In today’s data driven world, high quality data is key to the continuous success of an organization and vital for its transnational and operational processes. Yet according to Dun and Bradstreet, more than 50% of companies are still facing data quality challenges.
As more organizations are increasing their adoption of digital transformation initiatives, the demand for data quality requirements and data tools is among the top three priorities for business leaders.
Poor quality data has a direct effect on a company’s bottom line with lost sales, missed opportunities, incorrect insights, non-compliance, and poor customer satisfaction.
Data reliability and fitness may be affected by the way data is entered, handled and maintained. Identifying common data problems and analyzing the related root causes are often the first steps in any data quality initiative.
Here are five ways to improve data quality:
Have a DQM Strategy – this is necessary to define the mission of your initiative in line with the overall company strategy. It should outline what systems are included, who will be involved and how will the strategy be executed, monitored and measured. This does not need to be complicated; a simple one-page plan will be enough to provide clarity and guidance.
Focus on Master Data Management – This is where the ROI for DQM and company-wide digital transformations can be realized. By focusing on the completeness, reliability, uniqueness, consistency, accuracy and timeliness of master data such as customer, product, supplier and partner data across all downstream systems, organizations can reap real benefits of optimized customer experience, enhanced analytics and informed business decisions.
MDM projects can start small and gradually grow as the organization data maturity model evolves. MDM brings a systematic approach to data integration that ensures the consistent use and reuse of data.
Implement Data Governance – together with MDM, data governance ensures that business users make decisions based on high quality data. Well documented policies and procedures together with clearly defined roles and accountabilities and properly trained data stewards, ensure that the continuous integrity of master data is always supported and protected. To a large degree, the success of a data governance strategy depends on the success of its associated data stewardship efforts.
Data governance also ensures that both data security and compliance requirements are addressed and maintained.
Regular Data Sanity Checks – ensure that periodic data monitoring and cleansing are carried out where data is reviewed, updated, standardized, deduplicated to create a single data view. These should be outlined and included in your DQM strategy.
Issue Management and Resolution – by implementing frameworks that provide the timely management and escalation of issues through to resolution. Example:
- A regular issue log that is updated and maintained on a regular basis
- Creating a knowledge base of common issues, with route cause analysis and relevant fixes.
In addition to the above, continuous improvement initiatives can be defined and executed to maintain data agility in line with business and organizational development.
As the analytical landscape of organizations are becoming more complex, due to the increase in the volume of data collected from various sources and the continued implementation of new solutions, the need for consistent reliable data is becoming a top priority for organizations so they can quickly respond to changes in market dynamics.
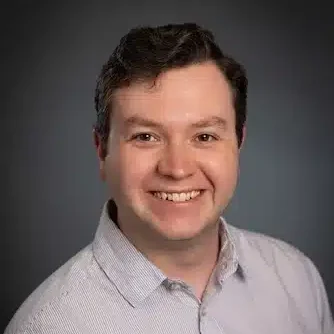
Forrest Brown
Forrest Brown is the Content Marketing Manager at Profisee and has been writing about B2B tech for eight years, spanning software categories like project management, enterprise resource planning (ERP) and now master data management (MDM). When he's not at work, Forrest enjoys playing music, writing and exploring the Atlanta food scene.