Episode Overview:
Episode Links & Resources:
Good morning. Good afternoon. Good evening. Good whatever time it is, wherever you are in this amazing planet of ours.
I’m Malcolm Hawker. I am the host of the CDO Matters podcast, and I’m thrilled that you have joined us today.
Today, we’re gonna talk about something interesting called the data doom loop. And to share his insights on the data doom loop, we have mister Ken Stott. Ken, welcome to the, the podcast.
Well, thank you for having me, Malcolm.
Yeah. I was very intrigued, when when Ken reached out and said, hey. You know, I wanna talk about some of the things that I’m seeing within within large organizations.
And, he had me at DataDoom loop. It was a topic that I’m interested in discussing more. Maybe we’ll get into other topics of discussion as as well. Just a little bit about you, Ken. You’re the the field CTO at Hasura.
You are a company. Correct?
We are. That’s a way to explain it. Okay.
Okay. Oh, well, I don’t wanna drastically oversimplify. What’s what’s the elevator pitch then?
It’s more of a universal data access layer. APIs are part of it.
Okay. Well, that’s certainly something that we can talk about if we still have time after we talk about the data loop Dune loop because this has been a topic that I have been really closely following for a while now. Just kind of talk about data access, data virtualization.
Last episode, I was talking with the CTO of Denodo, Alberto Pan. Yeah. And, I don’t know if you run into Denodo, but we were talking about yeah. Logical data layers and this this this abstraction layer that sits between operating systems and data warehouses or sits on top of data warehouses as it were to be able to better access data, that that’s something I think is increasingly relevant in an age of of AI when we’ve got more and more and more and more data.
Yeah. Yeah. Absolutely.
We need to govern it, and we need to put our arms around it. But let’s let’s start with the topic du jour. If if we will, share with me what is the data doom loop, and why should a CDO be aware of it?
Well, first of all, it’s meant to be a little provocative, so I’m glad that It is. Glad that it provoked you.
So this is my theory.
In large organizations, they’re perennially unhappy with the results of their data their data ecosystem.
And because they see that they’re unhappy with it, they, they get interested in spending more money in it into it. What they do is they find new tools, new ways to do it. They layer it on top of that and actually create a more complex environment, which ends up making them unhappy about the results of their data ecosystem.
And this loop has gone on for a very, very long time.
And I think, I think there are some there are some data that supports this idea.
So, for example, over the last three years, spending in data has gone up at least ten percent per year. It’s projected to do the same again next year. And if you look at data maturity scores, pretty much flat.
Right? People are spending a lot of money, but they’re not getting results.
I I completely agree. I this is actually something that I’ve touched on in a few of my presentations over the last couple of years, which is the the idea that we’re kind of like, I would argue we’re in the golden age of data and analytics. Right? Like, our our companies desperately want us to drive value from data, to use data as a lever to transform their organizations, but survey after survey, there’s data to suggest that we’re not progressing to that. So I when it comes to technology, throwing more technology at the problem, which is what you’re saying is is kind of the underlying premise of the of the doom loop, I I have a hard time thinking that anybody would argue that because we do love to throw technology at the problem.
But let’s let’s let’s peel the onion a little bit on that because when you try to do the inverse, right, when you when you try to may maybe not make it about technology and make it about maybe people or process, I think you could argue some of the same outcomes happen. I think you could technically argue that maybe a data mesh movement, for example, which was which was, by their definition, socio technical, not just technical, but but we’re touching on the people aspects.
One could argue that’s pretty complex too. So is this a darn if you do, darn if you don’t situation? How do how do we get out of this?
I agree that when you get on the people and process route, as a technologist, frequently, it turns out to be unsatisfying in one way or another. Right? It’s extremely difficult to engage all of the pro all of the stakeholders. Right? Align them around these technical objectives and deliver against that. The I I think one of the issues with the our data ecosystem is the diversity in the range of stakeholders that we have to satisfy.
And I think what often happens is is people try to engage from a people process perspective. What they end up doing is creating kind of islands of technology that are kind of about eighty percent solution, but they fail to get the final integrations in place, and this is kinda where it falls down. It’s all the all the people strangling strangling. Struggling, let’s say. Strangle might be another interesting idea. Could be. Struggling to to actually fill those gaps, between these sorts of items of technology.
Well, herein lies the the the either ends of of the spectrum, I I think. Right? So on one end of the spectrum is this giant kinda centralized, monolithic, big, hard to update, hard to manage, hard you know, arguably not responsive to change and difficult to innovate, one end of the spectrum. The other end of the spectrum is what you’re calling these islands of technologies, which one could argue was a highly, maybe, federated, for lack of a better word, a a highly federated ecosystem where you’ve got now you’ve got a whole bunch of technologies to manage. Is it am I articulating kind of this trade off well?
Yeah. I think you’re getting there.
And I was thinking more functionally, things like governance systems versus data delivery systems, etcetera. But, also, it could be organizationally, you know, markets versus trading versus loan systems, things like that.
I do think it’s an integration problem, and I think data mesh was kinda on to something, because you cannot scale without federation.
Right? You a monolith is never gonna work. Centralization has diminishing returns. That’s been proven over and over and over again.
And yet, by the way, it’s everyone’s favorite approach is to is to centralize.
But the problem is is is the integration that would support a federation solution, kind of what was articulated in data mesh, just hasn’t materialized to a level that people can kind of comprehend it, use it, manage it, integrate it into people and process to get that sort of ideal state that, Shamik Dehghani was, trying to articulate in data mesh.
So you articulated what you what you say is that the core of the problem as as an integration issue. And and I I think I agree, but let’s let’s let’s explore a little bit.
My biggest gripe well, I have I’ve got two two bigger gripes with with the data mesh, but one of them was the coordination that is still going to be required across domains for cross functional use cases. Right? Sales marketing sales, marketing marketing finance.
The idea that those groups would just, like, naturally want to cooperate with each other and and integrate, for lack of a better word, I just did I just don’t see it. Right? Like, you have to, at a cross functional level, to do quote to cash.
But but managing that is is highly problematic and arguably why I have a paycheck because I focus on MDM, right, and coming up with common definitions and and trying to integrate at a data layer. Right. So I think I think you the the integration is is is maybe what you you’re onto, but then we can start to get into philosophical discussions about, you know, common languages and and ontology, the definitions. You know? And so what do you where where are you from that perspective? I mean, what what is the best way to integrate across domains?
By the way, you hit on something else, which is is are is everyone motivated to actually solve this problem? Maybe they’re getting paid to to continue the issue.
But, yeah, I think that I think that when you’re again, I I do I’m kind of a mesh proponent.
The problem is the tooling hasn’t gotten us there. The philosophies to underlie haven’t matured quite yet. Right? I do think that, each domain in a semantic layer in your was it marketing and sales for those that you mentioned?
Sure. You can go with that.
Yeah. They have to they have to sort of own their definition of data. They have to advertise that in sort of a semantic layer sort of form.
So everyone loves the phrase data products now, which, by the way, I see as rebranded data mesh. If you dig into data products, they they still require you to do all the same things. It’s just it’s just flipping the script a little bit around to the value side of it as opposed to the architecture side of it. But but I think that has to happen. There has to be, again, some level of automation to take the individual, sort of semantic layer definitions, glue them together with relationships that that the individual domain teams define, and there has to be some oversight across the universal semantic layer that you’re building to determine that the the semantic differences aren’t sort of becoming overwhelming so that you continuously are refining that universal semantic layer to something that, most stakeholders would be able to comprehend.
So so this is another thing that we’ve been talking about a lot on the podcast. And I didn’t intend for us to go towards semantic layers, but but here we are.
We’ve had a lot of conversations recently related to semantic layers, related to graphs, related to ontologies.
I’m intrigued by this idea of what’s called knowledge management and how to bring the world of knowledge management closer to data management.
But when you talk about bringing these world together and and having some of the semantic differences not be what I’m hearing you say is having them to the point of not being meaningfully different.
Yeah. Yep.
May may maybe. Like, customer and account. If we can agree at a conceptual level that those are kind of the same thing and we just find a way to link them, those are that’s fine. Good enough.
But there’s still there’s still there can still be bear traps there when you start talking about core differences in definition.
Do you agree?
Well, because well, customer and account is a great example. They are clearly not the same thing.
Right. Right. Right.
Maybe an example, but party and and well, you know, party Yeah.
I don’t know.
Counterparty Yeah. Customer.
Yeah.
Those are all those are all, legal entities. Individuals are legal entities of some sort. So, yeah, there’s certainly there’s certainly ontologies that can tie all these things together, of course.
But I think sometimes it’s not an ontology. It’s just a preference in term. Those are the things you really want to kind of squeeze out of the system. Right?
The other thing when we talk about a semantic layer is it has to be operationalized in some way so that when people are asking for data or talking about data or coding those things, they’re all using the exact same terms. And I don’t know and I’m sure you’ve been in this situation, but if you’re like a support person and you get a ticket from somebody saying this thing and this system happened in this way, ninety percent of their time is usually trying to translate the, end user’s language to describe that into the way the developer maybe coded something. Right? And these these translation layers are incredibly expensive.
And so the the more we can reduce those translation layers, it adds value across your entire organization.
I that makes that makes complete and logical sense to me. Something you touched on there, I think, is is interesting, which is if it’s just a different label, that’s that’s that’s one thing. But if if we’re talking about fundamentally different definitions, that’s another. I think for years and years, we’ve we’ve we’ve tried to attack this problem from so many different directions. You know, fifteen years ago, it was this heavy handed top down kind of, you know, single single Mhmm. Single something to rule them all. Well, we don’t that didn’t work.
And and now where it seems like the we’re we’re trying to say, okay. Full and complete federation. Let’s let’s go with that.
I don’t I don’t and and I do I keep coming back to the original kind of premise here of of this these two ends of the spectrum and how do we find the the the the nice amazing sweet spot in between the middle of all of these. And I’m not entirely sure it’s an either or. I think it may be a both.
Yeah. I think the solution is not to change.
It’s to add some things.
I think our overall architecture is missing components.
Right? It’s perfectly fine to centralize to a certain level. It makes total sense. There are there are use cases that that you ought to do that. Right?
But the parts that are missing in the overall architecture are really these integration layers. It’s data access.
To me, it all comes down to having the right data access strategy in your organization, and that can you can think of that as an as a semantic layer that has been operationalized, creates the lingua franca that all organizations talk about, and you can do work using those terms. Right? And to me, that’s the big missing component is some and, some form of universal data access access layer that allows you to to propagate those semantic term that semantic terminology across your organization and to operate using those terms.
So may maybe we need to press on the definition of access because I because I’m hearing you say because because access to me maybe is a little bit of a loaded term because, you know, there’s access and identity management. And and maybe some might be listening to this in the podcast and thinking, okay. Well, you know, I’ve I’ve I’ve I’ve already got a tool for for access management. But what you’re saying goes well beyond just map. Exact exactly. What you’re saying goes well beyond that.
And, actually, even delivery access.
I’m sorry, Tim.
Yeah. No. That’s no.
And and I’m glad I’m glad we’re talking about it because because what we’re talking about here is not just are you you seeing what you’re supposed to be seeing, which is important, but but it’s also if if I’m searching for something or if I’m doing a SQL query for something or if I’m if I’m talking about something as I’m typing notes into a a fee a free text field in a CRM, whatever that whatever that use case is is that the terms that I’m using or the terms that I’m looking for or my intent accurately or maybe not intent or or what is being represented is consistent with some idea of a governance policy related to that thing.
Mhmm.
Yeah.
Okay. Well, so it’s interesting then.
What what would you say to and sorry. We’re getting really philosophical here.
Like, salespeople are using a CRM today. Right? And and salespeople have their definition of things that they’re using day in and day out.
And I think we we run into it’s not problems is not the right word, but we run into the difficulties when we need to change that operational definition to conform with some analytical definition Mhmm. That may exist at more of a cross functional level, getting back to my my concerns about the mesh. Mhmm.
How do you make those two things coexist happily?
The the the the functional definition and a cross functional definition, how do you make them exist? Is that what you mean by an integration challenge? Can you just can you just, like, logically link those things together?
I’m struggling with an example.
So first of all, I don’t think we’re gonna police the language that people use to talk. You know? Right? Agree.
When we type in a free text field, some some poorly articulated explanation of something, that’s gonna be the way it is.
Right? We’re talking about the definitions of data at rest or in motion so that when we apply a label, the label, is a is a is a good, a good explanation of the information that’s contained within that element. Right?
The other thing I would say is I don’t think that there’s a huge number of elements that that are cross functional. Right? But I do think that the cross functional ones are the ones that deserve the most attention.
So, again, my theory is that if you’ve operationalized your semantic layer, you now can observe how these terms are potentially being linked together, and you can apply other kinds of automation to determine if if meaning is being misconstrued, if meaning is being obscured in some way in the way people are describing data elements.
I I think you’re on to something there, which which I which I find interesting. I kinda I I like the concept of data observability because because it can be applied in so many different realms. So data observability is, you know, I I look at at at signals, for lack of a better word, maybe exceptions, alarms, triggers, something. I look at signals in a pipeline for and I may be even using some m ML or something else on top of it to start predicting when the pipeline might fail based on on signals that I’m seeing.
What I heard you say, and I’m I’m putting words in your mouth now, but I’d love to hear your perspective, which is, well, maybe we can do the same thing with how data is being used in the organization from more of a kind of a quality profiling perspective or some other sort sure. To look at and say, uh-huh. There’s something amiss here, and maybe that process is gonna end up running slower, or maybe that process is gonna fail because of some oddity within the data.
Is is that kind of the the concept you were trying to I think, again, if you’ve got some sort of data access layer, you can observe how it’s defined.
Right? So it’s built using some sort of federation principle.
Right? There’s a focus on the relationships, cross domain relationships in particular to determine if there are sort of semantic differences you need to sort of drive out. And not only is the building of that thing observable, but as data flows through it, you can observe the patterns that people, have a specific interest in. And all of these things, you can you can do so many things from anomaly detection, data validation, right, right at that sort of final egress point.
And I think that’s the big thing people are missing, is they’re missing this this sort of ability to dip into what people are asking for in data across an entire organization and see if it makes sense. Right? See where things don’t seem to be maybe they’re drifting. Right?
It used to look like this. Now it’s starting to look like this. Right? Or maybe people are combining things that we could probably figure out that there was temporal differences, and that’s not really a good way to combine data.
No one can see any of that today in most modern architectures.
I I love what you just said. I I love it because it it it aligns to what I was trying to kind of articulate around in some idea of of observability, and you were saying observe the data.
There there’s I heard two things there.
One was what I could loosely call kind of buy side signals.
Right? Like signals that that that people whether it’s typing it into into a search box or whether it’s chat chatting with ChatGPT or or or whatever. Like, in in the in the consumer realm, there’s there’s all sorts of intent signals. Like, I can go buy data about what people are searching for in the web and use that as a proxy to understand what do people want, not want.
So I heard you say, one, we could be doing the same thing in data to better understand what people are looking for, searching for, using great analogy.
Yep. That’s one. So that’s the buy side. That’s the consumer side, but also in the supply side, like, within our pipelines or within our processes or with our data quality processes or MDM processes to see, does that does what’s happening on the supply side actually align to the buy side?
So, again, I think that if everyone has a responsibility to sort of publish their semantic layer, right, along with expected relationships with other, data domains, reference data domains, or other operational domains, right, transaction domains.
We can we can evaluate I lost track of the question.
Why don’t we back up for a second? Maybe we’ll edit this one. Tell me the question again.
Like, understand understanding the signals to evaluate. Right?
Are we are is how is how we’re managing and governing data aligning to I I think I remember.
The reality of our organizations, whether that is from what people want or even necessarily of how the business is operating, which I think is an interesting other angle.
Yeah. So so, like, again, I think this basic activity that I’m describing, you can drive so many potential control functions from it. Right?
You can you can build governance from it. So you can build catalogs, search tools. Right? You can build processes that look for semantic differences and make sure that those are resolved in some fashion. Right? You can, you can look at how people use data, how they combine data, and evaluate whether or not there are problems with the way that they’re doing that.
You can, you can, again, you can look for things like temporal differences or other things which are, perennially, the problems that people run into. Right?
Yep.
You can drive you can drive a single consistent identity and access management over that data. Right? And then you can determine if the right people had access to the right data. Those are all things you can do as you start to think about how do I build some sort of understanding of who’s accessing what data and how they’re combining it. I think this idea of how they’re combining is the thing that escapes everyone.
Right? All this stuff goes ultimately into things like BI tools or applications where people do all kinds of things that you’re not perceiving.
But if but if your ability to compose that data, right, and maybe aggregate it inside this access layers, now you have visibility into composition.
And when you have visibility into composition, you know how people you know how people imagine data should be combined to solve a specific problem.
Okay. That’s interesting because, like, we have a bit of a challenge in that once we’ve published the dashboard or once people have exported that dashboard into Excel.
Right. The horse is kind of out of the barn, and heaven knows what’s happening on the desktop with with what they’re doing with that data. You just use the word compose or maybe they’re refactoring the data or they’re doing something else to it like that. Like, whatever is happening in that secondary use or the the pivot that they’re doing outside of the dashboard in Excel, that’s it. That so that’s that’s that’s interesting.
Problem. That’s half of your problems are starting now.
Yeah. Oh, for sure. Yeah.
We Yeah. This this this lack of understanding of the last mile of data, which no one talks about, honestly.
Right? Yeah. There’s picking up so many problems there that you that you’re not seeing. Right? We all focus on, like, data quality ingest and building out some warehouse, etcetera, etcetera. All really important things, but it’s not solving all the problem. It’s solving just a part of the problem.
Right? Right.
That that could end up being the title of this of this podcast, the last mile of data. As much as I love the data doom loop, I because I think which which, by the way, I fully agree with. And and I do see that as a as a bit of a little bit of a downward spiral that is hard for companies to pull out of. But the last mile of data for sure, like and and I’m not so I think historically, we we we’ve tried to kind of control that, and may maybe maybe the answer is is to just better observe using your word.
Yeah. Yeah. Absolutely. So even if say so I like the idea of having an access layer that can do joins composition.
Right? I like an access layer that can support maybe multiple query languages. So you can do SQL, but maybe you could do GraphQL. Maybe you could have just predefined rest endpoints, whatever, as as a way for you to access data.
But all of those all of those languages imply, combining data. We can observe how data is combined when they get there. If you don’t have those services in your access layer, people start building it outside into sort of rogue systems and other things. So you wanna have these a rich set of access services that encourages people to use them and not build their own stuff so that you so that it escapes your purview.
Yep.
Oh, oops.
Delicate choice of words on purview.
Sorry.
Oh, sorry.
That’s that’s okay.
Oversight.
Yes. Yes. Oversight. I’m I’m I’m just I’m just pulling your leg. I’m just pulling your leg.
But per Purview is is is is is obviously a data catalog product from Microsoft. So but alright. So you’re you’re you’re field CTO, which means you’re out there. You’re in you’re in a hotel today, which means you are out talking with clients all day.
I am. Yeah. Yep.
Okay. So what are you hearing out there, Ken? Like, what what what’s you know, obviously, everybody’s talking about AI. What what are what are some of the if you could kinda summarize the the two things that you’re hearing the most out in the out in the field from a major challenge perspective. Obviously, you’re coming at it from the perspective of your company’s technology.
Mhmm. But I suspect a lot of the the questions get a little bit broader. What are what are some of the the things that are keeping your clients up at night?
Oh, so by the way, I mean, similar to you, Malcolm, I think, I I try to not sell as a field CTO. My my my, remit is to really talk to people about the strategic objectives they have within kind of the data management space Yep. And try to align our products with other people’s products to to help them solve their problem. I’m kinda like a free consultant. I just happen to be paid by.
So I do this all day long.
I can tell you one of the more interesting things that someone told me just last week was they were really lamenting how much manual effort was put into regulatory investigations.
And it it it was just driving them a little bit bad.
But I would say it’s it’s been my experience as well.
You would hope that with all this data governance, with all of this data to support how data is being used, when a regulatory investigation comes in the door based on data, that you could answer it pretty quickly.
But, no, typically, we’re bringing in McKinsey. We’re bringing in Deloitte. We’re carving off fifty people from, you know, various teams, and they’ll spend a couple of months to develop an answer to this question with thousands of spreadsheets and, you know, all these all this sort of effort that goes into it. And, ultimately, they they frequently don’t develop a systemic solution to it. They just answer the question. Right?
And all of the time and money to do that, if we could redirect that into systemic solutions, it could be so much more satisfying and powerful and and value adding to our organizations. Anyway, I extended a little bit beyond what that person told me, but but that I thought that was a fascinating conversation.
Well, so, I mean, that does kinda touch on the integration challenge because I suspect a lot of the challenge there is is data silos. Yeah?
Yep. Absolutely.
Okay.
That’s And that last mile. Right? I’ll yeah. All what always ends up happening is is the the question always turns out to be that last little bit that went into sort of developing a regulatory report or something like that. And oftentimes, those systems aren’t as observable as sort of a a core part of your data ecosystem.
Interesting. Interesting. Well, so I I assumed, naively, perhaps, that the your answer was gonna be, well, everybody’s talking about AI.
It always comes up. It is not it is like, it’s not necessarily the majority of our conversations.
I think everyone just recognizes that AI has some potential solutions within data. Certainly, that’s part of our conversations. Like that semantic like like, finding semantic differences is clearly a great, AI, solution that you can apply into data.
Sometimes the conversation is about data prep for AI and building better data prep environments for AI. It’s not the majority of the conversation, though. Like, at at most, you know, somewhat less than that.
So where are you on on the data prep for AI thing? Because I think we’ve got a little bit of a hangover hap happening in in the world of of, let’s just call it, legacy or more traditional data management, which which seems in theory to be not that well suited to Gen AI, which likes a lot of text.
And, you know, legacy data management is is generally tends to be more structured.
Where where do you sit on that when it comes to to data prep and and, you know, whether it is your technology or some other technology? Do you are you hearing clients out there talk about having to get their arms around all this text data floating around in their organizations?
Not. Specifically. But, no, I think the things you’re seeing make a a lot of sense. The rise of data lake houses, they’re pretty organized around building data prep for AI, right, for building AI datasets. That makes tons of sense. I think that fits below most of the things we’ve been talking about.
Right?
Frequently, these are domain level issues where I’m in sales and I’m, you know, gathering all the sales data, and I’m trying to develop some sort of AI data that I can feed into an algorithm.
Sometimes they’re they’re cross domain.
And in that case, I don’t think most of the heavy lifting for AI is particularly cross domain. By the time you get there, these are these datasets have been reduced through those sorts of other sort of domain level sort of analytical processing.
But but, yeah, that that’s sort of what I’m seeing on the It’s it’s it’s it’s not it’s not the be all and end all for for people that are trying to solve problems that your companies help solve. And and I’ll be honest It’s super critical, and they’re spending a ton of money, but it just doesn’t turn out to be the problem that I’m engaging them around, and that just doesn’t isn’t the number one thing we’re discussing.
Well, one could say one is is is more of the foundation of the house, and the other one is deciding what fancy feet fixtures maybe to put in it. Now I’m I’m kinda downplaying AI. That’s not that’s not fair. But from the perspective of the domain centricity there around AI, that I could not agree with more, particularly on the marketing side, right, where there are data scientists sitting in a marketing function where they’re trying to build propensity models, for example.
And they don’t have to deal with any of these cross functional challenges. They don’t have to deal with necessarily with with with how finance defines a customer. All they can look at is their sales transaction data and say, okay. You know, did they buy it?
Did they not buy it?
About eighty I I’m just making these numbers up. But to me, about eighty percent of all the AI activity is domain based.
When you’re getting into cross functional, you’re usually using more distilled datasets or or your or you’re in a domain, and then you’re you’re maybe pulling in a little bit of cross functional data. But, again, it’s more distilled datasets. But your big, you know, sort of large dataset is your domain dataset. Yeah.
Now that said, I think to do some of the things that we were waxing about earlier from a cross functional perspective and plucking out and observing kind of the the the state of data and the state of your business, I think to do that at scale, you’re necessarily gonna need to rely on some form of AI to look at transactional data, to look at time series data, to look at to look at all of this in in, you know, relatively scalable ways, right, with a ton of ton of data. I think AI is gonna need to be to be applied there.
But So all that observability data is a really rich dataset Right. For AI. And I think I mentioned it before.
AI as a solution to, you know, managing our data ecosystem has lots and lots of promise. But you’ve got to build some observability layer, right, that shows how your data is being used, and it has to be using some common terminology. So that’s why the semantic layer is important. So if you can do all of those things, now you can go to town with AI and drive out so many fascinating insights.
And on that, that is a great note to tie off on. Thank you, Ken Stott, for all of your wonderful insights. Thank you for indulging me in our philosophical discussion about definitions and ontologies, and, I’ve really appreciated your time today, Kent.
Well, wonderful, Malcolm. I and I, I thank you for allowing me to, spout off a few of the bearings.
Love it. Alright. So if you stay with us this far, thank you. I hope you’ve enjoyed another episode of the CDO Matters podcast.
If you haven’t subscribed, please do so. If we’re not connected on LinkedIn, please reach out. Connect with me on LinkedIn. I share a lot of content every day on LinkedIn.
I’m posting podcasts. I’m posting white papers. You name it. I would love for you to join our Studio Matters community on LinkedIn.
With that, I will leave it for for now. Thank you for tuning in, and we will see you on another episode of the CDO Matters podcast sometime very soon. Thanks all. Bye for now.
ABOUT THE SHOW
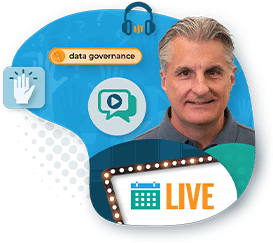