Key Takeaways
Data modeling provides a structured blueprint for organizing, storing and managing data — starting from high-level conceptual models to detailed physical models tailored to business and technical needs.
The modeling process involves identifying domains, properties and relationships to accurately represent how data elements interact across business systems, supporting consistency and informed decision-making.
Effective data modeling improves analytics, streamlines business processes and supports better data governance, serving as a foundational step in successful master data management (MDM) initiatives.
Company data stores tend to grow organically due to the use cases of business units. But when an organization gets serious about overhauling their data management processes to increase efficiency and decrease costs, building a data model is often the first step.
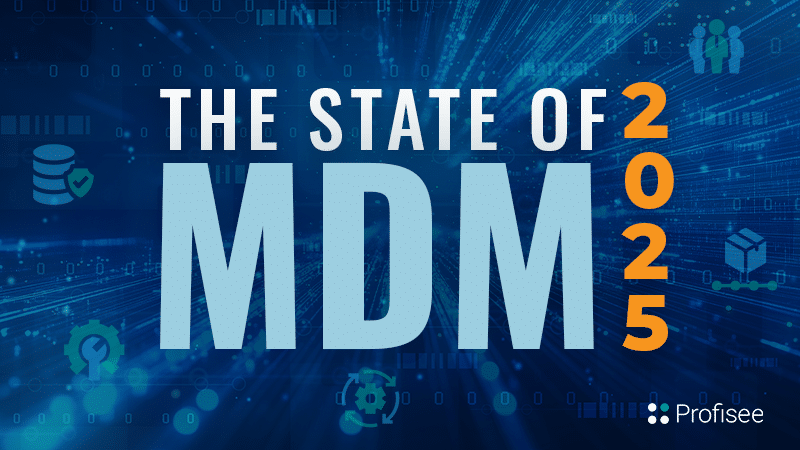
The State of Master Data Management (MDM) 2025
What Is Data Modeling?
A data model visualizes how you structure, store and visualize data so you can access it usefully later. Data models can vary in detail and complexity depending on their use case, and these models should change in structure alongside the business needs to reflect the actual state of data in an organization.
Data Model as a Blueprint
When you add another room on to your house, the architect on the job will draw up a new floor plan so everyone on the project knows where the new room will sit in relation to the existing building, where to locate the doors and windows and the overall shape of the new room.
Data models act in much the same way, showing where the company stores data within its digital landscape, what the relationships are between the pieces of data and how to structure the data before placing it in storage.
Data Modeling Types and Examples
Data models have varying levels of detail, depending on the use case and the data initiative’s level of maturity. Here we define the three main types and discuss their use cases.
Domain or Conceptual Model
A domain data model or conceptual data model has the least amount of detail and should be used for planning purposes and information gathering across the organization. For example, when the company knows it needs a data management system but needs to continue investigating the vital data needed for each department, it may use a conceptual data model as a starting place.
The domain model will show domains, properties, relationships, and business rules that will apply. While it’s a high-level model, the best domain models clarify the structures and business rules that business users will follow.
Logical Model
A logical data model takes the conceptual model and adds detail that defines the business rules to follow (each vendor must be connected to one or more products; no product can be assigned to more than one category) and how data should be structured within systems.
The logical model contains detail informed by the business systems and departmental needs as well as the needs of the data management systems. This model will help data analysts and data stewards build the tooling within analytics and visualization software.
Physical Model
A physical data model documents the technical specifications of the data management system. In our blueprint metaphor, the physical model would show everything needed to complete the house: electrical wiring schematics, paint colors, drawer pull styles. Database engineers and developers will use this model to build the databases according to business needs.
The physical model needs technical details about connections to business systems, data storage and transfer methods and any other information needed for the data systems to digest, store and dispense data to the appropriate business users.
The Data Modeling Process (Step by Step)
Let’s look at an accessible version of a database that many people use every day: the spreadsheet.
In your spreadsheet document, you have the default table with rows and columns. Build a list of your company’s products on the first tab, your company’s vendors on the second tab, and the company’s customers on the third tab.
Across the top row of each of those tables, you identify the types of data you want to document for each table as the column header.
Then, build out your table with the information you have by putting records into your table.
Congratulations, you’ve just built a database! Let’s look at how we retroactively build a data model for your database.
1. Identify Domains
Each tab or table in your database spreadsheet is a domain. A domain is a high-level block of data that helps data teams logically group important data. Products, customers, vendors, store locations and employee information are examples of master data domains a company might have.
Domains also translate to your golden record or vital business data that master data management (MDM) solutions organize and regulate. Because domains ideally remain consistent over long periods of time and across departments, they make up the foundation of a solid data strategy.
2. Identify Properties
Properties are the column names you identified in each of your tabs. They describe the domain and the types of data to document for each record. Useful properties may look different depending on the use-case. While sales may make all their vendor requests by phone, perhaps finance also needs the fax number to verify documentation.
Every table must have a primary key, a unique identifier that sets each record (or row) in the table apart from the others. In our example, the product ID, customer ID, vendor ID and category ID columns house the primary key.
3. Identify the Appropriate Model
The model you choose should reflect the state of your data. Some common models include:
- Hierarchical
- Relational
- Object-oriented
- Entity-relational
- Dimensional
Each of these models has benefits and drawbacks, but the ways that your domains and properties interact will help the team decide which is best.
4. Map Relationships Between Domains, Properties, Records
The existence of a primary key implies the existence of a secondary key. We’ll call this the foreign ID or the relational ID because it shows the relationship between the record and another table. In our example, the shabby chic product has the category ID of 543, the identifier for a dresser.
Relationships between domains, properties, and records can be 1:1, 1:N (one category has many products) or M:N (many customers purchase many products).
5. Test and Revise
Once you’ve built the model, begin working through the business rules and requirements requested from the departments. Where does the model need adjustments, or where can appropriate workarounds or substitutions be made within business processes to lower complexity or increase speed?
Data Modeling Best Practices and Technique
Data modeling is an iterative practice that should grow with your data systems. Consider implementing some of these techniques and best practices:
- Engage stakeholders across the organization to gather data requirements and understand how different departments use data.
- Build from conceptual to logical to physical to increase complexity as required.
- Model based on data, not on experience. Your team may have experience working with relational databases, but if the data says object-oriented, go with that.
Model with analytic and business goals in mind and work with the complexities of those.
Data Modeling Benefits
Companies that document their plans have a better chance of success because new team members will have context and the team can analyze decisions and results. While it’s a good idea to build a data model for the sake of planning the initiative, data models also have the following benefits:
- Better analytics due to more reliable and consistent data
- Increased organizational cooperation around data processes and goals
- More efficient business processing
Fewer errors and rebuilds for database and business intelligence teams
A Blueprint for Success
Data modeling is just one piece of a larger data management and governance plan. Building out the model with golden record data first can speed the implementation of a master data management (MDM) initiative. The documented business rules and relationship structures will inform governance and database plans.
Guide: The What, Why and How of Data Governance
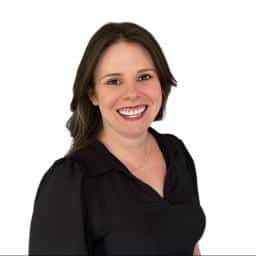
Tamara Scott
Tamara Scott is a writer, editor and content strategist with over a decade of experience located in Nashville, TN. Tamara holds a Master's in English from Belmont University, formerly served as Director of Content for TechRepublic, and her work has appeared in ServerWatch and EPI-USE.com, among others. When she's not crafting SEO-informed and conversion-ready content for SaaS and IT service companies, she's probably at home on her pottery wheel. Connect with her on LinkedIn.