As an avid consumer of IT and business news, you surely have heard the adage “garbage in garbage out.” The concept is simple: the quality and relevant use of any analysis, analytics or business output is a direct function of the quality of the input data feeding the model.
This oft-recited phrase has been around for a while, but it still holds an important truth today. Whether pursuing a digital transformation or taking advantage of technologies like artificial intelligence (AI), machine learning (ML) or the Internet of Things (IoT), organizations need a strong foundation of trusted data to achieve their business goals.
After covering the data governance and master data management (MDM) markets for several years — as part of helping clients build business cases for governance MDM — I took a step back to reflect on why the state of data in most organizations is as dismal as it is.
Read on to see why there is such a challenge in demonstrating the value of trusted data available across mission-critical operations and analytics in an enterprise — and how you can kick your own dirty data to the curb.
What is Garbage In, Garbage Out?
The concept of “garbage in, garbage out” (GIGO) is what it sounds like: If you feed your model garbage, you will get garbage. Put another way, in data and analytics and in life, “you are what you eat.”
“Garbage” could be data that is inaccurate, incomplete, inconsistent or otherwise not up to par with any of the 65 dimensions of data quality, as defined by the Data Management Association (DAMA).
GIGO becomes a real problem in the context of digital transformation, especially as new technologies like generative AI raise the stakes for what can go wrong when outputs are bad. Think:
- Missing sales opportunities due to under- or overestimating demand for a product or service
- Wasting budget on ineffective marketing campaigns
- Failing to comply with privacy regulations by sharing sensitive information with the wrong people
- Missing revenue targets due to misreporting
- Receiving flat-out false information
Even without something as sophisticated as an AI model, garbage outputs can still cause headaches for aspiring data-driven organizations that rely on data and analytics to inform impactful decisions. More on this in a bit when we consider some real-world examples.
History of Garbage In, Garbage Out
Though the first recorded use of the phrase “garbage in, garbage out” dates all the way back to 1957, it was popularized in the early days of computing and recited to the point of cliché with the rise of data warehouses in the 1990s.
Garbage In: Data Silos and Technical Debt
The succinct version of this story starts with the fact that most of us in any business environment have historically been compensated to optimize business processes in silos, whether we realized it at the time or not.
For those of us fortunate enough to become involved with information technology during the last half of the 20th century, we were allowed and encouraged to automate these silos.
This resulted in tremendous productivity gains when all those processes were aggregated, and truly little attention was paid to the technical debt that was created when each of those new business application systems took its own set of data with it.
ERP and other application suites made significant strides towards at least co-locating logically similar data within their databases, but little if any capabilities were built in to enforce broad-based data quality and semantics across the supported business processes.
This resulted in a different set of “logical” silos within a single physical data store. Concurrently, specialized applications such as CRM arose, again increasing productivity in isolation but again complicating the issue of trusted and, therefore, reusable data.
Garbage Out: Data Warehouses, Data Analysts and Data Quality
The technical debt of poor data quality was first widely exposed by the advent of data warehouses and data marts in the 1990s and the first attempts to consolidate and reconcile source data from these various data silos for use in even basic reporting and analytics.
Nascent data analysts discovered that the data in these systems did not conform to the ostensible rules within each system. Worse, the meanings of seemingly similar data attributes across these systems bore little resemblance to each other.
Today, as enterprises now pursue strategic initiatives like digital transformation, they have increasingly discovered that the status quo of largely untrusted data can no longer be tolerated if they are to implement futuristic capabilities and automation of their business processes and analytics.
The technical debt of poor-quality, mission-critical data must now be paid. Indeed, it is no surprise that 60% of organizations reported under-investing in their enterprise-wide data strategy, preventing valuable data from being broadly used, according to a survey by Harvard Business Review Analytic Services.
Examples of Garbage Input
As mentioned above, garbage input really boils down to data that fails to meet any of DAMA’s 65 dimensions of data quality. An article from Dataversity breaks those down into six core dimensions.
Here are some examples for each of those dimensions:
- Accuracy: Does the data match up with reality? Can you verify its accuracy by cross-referencing the data with a source you know to be true? For instance, if your organization has an e-commerce business vertical, you might use a service to verify shipping addresses, like Bing Maps Locations API.
- Completeness: Is all the information there? For example, a complete US address should include a street number, city, state and ZIP code. A data point missing any one of those pieces would be considered incomplete.
- Consistency: Is the data the same across every location where it’s stored? For instance, if you compared a customer’s shipping address data from your CRM against the same customer’s shipping address from your ERP, you should get a perfect match. This goes not only for having the correct address, but also for the address being formatted consistently. For example, if an address is formatted as “Louisiana” in your CRM, “LA” in your ERP, and “La.” in your HRIS, the data would not be consistent even though each system uses the correct state in the address data.
- Timeliness: Is the data received in a timely manner? That is, when it’s required, predicted or expected? To continue using the address example, you would need to receive the shipping address when a customer places a new order so it can be sent to your order fulfillment system.
- Validity: Does the data adhere to pre-defined business rules? Let’s say your organization’s order fulfillment system requires nine-digit ZIP codes as opposed to the standard five-digit ones most people use. If an order was placed using only a five-digit ZIP code, the data would be invalid.
- Uniqueness: Is the data only stored once in a single location? We’ve already discussed the problem of data silos, but here I’m referring to duplicate data in a single location. For example, if a customer has two shipping addresses on file — one for home and one for work — you would want both addresses to be linked to one customer record in a database instead of creating two customer records with different addresses for the same person.
This is by no means an exhaustive list. It’s up to data stewards to decide which dimensions are most important to them to define what makes for good or poor-quality data at their organization.
Where Does Garbage Come From?
Data silos are a problem for data quality, but it would be a mistake to identify them as the root cause of the problem. So-called garbage can come from a variety of sources.
Here are some examples I’ve seen over the years:
- Mergers and acquisitions, or any time data is imported from an external source that doesn’t conform to existing corporate standards
- Data entry errors (human error)
- Conflicting or different rules for data validation or verification within a system or across multiple systems
- Lack of integration across systems within a complex business process
- Absence of a data governance organization or shared governance policies in an organization
How Does Master Data Management (MDM) Solve Garbage In, Garbage Out?
Thankfully, there is a solution to the problem of GIGO. Master data management (MDM) is all about creating data you can trust by remediating instances of low-quality data making its way into a business or analytical process, and it’s excellent at breaking down data silos.
Here are some specific ways MDM can help you kick your dirty data to the curb:
- Data quality: Think back again to the six core dimensions of data quality we talked about earlier. A master data management solution can help you clean up your data so that it meets those standards.
- Data governance: An MDM solution is not the same as a data governance solution, but the two go hand-in-hand. If data governance is about creating policies for defining what makes for clean or good data, MDM is about enforcing those policies. MDM gives you a single location to configure data quality rules and ensure they’re applied consistently and automatically across the enterprise.
- Data enrichment: Data enrichment is the process of taking the data you clean up with an MDM solution and improving it with supplementary information. You can theoretically solve the GIGO problem without data enrichment, but it’s still valuable if you want to make the most of your input data.
- Data integration: This is the primary way MDM breaks down data silos. Instead of having garbage data spread across the organization, MDM serves as a single source of truth for accurate, trustworthy data.
- Data stewardship: Master data management provides an interface for data stewards to remediate data the MDM solution identifies as garbage.
- Hierarchy management: With MDM, one steward’s trash is another’s valuable insight. Hierarchy management is a feature in many MDM solutions that lets you manage complex relationships in your data to better understand the full context of the organization’s relationships with customers, suppliers, assets and materials.
- Workflow automation: Manually reviewing potentially garbage data can be an enormous time suck. This is where workflow automation shines by providing a means to orchestrate reviews and approvals of data flagged as potentially garbage across complex business processes or workflows.
Multidomain MDM platforms take this even further by providing the data model flexibility needed to allow the business to develop a common data model that accurately reflects both the current and desired future states of the business.
If you want to resolve your organization’s technical debt and fully enable any digital business transformation, implementing MDM and data governance is critical to taking out the trash of dirty data.
Learn More About Data Quality
Dig deeper on the individual dimensions of data quality and understand the value of fixing data quality issues at the source in our 15-page guide on the What, Why, How and Who of Data Quality.
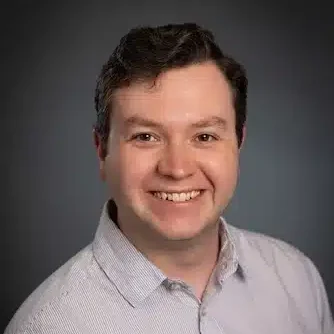
Forrest Brown
Forrest Brown is the Content Marketing Manager at Profisee and has been writing about B2B tech for eight years, spanning software categories like project management, enterprise resource planning (ERP) and now master data management (MDM). When he's not at work, Forrest enjoys playing music, writing and exploring the Atlanta food scene.