There’s a palpable buzz around artificial intelligence (AI), with many heralding it as the next big thing in technology. In several of my recent conference presentations, I suggested AI is fueling a new “golden age” of data and analytics and that CDOs are uniquely positioned to benefit from this unprecedented momentum. I also suggested that I believe AI will form the foundation of a new business “operating system,” not unlike the internet or cloud computing. So, when it comes to AI hype, I’m a believer.
However, not everyone shares my view on the current state of AI. In this article, I’ll attempt to lay to rest rumors of an AI bubble and make the argument that — while there are some similarities between the dot-com bubble of the early 2000s and what we’re seeing now with AI — there are enough significant differences between the two that we should think twice about conflating them.
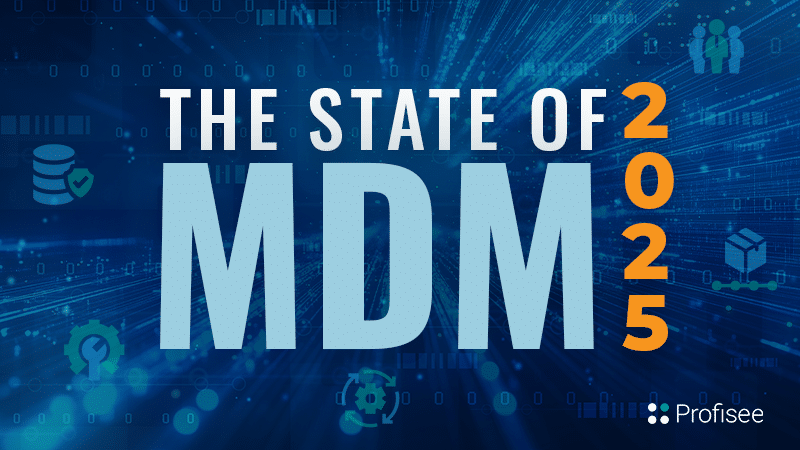
The State of Master Data Management (MDM) 2025
AI Bubble: Fact or Fiction?
Recent discussions have surfaced about the so-called “AI bubble,” where many are suggesting that the hype around AI may be outpacing reality. For example, the CEO of Baidu recently suggested that AI is indeed overhyped and that the bubble will soon “burst,” leaving 99% of the companies in the AI space bankrupt.
Data futurist Bernard Marr recently penned an article for Forbes on the issue, where he noted “there’s a lot of speculation that the AI ‘bubble’ may be about to burst,” citing recent stories highlighting the massive discrepancies between the money being spent on AI infrastructure compared to earnings of AI companies as evidence of a potential crash.
Another pundit recently suggested the financial market for gen AI-based systems would collapse before the end of 2024, suggesting companies now valued in the billions will become worthless overnight — not because of excessive spending on infrastructure but because, as he states, “Gen AI could be a dud.”
The AI Bubble — Myth or Reality?
The term “AI bubble” has been thrown around frequently, drawing comparisons to the dot-com bubble of the early 2000s — a time I’m very familiar with. I started working in 1995 for an internet startup that would eventually become the global default for accessing the internet, America Online (AOL). My career with AOL lasted over a decade — between 1995 and 2005 — where I had a front-row seat to both the explosion of the internet and the bursting of the internet bubble.
The similarities between the boom of the internet and the boom of AI are striking. In the late 1990s many extremely well-funded telecommunications companies dumped billions of dollars into internet infrastructure, deploying the millions of modems, switches and network cables needed to wire the globe over dial-up connections. Then three years later, all that infrastructure was ripped and replaced with newer, faster technologies to support broadband connections. At the time, everyone was talking about how both unwise and unsustainable those investments were.
At the same time this infrastructure was being built out, countless numbers of small internet companies — many without any sort of business plan or even a viable product — were getting funded by eager investors hungry to jump on the internet bandwagon and become the next dot-com millionaires. The list of companies that went from boom to bust is long, including Webvan, Pets.com and Excite.
As the bubble of 1999 transitioned to the bust of 2000, most of those dot-com companies, including several smaller infrastructure providers, went bankrupt, leaving their investors high and dry. Yet, most of the bigger telecom and networking companies, like AT&T and Cisco, weathered the storm and continue to make significant profits off those internet infrastructure investments to this day.
Lessons From the Dot-Com Bubble
The dot-com bubble offers valuable lessons for today’s AI landscape. Initially, over-expectations led to a market correction, but eventually, the internet economy matured and became deeply integrated into our lives. AI is undergoing a similar maturation process. By learning from past technological bubbles, we can set more realistic expectations and better harness AI’s potential.
Will many of the smaller software and analytics companies focused on AI go bankrupt, as we saw with the dot.com crash? I suspect some will — but the impacts will be nothing like those we saw in 2000, given the risk tolerance of investors has drastically changed since the 2008 financial crisis.
Back in the early days of the internet, money was flowing freely, and anyone with what sounded like a decent idea could get access to capital. That dynamic has changed, and the bar has been raised for VC and PE investing in AI companies, even while the mania remains high.
Considering the money being spent on AI infrastructure and data centers, I do not see the same thing happening now as what happened in 2000. In 2000, some telcos went bankrupt because they spent billions on deploying excess infrastructure where there wasn’t sufficient demand. This time around, there are two factors significantly limiting the ability of the data center industry to build an excessive amount of capacity by comparison to growing demand:
- Access to GPUs
- Access to electrical power
The first issue has resulted in massive stock market gains for Nvidia. The power issue is so acute that it’s motivated both Microsoft and Amazon to invest in nuclear power plants.
So, is the complete collapse of the market for AI solutions imminent? I very much doubt it, but that doesn’t mean there are no risks associated with companies looking to operationalize AI at scale within their respective organizations. The demand for AI and the automation and scale it enables may be here to stay, but the ability of companies to successfully deploy it remains the biggest risk to the viability of the industry in the short term.
DATA INSIGHTS,
DIRECT TO YOUR INBOX
The Importance of Data in AI Success
When it comes to potential risks for companies looking to implement AI-based solutions, access to high-quality, trusted, and well-managed data that can be used in the creation of new AI-based solutions — including chatbots and copilots — tops the list. A focus on core data foundations within a robust architecture is essential. A good example of this is a data fabric, which supports all data management use cases, including master data management (MDM) and data quality (DQ).
However, it’s equally important to understand that multiple forms of AI have different data requirements. More traditional forms of AI, including causal and predictive AI, rely on more structured data. Generative AI solutions require unstructured data. Having a data management program scope that extends across both structured and unstructured data is critical.
Start Preparing Your Unstructured Data Now
This focus on unstructured data as the key driver of gen AI success is a massive wakeup call for all data leaders. Efforts should be underway now to start discovering, profiling and tagging unstructured data assets at any company looking to use their internal data to fine tune the behaviors of gen AI-based chatbots or copilots.
Systems which expose complex relationships in data — including all forms of semantic layers and MDM — bridge the gap between structured and unstructured data. The insights an MDM tool provides around the quality and accuracy of core master data (like customer, supplier, product, etc.) paired with the complex master data relationships managed in an MDM tool will be critical to help ground the behaviors of all AI-based solutions.
For gen AI solutions, this will be done through complex RAG patterns. For non gen AI- and ML-based solutions, this will be done by building models on top of only the most trusted and accurate data available within the organization — consumable golden master records.
Not focusing on the quality of the data ingredients used by AI-based solutions will result in AI products that fail to accurately or reliably predict, represent reality or provide accurate responses. So, while the wider AI industry will surely survive any potential bubble bursting (just as the internet survived in 2000), corporate AI efforts built on top of data ill-suited to support AI use cases may not be as lucky.
Practical Steps for AI Investment
To limit the risk of your corporate AI bubble bursting, consider the following.
1. Start Small and Scale
Begin with manageable AI projects and scale based on success. Identify appropriate use cases and set clear success criteria. I absolutely love the book “Your AI Survival Guide” by my friend Sol Rashidi, as it speaks specifically to making sure you choose the right use case for early stage AI efforts.
2. Focus on Data Quality and Governance But Adapt Your Frameworks
Implement robust data governance frameworks to ensure data quality and security. Data leaders must guide AI initiatives and mitigate risks. Expand the scope of any legacy quality, governance or management frameworks into the domain of unstructured data to ensure you can inventory, profile and tag this data as a starting point.
In the long term, consider adapting your processes to apply data quality rules, including data validation, to all unstructured data. Focus on MDM and managing master data relationships but look to slowly integrate more knowledge management-based approaches within your broader data management and governance frameworks.
3. Leverage Existing Tools and Technologies
Use pre-trained models and existing AI tools to reduce costs and complexity. Frameworks like retrieval-augmented generation (RAG) can enhance AI implementations. As part of this, you need to get comfortable with the use of knowledge graphs and vector databases as essential tools within RAG processes which can help chatbots and copilots provide more accurate and consistent responses.
The Future of AI Investment
AI investment should be viewed with a long-term perspective, focusing on sustainable growth rather than quick wins. Continuous innovation and adaptation are crucial. Emerging trends and technologies will shape the future of AI, and staying ahead of these developments is essential for maintaining a competitive edge.
Set Your AI Investments Up For Success with Good Data Management
While the AI bubble may seem to have burst, the reality is that AI, when backed by strong data management practices, remains a worthwhile investment. Data leaders and organizations should approach AI with a balanced perspective, leveraging lessons from past technological evolutions to maximize their AI initiatives’ potential.
By following these guidelines, organizations can navigate the complexities of AI investment and unlock its transformative potential. Let’s embrace the future of AI with informed optimism and strategic planning.
Subscribe to the CDO Matters Roundup Newsletter
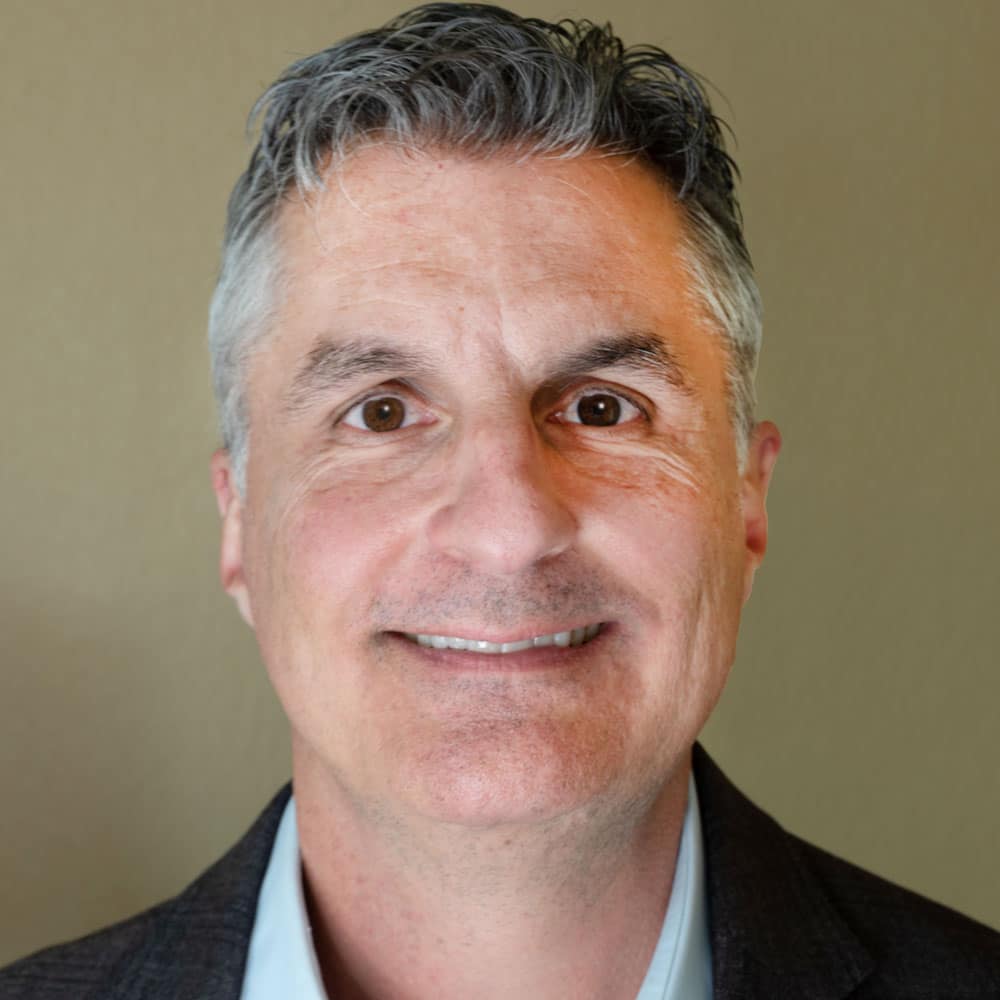
Malcolm Hawker
Malcolm Hawker is a former Gartner analyst and the Chief Data Officer at Profisee. Follow him on LinkedIn.