Episode Overview:
Malcolm Hawker and Apurva Wadokar of Autodesk dive into what it takes to build a data function that keeps pace with change, sharing hard-won lessons and forward-looking strategies along the way.
Episode Links & Resources:
Good morning, good afternoon, good evening, good whatever time it is, wherever you are on this amazing planet of ours. Thank you for joining the CDO Matters podcast. I’m Malcolm. I’m gonna be your host for the next thirty, forty minutes. We’ll we’ll we’ll see how long we chat today. I’m thrilled today to be joined by Apurva Wadodkar . She is the senior manager of enterprise data at Autodesk.
And we’re gonna have a great talk talk today about kind of evolution in the space, what Apurva’s seen over the last twenty years or so that she’s been doing this and managing a large team of data experts focused on MDM, governance, analytics, a whole bunch of other stuff. So we’re gonna have a wide ranging conversation today with somebody that is knee deep in doing the work, which a role which I I’m familiar with as well, but I’m super excited to to get a fresh perspective of Perma. Welcome, Studio Matter.
Thank you for having me, Malcolm, and I’m really looking forward to our conversation.
Me too. Me too. Well, why don’t we start and I mentioned your lengthy tenure in the data space. Why don’t we start with just a discussion of of some of the major evolutions that you’ve seen, some of the things that you’ve seen change over the last few years as as your role as a data leader?
Oh, absolutely. And I’ll I’ll tell you, I won’t even touch AI just yet. Okay. You go for an afternoon nap, and then there is something new, isn’t it? So so much so much is changing so ridiculously fast. But data, on the other hand, also has evolved, and I I bet you have also gone through similar transition. Remember the time when hundred percent of our data was on prem?
And we I remember we used to take, tape backups.
Remember that a Doctor was a big deal? Oh my goodness.
Then thank god for or or rather the the big trees for creating cloud. Right? That was a phase, which was, I think that that was a time when things started to get really fast, modern, and our cycle times were getting reduced on lead times.
Fabulous space, streaming analytics, you know, and and that was my way of, evolution also is with all these, products or services available in cloud, everything was a click of button. So we brought in streaming analytics.
I remember GDPR, what a what a time that was in getting everything compliant, massively parallel processing databases.
You know, so much time was saved in just partitioning and indexing schemes and designing around that. Remember how we used to go for data modeling with all me deep into those sort of topics with Snowflakes of the world and, you know, the other MPP databases and cloud, all that went away. So much time saved.
So love love that. And now this year, I’ll tell you, Malcolm, we are seriously looking at the lake house sort of, you know, architecture in certain space where we already have tons of data in s three buckets, and we wanna make it agnostic of the processing layer. And so we’re doing some work in that space, mostly researching and seeing how we can leverage it.
And, also, now databases are equipped with predictive functions inbuilt.
And, so I wanna I’m I’m really curious to explore that space as well. So yeah. And who knows what else will happen this year. Right? Right?
Indeed. Indeed. You you’d mentioned tapes. And I remember having a very interesting conversation once because we had lost the tapes. Oh. And we were we were getting audited, and and we we we were cutting everything back to to to tape, literal tape. And and if you’re hearing this and if you’re younger and you’d be like, okay.
Yeah. What tape?
This is just this is just a metaphor.
This is a metaphor. You’re using the word tape for, like, a metaphor for a hard drive. No. No. The tape.
It’s a false tape.
Yes. Like like, actual actual cassette tapes. Right? Like, this is this was our archive. Right?
This is our archive strategy. And I remember we were getting we were getting audited. I I can’t I don’t I don’t remember what the audit was.
But but we’re like, okay. Well, let’s go find the tapes. And we’re like, oh my god. Where are the tapes?
And we had them we had them in a, like a storage locker with a whole bunch of our decommissioned desktop equipment.
And, like, in, like, in, like, one of the rental places that you would use if you were, like, moving and you needed to store, like, your furniture, And we we we we we found them in in a in a storage. We found the tapes in a storage locker after sending out somebody on a wild goose chase for several days, but we had to go find the tapes. So, yes, I I remember the tapes with great with with great that’s that’s hilarious. So you’re you’re leading a large team.
Right? You are heads down every day. You are helping to find roadmaps. You’re helping ex execute.
You’re helping do MDM. You have all these things, and just leading a large team would would be would be work enough. But with all of that’s on your plate, how do you stay up on all of the changes of the technology? What what what are you doing to stay fresh when it comes to all these changes?
I love that question, and I’ll tell you, I love to ask that to to everybody. So I would love to hear your how do you deal with that as well?
Here’s here’s what I would say, that in order to be technology savvy, you need to first be technology aware.
And so to me, that’s my number one plan is open up as many channels as I can to, you know, get get that information. All you need is a word, and then you dig, oh, what did that mean? You know? Get into nitty gritties of it. So I am I do tech news pretty diligently.
I am hooked on influencers on LinkedIn, YouTube. That’s how I found you, isn’t it, Malcolm? I was like, this guy looks like he knows his deal.
Podcasts, you know, as many things, as much as many windows as you can open, right, to be exposed to that sort of newness, for a lack of better word, is what I like to do.
Second second part then is once you have information or something like that has happened, now go and deep dive into, and it came. I get into a rabbit hole of things. You know, I just and I’ll give you a simple example. Deep Deepsea came out, and they were saying, oh, this is fast, and they’d only five million dollars to, you know, train it.
Inference is cheaper. So I in coming weeks, I started to look at, oh, how are they even doing this? Right? You have to ask you have to be curious and ask questions.
And in initially, I was not getting those answers online, but now you will see there are a lot of things people are preparing and putting out there. There are articles coming out, blogs coming out on it.
So I being from data science background, I am aware of transfer learning concept, where you take one model and then its learnings are transferred to another similar model, same size. But I did not know about a concept called distill distilling, which is taking a bigger model and, you know, training a smaller model.
So is that what DeepSig did?
Is that That’s what DeepSig did.
Okay. Okay.
DeepSig. And I have to learn, obviously, more about it, go much deeper, but that was a term I just, you know, stumbled across because I was curious. So how did they train for cheap? Another thing I recently found out is DeepSeek has six hundred seventy one billion parameters.
That’s not a small model.
No. It’s not.
Right? So how is it how’s Inference cheaper than your GPT three point five? I think it’s about the same billion billion parameters.
So they are they are, you know, they have they’re using the architecture where only a few parameters are sparked at it at the time based on the context. So only, let’s say, thirty billion parameters are really fired up, and that’s how it’s saving money.
Interesting. So we said we weren’t gonna talk about AI, but here we are.
Here we are. We can’t. We can’t get rid of it.
So I I I love that. You know, it’s it’s be curious, explore a whole bunch of channels. Don’t be afraid to go deep.
That that that makes me think of one thing, though. So you you you’re more technical.
I’m I’m less technical. So I was in a data leadership role for many years, but I was not technical. And I always struggled, and I I to this day, I I still struggle to a certain degree about trying to find the balance between going deep and not going deep. Because because like you, I can fall way down a rabbit hole, and it like, three days later, and I’m still looking up distillation, maybe, for example. You raise that example.
And and that and and that can be a big time loss. So do do you struggle with that at all? And if so, how do you find that balance between where do you go deep and where do you not go deep? Because there’s only so many hours in a day.
Yes. So I’ll tell you. Whenever I research, I research there has to be an objective to your research also.
Right? It’s not just the reading the whole everything.
And the objective there is, how can I how can I bring this and make it useful to my company?
Right? And so that so that’s one big objective. And second is general awareness. And if it’s an awareness factor, like, I won’t go anywhere beyond distillation and, you know, it’s called MOE, mixed something experience. I forget the word.
But I won’t go any more deeper into that because, hey, what is it accomplishing? Right?
But I will know why it is doing it. However, if there is a cause, somebody is talking about, you know, there’s a I I noticed you had another podcast on semantic layer. That is what I’m doing right now. Ah. Totally go deep into it and understand what you’re saying. I would I would do other research, all of that. So it is so purpose driven, and that’s what will determine, you know, how deep you go or not.
That is such great advice, and it is it’s advice that has worked for me. And if you would have asked me the the answer to that question, I would have given the exact same answer as you, which is have an objective in mind and and go only as deep as you need to do to solve a specific task. But if you think about it, Apurva, that’s really being agile. Right?
Like, that’s kind of an agile maybe a lean maybe more lean than agile, but it’s a very lean approach, which is, okay. I need to do this, and I and and this is what I need to accomplish this task and nothing more. But in another two or three weeks, you’ll probably need to come back and maybe learn a little bit more and on and on. And I just I just love that.
Who knows? Maybe in one year, I need to learn or not. Right?
Right. It’s such good advice.
One of my data scientists tells me, oh, you know what?
Distillation. I will know what the heck they are talking about.
And so that’s important.
It well, it is, and that’s that’s as a nontechnical person in a technical leadership role, that was my job, number one, was to make sure that I could at least speak the language, which is interestingly kind of tied to your research around semantic layers. I’d I’d and I’d be interested to talk to you in another few months about it again and to see where you are because that’s semantic layers, when you start getting into language and ontologies and meaning and context, and there there’s there’s there’s a lot there. But we we don’t have to double back on that.
So you lead a team, and everybody needs to stay up on all these technologies. I think everybody, whether you’re a leader or whether you’re an individual, participant, needs to stay up on these technologies.
How are you how are you kind of encouraging your team to do the same? Do you build in a certain amount of time for individual learning and development? Are you promoting your employees to go to maybe conferences or to be to be active on LinkedIn?
How does that play out as as as a leader from the perspective of of keeping your team, you know, informed of these technologies?
Yes. I’ll tell you when when you have a smaller team, it you know, with just with one on ones, you can accomplish some of this exchange of ideas and all.
But as as you start getting into layered organizations, it becomes hard because you’re probably not even meeting, you know, the core engineers that often. So what I like to do is bake it into the planning session.
And every year as we, you know, plan our roadmaps for all the different teams, I also have my direct reports create a upskilling plan for every single person on the team. And so we’ll say this person is going taking this particular training. I’m not too keen on having people do certifications, but it helps because, hey. Now they’re coming back with some something for their own resume. So if people want that, I’m happy to, you know, get some training budget on it.
Conferences is a big one now. We cannot send everybody to conferences. It gets expensive.
So we do that also very methodically. It’s okay. This person is San Francisco, let them go to that one. So and then, you know, in India and Europe. So we we do have and not everybody needs to go to a conference. That’s a big one.
We look for something smaller. There are so many smaller summits happening locally, so we we choose those sort of things. But really plan it into your just like your road mapping, you road map your team’s, so, you know, upskilling plan.
Another thing I’ll tell you I like to do is we, I like to put objectives, research objectives also on the team. Now I’ve carved out a very small capacity, and we research on topics. So we last year, it was fabric. Let’s look at what fabric has to offer and now come and produce the report that the all the team members can listen to.
Right?
We also did same with GenAI.
And and all I’ll do is I’ll tell them, hey.
Use these sort of tools because now that’s what we have started to, levitate towards, and create a POC on, let’s say, our survey data. Go find out action items and now bring it in Power BI report.
See if we can sort of summarize it on the fly. Now we are in business. We we are giving our customer success people some good good, insight into those comments because those comments, nobody is looking at at one, you know, one comment at a time. So let’s why don’t we create that as a POC?
And now so we did that in quarter four. Now we are taking it on roadshows and saying, hey. This is what we have done, client services. Are you interested?
We can do this similar thing on cases, give you insights on that, and so on. So so you you, you know, build out those, research sort of POCs or prototypes we build and then see if there is an appetite for it to become mainstream.
I so I I love that.
You know, one of my peers at Prophecy actually does a monthly book club where where everybody reads the same book a month, and then once a month, they just get around and and talk about that. But what you just described, like, maybe you could even take it to the like, having a kind of maybe a small hackathon even or something or where it’s like, here’s the problem. Hey. Go on. Try to find a solution to this, and then the winner gets to actually go pitch a POC to our customers.
I I love that. What you described was more of a kind of a month maybe a a research initiative where it’s this month, hey. We’re gonna go and research this and then come back and present your findings to the team.
I I love all that. You’d mentioned, you know, San Francisco, India. Is is your team globally dispersed?
Yes.
Do you have folks all over?
Yes. In all continents except Africa.
And then So how do you maintain cohesion with with everybody?
How do you how do you keep a a team vibe? Do you do you bring everybody to Detroit once a year? Or or how are you managing cohesion?
I’ll tell you. So the you know, travel is a challenge, I’ll be honest. Yeah. So we may not be able to get, people to travel every year.
But we I like to do, you know, at least annual, planning road map planning with the teams. So we come, we create murals, and we, you know, sort of brainstorm what went well, all analysis. Right? The rose thorns birds.
Everything will go into place. So we I do that quite a bit. And, also, all hands help where, you know, we we tell them, okay. This is our quarterly every quarter also, we have to revise our roadmaps. This is what is happening quarterly. This is as a whole, we are organization we are going towards.
You know, that also needs quite a bit of communication.
People forget, you know, what are our objectives here? These are your objectives and say that repetitively.
And then we also have, you know, monthly, meetups at hubs. So in Bangalore, we’ll have meetups, Singapore, California, and so on. So so, yeah, we we try to get that sort of community aspect.
We put the community that’s available there, and then the rest is online.
Thanks to the Zooms and the Teams of the world.
It’s funny. You mentioned, Rosebud Thorn. I hadn’t we used to do I did that.
I forget what team I was with, but but that was a big part of our of our planning exercise.
And I hadn’t thought of that in years until you just said it right now. Like like, what category? Is this a rose? Is this a butter? Is this a thorn? To to kinda help you understand.
You get so much content out of it. You know?
Oh, oh, yes.
This had happened as an opportunity. And I said, oh, you never thought of that. Good.
Yeah. Yeah. I I I love all that stuff. I just hadn’t thought of that in a long time, so thanks for the memories there. So in in in regards to kind of team development and and building out a team, how do how do you identify kind of the the future leaders in the team? What what are you doing to identify and build and develop the person who will take your job, and how important do you feel that is as a leader of of of a data team?
Oh, I tell you, I I take personally take succession planning very, very seriously.
And it is I also have my own, you know, sort of, it it’s good for me too, and I’ll I’ll tell you why.
I wanna make sure that I am not becoming a bottleneck in any which way for things to happen. Moment that happens, I’ll start getting calls in the midnight, and that’s not good for me or the person who’s trying to get things done. So that’s number one. Make yourselves try to start making yourself redundant.
And, so succession planning comes very handy in this.
You know, to simplify the concept, what I would do let’s say I have done a particular task for this brand new thing. I did it. I have done it for six six months, one year. Now it’s codified. It’s already.
Now I’ll give that to, my, you know, one of my leaders and say, hey. Why don’t you handle contract management from now?
Now I’m by doing that, now I’m exposing them to procurement processes Mhmm.
Finance. Right?
Keeping an eye on licensing, all those good things. Right? And I don’t have to keep doing it because, hey. You know? Somebody else can learn.
Right?
And now by keeping them you know, pushing that out of my plate on on the next person’s plate, I my now plate is empty. Now I can take something new. So I last year, I went and joined AI Center of Excellence.
I said I’ll I’ll be representing, the data community, and, let’s do it. And so I I got in. We had security, privacy, and a legal sort of pillars. We did not have an architecture pillar because everybody was learning. Right? What are you gonna say?
So I said, you know what? Let’s at least put our learnings together and come up with a pattern so everybody can learn from. And I had several meetings with, with Microsoft, and I love Microsoft. I tell you.
They are so awesome. They just give everything handy right from, you know, hosting to monitoring. Everything is there. So love it.
So, anyways, you know, the now I got time to do that because I had taken time to, you know, shift down, and train the next next line of, managers to take on the job. Couple of more things I’ll tell you.
The most time that goes out is not in technology.
My most of my time goes in alignment.
Right? Influencing people. Somebody is working little slower. So but, you know, talk to the team leader and say, hey.
Can we just fast or hey. Can you get this work done for us? The that’s not their priority. It’s my priority and the team’s priority.
So alignment is a big, big chunk of my life.
Right? It’s amazing it’s amazing how much time, you you know, all leaders but I and I I wanna say this is especially dated, but maybe it’s not. Maybe it’s all leaders, but the politics aspect.
Right? Politics. Exactly.
Yeah. Politics and sales.
Exactly. Exactly. So, so I also plan out what somebody and this is goal setting. Right? Is you have to have these sort of relationships built.
I should not even or even for our principals, right, who are, like, senior engineers, principal is a big role. They should be able to independently go work with enterprise architecture and other stakeholders architecture aspect has to be fixed. And so reduce these cycle times where we are spending so much time in going back and forth and back and forth. Seal the deal.
Be influential. These are targets I’ve actually given for my principles is you need to be able to build relationships and influence with these five, six people, let’s say. Right? With a very, very, smart smart goal.
Communication.
This is a big one, and I’ll tell you it’s all the I’m learning it too. But brevity in communicating your plan or status.
If you have twenty laundry items to say, you lose people.
Top three. No more than top three things you’re gonna say. Now you decide what those top three are. How do you categorize it?
Don’t give me twenty things, and I you’re gonna lose it. Lose people. Decision making is another one. What sort of decisions we wanna okay.
You are you are in India time. I’m in US time. You don’t have to wait for me to come in office to make a decision.
Delegation of decision making also is important, and you have to sort of codify it in the process.
So so many aspects is not just technology. It’s, you know, all these different and then business acumen is another another good one is you need to gain as much knowledge. And there, you can just name the topics.
And, oh, you need to know about Qualtrics. How are we getting survey responses back or active user, whatever that might be.
So So you’d mentioned a few key things.
You you you mentioned in at the beginning and then right at the end, which is delegation for one. So you’re you’re gonna you’re gonna see who excels and who doesn’t excel when you are delegating. Right? And for a lot of data leaders, that that’s that’s kinda hard to do because you you’re you’re giving up a portion of what could be perceived as your power. Right? So but I would invite all data leaders, to to take that great advice because you’re gonna see pretty quickly, you know, who who who the stars are and who who who needs maybe a little bit more development. And the bonus, as you rightfully pointed out, is it takes work off your plate.
So so win win win. Right? The other key thing that I heard you say is not just delegate, but also empower.
Right? And I think that that’s that’s a critical part of of the delegation because I’ve been in situations where things have been delegated to me, but I wasn’t actually empowered, which is which it could end up actually doing more harm than good because you’ll go running towards a task. And then if you’re not empowered to actually do the deal, as you said, if you need to go seek approval to get it done, well, then you’re really the the delegation was not a real delegation. You’re you’re still you’re still controlling that power.
So I lost the wall.
Yeah. Yeah. So so such such great advice there. So well, when looking back, I mean, you’re talking about some of the things you’re working on.
We’ll talk a little bit about where we’re going. You’d you’d mentioned Microsoft. Maybe we’ll talk about some of those things in a bit. But just looking back over the last year, what what are some of the things that that you’re really proud of? What are some of the the bigger lifts that you’ve made as a team? What what would you love to share?
Yeah. I I tell you, Autodesk is, it’s a very very tech savvy company. Everybody can is capable of doing their own data work. And couple of years ago, you know, we had a lot we had built silos, and there were no one source of truth that we could rely upon. And And so we have been working on creating this foundational supermarket of datasets.
And we are last year was year two. Now it’s gonna be year three, but then again, keep building on those products.
So we have all these sorts of so, yeah, that that was something we did. And, you know, as we started, we took inspiration from data mesh and medallion architecture. You name it. We made it our own. And, all that is now hosted in, Salesforce and Snowflake.
And, yeah, we have a nice governance program around it, which I started around the same time when we got, you know, this whole idea, running.
We needed a lot of governance, a lot of quality checks. But now, really, you know, as the adoption is now starting, we are seeing the rubber meet the road and, now now oftentimes.
Where is that adoption happening through? Is that happening through dashboards you’re providing? Is it happening through like, people are accessing through a data catalog? Where where are you actually seeing the adoption? Where’s the rubber kinda hitting the road?
Yeah. And I’ll tell you, we have several personas who are leveraging this. One persona is other teams who are reliant upon these base data, other other smart development teams, right, who are who are core technical teams.
They are now moving from their whatever old, tables they were looking at to now new tables, right, that we are, providing, the our tomatoes and potatoes layer. They’re using our ingredients now, which are fresh and call it high quality and so on.
Then there is a second layer of people who are in reports.
Right? Now they have to also start looking at the data source from our products.
And so and those changes are happening because now they are looking at the analytics sort of schemas, which, again, somebody else owns, but now start looking at these tables instead of those.
And then finally, there is analyst layer who really doesn’t they don’t care where the data is coming from as long as they have the buffet of objects to choose from. And that’s the last piece I’m working on now is how do we make this available to us, you know, our analyst community without them having to know join this and join that and use that table and all that? How can we make it easier and in a very marketplace sort of, fashion that all they do is pull objects in a report, Power BI report in our case, and they just do the visuals instead of creating models, semantic model in the back end.
Mhmm. In interesting. Do you have so you you’re you’re a centralized data team. Yes. Sounds sounds like you you are. Do you have data and analytics functions in each of your business units, And do they have a certain degree of authority? And if they do, how do you sync between the two?
Yes. Yes and yes.
So here’s how we have sort of segregated the duties. Right?
Any ingestion of data will be done by the teams that we have, enterprise data data hub teams.
So nobody nobody other than this team is gonna go to Salesforce or SAP to get the data.
Okay. Okay.
Right? So we’ll do all that ingestion, and we’ll give you the now, for example, orders table. Orders we have five, six different channels, let’s say, from where orders are being placed. We wanna combine all that data now. This is a solid orders table, good for the entire organization.
Now you use it. Now the analytics community can use it the way they want, and you don’t want to tighten everything. You know? You want a little bit of liberties to do their own sort of, development and do their own thing. So that’s go ahead and do that. Right? But ingestion piece and making those products available will be solely this team.
Okay. So you are acting, in essence, as kind of a central broker or or provider of all the data, but you are doing the governance.
You are doing the quality. You’re doing the ETL. You’re creating the marketplaces.
But where where the decentralized or or more federated, probably more appropriate, data teams are the ones that are building the dashboards, that are doing Power BI, that are maybe even doing the data science. That’s a good question. Where does where does data science sit in both places?
In both places. Yes. We again, data science is, you okay there?
Yeah. I’m good. I’m good. I pardon me. I’m good. Thanks for checking. I got some water.
So data science, again, can sit wherever it want. It sits in application layer. It sits on the data side. Again, you know, data science and analytics is something we have said.
Okay. Yes. Let’s let’s democratize it. Let people have, you know, their own power autonomy to do it because it’s so close to the business.
Right? The stakeholder wants to see the report in exactly this way.
You know, the central team cannot we don’t care. Do it.
Okay. So from a data science perspective, you you mentioned, you know, Lakehouse. You mentioned, you know, Snowflake.
Are are you worried about data scientists doing duplicate work when it comes to things like quality, MDM, maybe even governance where they are just are they gonna be access you said you can’t access raw tables.
You’re gonna be using the ones They are fresh potatoes and tomatoes.
Use it for your recipe, and that recipe could be seriously, that’s how I like to tell it. Tomato. That recipe could be your analytics recipe, or it could be your, data science ML model. Right? Just use it.
But but the classic data management task, like like quality, for example. Like, let let’s just say that you’re doing some sort of good quality task or even basic e ETL.
That’s happening in the centralized group before data scientists are touching the okay. Okay. You’re you’re nodding. Okay.
Definitely. And we what we are trying to do here is the technical metrics. We’ve and as per our governance program, we have established technical metrics mandated for everybody who’s producing data. If dataset is in production, it has to go through all the mandated technical sort of tests.
And then business metrics is another layer we are trying you know, we are again we are getting in the practice is define those because businesses are gonna say, oh, look for the nulls or look for if the, you know, state is m, you know, Michigan, MI, and, you know, different flavors of it. It has to be business are gonna ask you that. Business might say, oh, you know what? All the survey data that’s coming, in the table, I want to connect it back to some sort of a customer and give me at least ninety eight percent of connectivity between those.
Now that’s a business metric. So if now it falls down to ninety percent, now the team has to look what’s going on and then let the business know, oh, you know what? Something has happened or people are not putting their name, whatever it might be.
Yeah.
So those data scientists aren’t going straight to source. They’re come they’re coming to you. Okay. Love it. Yes. That’s that’s I’m seeing that more and more.
Just presented a use case a couple of days ago with Gartner with Raju from Lexmark where they’re using Databricks, but they’re using the exact same thing.
And a lot of our larger comp customers, it’s the exact same situation where previously, the data scientists were going to the source and were doing all of their own DQ, all of their own transformations, all of their own governance, even applying, like, their own definitions to anything however they needed to. And so many companies are trying to get away from that and put some controls around what happens in the data science world. So that’s okay. That’s interesting.
Let’s let’s save the the AI goodies for for last, or maybe maybe we can’t. I I don’t know. But, since we just talked about last year, what are some what are some of the things that are that are top of mind for this year’s road map? Where where you where are you focused for this year?
This year, I want to really start leveraging some of these Gen AI, you know, prototypes we had built. I want to build on top of it and try start start offering it to our business partners on on the data we already have. We because we specialize in customer success, my team is, solely customer success and client services space. And so I wanna bring some of this these capabilities in our space.
So that I’m really excited about that. We are obviously gonna keep building our foundation foundational layer with data products. We have tons you know, that’s our main bread and butter.
Mhmm.
Yeah.
So when you talk about the the you know, taking the next step with GenAI, what what does that look like to you? Is that are we talking about kind of trying to do some of your own fine tuning on an open source model? Or you you’d mentioned Microsoft before. Are you thinking about maybe more complex rag patterns on top of, like, a like a, you know, an open AI? What do do you know what flavor it’s gonna take this year and and how you’re gonna approach it?
Now let me tell you, I’m on the enterprise side. I’m not in the product side. Now Okay.
If you ask somebody on the product side what they’re doing, they’re gonna tell you a different answer.
The customer facing stuff, you mean?
Yes. Right. Okay. Okay. Products.
Yeah.
There’s a lot there’s a whole different flavor. Yeah. My customers are my Autodesk employees. Right?
Yep. Yep. You know, right, finance, marketing, sales, and so on. So how the way we are trying to use, AI or Gen AI in our space is to cater to them.
So now, I gave you an example of survey responses. There’s tons of survey response comments that are coming in. We want to highlight what action items are there, categorize them, and say, oh, these are the flavors of comments we are getting. These are the themes.
And here’s here’s the action item, that you can follow. Now I wanna say, okay. Let’s go to and now all this has happened on the data side. Right? We’ll actually go in database. On the reporting side, now because of the Copilot, there are some, the features in Copilot, the the visuals that you can pull in Power BI and say, okay.
North America, this particular segment of customers, show me what’s, how are they feeling, and what are the action items I need to it’s gonna summarize it on the fly, the actions, the the comments. And so now if I’m the customer success manager for that region, I now know, oh, this is the sentiment, and, I should go and now take this forward and say, oh, people are having trouble, downloading our our, tool our product.
There is see what’s going on here. Right? And they can curate that message to that team, email them, or or set up a meeting. It becomes very easy, right, because you’re highlighting all these points.
So so that’s the intent is find, you know, sort of prescriptive prescript prescribing the, you know, what to do next. That is the intent.
So I suspect forgive me if I’m going a little too deep here. But so you’ve got a bunch of customer feedback probably sitting in long text strings, I assume, in a CRM or multiple CRMs.
You’re gonna deploy a custom Copilot perhaps on top of Power BI, maybe not. Doesn’t matter. But you’re gonna build a custom Copilot. Are you using Vue, been playing with is it OpenAI Studio or some other tool for for building the Copilot? Have you played with any yet?
We have so, we have brought all the data into Snowflake. That’s right. So it’s sitting in the database.
Second layer would be running action item, creation for each comment. Right? And that will happen leveraging Microsoft’s OpenAI.
K.
Simple LLM.
There is no Yeah.
Yeah. Nothing. It’s so simple. Yeah. Right? Categorize it and continue creating that content in the database.
Step two will be on top of that Power BI, which is equipped with Copilot visuals, which can, on the fly, summarize things for us.
That’s the ecosystem.
When when I’ve played with this, open AI studio, build a Copilot, I was it was so simple. I I was blown away by how simple it was. I you know, able to to pick a source to ground the behavior of the model, and the source could have been is is just like some text blob somewhere.
Right? And where that is being used to help ground the behavior, that could be all the data around all of those customer interactions that you’re talking about.
So the the lesson here to anybody who’s listening is if you’ve been kind of dragging your feet on AI or dragging your feet on GenAI, there there are tools out there to be up and running. Now this is an internally facing, you know, use case.
So, you know, I suspect you are managing expectations appropriately internally and saying, hey. We’re we’re this is a POC, and we’re learning as we go. You’re you’re laughing. So it sounds like this is by design, this internal focus first and to see how things work. Yeah?
Absolutely. And and that’s where, you know, that’s what my job profile is, is make sure we are we have all the data and insights and prescribed, you know, knowledge that we can part to these people so they can go and do their jobs well.
So Are you concerned at all about the, for lack of a better word, quality of of that data?
And and and if yes, are are you thinking, you know, in in the coming year about how to to overlay a data quality process on on top of that?
Because this you know, I was just at the Gartner conference, and everybody’s talking about AI. Yeah. And they in the keynote speech, like, this is where there’s, like, five thousand people in the room, and everybody’s looking at the giant screen. There’s this one stat that came up and said data quality is is is perceived as the number one inhibitor of companies in their adoption of GenAI.
And the use case that you just described, fairly simple, I I would think, but I I would still wonder, okay. Assuming that somebody’s typing something into a CRM that is describing a customer experience or describing their experiment experience, I suspect there still could be concerns related to data quality there.
Are you thinking about that?
Absolutely. And this is what you know, these are the type of monitoring metrics I brainstormed on with Microsoft the entirety of last year. Mhmm. Once the model is in production, how do you know it’s doing its job? Right?
And so couple of things. One is thumbs up, thumbs down. That’s absolute mandate. Right? Yeah.
Because you you wanna hear. Otherwise, you’re reading every literally everything. And what if becomes millions of comments coming, then what are you gonna do? Right?
It’s impossible to scale that way.
So do that. Second is, and now this was very specific to RAG is groundedness. How much to calculate hallucination. Right? Is the summary that the LLM is providing, does it have a backing of the source?
And so what what happens in a reg model is it will let’s say I’m asking, what’s the policy for, you know, vacation?
It will go and check where the vacation policy are. It will get that extract out of the vector database and then use it to summarize it. Now what I do is I compare this and this to see how if they are matching or not. If they’re not matching, this guy’s hallucinated because this is my data. Right? And so that’s a metric that, is given out of the box by Microsoft.
All you need is call an API and say what’s my crowdedness cord, zero or one. So easy.
Yeah. So that’s interesting.
But the only way they could be speaking of rabbit holes, the only way they could be doing that is by using AI to to assess.
AI as a judge. Exactly. Yes. Exactly.
Okay. Alright. That that okay. I need to I need to think some more because that that seems interesting.
Alright. We are now getting into agentic AI is one AI is determining the output of another AI. You know, LLM is determining the input of another LLM, and now they are working together. That’s another thing on my list, by the way, is to come up with some use cases for agenda AI that we can use in data space.
There’s such a paradox there, Perva, because I would argue that at scale right? Like, another stat that Gartner shared was that eighty to ninety percent of all corporate data, all data is unstructured. Right? It’s sitting out there. It’s on SharePoint servers and PDFs and video files, and who knows? It’s it’s all unstructured or arguably semi structured, you know, maybe HTML, XML, but but it’s all out there, and it’s mostly ungoverned.
Right? Like, nobody’s checking to see if the Word document that is stare share put on the SharePoint site that talks about, you know, maybe customer frequently asked questions or or who knows?
But nobody’s really checking from a data quality perspective. Is that stuff accurate? And I think that at scale, the only way you’re gonna be able to do that is to use AI.
Right? It’s for you you said for AI to be the judge, and it’s interesting. You would want to assess the quality to make sure that that data is good for AI, but the only way to do that is to use AI. I mean I mean, I don’t know. Yeah. It’s my head explode.
But I’ll tell you one thing.
It’s people say that, and that’s the truth, that a lot of unstructured data is there more so than structured. But most of the usable data is is structured. Right? What do people want to know? Well, how is my company doing? How many orders do we pay? All that is structured.
Yeah.
And so people are happy and getting their, you know, sort of inputs to to make those decisions they are making. A Wiki page is not really gonna help me that much no matter how wonderfully quality you know, wonderful quality aspects I get to it, and that’s why it’s ignored.
Right.
Right? So that’s just my two senses.
You know, you have to really find the reason why somebody should care, and then once you find it, then you find what’s the It all comes back to a business case.
Back to business.
Always comes back to a business case. I think that’s that is a great way to end. Apurva, thank you so much for spending an hour with us today on a Friday afternoon. I really appreciate it. You said some fantastic advice, some fantastic insights.
Thank you for, for joining us today.
Thank you for having me, Malcolm. It’s always so wonderful speaking with you.
Alright. Well, to all of our listeners, please don’t forget to subscribe and to click the speaking of thumbs up, thumbs down. Hey. This matters. The robots need to know. Thumbs up, thumbs down. If you could do that, we would appreciate it.
We do this every two weeks where we are sharing insights from data leaders around the world about what you need to know to become a better data leader, a better CDO, and to build great teams. So thanks, Apurva. Again, we will see you on another episode of the CDO Matters podcast sometime very soon. Thanks all, and bye for now.
ABOUT THE SHOW
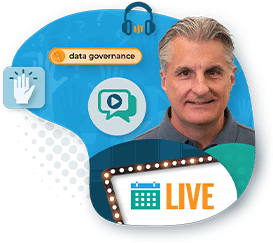