get out of the business of managing servers and so on.
Is it correct to say that it’s this kind of hyper virtualization layer? Would you would you call it a virtualization layer, or is that an oversimplification?
That’s a great question.
I don’t think that I would use that term virtualization layer, but I can see where it might be applicable.
But I think it’s more just taking a serverless model, and applying it to this. And then all of these are and the reason I say virtualization because when I think virtualization, to me, at some point, there’s some sort of translation that’s going on between a language to another language or, you know, something like that. Here, I think it’s just more about having the capacity and then running individual and getting people out of that management area.
So more of a SaaS based model than a virtualization based.
Okay.
Fair enough. Because virtualization doesn’t for a specific technology and okay. I I I get it. One of the things that kind of and and, again, I’m gonna nerd out a little bit here.
One of the things that blew me away in one of the demos that I saw early after the release of the the fabric was in essence, and I’m I’m gonna kinda done it dumb it down because I need to do that for me to understand it, was in this case, it was a Microsoft architect, but it could have been anybody that was executing SQL queries against a native graph store. Of course, it wasn’t native graph. It was Parquet data Delta Lake. But but the the the data was graphed.
Its raw form was was graphed that had been but had been brought into the one lake, and I can write any query against it. Right? I could I could and to your point, I could access execute a spark workload against it. I could run a SQL query against it.
I could run GraphQL against it. It doesn’t it doesn’t matter.
It all just works, and you’re and you’re nodding.
And then and then when I think about that, and and I think about it a little bit more, and then I think about it a little bit more, it it seems to me like in many ways that some of the micros what Microsoft has done here is really kind of distilled away, to a certain degree, some of the differences that exist across database management systems.
Yes.
Okay. You agree. Right? So it could be graph. It it it could be Cassandra. It it could be any whatever.
It doesn’t matter. Right? That that if I know basic SQL or even if I know power just I logged into Power BI. Doesn’t matter.
I I can I can access that data in whatever format it’s in?
Yes?
Yes. We we bring it down and essentially put it into that Delta Lake format and then standardize on that. So you abstract away these different proprietary data storage layers, and therefore, it makes the data much more interoperable is what I hear you saying.
Yes. Well, interoperability is is key. Right?
But then when I think about these is, like, we we’ve been holding on to this idea forever and ever and ever that use case defined storage pattern.
Right? Yep. That you you had an analytics use case. And and largely, I’m just gonna split it into two, operational versus analytical. But you had an analytical use case.
And, and that that inferred a storage pattern of blah. Right? Mhmm. Right?
It inferred a data warehouse. Right? Like, some sort of data warehouse. Right?
And and then there was a different you know, I I don’t know what I don’t know yet. Right? I I I I need to handle a lot of data. Well, that was kind of like the data lake.
Then you’ve got operational data stores out there that could even be like like a Salesforce or, like like, object based. You had kind of, like, use case was was was informing management of the data, how it was actually managed. And then I think about the the the Microsoft Fabric, and I was like, okay. All of those differences have kinda been marginalized?
I don’t know if that’s the right word.
And maybe this is a wild quest this is a wild question. DC go ahead.
I was just gonna say, you know, I I think, I I agree with you. And, right now, where Fabric is set up is that analytics layer, but it makes bringing data from your operational databases in a lot easier.
It has things like shortcuts.
So if you have data stored in Amazon s three, you can add a shortcut to your One Lake and then get access to it as if it were there.
And then we recently announced something called mirroring where you will be able to take different database technologies and have, you know, essentially have that data available in fabric also.
Well, that kinda leads me to this the question which is, do you do you see a future? And and this is maybe an oddball question, but where there really isn’t a functional difference or at least an operational difference between data stored for analytical purposes and data stored for operational purposes.
That I’ve been asking myself that question a lot.
Oh, good. I’m not crazy. Okay. No. Not at all.
Not at all. And I’ve yeah. Because when we look at these operational datasets and you they have to have different patterns and availability. And and like you said, you essentially infer a data storage type based on the use case. Is it read heavy? Is it write heavy and so on?
And so I’m not quite sure where we’re headed there.
I have to be completely transparent. I have no knowledge of this on the Microsoft road map, so please don’t read into yeah. Exactly. Please don’t read into Microsoft is saying this. This is just Eric. Eric’s we fold, not Microsoft Eric.
I am hopeful that we will get there where we start to have this kind of if we can get the operational efficiency for our operational data stores and still being able to write and read in more of this lake house format.
I’m hopeful that we’ll get there, and that’ll truly unlock this, the theoretical idea of fabric that my data is always available to everyone in the organization regardless of application or so on.
Although then that gives me my own set of heartburn.
Now it even becomes more important to think about data governance and master data management to data quality because the faster I make my data accessible to everyone, the more I need to make sure I’m doing these checks upfront. Otherwise, we could be making wrong decisions.
Bingo. Yes. So high risk, high reward. You basically just described MDM.
Mhmm. Right? Yeah. Because MDM is this very unique thing that exists at this nexus between analytical workloads and operational workloads.
Yes.
And it actually does both. Like like, unlike a lot of other things in the in the BI and analytics world that just do the reports, MDM sits literally in the DMZ between operational and analytical.
And the data having to be right. Right? Having to be trusted, consistent, accurate, curated. Like, that’s the world of MDM, and MDM people have known this for a long, long time. And we sit right in the middle of those two worlds.
But I’m super excited about a future where maybe on the back end, the data is how it’s always been. Right? Maybe it’s sitting there in files based stores. Maybe it’s sitting in graphs. Who knows? Right?
But that to any anything or anyone consuming it could be a could be a a a an an agent for an LLM, could be an end user, could be an application, could be just a random query from somewhere, but anybody who using it is is experiencing that same experience. Right? And the speeds and the throughput are there to support it. I think that’s one of the one of the concerns. Right? Like, high read.
Right? Yeah. Absolutely.
In in my in my opinion, anytime where it’s where it’s only been compute that was the the gating factor, that always goes away.
Yep.
I think. I I don’t know.
A a kind of a a question more of a more of a statement from from Marcus. He said that the fabric separates data from compute. Do you agree?
I think I think you said that.
Absolutely. And I think, you know, I got a question from a CDO once asking me, you know, why are we talking about data lake house? Like, what why is this a thing?
And, you know, I walked through. You know, we had data warehousing, then we came up with Hadoop. All of these were tightly coupled. Then we came up with this data lake, but we still had our data warehousing. And so the promise of a lake house architecture is this, where I can truly get separation between my storage and compute, have the right compute for the right workload at the right time, but have my data separate. So I would absolutely agree with that. This is just the the next step on the path we started with Hadoop, all those years ago?
Oh, Hadoop. You said the h word.
I I could I could be hypercritical of Hadoop, well, because I spent millions on it and didn’t quite nail down the use case.
Yeah. But that’s I met you. I met you. I met you. That. Yeah.
Yeah. Was wasn’t wasn’t wasn’t alone. The the joke I used to tell and still tell about Hadoop is that for a lot of companies, it was an answer desperately seeking a question.
Yes.
But I do agree that there that this is this is a kind of a natural evolution. Right?
And and I think, like I said, I guess it’s a question. You know? Like, what for a CDO who who’s out there or a data leader who is saying, okay. This is just another shiny object.
Right? It’s another you you just you just said Hadoop. Right? This is just another Hadoop, and that kinda came and went, and it was a flash in the pan.
This is just what what would you say to to that person if they said that about the fabric?
That I, a, start by saying, like, I get it. A lot of people saw these fads. Like, I get your skepticism there.
I think the difference here is that the underlying technology, we’re not you know, this idea of separating the storage compute and then choosing the right compute is different than we had with Hadoop, where we were tightly coupled. We had to have things stored on HDFS. And if you needed more data, you had to grow your cluster, where, really, we can just start to have all of our data accessible in the one lake regardless of where it’s sitting.
We can get the copies into the one lake or, you know, mirrors or shortcuts or whatever it is.
And then we are not constrained by the technology on top of that. So, like, we saw Spark coming in on top of Hadoop clusters and then gradually, you know, moving more towards the where Spark is today, I think with Microsoft Fabric, we will have the ability to add new workloads in this same experience going forward.
Right. Okay. So not just another shiny object. And and I would actually Totally. I would actually I would I would second that. I think in many ways for for a lot of people, the fab the fabric has been has become a bit of a synonym for a data mesh, and and and I would I I would say that the no.
Abs absolutely not. Separate conversation to be had about mesh versus fabric, but these are two very, very different architectural paradigms here. A a a data mesh would call one lake an anti pattern.
It it would actually call it that. I don’t I don’t tend to agree.
Hub architectures work for a reason.
Yep.
Just ask United Airlines or Delta Airlines. I mean, you know, ask anybody who does networking for a living. Hub architectures exist for a for a reason.
There may be promise in the road down the road and peer to peer, but I don’t think we’re we’re quite there yet. So mesh, complete decentralization.
Fabric, I would not I would not argue was a centralized management pattern, but at the but at the same time, it it doesn’t require you to blow up your or decommission, I think, is maybe a better word instead of decommission your your your data lake Yeah.
In your warehouse.
So these are two very different things.
Yeah. The the one thing I would say with Microsoft Fabric for those data mesh enthusiasts, we do give the option of, you know, defining data domains. So you can still have kind of that federated governance and access and all of that kind of built in, but you’re using a consistent set of tools and architecture underneath. So it can, you know, absolutely still help look at your business in that mesh type way, but potentially, you know, make the management of it take away some of the overhead that you might need for a full mesh implementation.
Right.
I’m not saying that it is mesh or isn’t you know, I’m just saying that when I look at data architectures, all of these patterns, personally, I try not to be a purist on any of them. Let’s find out what works for my company, for my use case, and use that.
And if the pure implementation of mesh is what is needed for a particular use case, then absolutely. Go go build that implementation.
But I think for the majority of use cases, that’s not a requirement is what I’ve seen.
Well and kind of your your non purist approach, like, use whatever works.
Love it.
I think, actually, that’s the way most people think. And even the people, I would argue, that started, let’s say, twenty twenty two as completely having consumed all the mesh Kool Aid, are now to the point of being where you are, which is, hey. This idea of domain centricity, that that seems to work. Right? That makes sense. We fifteen years ago, we called it a data mart, separate issue.
Yep.
But this idea of domain centricity, I I like that. Right? I like giving I like giving people in sales and marketing a little more control over their lead data, for example. Yeah.
So I like the idea of of domain centricity. I like the idea of having governance accountability. You could call it a product owner if you want, but I like I like those things. Then there’s these other things over here, which is like, oh, wait a minute.
Hold on. Federated computational governance. I don’t even know what that means. Sounds extremely complicated and not that practical.
Maybe we should let that one go. Yes. So I think your pragmatism is actually reflected in the pragmatism that I’m seeing in the market, which is this is a good segue away from the fabric into more just kinda general trends that we’re that we’re seeing. What I’m seeing is that people the only thing that’s left of the mesh is what you just described, domain centricity.
And and the domain centric focus, a lot of the other things have just kind of gone away because they’re really, really hard to implement.
What are some of the other things over the last year, you know, as we come to the end of twenty twenty three?
In your conversations with CDOs and others around the globe, what are some of the key things that you’ll take away from twenty twenty three other than AI? Because we already talked about that.
I was gonna say that that’s, like, ninety conference without, you know, most of the agenda being about AI. But I think that’s fine. I think that’s where we’re at.
But I think that’s fine. I think that’s where we’re at.
I do think that, you know, one of the big things that I’m seeing when it comes to talking to CDOs is we still struggle in the data world with being able to articulate our business value and being able to reflect back to the business. Here is why you need to invest in your data.
You know, I I frequently try to get people to separate the idea of data from technology.
I think it was, Tim Berners Lee who said data is, I can’t remember how you said it, so I’ll paraphrase it. But data is the precious thing that will live long after the technology has gone on to something else.
And so we have these datasets that last for generations in different technologies.
And so I think the CDO role is about seeing data for that. It’s its own thing, and the technology is just where we store it.
And so I think we’re seeing a lot of that.
Shelley, I see systems come and go, but data is forever. One hundred one hundred percent agree with you.
And in the modern corporation, I feel like data is the ultimate communication vehicle. That’s how our sales gets to our manufacturing, gets to our shipping, and so on. It’s through this data.
And so for me, twenty twenty three is mostly about AI. I’m talking about AI and how do I implement that.
But continuing to struggle with the foundations, that and figuring out how can I show value to my organization outside of AI and get the organization to invest? I don’t know if you’ve seen that also, Malcolm.
Absolutely. I mean, I was I was given the the unique honor of of getting to travel the world this year, making stops in several, MTCs, Microsoft technology centers, meeting with CDOs and data leaders around the country. And, yes, two things that jump out to me, what you just said.
One, foundations.
Right?
Blown away by how many companies.
And and and and I guess I shouldn’t be, but but but I was. Whether I was talking with people at the Gartner data and analytics center, whether I was talking to people at at MTC events, doesn’t matter.
I was blown away by the number of companies that are like, hey. We’re just beginning our journey.
Right?
Yep.
Like, our or whether that was an MDM journey, data quality journey, for many, it was a data and analytics journey. Right?
Like, we’re Absolutely.
And I was like, wow. Like, hey. That’s amazing. I’m glad you’re here. Welcome. There’s resources. We I’m here to help.
But that but for those folks, it was, okay, foundations. Right? How do I where do I start? What comes first? Governance or data management? By the way, that’s that’s that that’s been my number one LinkedIn post this year, has been this I I asked the question, what comes first, data governance or data management?
That was my number one. But but that speaks to exactly what you just said, which is foundations. Right? Like, how do we do this stuff?
How do we get a governance program off the ground? How do we manage access and security? Like, how do we how do we do like, what’s that? Do I need a data catalog?
If I implement MDM, do I need a data catalog? And, like, all these questions. So so that was certainly some something that I heard and, you know, thrilling to see that a lot of people are are doing this. And, frankly, when, when I was in London, at at a at a Gartner event, a lot of those exact same people were saying, hey.
We’re just starting our analytics journey, and we are doubling down on Microsoft.
I heard it over and over and over and over again, which which is which is great. But to the value question, why why do you agreed.
It’s it’s a it’s a it’s a constant thing. It it’s funny.
You you you noted what what Shelley said about, you know, systems coming and going, but data stays forever.
Data people kind of actually use that as as as one of the reasons why data doesn’t have value because it’s not scarce.
Yeah.
Right? Right?
What do you think, Eric? Is is why is this such a thing? Because I’ve been in this space a long time, and it’s still a thing. Right. Why is that that that the the the value problem why is that so pernicious?
Yeah.
You know, when I think about it, it really comes down to you know, when you compare to other parts of the organization you’re talking in front of the CEO, it might be easy to define like, hey. My sales team drove x million in revenue, or my operations team delivered, you know, x widgets in q three.
And for the data team, if you say, you know, we created x data products, tying it back to how that really impacted the business can be a little bit harder to make that leap because a lot of the things that we’re doing, especially around data governance and data cataloging and so on, The impact and the value may not be intangible, like, here is how we moved revenue forward. It may be more in things like, hey. When you ask a question, we can answer it a lot faster than we could before, and we allow the company to be more agile. And so trying to quantify that, can be challenging.
But that’s one of the reasons that I’ve been, you know, excited about looking at data through you know, we have been started talking about data products.
And I’m still I asked a bunch of CDOs at the CDOIQ, like, okay. What what is a data product if you had to define it? Because I think we’re all operating under different definitions.
And to me, what I heard boiled down to essentially, let’s take a data management or data life cycle management approach to data.
And part of that will be we don’t just throw a report and forget about it. We retire things when they’re done. But I’m hopeful that we’ll be able to then start leveraging value if we take that approach and kind of defining it better. Here’s how many people are accessing this data product. You know, it’s used elsewhere, and we can start to then demonstrate to our board and others that our ability to govern and, access and have quality data helps us move faster and helps us meet our business objectives.
And I I often say that, you know, at the end of the day, our goal as CDOs and as data people should not be a great data strategy.
Our goal should be a great business strategy that’s infused with data everywhere and that we are helping to unlock that business strategy as a result.
I love it. I love it. So you talked about data products, and I’m glad you did, because to me, that that’s one of my key takeaways for for twenty twenty three for sure because I heard a lot of the same feedback as well over the year.
You you talked about I’m paraphrasing you again, but you you teased the idea that maybe maybe this focus on products could be the bridge back to the world of value realization or at least value quantification.
Yeah.
Because if I think about it, if I had to sell a product and the sales the sales of that product were what depended on whether I had food on the table or not, I would probably be really, really focused on customer success.
Absolutely. Yes.
Okay. We on on on this, we agree. I’ve been having a lot of posts on LinkedIn recently on this very topic. It’s it’s a sticky issue for a lot of data folks.
When you talk about data data products, there’s a lot of kind of hand wringing online about how do you actually define it.
Absolutely.
How do you well, how do you define it?
Yeah. Like I said, so it was interesting when we asked these, when I asked these CDOs, we had, like, not a debate, but there was an, you know, questioning each other. What kind of report be a data product, or is it just a table or, you know, getting down into the nuts and bolts?
And for me, again, my my definition is more of just taking a product management approach to data.
And if that’s at the table level, at the report level, at the Excel spreadsheet level, like, I don’t really care what level it goes to. But it’s more of understanding that this product, what we’ve put together, will have a beginning, a middle, and an end.
The end is, you know, often really important that we need to know when it has reached end of life cycle and decommission it so we don’t have a prior organization that I was at in our data warehouse. We had four different date dimension tables.
And I to this day, I don’t know what the difference was.
I just know that I had to use this one or it was Roman and Greek.
Right? I mean, that Totally. Right? Like or yeah.
Yeah. And so my my guess is that it was created, and then it didn’t get deprecated, and it was just kinda floating out there forever.
That’s that’s, but that’s oh, that’s awesome. Four different dimensions per day.
You you touched on something.
Well, you I’m I’m I’m I’m kind of moving forward. I’m I’m fast forwarding the tape here. But you Yeah. But one of the things you also just inferred with product management and product life cycle management, is sunsetting products. Right? That’s basically I’m I’m paraphrasing you.
Yep.
But that starts to veer into as well maybe even the idea of sustainability.
I know that sustainability is near and dear to Microsoft’s heart. They have a goal of of of being, net net zero.
I I forget what year Sontra said. I wanna say it’s, like, super aggressive, like, twenty twenty five. I won’t hold you I won’t hold you, but it’s Yeah. It’s it’s very aggressive.
Yeah.
So it’s it’s near and dear to Microsoft. It’s near and dear to everybody. But what I what I heard you say is that that taking more of a product management approach could even help address that because we got a data hoarding problem.
Yep.
I mean, if you can say, hey. This data is not being used. It’s just collecting digital dust. It’s never been used.
It won’t be used. A product manager would look at that and say, hey. Listen. Why are we paying storage and compute for this thing that is just collecting dust?
Nobody’s getting any value from it. Maybe we should decommission this or archive this or go put it on on tape. Do people still put data on tape?
I just asked that. Yeah. I just asked that recently. I can’t remember who it was, but they sell tape.
And I was like, do do people still use this? Oh, yeah. Absolutely. It’s used all over.
I’m like, oh, got it. Yeah.
Because, yeah, it was like For certain use cases, I think, like, if it if if it can never ever die, like, truly, right, but, like, you need to keep it forever and you but you don’t wanna have it, like, sitting in your days of tape.
I mean, that’s what I used to do. I mean, when when I was running an IT function, this was a while ago. I mean, it was like throw it on the tape and stick it in the in the store’s locker.
Okay. Still being used.
We’re down to our last thirty minutes. I want to say thank you for everybody for attending. Thank you for the dialogue that we’ve got going here on our Goldcast platform. Melvin, you asked a really deep and insightful question. If you’re still on the line and if you’re still listening, I will follow-up offline, regarding to kind of the garbage in, garbage out. Does that really apply in the world of highly probabilistic AI driven things?
I’ll take that one offline. I’ve got some specific thoughts there. Great question.
Yeah.
Great questions from others as well. Thank you, Jennifer. Thank you, Marcus. Thank you, others, for interacting in the chat.
In our last two to three minutes, what’s the best part of your job, Eric? Doing what you do, working where you work?
Yeah. The best part of my job is is I love working with different companies and and seeing the different problems that they have.
Some that are unique to them, some that are endemic across the industry.
But I I I just love it, to be able to see all of these different how we’re all working together. And the other part that I love about just the data community is that when we go to these events or conferences, even competitors in the data community will share, like, hey. This is we ran into this problem. How’d you guys solve it? Like, it’s very much you know, we’re trying to help each other and build each other up. So those would be the two favorite parts of my job is just being able to see all these differences in different companies and work with them and help them, and then just being a part of the data community.
I’m I’m with you on that. And it’s thanks to the data community that allowed me to meet you, and I’m grateful for that. We actually really do have a community. I completely agree whether that community is expressed through LinkedIn, whether that is conferences, whether it’s it’s I I really do feel it. It’s actually quite palpable.
And and I’m grateful for that. Just a few logistic things before we end up. Please keep in mind if you enjoyed this, we do this the last Friday of every month. We do a CDM Matters live. Sometimes it’s just me. Sometimes it’s me with engaging guests like Eric.
Don’t forget to check out our content on YouTube, on prophecy dot com. We do the CDM Matters live. It’s posted to all the podcast providers of your choice. You name it. We’re out there as well. We’ve got great guests. We talk to CDOs, data leaders, business leaders about their biggest challenges.
Eric, thank you so much. It’s it’s been awesome to to talk with you. Really appreciate you taking an hour out of your busy day. Happy holidays.
Yeah. Likewise. Thanks for having me. I enjoyed the chat. And, anytime you wanna call and nerd out on AI and data management, you know I’m up for it.
Be careful what you ask for. Thanks everybody for tuning in. We will see any everybody again sometime very soon on the next video Matters live. Thanks all. Bye for now.
Thank you so much. Bye bye.