Episode Overview:
Episode Links & Resources:
Good morning. Good afternoon. Good evening. Good whatever time it is, wherever you are in this amazing home planet of ours. I am Malcolm Hawker. I’m the host of the CDO Matters podcast.
I’m today joined by Jessica Talisman, who’s gonna talk with us about knowledge management. We’re gonna get into some fun stuff today. We’re gonna talk about the intersection of library science into data and knowledge management. We’re gonna talk about ontologies.
We’re probably gonna talk a little bit about AI. Can’t can’t can’t avoid AI. It’s kind of the elephant in the room. Jessica agrees. Okay. It’s fantastic.
And we’re gonna get into some very, very interesting topics today because I think that knowledge management is something that we all need to start figuring out as data and analytics professionals.
And there are there is a path already tread, Jessica? Tread?
Yeah. Yeah. Paved. Paved.
The past test of tread. Treaded? Tread. Tread. I don’t know. Tread. Yes.
Tread.
Yeah. Yes. I have a master’s degree as well. You you you probably can’t tell because I I speak like I just learned English.
Yeah. And that’s cool. I I blame my Canadian roots. Jessica, hi.
Hi. Thank you for having me, Malcolm.
Oh, well, thanks for being here. So Jessica and I were together not this past weekend, but the weekend before.
You’re gonna see this in April, at which point I mean, this is already several months in the past. But we were given the honor of presenting to not together, individually, but at day to day Texas in Austin a couple of weeks ago. And I was lucky enough to attend Jessica’s presentation, and I was like, yes. Yes. Yes. Yes. I’m sitting and I’m sitting, and I’m like, I’m I’m I wanna get up out of my chair, do the wave, high five, and all sorts of other things because I think what Jessica has to offer is incredibly valuable.
Day to day Texas was fantastic.
And I immediately connected with Jessica on LinkedIn and said, hey.
Will you share your wisdom with our audience? So there you go. There’s the setup, Jessica.
I’m blushing.
Okay. Alright. Well well well, good. And then hype hype hype hype has successfully delivered.
Why don’t you spend just a few minutes just kinda describing at a high level, and then we can we can dive down into some of the specifics related to your presentation. But why don’t you share with the audience just kind of at a high level what you presented at day to day Texas and why you think it’s important to data people?
Sure. So the the title of my talk was we are all librarians. And this was a tough one to arrive at in terms of presenting the idea because, of course, I don’t want to offend librarians who work very hard within their field and to gain the qualifications that they have. And they’re on the front line every day making data, information, content accessible, reusable, maintaining repositories, what have you. However, something that I’ve observed in just from my day to day work and being involved in different aspects of of the data field is that we are actually all being asked to be librarians. We are all being asked to care for research, information retrieval, structuring, organizing assets.
And the core principles and tenants of librarianship are about organizing, the discipline of organizing, and methodologies for making information findable, retrievable, accessible for humans and machines, and that includes AI for that matter. So, that is the core those were the core concepts that I presented.
And I also presented ways to get there.
So, in fact, it’s it’s not rocket science, but it does take patience and there is a process. There’s a codified process in how we organize and structure information to benefit humans and machines.
So why would that offend librarians?
Well, librarians will tend to don’t work, I should stipulate, who do not work in the corporate space.
The librarians yes. So, you know, it it’s interesting because there’s a little bit of a divide between those two worlds. There are data librarians, but the librarians that are in the front lines every day of academic libraries, of public libraries, of school libraries, they live in this ecosystem that’s about making information, data, knowledge accessible, retrievable, and interoperable within the outer world.
It’s outer world versus inner world.
Oh, okay. That’s interesting. I thought I thought you were gonna say that there’s more kind of, like, from a librarian perspective, more more of a kind of a public service mission.
So I’m not interested in a profit motive.
Yes. There is a public service because it is does this something that’s interesting about libraries in general, no matter the type of library? You know, libraries have been around really for more than three three thousand years. That’s a pretty long history in the data space if we think about it in those terms. And the whole idea of having a democratic accessible place where all people can access information and data where conversations can happen. I mean, I don’t know the last time you were at your public library, but that yeah. Exactly.
And so right. And so librarians provide access to the Internet for kids, for people looking for jobs. They have resources for job hunting, for resume building.
Libraries have fab labs. I don’t know if you’ve got, you know, makers’ labs or makerspaces where you can learn three d printing, things like that. So as a service, like, information, data, knowledge takes so diff so many different shapes and and formats for that, you know, in terms of how humans in general need access to information and knowledge.
Librarians supply and have been the stewards of cultural institutions and cultural technologies since three thousand plus years ago. So it could be anything as simple as from paper.
The that’s a technology.
Paper was you know, and the idea of codifying ideas and knowledge or information and making it accessible, technology has progressed, and librarians have stayed lockstep with those progressions, adapting and adopting different methodologies for being able to, again, make information, data, knowledge accessible.
Well, as much of the technology may have changed, some, I would argue, and this is one of the reasons why we’re talking today, some of the core challenges remain the same. So even though that long form text, that book, may be digital, Right? It’s still not in a format that is easily readable by a machine per se. Right.
Exactly.
So so that’s to me, that is the, like, why why library science needs to matter more than ever before Yeah. Because eighty percent of our data this is this is me. I don’t know this to be fact, but the Pareto generally applies, is probably gonna be out there in a lot of these other formats that that do lack structure.
Yes.
So if you were hired to by somebody to say, okay.
Alright. I’ve got this corpus of data, knowledge, narrative, stories. Who knows? I’ve got all this stuff out there.
Maybe it’s medical records, in PDF files. May maybe it is actual novels somehow, some way. Maybe it’s medical records. Maybe it’s, who knows?
And you were hired by somebody to tell okay. Help me make sense of this. Is there a framework that you that a librarian would typically apply to go about solving that problem?
Sure. I mean, I don’t wanna get too much into the weeds because there is librarian a librarianship involves a lot of abstraction and concept models. So I to be fair, I don’t wanna confuse anyone listening, but there is a core conceptual model called, which is functional requirements of bibliographic records.
And these are formatted and standardized records that usually assume one or two machine readable formats and are made available widely through these linked data landscapes that serve as the core of of the information that we have on the Internet, which happens to also be what is primarily used for training data for AI or LLM models.
So if I go in, I’m gonna look at I’m gonna I’m gonna perform, for example, content analysis, corpus analysis. You can think of it that way. You’re gonna find all the different file formats, standards, anything that exists within an institution or an organization. There’s always a starting place, and you have to put your finger on that starting place. What is the state of these assets or content or things right now? Are they machine and human readable?
When you do content analysis, you’re looking across all the different machine readable formats and files. You’re looking at how people access, where people access these, and you’re also looking equally at how people access, which also involves machines.
And then you have to mitigate or you have to bridge the gap between the human and machine accessibility to make sure that both of those actions are are supported.
That is the primary focus.
Then you look at how these things are findable, the actual, vehicle for findability, for example, for machines and humans, how it’s packaged, how it’s described.
And that’s where we get into vocabularies, and that’s a much longer answer in terms of, like, that, you know, that idea of how we how we clean, transform, process, structure, and inject and imbue meaning so that we can have a really robust, elegant system that can handle all the different aspects of search and findability.
So, obviously, discover you’re saying findability. I yeah. I think interchangeable with discoverability, maybe even even the next step would potentially be profiling. Maybe there’s different word for it in knowledge management there. But so that so that’s key. You’re gonna need to do this at scale, quite obviously, you’re gonna need a tool to do this at at at any sort of scale, and I suspect there are, you know, widely available to well, I know in the data management world, there are there are tools available to do this.
So I go out there and find a whole bunch of stuff, and let’s say I find a whole bunch of web pages. Let’s go back to the GenAI example because I I think it’s a perfect one. There’s all these web pages out there where the data is sitting in HTML.
Arguably, reasonably, it’s got some structure, right, in in terms of of HTML. HTML.
You know, semi structured at the very least. But I want a I want a machine to try to make sense of what’s there and try to put some structure to that. One of the things that I loved in your presentation, when you when you presented this kind of this kind of four step I think this is what you were calling the on is this the ontology pipeline? Where is it?
Ontology pipeline.
Okay. The ontology pipeline. Trademark.
Yes. Trademark. Copyright.
Where where you start from those controlled vocabularies Yeah. And and and work your way towards the end of that process. Why do you why do you describe what that process looks like?
It it’s something that is often skipped.
We hear a buzzword or we know things need to be structured, and we’ll skip to the end step of, for example, an ontology. That is something more common than not within the knowledge management space or information management space.
And what’s interesting about that is how can you structure so so understanding what each of these aspects, each of these stages and steps involves is critical because they’re incremental and there’s dependencies between each step. You cannot progress from controlled vocabularies to metadata standards because, otherwise, how do you know what you have or how you’re gonna describe things? It’s just an unknown. It’s a black box at that point. And then if you’re going from metadata standards and and the next stage in the maturation is looking at and it’s interchangeable. This is the one thing that can tend to be loose as it could either be a thesaurus or a taxonomy.
A thesaurus has associative relationships within it. A taxonomy has hierarchical relationships within.
So I personally will go to taxonomy because I want the hierarchy before I start extending and creating those relationships outside of the parent child relationships.
For many people and many systems, that may be enough to stop at taxonomy. You know, we don’t need over overly complex systems. It depends again, and then this is such a a line, but it depends on the use case. It depends on the application. But if you simply need a classification structure by the time you get to a taxonomy, that may be enough.
But if you want to take it a step further and you go to the source and you start start to create relationships across a knowledge landscape.
So it’s simply something that x is related to y. We have created a relationship.
We haven’t defined what type of relationship other than they’re related.
The final stage is ontology, and the ontology has more dynamic extended relationships that break the boundaries of parent child, break the boundaries of just broad associative relationships between things. It’s more of a complex model, but it needs those first steps. It needs the controlled vocabulary, the metadata standards, and it needs the thesaurus and the taxonomy in order to mature to a more complex robust model that because because and this is key. Ontologies introduced logic.
That logic can break, and that is the most important thing. So if you haven’t thought about how you’re gonna reconcile similes or synonyms, if you haven’t figured how you’re gonna reconcile acronyms with the spelled out version of that thing, you’ve introduced messy ambiguity with the ontology, and you run a really high risk of that logic break breaking.
Now the thing most people don’t realize is once you have ontology, you have a knowledge graph.
That is not overly complex. You’ve arrived.
When people say knowledge graph, are we talking about the graphical interface? Are we talking about the pretty picture?
Or, you know, are we talking about the whole knowledge management system, which are those steps that I presented? Because you should be able at the end to query your knowledge graph and find the taxonomy, find the code controlled vocabulary, find the thesaurus.
You should be able to discover all those layers so that if something goes wrong, something’s broken, guess what? You can fix it.
So okay. Just to recap because there’s there’s a lot to chew on.
There’s a lot.
Yeah. So so I I have been tasked to classify data in a highly unstructured way or or or semi structured. It doesn’t matter.
And what I heard you say was starts with control vocabulary, definitions.
Yes.
What are your words? Yes. What are your terms?
What what what what are the what are the words? What are the terms? Then you also mentioned metadata. Right?
The things that describes the things. Right? Is it is your I assume your definition of metadata is the common. It’s the things about things.
Okay.
Yes. Yes.
Then you went to thesaurus, which would be how things relate at a definitional level or or similar at a definitional level. Right? Yes. Mhmm. Yeah. I’m I’m there so far.
And then you went to a taxonomy, which is is a I’m I’m I’m struggling between physical and logical, and I think it could be either. But there but there the the relationships between these entities that are defined within the thesaurus. Right?
As parent child. Yep.
Yep. Genus, phylum, kingdom, you know Yep. These these things. And then you went to an ontology, which is more of a conceptual map of how things conceptually might relate to each other.
And then you codify that with the ontology. You’re defining it.
Okay. What you said was is that we have a tendency just to jump to the end, and let’s go build a knowledge graph. So I’ve got really smart data modelers out there who can go define the nodes and define the edges and the relationships.
And then so I’ve got my kind of my my graph data model, and then I’m just gonna run the knowledge graph and poof, here you go.
And what you’re saying is is that if you miss steps along the way, you don’t do the diligence to make to do the definitions. For example Mhmm.
Well, what you described is the ontology could break, but I suspect I suspect it could break regardless if you had things well defined or not. But are you giving your example, like, when when it breaks, is that a situation with a word like bank?
Right, or fire? Yep.
Yep.
Is that an example of where something would break even though you have fire defined multiple ways? Right? You’ve you’ve got it as termination of employment. You’ve got it as the thing to cook our food. Is that an example where something would break?
Yes. Because the type of relationship, that’s what where complexity Okay. Complexity is introduced with ontology because you have to resolve and manage your entities. Everything’s an entity when you start with ontology, And then you get to define them as classes, properties, attributes, relations, like event structures, whatever it is. But you cannot assign those roles, those jobs to the entities until you define them. Because, otherwise, what’s the difference between fire and fire? Great point.
Yep. So so that’s I I find that really interesting because in my world, often people start at the beginning and they end there.
Right? Meaning, they will they will define a customer, and often they will say, here’s our definition of customer, and it will just be a single enterprise wide definition of customer that is only really relevant in one context.
And and it that’s typically the c suite or whoever’s paying the bills or whoever has the most power in a meeting or whoever gets to complain the loudest. This is how we’re gonna define a customer. But that definition of customer is inherently and necessarily contextually bound.
Yes.
Right? It’s it’s bound within the context of this process or this workflow Yes. Or this report, which is inherently a relationship. Customer is related to a product in in this way through this ontology, through this experience. And if you’re just defining customer one way, you’re missing the entire picture.
Yeah.
Yes?
Yes. Absolutely. Because there should be an the ability to support multiple definitions of the same thing in context.
The context when you are in when you are defining context, that stage happens truly at the ontology stage.
If you have multiple ways to define customer, then you capture that in your vocabulary and not to, to make things too complex. At the thesaurus stage, you can take customer and put parentheses after each definition of customer, assuming that there’s multiple, and disambiguate by attributing or showing or having some sort of signal of how this customer is different than this other definition of customer.
Organizing, structuring, and defining that in a flatter structure or a simpler structure like taxonomy or thesaurus is much easier than trying to do that at scale in an ontology because by that point of the stage, you may have thousands of defined concepts.
Right? Yeah. Much easier to work with that really nice robust defined set than to go to the end and be like, okay. Let’s define these here because Yeah. The logic will break. And and another thing that this extends to not to introduce too much complexity again, but the thing with AI is that LLMs are trained on the web.
So often when you have this is our internal definition of customer. Customer. Does that match how it’s defined in the outer world in the Internet? Does this match definitions?
Because, ultimately, the the tricky thing about AI is you’re also having to reconcile your internal definitions of things with the outside world.
Exactly.
So So I I there’s there’s there’s so many similarities here even though what you described was the kind of the librarian’s process for classifying things, when we talk about customer and and ambiguity around customer, the data people out there are screaming, well, that’s a data model problem because because customer is an imperfect way to articulate the thing you’re describing. Because the thing you’re describing is probably a person.
It’s a person and a relationship. It’s it’s a combination of a person plus some sort of business relationship. Maybe today, maybe in the past, maybe tomorrow.
That’s probably prospect. But it’s customer is in and of itself is not the lowest level of animacity that you could get to in describing the the core thing, which is a person probably or a company.
So I do see an analog here with with effective data modeling in how you go about that control vocabulary.
Customer is gonna be part of the vocabulary. Don’t get me wrong. I’m not suggesting that it’s not. It needs to be defined.
But it seems to me like there is benefits here from breaking things into their the the kind of the smallest component pieces. Is this is am am I right?
Yes. Yes. And so, like, you could theoretically have a controlled vocabulary that describes customer and another controlled vocabulary that describes people.
Yeah.
So you could create multiple controlled vocabularies that mature into your thesaurus and taxonomy.
And then the fun begins with ontology and connecting those things. Or if you think about it this way, remember, thesaurus creates the associative relationships.
So you start by connecting the vocabularies using a thesaurus.
If you think of a few I’m gonna give the example of a book, like an actual book. It it could be any format. It could even be digital, but there’s an index.
Yeah.
A cook a cookbook or a recipe book is a great example of a thesaurus at play. Because you may look up that recipe, and I’m gonna depart from from the customer example. If we go to, like, a recipe book and you’re like, okay. I wanna know all the recipes for chicken.
And usually, italicized and dented underneath, it’s gonna have please see or see also, and it’s gonna tell you where to look for that. Those are equivalent or associative relationships. If you’re looking for this, look here. It’s the same thing.
You’re looking for chicken, chicken recipes. All of the chicken recipes are here. And so you’re going to follow those associative relationships to follow the equivalencies or to reconcile. When we talk about entity reconciliation. We’re looking for, first of all, what’s the same and what’s different.
Well, I’m I’m smiling because that’s something near and dear to my heart in in the MDM world. We we we do that all day every day.
I I I feel compelled to to this point for all of our listeners just to to give the why should I care, speech. Now this this seems this seems interesting, and and maybe so far, I’m listening and I’m saying, okay. Well, that’s that’s interesting, and you you’re suggesting that I should become more of a librarian. Why?
I I think the the answer to me is is that there’s all this data out there in in very in various various forms of of structure, and there’s a ton of gold in them, Dharrhills.
And we have a whole bunch of legacy processes we use to manage and govern data, including things like checking its accuracy and its quality, enforcing specific standards related to it, enforcing how people interact with it even. Right? How do I find it? Right?
So there’s a lot of reasons why you should probably be thinking about this. The greatest one being, if you want to apply governance, I’m just loosely saying governance. This is this includes things like data quality. It even even things includes things like data access, the findability you were talking about.
If you want to start looking at all that data because there’s value there, I would argue you need to if you have any aspirations to to use that data to start informing LLMs.
Right? If you want to build a chatbot, a customer service chatbot that uses your customer service FAQs that are probably stored in HTML, that are stored somewhere. Who knows? Right? That maybe may or may not have been updated any anywhere anytime in the near future. If you want to start deploying these chatbots and using LLMs to consume that data, you need to make sure that data is accurate, is consistent, is trustworthy.
Yes.
And the only way you’re gonna do it at scale is using machines.
Yes. Hundred percent. Hundred percent. And you don’t know what you have till you know what you have.
Like, that’s the other thing is understanding holistically what the landscape looks like and being able to account for what everyone has. Now something I see very interestingly people are okay. So within the machine learning and AI space, you mentioned chatbot architectures. Classic chatbot architecture question banks.
Yep.
Right? And that is an example of, again, you are hard coding or codifying.
These are the questions, these are the answers, user query comes in, you map it, or you let it Like a decision tree, you mean? Like like a decision tree.
Yeah. Right. K. If you have a taxonomy to support that, you know, that becomes much more efficient, but still it’s very constrained.
It’s not dynamic, and I would argue that’s not really I AI yet, at that point. So the reason we do this is to create a more fluid dynamic ecosystem, knowledge ecosystem that breaks out of the constraints. Most of the reasons we go to those question banks, which is a very sort of antiquated architecture for chatbot. And and one of the reasons we do that is that our data systems are also flooded with syntactic data.
Right? It’s not structured in a machine readable format. You can’t you can’t assume meaning. I mean, you can as a human or as a person working with the data.
You’re like, oh, the SQL statement makes sense. But does it make sense to a machine? Not really. Because it doesn’t it’s not in your it’s not within it’s it’s not machine readable.
That is not machine readable from an LLM type standpoint or from machine readable search and and findability systems, like any sort of machine readable ecosystem. So the goal the secondary goal is that these processes that I talk about, in these steps also prioritize the structure so that it’s it’s readable by both humans and machines.
So that is one of the key aspects. And so the dynamic you also have to break out of the constraints of table tables. That’s another big one.
Otherwise, yes, you will end up having to implement that type of chatbot question bank architecture with hand mapping. You know, you see the craziness like hand mapping of of mapping and hard coding user queries to SQL queries to question banks and, you know, these really complex architectures that constrain models and constrain systems so much that you end up with a very a very simple and narrow use case where, again, it’s it’s just I mean, it’s logical, but it doesn’t really have the ability to scale and serve multiple purposes and help to imbue knowledge information and knowledge and context for machines.
So we just described a let’s just call it a framework. I I like the ontology pipeline. Okay. We just described a process that you could use to start applying more structure to that unstructured data so that you could allow your governance initiatives related to that data to scale, to support it Yes. To extend the governance functions that maybe you have historically limited to rows and columns or limited to your CRM databases, your ERP your data lakes, warehouses, and extend it into your SharePoint servers potentially Yep. With with the right tooling.
So there’s that. But what do you think about you know, I I see a lot of people, and I saw a couple of demos of this at day to day Texas that were kinda kind of a little mind blowing.
What would you say to me saying, well, why can’t an LLM do that?
Right? Everything that you just everything we just described, is that a viable use case to say, hey, LLM.
You could build me a taxonomy for this.
Right? What what would you say to that?
You know, I think LLM’s partners or tools are great. You know, I presented at Data Day Texas tool, an open source tool called OpenRefine.
Yep.
That has eight yeah.
Right? And it has eight different clustering algorithms. So I will I will say that I think it’s important right now, and this is just I’m not gonna get on the soapbox. But if we look at the environmental impact and cost of using LLMs, it’s pretty extravagant to use an LLM for that given the output.
The other example that I included was the idea or the category of accessories.
That’s something when you give LLMs, you know, your data to try to structure, it’s gonna come up with its own ideas of categorization based off of your underlying data. Say that that’s not well defined at all.
So you’re asking the LLM to infer or to imbue meaning into your data and structure it accordingly as a hierarchy to assume who your sister is, who your mother is, like, all of the characteristics about you.
The problem that tends to happen is that that assumption can tend to either paint data in the quarter corner.
LLMs do not have insight into your data other than what you’ve just fed it and asked it to classify.
So accessory as a high level category with everything that could be faceted below or categorized below is very ambiguous.
It’s already ambiguous.
So the idea of taking a primary step and using a tool like OpenRefine to use one of the eight clustering algorithms to figure out what the themes and the groupings looks like might be a better step preprocessing step before then handing your data to see how LLMs are gonna structure because you’d be surprised. I think that we are throwing too much power at at seemingly more simple tasks.
And so I’m I I do challenge the idea of using LLMs as a tool for everything. Is it too much? Is it not appropriate? Is this the right place to insert LLMs? And, also, if LLMs then structure your taxonomy and then you use that as you as the word or the scripture for your organization and how you structure data, then you’re also using AI generated.
Of course, you’d need to process to make sure that your taxonomy is not AI generated, but you do have to make sure that it also validates, that you’re not introducing recursive loops and relationship clashes in your taxonomy structure.
And another thing maybe to consider, this is a I had this talk over a, probably multiple cocktails, at day to day Texas, and I will forget I forget the gentleman’s name, but he was he was presenting talking about data modeling.
And I I I was being a little provocative as I typically am and saying, yeah. I think we can automate data modeling. And and he gave a very, very cogent response to that of maybe some, but we only know what we know today, and humans are actually really good at thinking into the future and what we might need in the future.
Exactly. That’s a great point. Because your coverage model, that’s called a coverage model in the library and information space, is that after you do your content analysis or your your asset analysis, you figure out what the balance like, it’s gonna be heavier in some areas. If you think of a CRM system, you’re gonna have areas that are more weighted or heavier and more robust than others that maybe aren’t so well defined.
So, you know, looking into the future includes covering things that maybe aren’t represented within your system right now, and that’s key. Because if you’re gonna pick up signals about your data, you often have to model maybe what’s not you have to complete the picture.
And that’s something that LLMs cannot do. They cannot really complete that picture because they can’t totally see into your organization and make sense of it all to complete that picture. Say that say that your your company is gonna expand into a new area or they’re projecting to or your company is gonna offer new content type or something as as simple as that. You have to have a way to extend it to make it scalable and extensible, and that’s really important. And so that’s where I go to, we’re throwing too much fire at the problem.
And that simpler there are really robust it’s still clustering is still machine learning. Why do you have to ask an LLM to do it? Like, this tool is super simple. It it’s not rocket science.
Well well so that’s it’s interesting that you should just say that because this this this touches something dear to my heart, which is just well, let’s just call it matching. You could call it clustering too. That’s fine. Entity resolution, putting putting like things into into like buckets, creating clusters of like things.
It’s it’s rather interesting because there there are many in in that world of of entity resolution matching clustering who who think that LLMs are maybe maybe a good way to solve that problem.
And I think it’s very much a reaction to the fact that most of the algorithms we’ve been using to do that haven’t really changed much in twenty years. Right? Like, right, like, they’re all they all look largely the same, like this Levenstein distance algorithm, Jared Winkler, Soundex, Phonex. Like like, all these things haven’t really changed that much.
And I think you could I think you could argue that that there’s a reason for that potentially. Mhmm. Right?
And that broken.
But I I think the problem is is is that it’s not perfect, but nothing is.
Nothing is. Right. Nothing is.
Right. Like, the I’m Go ahead.
I mean, it it reminds me of was it the was at Sesame Street. Some of these things are not like the other. I mean, this is something that, like, comes into the picture, you know, from the very beginning of brain development. It’s how we form our cognitive models in our brains. It’s how we make decisions.
So to, to give that work to a machine like an LLM where we don’t really know how it’s finding or matching these patterns and to take that as the word, that’s something where human in the loop is critically important because if you wanna set your foundation, if you wanna get off on the right foot, like, if I went on a blind date, I’m not gonna marry that person tomorrow. Right? I mean, that would be foolish. So it’s the idea of, like, having, like, full line of sight into your data ecosystem, understanding now here’s an interesting point. If you were just as an experiment in putting your finger to the wind to give you know, start to structure your data and define it well, and then give it to the LLM and see how the LLM does it. Is that gonna create more work for you or less?
Right. Right.
Or is it a Right. Is it is it a value? Like, what’s the value that it’s delivering? Is it is it doing the same as clustering?
Or or listen. Yes. Extremely valid question. Extremely valid question number two. Can you explain it?
Yep. Explainability is really important.
Right. K. So so Mhmm. So just because you can use the technology to do it doesn’t necessarily mean you should. In my world, explainability is everything. If you if you if you if you put in the MDM world what is called a gold master record in front of somebody Mhmm. That is an amalgam of fifteen other records, and somebody looks at it, and they’re like, why am I seeing what I’m seeing?
Right.
You can’t explain it.
If you can’t if you cannot say this is why you were seeing what you’re seeing, your credibility shot. Yeah. And and and and if we don’t have credibility in the data world, well, I mean, that’s a really, really it’s it’s it’s gonna put you on your back feet and not a great place to manage your career from from. So, yeah, words words to go wise. Yeah.
And and, like, what’s interesting to to, you know, the equivalent in the library and information science space to what you’re talking about is something that’s called authority sources and authority records.
Yeah.
And that’s what’s really interesting is you have throughout the Internet, there’s this web that is the underpinning of the Internet and was something that Tim Berners Lee has talked about since, I don’t know, two thousand one is the principle of linked data and authority sources is the idea that and they and these exist. Think Wikidata, not Wikipedia, but Wikidata, which governs Wikipedia.
These authority records are rich ontologies, HTTP identifiers, that resolve and manage entities across the information space or knowledge space or the inter Internet.
So entity resolution at scale, what’s super interesting in the real librarian world, in the real cataloging world, is that the there’s a huge large network of libraries, institutions that could you know, including Google and Amazon. They’re all participants in this in this ecosystem. It’s called OCLC, WorldCat, which is the WorldCat catalog, that you can go on WorldCat, and you’re not only gonna find your books Amazon, Better World Books, you know, Google Scholar, all of those things, but you’re also gonna be able to follow and find the book in any academic or participating library, which is pretty much all of them on the Internet because guess what? It’s all a networked knowledge graph that reconciles and manages entities using linked data or which which is what drives authority sources. So how do we gain authority from a data perspective?
You know, it needs to be well defined.
Yep.
It needs to be authoritative.
Right? You it has to check out, and you have to be able to manage it.
I think that’s something that we overlook and define it.
It’s it’s so strange. Like, I’ve been doing what I do for, like, a long time.
And Yeah. I’ve been having discussions about creating clusters and and the rules used to do it. I’ve been having discussions about systems of record. Mhmm.
Right? What you could call an authoritarian or or authority. Right? And and and the assertion of authority.
This is this gets interesting when it’s like, okay. Here’s one record and here’s another one. Right? In the MDM space, it’s like, you know, which is the authority.
I I’ve been having these discussions. I’ve been having discussions about definitions and and and what you call the the kind of the thesauri. I’ve been having all these discussions, but I’ve never once said, man, I could use the help of a librarian.
And I didn’t say that negatively.
Right?
It’s just it’s like you were on the other side of the, of the mirror, and I didn’t know you were there.
Yeah. Because Hey. I think that we don’t think about it. We don’t think about, like, how information, knowledge, and and those spaces, how it transfers into the technology space. It’s not something that is truly apparent.
And while librarians have been a part of, like, implementing and structuring and scaling these really complex architectures, the practices have not been exposed, unfortunately.
Librarians that goes back to my original point of, like, librarians tend to exist and live in that other ecosystem that serves the public, that serves institution, that that serves in curating, collecting, archiving, and making these things available.
And so there are not many of us. There are some of us, but there aren’t many of us who are working in the technology space directly with large enterprises.
By the way, Jessica works at Adobe.
I work at Adobe.
Where she’s a senior information architect. Yeah. So so this is where, you know, there are there are some of us out there actually doing this. I I think I wonder if maybe part of the, for lack of a better word, problem.
And I’m not saying that there’s a problem, but but the the kind of the bifurcation of this world, perhaps, on the side of the of the librarians is just the scope, the immensity of the scope. And there’s been initiatives out there, I mean, like, you know, the World Wide Web Consortium. Right? And you mentioned Tim Berners Lee, and and you mentioned about metadata standards for all of this data.
And and there’s so many of them. And I’m and I’m not trying to be glib, but, you know, this is the old quote about, you know, standards are great. There’s so many to choose from.
But in my world, I can kinda put a fence around it. Right? And my fence is where my corporate firewall is. Right?
I’m just trying to figure out the stuff in my world for quote to cash. Right? I don’t I don’t need to categorize every book ever made and every word ever said and every word in English language. I just need to figure out how to how to make my quote process run faster.
Mhmm.
So may maybe that’s one of the break points here, which is which is I haven’t felt a need to embrace that world because I’ve got my head down and I’m doing my stuff, But I think AI is gonna change all of that for the very reasons that we just discussed earlier.
Yeah.
Because in my world, MBM, master data management, what we what we say in my world is that, know, we focus on the twenty percent of the data that’s driving the most value, the data that is shared widely, customer, asset, location, the nouns that are the most common and and need consistent semantics across the organization. And that’s kind of been convenient, and it’s it’s also been effective because it’s it’s the data that’s used most often, and it’s used and it’s widely shared. So we need to have common definitions around it.
But I but I what I see evolving is that all of those practices, entity disambiguation, data quality, rules, classifying, discovering, profiling, accessing Mhmm.
It needs to be about everything.
It can’t about everything.
Yeah.
It needs to be holistic because, like, we have to reconcile our own data with the outside world because the reality is, LLMs are trained on the outside world. You can’t change that fact.
And so that expands the definition. But I also ask people if you look at, you know, the the beginning of digitization on the on on computers and the Internet network systems. Like, librarians were the first to really go there. They were the first to go there. And when we practice, when we exist in our own little niches and spaces, I think we’re all being pushed to look outside at other examples of where this has been successful.
Where has this been successfully implemented, and what does that system look like, and what does that system entail?
Yep. And there has been a trail blazed there already. And it’s called library science. It’s called knowledge management. And this is gonna be something I keep talking about in twenty twenty five because we’ve gotta get our worlds closer together, and I hope it’s conversations like this that start that trend. Jessica, thank you so much for spending time with us this afternoon.
Thank you, Malcolm.
I can’t wait to have more conversations. I can’t wait to run into you again in another conference and and keep exploring how we can, how how we can bring our worlds together because I think everybody’s gonna benefit.
So thanks so much.
Thanks Malcolm.
All right with that if you’ve stayed this long please subscribe if you haven’t already, do the like and all the things that the algorithms like please if you thought you got value from this conversation.
Otherwise, I hope you will join me on another episode of the CDO Matters podcast sometime very, very soon. Thanks, everybody, and bye for now.
ABOUT THE SHOW
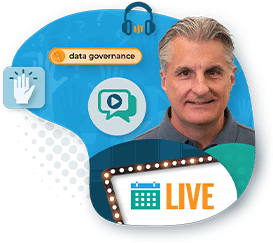