Updated on April 19, 2024
Data managers play defensive tackle in the fight against poor-quality master data. They’re trying to fill data gaps and keep bad data from making it to the end zone, so to speak. They have as tough a job in the data management trenches as athletes do on the gridiron.
Falling down here has serious consequences. When master data is inconsistent or incomplete across systems, the entire company is affected. Bad data hampers the work of everyone. The data analyst building the business case for data’s role in digital transformation. The business analyst who has to make sure that the right data gets to the right people at the right time. The CEO who wants to boost bottom-line results through analysis of a single, clean, 360-degree view of customer, product
or other master data.
Unfortunately, without the right technology, tools and processes, data managers have a hard time—and spend a lot of time – trying to master data quality issues. Too many users contribute various interpretations and identifiers of master data across multiple applications with different backend databases, which may reside on-premise
or in the cloud.
It’s a tall order to manually identify, clean, dedupe and synchronize data, and set it up to be simultaneously maintained and easily shared across systems and business units as well as dropped into cross-functional enterprise workflows. When a drop in the stitch leads to a lack of consistency for critical reference data – whether covering single or multiple domains – processes can be negatively affected all down the line. There can be no good results when bad information informs reports, dashboards and analytics.
Five Will Get You Ten
Protip: Data quality issues stemming from problems like these can be overcome with smart master data management practices and applications to provide accurate, consistent and complete master data across the enterprise.
With that in mind, use master data management to solve data quality issues with these technology-focused practices:
1. Simplify cleaning and standardizing master data
The modeling process, which builds on defining the contents of each attribute and mapping each source system to the master data model, should define the transformations needed to clean source data. This is a requirement for creating a master list. Cleansing includes normalizing data formats, standardizing values and replacing missing ones.
2. Get that golden record
Highly accurate MDM must have a laser-focus on enterprise-scale matching strategies to eliminate data duplications that can reduce the value of a merged master data list. From an MDM hub, rules and processes must be defined to determine which attributes of matched records from multiple sources will create the single, complete master record – aka the Golden Record.
This is the way to harmonize data values from designated authoritative systems (a “single sources of truth” for a particular domain, such as a CRM system) and serve them back out to all related source system records for ongoing consistency. Matching or business rules also can be leveraged natively as part of workflows, ensuring data consistency across complex business processes.
3. Capture metadata and maintain it in a central hub for easy access to definitions, descriptions and associations that describe the master data application
Managed as an MDM model, metadata – models, versions, entities, attributes, hierarchies, business rules and so on – provides a way to understand how data in one system maps to data in another and how systems cooperate on information delivery. When all changes to metadata are synchronized, users always have the most up-to-date definitions; when they are logged, it is possible to understand how changes will impact systems that manage data.
4. Use a single MDM platform to manage multiple master data domains
Customer, product, asset, and supplier are just some of the master data domains in a typical enterprise. Traditionally, domains such as these have been individually covered by separate MDM platforms. The multi-domain approach, however, provides a consistent data stewardship experience across all domains, while simplifying the sharing of verified reference data across domains.
5. Facilitate proactive data stewardship
Data stewards can’t single-handedly catch all data quality problems. Sometimes they need data quality issues lined up for them in a queue. Alerts about issues then can be automatically posted to them via email with links so that they can fix
these items quickly.
Remember, it’s the last ten yards in the red zone that makes the difference between winners and losers. Use these 5 master data management strategies to cover the last ten, enter the red zone by solving data quality issues and emerge as the winner!
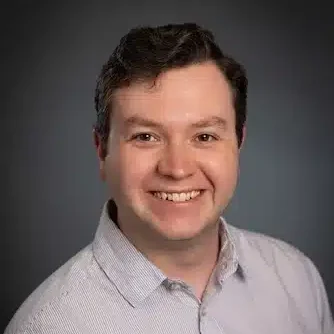
Forrest Brown
Forrest Brown is the Content Marketing Manager at Profisee and has been writing about B2B tech for eight years, spanning software categories like project management, enterprise resource planning (ERP) and now master data management (MDM). When he's not at work, Forrest enjoys playing music, writing and exploring the Atlanta food scene.