Episode Overview:
The explosion of LLMs and AI is causing a tectonic shift in the world of data and analytics, and on this episode of CDO Matters, Malcolm and Jon Cooke discuss how CDOs can leverage the discipline of product management to best capitalize on these massive changes.
From data products, to the data mesh, and beyond – Malcolm and Jon enjoy a lively discussion on the many evolving attributes that will increasingly define a world focused less on data (and datasets), and more on knowledge and business insights.
If you’re a CDO and you’re interested in learning more about how your operating model will necessarily need to shift over the coming years to adapt to a new AI-driven world of insights, then this episode is a must-listen.
Episode Links & Resources:
Good morning. Good evening. Good afternoon. Good whatever time it is, wherever you are. I am Malcolm Hawker. I’m the head of data strategy at Profisee Software and the host of the CDO Matters podcast. I’m thrilled to be joined today by John Cook, who is the CTO founder of Dataset.
John, how would you define Dataset? Or do do you see yourself as a data analyst consultancy mostly? What what what what’s the elevator pitch for Dataception?
Thanks. Yeah. Thanks, Malcolm. So, I actually started Dataception, two thousand and nineteen after leaving Databricks and after leaving PwC, building a, an AI and data practice there and that kind of stuff. And the goal for Dataception was was really to and I know you hear this a lot about, you know, bridging the gap between business and and technology and data, but that’s something I’ve been doing for twenty years and did it, you know, at PwC.
What I was really interested in now is actually building customer facing analytics quickly, iteratively, very much now what we’re talking about in data products and that type of stuff. That was what data Dataception was founded on fundamentally. We had to actually deliver a a a piece of custom analytics to a business within days, weeks, months, that type of stuff, and it’s right around all that. So that’s what the the mission statement really is for for data ception. If you look at some of the the, architectures and posts I’ve done back in two thousand nineteen, It’s all talking about that type of thing. So that’s that’s that’s really what we’re we’re trying to do.
Well, the the the the I think the marketing speak on your site says low friction insight.
Yeah. And I assume that’s what you what you mean by low friction insight is is getting something out there that works and be practical, and don’t take a fifteen month engagement to do it. Do it quicker. Yeah?
Exactly. Exactly right. It’s like slightly like we do with UX. Right? Where we do, like, prototype, we’re in front of customers.
We get feedback. We iterate around. Same with you in the startup world with product market fit. Same with you.
What agile is supposed to be. You know, the reality is somewhat different, but it’s it’s it’s exactly that. It’s getting stuff to the in front of the business customers, iterating around, delivering it, and not spending these, you know, multimillion pound, multiyear, you know, pro projects, which which we know have challenges. Right?
Yeah. Well, awesome. So we had a lot to talk about today. Thanks everybody for joining, for tuning in, for downloading, for listening.
Maybe you’re you’re taking a run-in the park. Who knows? Maybe you’re sitting in front of YouTube. All good.
Thank you for listening, for checking us out. If you get a chance, please subscribe. That would tickle me. That’d be awesome.
Give me good motivation to keep creating this content. We got a lot of to talk about today. I wanna talk about data products for sure.
Other than AI, data products remains the number one thing that I’m hearing people talk about in the data and analytics world. It was that way last year, and it certainly remains that way. So data products, love to pick John’s brain on that. I’m gonna wanna talk a little bit probably about the data mesh.
I’m not sure we can can avoid the data mesh if we’re talking about data products. Would love to also, explore some of the things that John has been working on from a data science perspective and a language model perspective. So those are gonna gonna be the three topics. So so stick with us for the entire episode.
That would be awesome.
John and I, boy, we have a a modern relationship.
In that in that like, I’ve been interacting with John for for well over a year now on LinkedIn, and, you know, I support a lot of this stuff that he posts. He supports a lot of the stuff I post. Every now and then, we’ll we’ll spar a little bit particularly around the data mesh, which I think is healthy and and which is good, but I’ve actually never been around you in person. But we’ve I it feels like I’ve I’ve I don’t know how many communications we’ve traded. Hundreds.
Absolutely. It’s like it was like a virtual relationship, isn’t it? It was like you relate, you know, electronic relationship. So no.
It’s great. I think there’s there’s a there’s a lot of, synergies, in our thinking, which is good. You’re a bit of a contrarian. I’m a bit of a contrarian.
You know, we tend to go against a little bit against the, the the mainstream, which is, you know, I think a healthy thing is we always need to test and kind of, you know, play back and shine a light on on, you know, on what’s going on. Yeah. Echo chambers, like, don’t do anyone any good really, and a lot of those in data, we we all know that. So, and so, yeah, I it’s it’s been fantastic sort of interacting with you.
I think we did actually we did actually we did a a, a Zoom chat at one time. I think I was talking to a a bar in, in Shoreditch. But yes. So I’ve I’ve learned that yet.
It will be in electronic.
Well, you were in a bar in Shoreditch. I think I don’t know. I think I was here in my cave in, in in in in Florida. But, yeah, we had we had a meeting of the minds with a with a few of, few of us like minded folks on LinkedIn, which was which was a lot of fun. But, yeah, no. Again, yet to yet to meet in person, but but I’m hoping this year, big data London.
Looks it looks like I’m gonna be there in September. If I can find you through the crowds, that that event is not Artemis.
I’m not Artemis.
Oh, why? Why? Are you tall?
I’m over I’m over six foot, and I’ve got the haircut, and and I normally have I normally have a, a a a a T shirt, which I I print, know, some kind of designs. So it’s last year. It’s a great data race. So, yeah, I’m I’m pretty hard to miss, to be honest.
See see, I didn’t even know these things. Like, you could you could’ve told me you were seven feet tall. I know. And I and I was like, really? I I had no I had no idea. Or or conversely, you know, not.
So Apparently, I look quite cool on on on Zoom.
Everyone’s not looking to me when they meet me. It’s like, oh, blind. It really doesn’t have to look at all. So I don’t know why because I’m low down in the chair or something. But I yeah. Too much easier since I’m I’m, you know, a little bit smaller than I am.
But anyway You’ve got a lower half.
I I can’t believe it. You’ve got a little anyway anyway, that that that’s funny. So so so speaking of your your t shirt, one of the one of the things that that you talk about often on on LinkedIn is is you’ve you’ve got this kind of a data product framework, which which I think is is really useful, and I would invite everybody to check out some of John’s posts on LinkedIn related to that.
John posts great content on LinkedIn, by the way.
If you’re not following him on LinkedIn, you most certainly should because he’s one of the people that actually puts a lot of effort in, I think, into the content that he posts.
Always provocative stuff, always in always insightful stuff. So certainly, most certains be checking out, John. I’ve only had one coffee so far today.
So, you still have my up, is it?
What’s that?
Your engine’s still warming up, is it?
It is still it is still it is still warming up. Indeed, it is still warming up. So let’s talk about data products.
High level question. How do you define them? What what makes data products unique to you, John?
So it’s it’s it’s really that’s a really interesting question. I mean, again, we could probably spend the whole whole whole time talking about this. So for me, one of the if we take a step back, so fundamentally, what I’ve been doing the last twenty years is working with businesses design analytics. I do use to do it in risk and banking. I used to do it I’ve done it with marketing teams. I’ve done it with across all different sectors.
And the thing for me is is there’s there’s two hard bits to to defining analytics with businesses. One is getting the businesses to actually describe what they wanna do, fundamentally. Just stealing down the actual requirement, the outcome, the business or goal into something that can be either prototyped or built or what that. The second bit is something we’re all guilty of in the tech and data industry is actually the time between that getting that understanding and actually delivering something of value back to the VX.
Right? Those those are the two things. Right? Now for me, I’m I’ve also been through a lot of, products, starts, startups, launches, and that kind of stuff from, like, product market fit all the way through.
So that product management kind of mentality, I mean, you you rightly recognize as Malcolm, because you’re an expert in the space.
Has a lot of lot of tools in that to actually enable that whole kind of journey between the two things. So for me, a data product or the data product management is is probably a better term. It’s just Craig Grimes thing. A prime O’Neil’s term is the product d way rather than what the actual what the actual product is It’s it’s super important to actually bridge that gap, to be able to get something in front of, a business user, customer, whatever it is, get the feedback, test it. And if you go into the data science world, it’s a very, very similar thing. You know, you do you’d come up with hypothesis, you experiment, you do feature engineering, you come up with models, and you constantly change, you continuously race, and it’s a similar sort of process. So for me, there’s natural dovetailing between product management and analytics, and a data product should be the product of both those things fundamentally.
And that’s so we I use that term in a data product pyramid, which is the framework you’re talking about, to describe the analytics to business people fundamentally. So I did a did a thing last, yesterday on, we did a better product workshop podcast, and I’ve described exactly what it is. So if I go to a business person, say and they say, I want to understand my historical performance, you know, my team, of my of my whatever it is. I said, well, you need need some metrics around that, some metrics that describe the historic historical performance.
I wanna understand what, like, future capacities. Oh, that’s a forecast. I want to basically make a decision. Oh, that’s a decision model.
Oh, I want you know? And I use the term the product as those components, as analytics, as forecast metrics in business terms to deliver a business value outcome that the business person understands fundamentally. And that to me is really what we should be doing. We shouldn’t be getting lost in the tech and data and this of everything else.
It’s about that taxonomy of language that describes what we’re delivering to a business person so they can take it in their own terms, understand the value, understand how it relates to what they do. So that’s really what I call a data product. It’s that it’s that type of thing.
So I I I totally and completely agree, and there’s some of the things you just said, there’s there’s a lot to really like.
One, you started by saying, what does the business really, really want? Right? So that to to me, that needs to be fundamental to a definition of any product. You need to be solving a problem for somebody. And if that’s not part of the definition, then I don’t know what you’re building.
You did touch on the discipline of product management is which is how we would build a product, which I do think is is is incredibly important. We could have an academic discussion whether or not that needs to be part of the definition of a data product, but it certainly needs to be how you go about it. So that’s another thing that I like. One thing I I started to really in in my head rabbit hole a little bit as I was as I was listening to you.
You raised a data science use case as an an example of a kind of a rabbit focus on focus on what a customer would want with building a model. Right? Like and and in this case, the customer you you you said a forecast. That’s a great one.
Where I wanna think about I wanna forecast how much stuff my customers are gonna buy in the future. Right? Right? An an easy one.
I I need to forecast future sales. Right? Look at looking forward, and I’m gonna look at the back, and I’m gonna I’m gonna build this model.
That to me is a perfect example of what you described. Right? Because you have to understand what the customer wants. You have to understand, you know, a lot of things. You need to even understand business dynamics. Right? You need to understand even maybe even economic forces, a whole bunch of things you need to understand.
Yep.
Yet in in the data world, we really get hung up around, oh, eighty percent oh, oh, and by the way, in that process, you would have to be iterative. You’d have to be prototyping.
You’d have to be going back and forth to the business and say, is this this what do you think about this? Well, I don’t really like this. I don’t really like okay. Okay.
Then I’ll go back to the lab. Right? And then I’ll I’ll turn the dials a little bit more. What do you think about this prototyping?
Right? And iterating. Sounds very agile to me. But yet in the data world, we seem to get hung up with the fact that that process takes a lot of time and often can be an can can produce unsuccessful results.
Like, it leads to these dumb quotes, like eighty percent of data scientists’ time is is wasted. Right? Or all these fifty percent of models don’t go to production.
Some is that a good thing or a bad thing? Like, it seems like there may be some tension there. Do you know do you know what I’m saying?
Yeah. Yeah. So it’s it’s it’s an interesting I I think it’s a feature of the of of the of the activity.
I I get quite, heated when people say, oh, eighty percent of the data’s time is wasted on cleaning data. No. It’s not. That’s not wasted.
That’s on them understanding the the data, understanding the business. Yeah. Data data science isn’t machine learning. It isn’t data engineering.
It’s basically building business facing solutions using algorithmic and scientific methods. And there’s a whole range of things you need to do to be able to you understand the business. You really understand the the, you know, the the data tools and approaches. There’s the prototyping, but it’s all the product and stuff as well.
So I need to go and understand, is it is does the business can I talk to business in their language? Can I actually deliver something that’s got back? Do I understand what that value is? You know?
Can I also communicate the fact that we’re using data science algorithms that we’re only do incremental, you know, improvements in accuracy? What does that mean to the business person? Because the business person might say, I just wanna, you know, pass or fail, but, actually, that’s not how that works. So can I communicate that stuff?
So there’s a range of stuff around that. And I think the the the the shift that we’ve gone through, what we are going through now in May two, this has been happening in kind of niche areas. We’re going from modeling, data science to solutions, ML and data science solution. The data science solution, you think about you’re delivering a solution, end to end solution.
This is where kind of the product comes in. It’s not just the model or the data or the algorithm or whatever it is. It’s it’s the whole end to end piece. It’s the it’s the support.
It’s the operating model. It’s the it’s the, you know, the the light the entire life cycle cycle of how you deliver that. It’s like you’re going back to any other product. You know?
If you’re so for instance, I I I caused a bit of controversy saying chat GPT is a great data product. It’s a very very complicated one, but it is actually a product because it’s a it’s a life cycle. It’s it’s like eight models underneath it, but it actually has customers. It’s got it goes through iterations.
You know? It has feature enhancements. It’s it’s got this living, breathing thing that people pay for fundamentally.
And data science traditionally has been you know, a lot of data scientists go, why don’t we do the modeling bit? It’s like, well, the modeling bit’s only you know, if you look at that, the the the famous Google one where you’ve got the model, which is, like, ten percent of the whole thing. It’s the end to end solution, and that that to me is the shift that we need to go to is going from a modeling mindset to a end to end solution mindset, and where the model is an important part, but it’s only a small part, and there’s a whole operating model around it.
Well, so let’s let’s let’s let’s kinda steel man the argument around why why ChatGPT isn’t a data product. Like, to to me, it’s like, well, of course, it is. But but I’m trying to understand why would somebody think that it wasn’t? What what would be the most compelling argument you could make to say that it isn’t?
I have to be honest, I haven’t heard one. The Okay.
And I’ve had I’ve had the problem.
The the the interesting thing is so what again, if we take a step back, let’s probably touch on the data mesh because we will go into that as well. So, obviously, five years ago, then it wasn’t just, the data mesh. It was it was a few other people who classify this, a packaged curated dataset being a data product given that nomenclature. Right?
That’s that’s what’s happened. Right? So, fundamentally, that was the product of, you know, dodgy pipelines and not Yeah. Data, data warehouses not being treated to the same level of, you know, robustness as software platforms and yada yada yada.
So we understand all where it comes from. What was what was proposed was a bit like what you said before, the sort of product management process versus the the product is that if we apply product management, then everything will be okay. We’ll get the robustness and stuff. It’s like, well, that’s more like the the robustness of the delivery of of what you’re doing.
So the design of well, you know, like I said, if I deliver microservice, it’s I I I can put CICD in there. I can put testing and putting put all the robustness around it, but it’s not doesn’t make it a product. So the product management, product thinking is actually the natural delivery and and design aspect of that of that piece, right, fundamentally.
So and that’s what’s caught hold, and and the industry has, you know, let’s be clear. It’s been driven a lot by vendors. It’s been driven a lot by you know, the the the weight of the marketing budget behind that term being a packaged dataset is is vast, and it’s quite fun. I have quite a lot of conversations on LinkedIn.
I’ve stopped doing that because it’s it does it’s it’s it is a bit it’s a bit of a time suck, to be fair. It’s and it doesn’t and it it makes me look, you know, it makes me look bad. But, the there’s all that marketing behind it, and that really is the only reason the only argument I’ve seen against it. Well, that’s what we call we are we now understand this is this is what it is.
And every time I speak to a practitioner, say, actually, it’s the wrong term. They go, yeah. It really is, but that the the market’s already gone there.
So, really, the only argument against using that term, and we should come up with a different term for these kind of these datasets. I I I can’t I think data containers is actually a much better term to describe exactly what it is. And it’s important. Let’s be clear.
I’m not back in the concept concept actually. Right? Right. It’s not curated, and it’s got all the nice, you know, technical features around addressable and blah blah blah, all that kind of stuff.
Absolutely not. It’s just not calling product because it’s confusing. Because when if I go to a business person, they we’re gonna deliver data product and it’s a dataset. They’re gonna scratch their head and go, what are we doing?
We we’re selling our data or are you what’s what you know, that type of stuff. So I have to translate that product term between business and tech team or data teams, and it’s we should be doing the opposite. We should be flattening it out.
Yeah. Well, so so man, to to totally agree.
What what I see in this split between kind of data data products a dataset as a data product and a real data product, you know, something that is solving a customer need.
Yep.
When I hear people say, well, you know, this is really about scalability. It’s about governance. It’s about reuse.
All those things are fine. Right? And and that’s okay, and we should we should be we should be shooting for that. But I think you’re absolutely right.
Calling that dataset a data product is is a little misleading because to me, like, I am a product person. I built products. Right? And I look at that stuff like raw materials.
Right? I I look at those things as, like, components. Right? They’re important.
They need to be governed. They need to be managed. You need to do that at scale. And what those components need to be a using a mesh phrase, you you need to kinda shift left to understand the nature of those components and to get them as close to the kind of business as as possible.
But that that doesn’t mean that those themselves are products because if they’re not delivering value to an end consumer, then they can’t I I would argue they can’t be called a product. So maybe we need to come up with another name. I I don’t know. We’ve already got too many names for everything we do.
But Great.
Great.
But but, yeah, this I think we we’ve we’ve struck on something here, which is, you know, this idea of a dataset versus a finished product. I love your how you describe a kind of a finished product as as incorporating a whole bunch of things. Because as a product person, I would consider that a fundamental of what I would call a go to market. Right?
Yeah.
If you have built something like a ChatGBT or integrating something as a ChatGPT that is so transformational that actually requires you, or you could benefit from working a totally different way in order to use the product and to get the value out of the product. To your point, like changing your operating model. Right? Or changing a governance policy for even simply.
Right? Like, to me, that’s a go to part of a go to market exercise that is a part of that product management workflow that makes total sense. So I I I think I think we agree. We’ve already got too many names for things, but but I think that is the core split here is data data data is data product.
And when I was at Gartner, they were they were they they they they’re hung up on data as a product versus data products.
Yeah.
So I don’t need the word data as a product term at all because it’s I don’t either.
I don’t I don’t either. It’s it’s it’s it’s just another layer of kind of, you know, I don’t wanna say complexity, but possible confusion. Anyway, so let’s talk about the mesh a little bit.
Yeah.
I I I know you’re a believer, but you you are pretty clear that the way you see the mesh would lean a little bit more towards the socio of the socio technical than purely technical. And and I’m and I’m quoting Zomak Tagani that she said the data mesh is a sociotechnical phenomenon for those who may not know. Do do do you agree? You seem to be leaning a little bit more on from from the perspective of kind of domain centricity versus some of the technical underpinnings of of a data mesh. Do you agree?
So first of all, I’m not a big fan of the word social technical because it’s Oh, are you?
You’re not? Okay. Interesting.
No. No. I’m not I’m not well, all all software I’ve ever built has an operating model. I have people in the office, It’s all social, technical, and my blood. But let’s let’s let’s move on let’s move on from that. Okay.
So but for me, the the interesting thing is so I started my this this journey into kind of composable business facing analytics two thousand and fourteen. I built a a a a management information solution for a bank, plus thirty or forty different departments, three thousand metrics, different domains of transaction reporting settlements, confirmations, that kind of stuff. Can’t couldn’t have done it in in a warehouse. Some people tried and failed.
And I’ve built these little composable engines that you could spin up and actually query them for a particular metric that met with a dashboard, this type of stuff across the different domains effectively. So this was built and, you know, we we we showed that and proved it out working and all this kind of stuff back in, let’s say, two thousand fifty terabytes, you know, thousand records a second. It was it was quite revolutionary and the the whole different story around why that why would that doesn’t exist today, but anyway, it’s it’s more political than anything else. But so I’ve proved out that whole kind of concept.
So I’ve been thinking about this for about ten years fundamentally, and I published something about five years ago called Synchronized View Architecture, which had a similar idea.
And to me, that to me is is the the the the the core of what the mesh vision is. So I believe in the mesh vision. I think there’s some challenges with the specificity of for the mesh. I think there’s a lot of pieces missing and that kind of stuff. This is why I believe actually from an architecture perspective, the architecture I’ve developed in about three or four years ago, had a had mesh and fabric components of them. But to me, the the the the the mesh vision should be people who own the use case building their own and deploying their own stuff.
All connected all connected, but actually deploying their own stuff. You know? And what’s happened, I think, with the mesh is, you know, the original mesh proposition was, you know, breaking apart of the warehouse and and Yeah. Like that.
We look at that. That’s what it was. And the the the, the value of the mesh is basically teams being able to share data domain teams being able to own the data and then share those datasets between. That’s really what.
And the the currency of that was effectively a data microservice, data product, whatever you wanna call it. It’s a data microservice. Those are the kind of the three key components of it. Now for me, the the value isn’t there is some value in people being able to share data.
Let’s just be clear. Silos are a bad thing. No prob the real value is the people who are in the use case to be able to deliver their own components on their own tech to deliver those use cases. Right?
So there’s there’s there’s there’s a sort of Venn diagram between my vision and the mesh’s vision. There’s quite a bit of overlap, but, actually, the the the the outcome is actually slightly different. You know? Mesh is about trying to get team to working together, you know, to share data.
I I’m more about how those business teams or those business facing teams, and that have to be necessarily organizationally, business and centralized, but business facing team deliver those those those things. And and I’m a great believer that composability, that compactioning up of the of the, the the outcome. But it’s for me, it’s not the data. It’s the model.
It’s the data. It’s the UX as a as a completely end to end piece fundamentally.
So I I’m a great believer in that. That to me is where the value is, really.
So I there’s I gotta admit, man. I I’ve got a bit of a love hate relationship with with the with the mesh. And and and, frankly, a lot of what I post comes out more the latter than the former. And I should probably embrace more of the former because the reality is is that if you as a centralized IT or a data and analytics function don’t give the domains the freedom to do what you just described, they’re gonna do it anyway.
Whether whether whether you like it or not, whether as as a you know, if you’re a VP of a more of a centralized data and analytics function, if you don’t give those business units freedom, they’re gonna do it anyway. They’re gonna go hire their own data scientists. They’re gonna go hire their own analytics people. They’re and they’re gonna go build the reports on and on and on. This is why First, Click, Tableau. I mean, I could keep going on and on, but this is this is why these solutions exist in the first place. I would argue to a certain degree because they they gained a foothold at a lot of functional units who wanted to do their own analytics.
So I think you need you need to embrace that. And if you don’t, it’s gonna happen anyway.
So I do like that. I do like the domain centricity. My my my challenge here is the is cross domain.
Right? And and the efficiencies that must and need to exist in order to support cross domain insights, both from an analytic perspective and an operational perspective as well. This is another thing that I have a concern with around the mesh is that it is that it doesn’t really kind of address the operational aspects of a of a business. But how would you how would how would you speak to that?
If you’ve got a client saying, hey, John. I I love this. I love the composability. I love it.
But at the same time, you know, our business processes traverse function, quote to cash, sales to finance. How do we bridge those?
So I think that, again, taking a step back, the the the domain centricity, I think, is actually a little bit of a I won’t call it red herring, but it’s actually there’s a lot of focus on the organization, a bit about domains only. And that comes from domain driven design, which has come from software, which is about people building software solutions on the transactional side of the fence to manage their part of the business process, and then you build your object model and this kind of stuff in it. And there’s right there’s some very specific bits around that. You don’t expose that whole object model to another part of the business process or another team. Right? That’s where domain that’s where DDU comes from, and that’s one of the the core underpinnings of the mesh philosophy. Right?
In analytics, we go go to the analytic, and I I tend to use the word transactional and analytical because, a recommendation engine is a operational system even though it’s an analytical workload. So so on the analytical side, you you almost always cost domain. I mean, it’s fundamentally analytics only by its nature, but trying to work out what’s happening in different parts of the business and bringing them together. Yes.
You’re almost always doing it. I totally agree it’s an Achilles heel in the mesh, right, fundamentally in terms of actually that that whole piece. Very much again. But again, you gotta remember what the mesh is doing.
It’s about sharing data between domains. So it’s not really about addressing, like, a big cross domain use case fundamentally. You know, you you have you have the mesh underneath it, which where the team share the data, and then someone owns the use cases. Their job then to cross the domains and actually do that.
And, listen, you know, we can get philosophical about customer facing versus aggregated versus source data products and stuff like that and that kind of stuff. That’s a bit of a rabbit hole in my mind. So analytics is all about that fundamentally. So I think from my perspective, without without solution, our architecture now, I think we actually we actually, have the domain being the the model, the actual the solution, and the data actually not being associated with the with the domain.
So a piece of the use case and spin up a, you know, data product, you know, call it take your forecasting. Yeah. I wanna get wanna do an an an order by sales forecast, for instance, you know. So I wanna go to order management.
I wanna go to sales. I probably wanna go to logistics to see what the the demand was, that kind of stuff. I want and and the datasets come from all those all those people, and then I build the model on top, and that all gets delivered as one as one package. So, actually, the the the, the the whole end to end solution is owned by the person who wants the use case, and that’s in that that particular domain.
The data itself needs to be supplied. Totally get that. That makes sense. And you have to have all the rigor around it.
But, actually, the domain bit is actually the whole piece. And, again, that goes back to what DDD is really about. It’s about deploying a whole application with its own model. And that’s the way we think about it.
So we take bits of data from each of those different three business areas. We combine them, materialize them into a schema inside the, inside the, the the model, you know, package, the model container, and we deploy the whole thing as one, fundamentally.
So we actually again, we kind of we sort of break the mesh kind of the the the the the guardrails a little bit, but, actually, that really worked because, actually, I mean, it’s you’re you’re packaging it altogether. You’re doing across the main problem. You’re still getting the, the domain team to own the data to deliver that, however they want to do that, but you’re actually owning that that cross domain piece as well.
So in essence, cross domain is a domain. Kind of.
So yeah. I mean, I the way I think about it I mean, again, we can discuss what a domain it’s about business process. It’s actually cross business process. Really, that’s that’s what we’re really getting down to. I want to put different bits of that bits of data, different parts of the business process, aggregate them either simply through a metric or through something more complicated like a machine learning model or what have you. I want to join those those pieces of I want to join those those pieces of business process together with the attributes that I want for my particular use case and then deliver that as a business facing component.
That to me is the is is the secret sauce, and that’s what we should all be doing in my mind. Everything else is and there’s, obviously, there’s layers of abstraction around there, and we sort of start talking about, but there’s a data architecture piece in there, or the data virtualization piece in there, which we talk about the fabric, how the fabric comes in, they’ll actually really solve that piece. So I think, actually, the fabric and mesh, those can put together. Suddenly, you get a really willing winning formula. But you’ve gotta have the the clear outcome of delivering an end to end piece. If you don’t have that, it’s it’s it gets a lot more challenging.
Yeah. Totally agree. The way that I look at it, and it it’s it’s not centralized versus decentralized. It’s not, you know, domain driven versus central IT driven. It’s it’s both.
And I think for I think for us, as data people, we tend to think very kind of deterministically.
Right? We we have a hard time with seeing things as two or three things as this at once. Often, I I I know I do. I kinda lean towards deterministic thinking.
It’s either all or nothing. It’s a single source of truth. Right? You know, in the NDM world, it’s like, okay.
Well, this is the one version of truth to rule them all. When in reality, there’s multiple versions of truth. There always has been multiple versions of truth. And that’s kind of I’m I’m paraphrasing what I just heard you say, which is that there is domain driven design.
There’s domain driven centricity. You can you can Yeah. You can support the needs of marketing while at the same time, you need to support the needs of your CFO who asks how many customers do we have. You can do both.
The real challenge, though, there is not technology. It’s governance.
Because now instead of one set of rules right? If you were just totally, completely centralized, top down, heavy handed, old school, thou shalt type approaches to everything, there’s one sets of rule one set of rules. Yeah. Right?
Yeah. But in the model that you’re just describing, there’s multiple sets of rules, and they all have to be they all have to exist. They all have to be documented. They all have to have policies, procedures.
They all have to have governance of over those rules.
And and that’s where I see the the biggest challenge for a lot of organizations is not not the fact that the technology can support it because we know it already can. But it’s that it’s a fairly mature governance approach to do what you you just described. Do do you agree?
Yeah. Yeah. Indeed. Indeed. So there’s that there’s actually two levels of governance here. Right? So, again, if you go back to where the mesh comes from, which is basically microservice, and I was doing service oriented architecture back in two thousand three, two thousand four.
We’re still in contracts and all this kind of stuff, which always makes me laugh when things like data contracts come. So hang on a minute. That’s back twenty years ago.
We’re back to the most.
I love it. Yeah.
We’re open, you know, all the WS specs around policy and all that kind of stuff. So yeah. Been interesting about and I and I actually delivered a number of service oriented architectures, across different organizations. I actually did one about two years ago, interestingly enough.
And the governance was always a key part of it, fundamentally, the the top level governance. So, basically, if I’m producing a bunch of reusable services, how do I make sure I don’t get two different teams building the same service? How do I do the versioning around that? How do I make sure if if one service contract calls, a customer ID one thing and the other one actually has the same how do make sure those two mean the same thing?
So service always had a governance layer to make sure, you know, the, you know, we last that last company we did, I had some service governor governor, you know, British governor.
But it was actually someone to actually make sure because it was a small company so we could actually do that. Make sure that if someone said, we wanna build a service, go, oh, well, I should just extend that one. Let’s put another version on it.
Are you sure that’s going the same thing? And, you know, that is that is something that was absolutely a key part. I mean, it was one of the key reasons that server actually service oriented architecture failed, actually, because they didn’t have this governance. Like, you end up with this chaos.
Right. You know, people building this sort of stuff, you know. And and it’s funny. I see this thing about microservices.
You know, obviously, it’s been around, you know, about ten years, but I’ve had many robust discussions with people building microservices architecture. Well, how are you doing the governance on that? So, oh, it’s microservices about decomposing application. No.
It’s not. It’s about service. The service bit is the bit we is the most important bit. The micro is actually an implementation detail.
You can do a service or a data architecture without microservices, believe it or not. People would say, oh, distributed monolith and get all hot under the collar of that. But you can’t. I’ve done that.
I did it twenty years ago. But the governance is always there. And we go into the data world. If you’re doing re any kind of reusable asset and you want them to talk to each other, interoperability, you need governance.
And I mean, there’s no point having someone producing a dataset, you know, microservice, data service, whatever, one side and then another one, and they call things different things. They’re not gonna be able to interoperate. You can get all the technology in the world, but you need that. You need someone to be the arbiter on what the what the protocol is fundamentally, which is the data.
You know, the the those those the the semantics and meaning. And that is a that’s a classic overarching governance piece. Otherwise, you’re just gonna end up with chaos.
And you just described the reason why most data marketplaces fail.
Right.
And and I’m taking a bit of a left turn here.
Okay.
And and and I’ve seen so many data marketplaces come and go. And the reason is because you can publish something, and you can even you can even put a contract sitting underneath it or or whatever. It doesn’t matter as Yep. You can publish the schema. You you could doesn’t really matter. If I’m a consumer of data and I’m just looking at something, right, here’s here’s a a set of data about, you know, turtles.
I don’t know. It doesn’t matter. Right? It’s like, oh, well, who published it under and and what rules were used to publish it? How do you define a turtle?
Right? Do do you recognize this this for like, maybe turtles are wrong. Yeah. Well, but you get my idea.
Like, if it’s date if it’s date about customers or people, like, okay. When does it last updated? What are the governance roles that go into this? How do you define uniqueness?
How do you define relationships that exist between the people in your table? So all that stuff, if I’m just looking at at at at a set of data, like, maybe a data catalog that just maybe has some basic definitions, of course, but you don’t you don’t know the underlying governance that went to create that thing. Can you turn around and confidently put that into production?
Well, if you’re a marketer, maybe. Right? Because the cost of being wrong, maybe it’s okay. Maybe I’m just using this for a shotgun marketing campaign where I’m I’m, like, I’m spraying the Internet with ads or something, and maybe it’s okay. And and twenty to thirty percent spill is fine. But if I’m in finance, nope.
And and and so so I think we’re touching on something here that is really, really interesting. Yeah. We ask CDOs to be half technical and half business.
When I hear data and and I lean on the business side. Right? It seems like you straddle right in the middle, which is awesome, by the way.
Nothing. Yep.
Yep. What when I hear data contract, what I think is is that thing that I have to click every time before I download the app.
Right? Like, the the the do you agree with the the other one. Yeah. To to terms and conditions.
So it’s like when I hear data contract, that’s what I think. I think, oh, man. Like, you’re gonna make me agree to use this like, before I download the report, I’m gonna have to click, do you agree to the terms of the use of this report? But when a when a data person hears data contract, they think what you just described, like like, Wistl, schema, like like like some other you know, these are the terms of using this this dataset.
Right? Here’s how to define the data, and and here’s here’s the field widths and the field types and all the stuff that would go into, like, back end stuff.
And so often, most of the CDOs, I think, really kind of fall on more on the data side. When we hear data contract, they think technical. But then there’s a whole bunch of others out there that think, like, wait a minute. Hold on a second.
But you have to be both. This is this is just this is fascinating. And I I get Yeah. How much is on the dataset versus data product thing as well.
Yeah. Yep. So just one that that is a great thread, actually. So if we just we just go back a little bit to actually the the understanding the the how the data was created.
So Yeah. One of my favorite kind of, paradigms or way of explaining this is Kaggle. Right? I mean, if you come across Kaggle, which is a, a data science kind of, solution where you go into their competitions.
You say, we’ve got this dataset, and we want to work and predict cancer and stuff. And there’s a fifty thousand dollar reward to go in and let everyone pause in and grand master this. You know, these people get these data science grand master titles because they’ve got one three table competition competitions, basically, around data science. But what’s really into I mean, they are they are there’s some real world act I think there’s a lot of kind of playing and, you know, just sort of, you know, vanity competitions, but it’s really good.
But it’s interesting. If you go into a Kaggle competition and you see that so here’s a base dataset. So we’ve got, like, a, you know, a bunch of cancer survivors, for instance. You know, cancer people who got cancer on, dataset.
Here’s how it’s made up. Right. Now you go and go and, and and predict, you know, cancer recovery rates or something like that. You go into all the projects around there, and you and you see all the working out.
You see how the data’s been transformed. You see how it’s all been done. And then here’s the model. Here’s the outcome.
You actually look into it and go, oh, I see what they’ve done there, and there’s there’s threads around conversations and stuff like that. That is what we should be going to fundamentally, in the data one. This is why my definition of a data product has those pieces in it because, fundamentally, I’m gonna get a bunch of sales data from, you know, from the sales system, what have you. I pick three three or four attributes out that I need.
I then transform it, and I package it up to a pipeline, build a model, put some UX into it, and then package it up all as a product definition, which we have in a platform, the entire definition and the process of that. I can see exactly how the data is used. I can see exactly what the transformations are. I can ask questions to the person who actually got the part and say, what what do you do there?
You know? It’s not stuck in some central lake or warehouse where all the aggregations are kind of lost in the business of time and all that sort of stuff. It’s got a very plot of short lineage chain that’s right back to the natural source system fundamentally.
And I can see what’s how it’s how it’s been done. So it’s not in the abstract. That, I think, is the epiphany we need to get to when, you know, the the the that we all needs to have. If you look at LLMs, that’s what they do.
Yes.
Think about it. Very opaque, but it is exactly they take the raw data and it goes through an encoding. It goes there’s a lot of preprocessing and encoding, and then it goes into into vectors, and then it goes into decoder, and there’s some training label data. And you can kind of sort of follow exactly what’s, you know, within reason, what’s actually happening, but still for the raw data.
You know? So you can see what the label is. Right? You can see kind of you know, you see some of the weight.
Obviously, they’re huge, so you can’t look at all of them. But it’s it’s it’s, ironically, it’s more transparent than a lot of data warehouses are. What’s that?
So you’re you’re you’re you’re touching on something that’s really interesting. And and to to me, well, so when I was at Gartner last week, this this podcast will probably up air in another month or so, but last week was Gartner here in the US. Right. And one of the key themes was was this idea of metadata becoming a first class citizen, whatever that means.
Right? But but but the concept there is to me, it’s all about context. Right? And you need the full it’s not just about creating a dataset.
It’s about all the metadata associated with that dataset. It’s about the lineage associated to it. It’s even maybe even about other things like context. Maybe you run a graph and and on and on and on.
But I I think the real value here, scale will be delivered and incredible value will be delivered if you and and I use the example of a data marketplace use case. You could use a data sharing use case. You could use any use case where where data needs to be shared widely in order to create economies of scale.
Or unless you have that layer of, let’s call it knowledge, right, using the the the data information, knowledge, wisdom kind of paradigm, unless you have that knowledge of the data, which would include the governance, which would include things like who created it, under what situations, what are your business rules for creating it, how do you define uniqueness, how do you define quality.
Until all of that gets packaged as a product.
Absolutely.
Like like that, to to me, is the uh-huh. Then we can start sharing data because you’ll be able to interrogate the data and know, oh, okay. This doesn’t really align to how I define a customer. It’s interesting, but it’s not how I think of the world.
Yeah. Metadata as a first class citizen, I think, is important to that. But what I find is really interesting is that we’re trying to take a a a really relational world and make it more like this l this LLM world. Right?
And make it more like a text world. So we’re we’re talking about vectors and graphs and and and meta metadata as a first class citizen. What can we do to our old school rows and columns to provide the knowledge that is sitting there buried in text and has always been buried there in text? I see I see you nodding.
And it’s these two worlds. I I I find it fascinating.
Absolutely. So you gotta remember, like, you know, relational databases, whether they’re colon or cloud or whatever, they are a temporal snapshot in time of state. That’s what they’re they’re they’re they’re snapshot in time of states, you know, and the rational model means my interpretation of what the state of a particular business process system, whatever I’m trying to describe, is a is a at that time, I’m gonna try and model what I think that state looks like. I’m gonna turn rational system.
Now if you think about business process and customer, I love customers are one of my favorite ones because you start look at the business process of a of a of a company. So, like, an ecommerce kind of or supply you know, manufacturing company, whatever. You get the the lead generation. You get the the, the prospects.
You get the close. You get the end of servicing. Then you get retirement. Then you get customer service, all that kind of stuff.
The customer object, business object, not to my data object, changes. It morphs based on the particular department or the particular business process that they’ll be doing it. So if the data changes, but yet we’re trying to have we’ve got this kind of snapshot, kind of static thing that there there’s a massive impedance mismatch between the two things fundamentally. Right?
And we’ve been driven trying to understand that entire business process or parts of that business process is a, you know, in a relational kind of snapshot model. And if you go to, you know, if you kinda go to the sort of microservices kind of vision and sort of the data mesh, what you know, that that that type and and the data product vision, What we should be doing with analytics, you’re putting probes in different parts of that business process, you know, from an analytics perspective, but the data’s gonna be different in each of those pieces. And what we’re and then we’re we’re giving the insight around what’s that what’s happening in that part of that process.
So I could be measuring a think about a a water pipe. You know, at one point, I’m measuring the flight. At one point, I’m measuring the, salinity. One point, I’m measuring the, pH value.
And then I then I’ve got some understanding about business. But then I need to get the context of joining at the top of that, above that. So, basically, joining pH versus the workflow versus because that gives me the business context of what’s happening at that point. We’re a long way from that from data.
We’re still in this relational static kind of world.
And that’s if we can break that apart, this is really what microservices were supposed to to do. They’re trying to break that whole kind of business into small component with a composable components of that business person then wire them together at the API level. At the API level, you’ve got actually got a business context.
Whereas in data, what you’re talking about, a lot of times you’ve lost it because sometimes you got the it could be an aggregation. It could be a big white table with two hundred columns. You have no idea about the business process. You hope there’s some sort of state flag in there or what have you, but maybe not. If you understand it, you know, but we’re still trying to it’s almost like we’re trying to compress three d into two d.
Right. It’s that kind of that kind of a problem. And I think, actually, we what we need to do is actually get away from this kind of static thinking. I mean, tech isn’t there.
We’re building the tech at the moment looking at this, but it’s part tech, but part process, part governance. You’ve got to solve all these kind of problems around it, which, again, I think one of the challenges with the mesh, it has this kind of sort of vision, but that’s those there’s some big holes in the in in the in the implementation of how you actually do that piece. And, obviously, we that’s something we’ve been doing for, you know, thinking about for, like, ten years. That to me is where companies need to go.
And we we I mean, we we label it. It’s coming called the composable lens sprites where you’re creating small composable piece from analytical workloads, transactional workloads. It could be microservice. It could be updated product as we define them, forecast this kind of stuff.
And you can continually, you know, like LEGO bricks. You kinda could reformat, reconfigure them as a business changes as but you’re doing small incremental, you know, deliveries. And, obviously, that gets challenging with large COTS applications like SAP and what have you. You know?
There are challenges around that. But, fundamentally, that’s that’s this is the, I think, the source of most of the friction between the data and the business. Well, this kind of I need to compartmentalize and kind of a static representation of this, but, actually, it’s a it’s a fluid business process.
Yeah. It it it it is. You’re absolutely right. I wonder I’ve I’ve been asking the question recently to a lot of really, really smart people, and I’m getting a a variety of answers. But I wonder if there’s a world where the applications themselves start to get built in a way that doesn’t rely on rows and columns.
That just dumps everything out to text as as a as a long form narrative.
Right? Right. Like like like Nancy in procurement just requested an invoice. Right? Like and and instead, right, like, instead of that getting dumped into a set of rows and columns, have that at getting dumped into literally a story of the business.
Yeah.
Right? Like, where where the data is being stored as a essentially, a narrative or a story.
Yep. That that that that AI can more easily consume, right, where there is knowledge embedded and there’s information embedded. I I don’t know. This is getting really high level and and totally conceptual. Maybe our listeners are like, man, these guys have gone to crazy town.
You know, we probably we’ve gone well off the reservation, but but but it’s interesting. We got back a few years, we look at triple stores. Right? That that was kind of going into that sort of world. Exactly. You’re actually now joining data with with semantics between them. Right?
The relationship and graphs now, you know, really starting to take Nancy issues invoice.
That thing.
Yeah. Still a little way to go, but it’s definitely going much more from the relational into the graphs of dynamic and their, and the and the the relationship is a first class citizen, and you can have different relationships and that kind of stuff. So this, I think, is is is really, really interesting because you you you are going into that world. It’s not just a knowledge graph. It’s not again, knowledge graphs, even though they’re dynamic, they’re still still static representation. Right.
If you’re tying together this kind of these kind of components, transactional analytical components in a graph, in a in a dependency, in a on your on your on your business process, you actually get the best of both worlds. And we something we’ve we’ve we’ve been playing with a little bit is around how this is hybrid graph where you actually have execution nodes and you have data nodes. And it’s basically mirrors the business process. An execution node could be a, you know, could be a, a data product.
It could be microservice or what have you. But you’re mapping out your business in real time with actual components, and you can reconfigure them. That is this is obviously less just like, you know, Star Trek when people at the wheel, but it’s it’s that to me is is, you know, that’s really what where we should be going. It’s that kind of where we are, have a faithful representation of the business analysis in technology, which is something we struggle.
But the problem with that, of course, is all that a lot of us technology is not guilty of this. We can’t we can’t compartmentalize that. It’s too it’s too complicated.
It’s too kinda it’s like, again, it’s this kind of, you know, visit visiting things in four dimensions is like Right.
It’s good. My my I can visit this thing in two dimensions in just about three, but four, oh, it’s too hard. So what we do is we try and compress this dimension dimensionality reduction same in data science. We’re trying to take five or six, ten features down to three features because it’s that’s what I can understand.
It’s the processing power required. There’s actually a lot less. And that’s what we do, and that’s why we still, you know, it’s it’s it’s hard to break out of that more because our just the the limits of our cognitive ability to actually understand that kind of space spatial awareness or that, you know, spatial dimensional model is actually very, very difficult. But if you take a step back and go, actually, it’s not a technology problem.
If you start with the if it’s a if it’s a business process and business process reengineering problem, and you actually then have the tech that actually allows you to map onto those with all the other stuff around it, then it starts to become a different thing. But the tech becomes almost like an implementation detail around that, fundamentally. But it’s this is kind of what where where we’re going, but it’s it’s it’s still a little way off. And, you know, part of that is obviously, I’m sorry, the business process.
But coming back to your, you know, text and narrative, you know, you can actually say that you’ve actually described that business process and narrative of, you know, the, the lead generate leads come in from this particular conference. The x x person’s qualified that lead and given, you know, and and hit the the five checkboxes for doing the qualification process. Let’s the salesperson’s now booked the demo. The demo didn’t go very well.
We’ve got some qualitative assessment, maybe even a quantitative assessment around the thirty percent. Did did the buy exit buying signal. You know, it’s you can start seeing that kind of that sort of text based narrative, but it’s it’s underpinned by a process. You’re describing the process in natural language as opposed to through some symbolic language like we’re doing, you know, in in data.
If I had a a giant bag of money, like, extra x x extra money and, I would going to Ireland.
Right? Sitting Sunday.
Right? Well, may okay. Maybe.
If I had a bag of money that I had to spend on investing in a company, hypothetical thought experiment, I I would be looking at ways of bridging old rows and columns world to this new AI world and this new kind of text driven LLM world? Because I kinda see I see LLMs, and this is a good segue in our last few minutes here.
I see LLMs kind of becoming this new operating system, and I know that that’s just this whole kind of drastically reduced view of the world, but I kinda see things Yeah.
Going that way. Yeah. Yet our whole world for decades has been and I just loosely say rows and columns, but hope you you you get my point. Like, this time this this shot of time of data that is where you’ve gotta infer a whole bunch of stuff about it in order to create the narrative, or maybe you have to run graphs on topic on top of it. Just try to understand some of the narrative, and even then, it’s incomplete.
But finding a way to bridge those worlds, that to me is, I I think, in the short term for classic data and analytics folks, that’s good that’s gonna be hugely, hugely valuable as and and but it does require a bit of a mindset shift. Like, kind of the multidimensionality of of thinking, and thinking that two or three or four things can all be concurrently true. Right? Yeah. What what they on the surface may may seem contradictory.
In our last couple of minutes and and we are running low on time, but I do know that you have you you you’ve spent some time as a data scientist. I looked through your resume. You actually have data science pedigree.
I know you’ve been doing some work on what could loosely be called smaller language models.
So I’ll run a hypothetical by you, and I’d love to hear your perspective.
I think that LLMs as a way to model, you know, language and a way to to to model how humans interact and think is, I think, is is useful.
But I think that there’s an evolution underway where we will get to more domain specific language models that are really kind of focused on maybe speaking unique languages, like I speak lawyer or I speak accountant, where you can build these things smaller and far more efficiently, and you don’t need seventy billion parameters and twelve thousand GPUs to do it, where you will have smaller models that are very kind of task focused at a domain level. Do and I think you’ve been doing stuff that kinda looks like that. Right?
Absolutely. Absolutely. So it’s into what’s what’s interesting about transformer based it’s largely transformer based. Large language models are all about breadth.
They’re all about the the the multimodal, the kind of let’s do let’s do lots of things a little quite well. You know? Let’s do let’s do this breadth rather than depth. My first, generative AI project was actually two thousand seventeen, and we’re using very simple modeling like naive face and stuff like we built our own taxonomy.
We wanted a very deep level, sort of bank, and we’re doing things like a a call center for investment managers who have deep domain knowledge. So we had to go down the the the taxonomy of of of language quite quickly, What to be mute and swap and what’s the what’s the swap rates and what that kind of stuff. Once you start going down deep into it, the the the, you know, it becomes exponentially more expensive to try and do that in a large model fundamentally because you need to throw so much data on it and and a lot of these domains don’t have a lot of data in terms of the taxonomy or or enough to nudge the weights to be able to do that.
So if you build a a smaller language model and it doesn’t happen then this is the other thing. It doesn’t have to be a transformer. So the transformer model is all about, you know, randomized pattern matching. Basically, look look at dimensionality between the different tokens right fundamentally and then picking what picking the have a random set.
If you actually use, language smaller language models and not just transformer based ones, attention mechanisms, but also you start bringing in some NLP and some heuristics around that as well, and you start guiding it around. And one of the ways of doing it is RAG, another way of doing it actually with with a graph model. You know, there’s loads of ways of doing this. Suddenly, you get a much smaller model, much much more focused.
You the training set becomes a lot smaller. The richness of context gets so much better, so much bigger, like, fundamentally. It’s it’s and and the accuracy goes up because, fundamentally, you’re only pattern matching or you’re only matching, you know, smaller numbers of terms, so you’d be much more accurate around it. Because, obviously, with machine learning generally, every percentage more accuracy has a, you know, an exponential more cost.
So you get to six percent, seven percent, and then going up to eighty percent is gonna cost you, you know, hundred thousand times more time than than that type. So it’s all about improving accuracy. With smaller language models, you have a much better chance of actually doing that. And so we we did one couple years ago doing generating job adverts, Did one which would get a credit score for, actually describe what the credit score was doing for and if you calculate the credit score, so it’s a mixture of machine learning and also language model of, like, a generative model actually generate the report and the output.
And that was a a combined model as well, which worked really well. So that’s the other thing around. It’s not, you know, these ensemble model models. You know?
We talk about mix mixture of expert, but also bringing classic machine learning, other you know? And, again, comes back to what talk about the whole solution. You know, ChatGPT is eight eight or nine models, you know, and this reason it’s made or nine because they’re so solving different parts of the problem. And that’s really where the the the the the the piece goes.
So if you had, like, imagine ten smaller models doing different parts of your piece, you can train all individually. You’re not trying to train one Uber model, thousands of people in far shore locations, trying to do labeling and correcting, all that sort of stuff. That to me is actually from a business perspective. Obviously, b to c is different from a from a b to b perspective, the enterprise.
That is a much more cost effective and actually much more focused and much more accurate way of doing stuff.
Brilliant. That’s where I see things going. Although, I would argue there’s still a bit of a throttle there from the perspective of access to capable data scientists who know how to do that stuff because because I I, you know, I’m not entirely sure those resources are readily available nor is there an appetite with a lot of companies to do that. But I certainly see that being the future from kind of domain building domain specific models that are focused on very, very specific problems, whether it is job advertising or or not.
John, thank you so so much for your time today. Man, I I feel like I could’ve talked for, like, two hours. We could be going on this philosophical rabbit holes about, you know, rows and columns versus text and mesh versus whatever in data products, data as a product. I I I love the conversation.
I hope our listeners got some value out of it. Really appreciate you coming by.
No problem. Well, thank a lot thanks a lot for having me on. It must have been an absolute blast. So, yeah, I hope we didn’t we didn’t get too esoteric in the clouds. But yeah.
Oh, no. Yeah. And and, also, we need a plug for your podcast. You just launched one yesterday. How do I get to it? Is it is it is it is it the data section podcast? What’s it called?
Cool. Data product workshop. It’s, it’s on LinkedIn. There’s a little page on there as well.
So, yeah, if you link to me, or, you know, just find it on LinkedIn. Yep. It’s it’s it’s the idea is we’re actually doing kind of workshops around actually how we actually do stuff. That’s so we’re trying to be a bit little bit different around, you know, some fantastic podcast like yourself, what we do, but this is about more around the doing and actually showing how how we do it.
And I’m sure that’s what I’m thinking. It was about data product storytelling and telling you how we do that. And the next one will be up around, funny enough, around Gen AI and data products. That’s that’s that’s probably the next one.
Yeah. So, yeah, love love people to tune in and just see how how how to do these things. You know?
Awesome. And that and that’s what I love about your content. It’s very specific, and and that, I think, people will get a ton of value out of because it goes from concept into how do you actually do this stuff. So awesome. John, thanks again to our listeners. Thank you for tuning in to another episode of the CDO Matters podcast.
Really appreciate your patronage. Really appreciate you listening. Please give us a like. Please give us a subscribe.
We will see you on another episode sometime very soon. Thanks all. Thanks, John. Bye.
Thank you. Cheers. Bye.
ABOUT THE SHOW
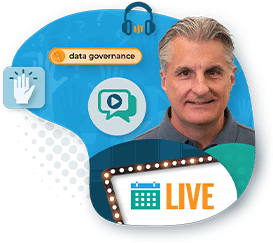