With every day that passes, I am increasingly amazed by the speed at which new adaptations and innovations are happening in the AI space. Last month, I was using AI to help me craft a witty joke about April Fool’s Day, and this month companies across a myriad of industries are having legitimate conversations about using large language models, or LLMs, to replace large sections of their workforces.
This is both incredibly exciting — and absolutely, positively terrifying.
I’m old enough to have been around during the explosion of the consumer internet the explosion of the consumer internet — in 1995, I was for working for AOL for those who even remember it — and the rate of change today makes 1995 seem glacial by comparison. It took the Internet 25 years to reach 100 million users, and it took cell phones 10 years to hit 100 million users. ChatGPT did it in 60 days.
While it’s far too early to have any reliable visibility on the level of impacts that AI will have, it’s abundantly clear they will be disruptive and transformational. This includes the world of data and analytics.
AI and Data Fabrics
Earlier in the month, I made a post on LinkedIn stating that I believe the data fabric will be how AI is operationalized, at scale, for most companies. This post was a summary of a recent episode of CDO Matters focused on the data fabric. If ChatGPT can use the entire internet prior to 2021 as a training data set, then it’s easy to conceive that all data from a given company could be used in the same way.
With LLMs built on models designed to focus on data management use cases, it’s conceivable that our data could start to help inform humans on the best way to govern and use it — which is exactly what data fabrics are. They are sell-learning, self-informing data architectures that leverage advanced technologies like graph and AI to allow for data to inform its own classification and use.
Two months ago, I said that data fabrics were 7-10 years from maturity. But thanks to recent advances in AI, now I think that number is closer to 2-3.
The use of AI to support complex architectures like the data mesh or the data fabric will require a LOT of planning and they won’t be implemented overnight. However, other AI-driven advances will need to be figured out much, much sooner — and the impacts on CDOs and data leaders are material.
AI and LLMs for Data Quality
For an example of a near-term, immediate impact on data leaders from AI, then look no further than the use of AI for data stewardship, quality and verification. I attended a demo this week of how OpenAI could be used to support data stewardship use cases — and while it’s easy for a CDO to visualize how a data steward could use a chat interface to ask an LLM questions about the quality of data, the process is far more complicated — and more problematic — than you might think.
Historically, a data governance committee would define rules for data quality. These rules would be based on attributes of data, like completeness, timeliness, uniqueness and accuracy. The rules would be configured, or coded, into intermediary systems — like DQ tools, ETL processes or master data management (MDM) platforms.
Sometimes the rules would be coded by engineers, and sometimes the rules were more black-box, where you would rely on the algorithms defined by a third party — often data management software providers, or even third-party data providers. The configuration of the system would be iteratively “tuned” to ensure the outputs of the system are aligned with the policies, where typically a governance committee would be heavily involved in optimizing the system to ensure it aligned with the rules.
Once the rules were set, the underlying logic of the rule would not. You could trust it to be consistently applied over and over.
In a world where AI is managing the execution of a data quality rule, things are a bit different.
First, when it comes to coding or configuring a rule into a system, in the case of MDM it’s conceivable that a data steward could largely assume a role historically held by a software engineer. Instead of using code to optimize the behavior of an algorithm, they would be using a series of prompts.
To avoid AI hallucinations, the more specific and granular the prompt, the more accurate the output. This shift of data steward from somebody managing exceptions to rules to configuring the rules is significant.
The next major issue regarding the use of AI to manage data quality rules is the behavior of the AI itself. Historically, once defined, the behavior or a rule would not change. However, if the training data used to manage the rule changes, it’s conceivable its behavior (and therefore its output), would also change.
Meaning — what fails a data quality rule today, may not fail a data quality rule tomorrow — even though that data itself has not changed. Historically, this would only happen if we change the rule, but unless we can configure some idea of continuity into the AI models used to enforce a data quality rule, the inconsistency of its behavior is problematic.
Where Do We Go from Here?
The rate of change AI, and the levels of impact on our data management processes from those changes, are all making my head spin. While every data management software vendor races to implement AI into their solutions for fear of being left behind, the implications of AI in the world of data management are a LONG, long way from being fully understood.
And we haven’t even started talking in earnest about some of the bigger picture issues here around AI-enabled data governance or data strategy. AI for data verification is one thing, but using AI to write data governance policies is completely another.
Meanwhile, the metaphoric horses are being let out of the AI barn. It feels more and more like we’re racing after the wild horses, with only a slim chance that we’ll ever catch up. If we do, it will be the most exciting ride of our lives. If we don’t, we’ll all be trampled.
I hope you had a wonderful May everyone — and my many thanks for your continued involvement in our growing community of data practitioners. It’s my mission to help data leaders excel in their positions and drive real value for their businesses, and it’s my sincere hope you’re benefiting from this content.
Have Questions of Your Own? Ask an Expert at CDO Matters LIVE with Malcolm Hawker
When it comes to growing a business, your critical enterprise data is everything. But that doesn’t mean you need a high-priced consultant or analyst to weigh in on your MDM strategy!
Join us every month for CDO Matters LIVE, where you can bring your burning data questions to the table where Malcolm will answer your top-of-mind inquiries about all things MDM, data governance, data fabrics, business value and more.
Register here for an upcoming session and learn more about our monthly event!
Malcolm Hawker
Head of Data Strategy @ Profisee
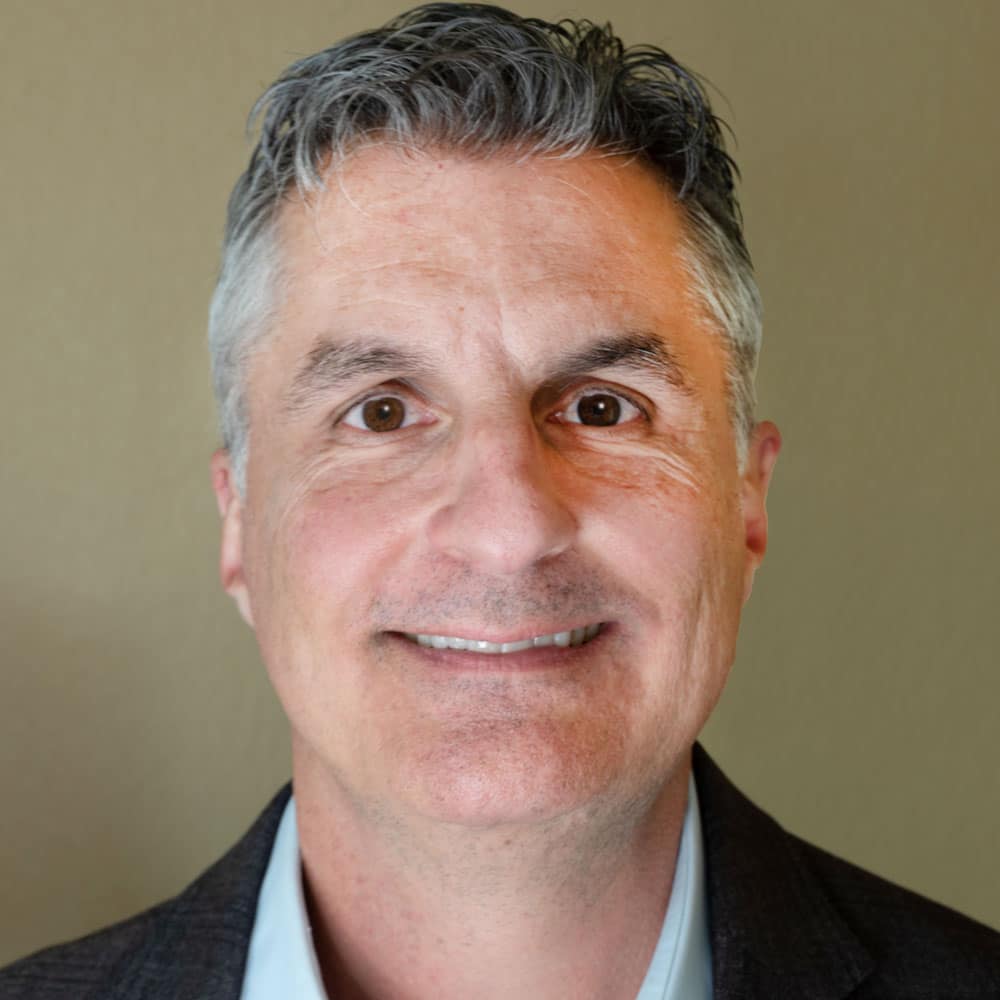
Malcolm Hawker
Malcolm Hawker is a former Gartner analyst and the Head of Data Strategy at Profisee.