Episode Overview:
In this episode of the CDO Matters Podcast, Robert Hawker (no relation – at least none that we’re aware of!) joins for a discussion on Practical Data Quality – which is all a programmatic approach to data quality that focuses on business benefits, quick wins, and the highest priority challenges.
Data quality issues are a major hinderance to the AI and digital transformation aspirations of most companies, so having a roadmap to resolve those issues is a strategic imperative for all data leaders. Please join Malcolm and Robert as they discuss a practical, value-driven approach to overcoming the perils of low-quality data.
Episode Links & Resources:
Hello, data universe.
I am Malcolm Hawker. I’m the host of the CDO Matters podcast. I am so happy you could be joining us today, maybe through your podcast provider of choice, the Spotify’s, Google’s, Apple’s of the world.
Maybe you’re checking us out on YouTube and you’re seeing my incredibly disappointing video quality of my camera. It seems to be going a little awry these days. I don’t know why.
Or perhaps it’s compression. I won’t ruminate on that. Thank you for joining us today. I’m really happy, to be joined by Robert Hawker. Robert is going to be talking today about practical data quality. He wrote a book on it.
That’s right. And is that listen.
This this oh, I’m holding up a book for everybody on, on podcast, but I’m I’m doing it in a way that, I made a LinkedIn post about this as well, and it was pretty much that exact look.
I really enjoyed the book. I read it maybe about six months ago, seven months ago. When did it come out, Robert?
It came out September September twenty three. So, yeah, Nick coming up to six months now.
Yeah. That sound yeah. That sounds about right. And I think I gave I was I had maybe a sneak peek copy, maybe a preprint copy. I don’t Yes. I don’t I don’t know.
But we’re gonna talk about, data quality today because I don’t it’s something that we all talk about and everybody cares about and everybody’s got an opinion on it, but I’ll be brutally honest and say that I’m not entirely sure people really kind of have their hands around it.
And it’s something that should be near and dear to all data leaders. So we’re gonna talk about that.
Before we get into it, let’s let’s address the elephant in the room, and it’s not AI. It’s there’s not a lot of hawkers in the world, and you got two of them on one podcast, which is which is a little crazy. Now my family, my at least on the Hawker side, most certainly traces itself back to the UK. You are in the UK. Yes, sir?
Correct. Yeah. I live in Reading, which is a little bit west of London.
Oh, you’re like the fourth person I’ve spoken to in the last few days that is from Reading. I just hired somebody Profisee from Reading. I’m working with this guy named Avan from Avanade, named Jed, who is from Reading. I don’t know why. All things keep pointing back to Reading these days.
Cool.
But, I I mean, is that a common name in the UK?
Do you see, like, when you were going to, like, school, are there other Hawkers that that are getting No.
No. I’ve I’ve never come across another Hawker at school. There’s a lot of Hawkins in the UK, but not not many Hawker, surnames. But I think I said to you before, in in the UK, you get signs everywhere.
I think you get it all around the world, but signs saying no Hawkers. And, I used to get people at school taking that very literally. In our local shopping center, we had the no Hawkers sign, and they if they spotted me in there, they’d be looking to chase me out as quickly as they could. It’s so sad.
Yes. I’m I’m sorry you had to deal with that because it’s because, you know, it’s our name. What are we gonna do? It’s not it’s not like, well, I guess we could change it.
But so so the the funny thing is growing up, I used to think that’s what our name meant. Right? Like, I used like, Baker, they they bake things. Smith, they make horseshoes.
Right? Like, I I thought Hawker was the person that that was, like, peddling stuff on the street. Right?
Yep.
And at least according to my aunt or aunt as we say often here in the in the US, she seems to think that we come from a line of people that are like, you know, Harriers, that are like like do falconry.
K.
Did you have you ever heard anything like that?
No. I I thought I I always you have the term in my head of, you know, traveling salesman. Yeah. And, and I’ve always thought it’s that, but I’m I’m much happier to go with your aunt’s interpretation. Well, let’s let’s go with that from now on.
That’s exact that’s exactly right. We we come from a long line of people who use birds to kill things for us.
Yes.
I’m I’m I’m I’m okay I’m okay with that.
Yeah. We’ll go with that. That sounds good.
A little more regal, but I I mean, I’ve you know, in all of my travels to the UK, like, I don’t think there’s any hawkers buried in Westminster Abbey.
I don’t think so, Malcolm. No.
Right? Like, it’s Yeah. It’s it’s not like we come from the gentry for for sure. Right? Yeah. Maybe we’re gentry. If we were the the the hawk people, like, you know, maybe we knew the gentry, but I’m I’m pretty sure we’re not part of that class.
If there’s any if there’s any gentry in my ancestry, it comes from a female line. Definitely not from that male Hawker line, but there you go.
Well, ditto ditto. So I got my name. Here we go. My our guests are kind of like you know, our subscribers are like, what are these crazy people talking about? We’re gonna we we will talk about data. But but fun fact, my my name came from a name from Scottish kings. So there were actually three Scottish kings named Malcolm.
One of them has had a nickname of Big Head Yeah. Which in gathers Cianmore, Cianmore Cianmore, Big Head. And Yeah. The place I was married, interestingly enough, in Canada is a place called Canmore, which was named for King Malcolm Big Head. There you go.
Brilliant. Well, I’ll tell you what. If we’re gonna talk about where Christian names come from, I have an an a a silly story there as well. So we had a dog before I was born. There was a dog called Robbie in the house, and I’m called Robert. And my mom has always called me Robbie, So I I’m pretty sure I was named after the dog, so like Indiana Jones.
Alright. So so from such humble beginnings, that our our our our podcast listeners and our YouTube viewers get to be given insights and experts and and expertise and knowledge from, somebody named after a dog and somebody named after a Scottish king with a giant head.
So let’s do it.
Fantastic. Let’s do it.
Alright. What I know this is a this is a loaded question, but but but it but it has to be asked because it was the title of the book. What in your mind, what does what does practical data quality mean? Sounds good to me. Put put it put it on paper for us.
Well, I just I I am I like to say that I’m a very practical person, first of all. I’m not someone that’s gonna do something theoretical for you. I get my hands dirty. I get involved, and I I I deliver things. That’s just who I am. And I think I wanted my book to be like that as well.
So what you find throughout the book is it’s filled with real life examples from various places that I’ve worked. So every single concept that I put into the book, I tried to very quickly then bring a real life example to that where you could see how you’d apply that concept. So there’s very and and, you know, there are templates that come with the book. There, you can see actual reports that I’ve used in the past, for showing monitoring of data quality.
So I tried to just keep it incredibly practical and usable all the way through. So that’s that’s where the title comes from.
I like I like that, and I like the I really enjoyed the real world stories that you shared in the book because I think I think for many, data quality is is far too theoretical and conceptual.
Right? It’s kinda like world peace. Right? Like, nobody’s gonna argue against world peace, and nobody’s gonna argue against data quality. That sounds good. I want more of that.
But but I think some of the challenges that we have is, like, what does that actually really mean? So when it comes to implementing a a data quality program, I’m a CDO. Maybe I have one. Maybe I’m inheriting one, or maybe I’m thinking about getting one off the ground. What what are the few things that I really need to be thinking about in order to take more of that practical approach?
Definitely. I mean, be before I answer that, I’ll just say one more thing about practical, here. Because, actually, I think of all the areas of data management, data quality is one of the most practical because you can very quickly just identify a set of rules that you know your data should comply with. And then you can very quickly, with any tool, like Excel even if you want to, measure how much of your data actually complies with that rule and how much of your data doesn’t comply with that rule. And then straight away, you can give a score to your data.
To some sort of business success. Right? So it just is a very practical part of data management. You know? Whereas if you think about elements of data governance where you’re appointing data stewards and you’re trying to get them to change the culture in their part of the organization, that’s really, really important as well. But it doesn’t yield the practical measurable results that data quality does. So that’s that’s another reason for the name practical, I guess.
But coming back to your question, what does CDOs need to know and think about data quality?
So I think the first thing that a CDO would need to assist their teams with on data quality is coming up with a compelling business case. And this is a really tough area because, actually, you don’t know the the whole point of data quality measurement is that you wanna find out how much of your data is good and how much of your data is bad. And so at the beginning of that, you know you’re gonna need to spend some money to do that, but you don’t actually know what the benefits are going to be upfront because you don’t know how the extent of the problem.
So, you know, coming up with that compelling business case, when you’re up against the project, you’re usually up against a range of other projects that want investment as well. And those projects have got we’ll be able to reduce the number of FTEs in this area by x, and that comes with a value of y. And, you know, it’s very tangible, measurable stuff. Whereas with data quality, when you just don’t know the extent of the problem yet, it’s really hard to do that. So we can talk a bit more about that later, but that’s one of the key things a CDO needs to be supporting the team with.
Then I think the next one is just tying into business strategy because, you know, you can you can take any set of data and you can profile it and look for issues. Hey, that field’s only got twenty percent of data in it. That field’s got a huge variation of field lengths, all that kind of stuff you can come up with. You can pick holes in any set of data that you want to. Right.
And then you can go and fix those holes. But actually, unless you’ve aligned to your business strategy, you could be burning time on things that don’t matter. So, you know, a big part of this work that the the senior leader needs to help with is understanding what what will really shift the dial for the organization you’re working in and how to link that to the data itself and and focus only on fixing the things that need to be fixed to drive value for that business strategy. So, for example, if, if you’ve got a business that’s trying to shift its customer base from direct end users to wholesalers or something like that, then you need to be there’s no point spending tons of time fixing the end user customer data quality, millions and millions of records.
The ones you really need to get right are the wholesalers and all their locations. So that kind of thing is very important. And and you sometimes as a senior leader, you find that your teams on the ground are disconnected from that strategy, and they’re running around fixing stuff that doesn’t necessarily need to be fixed.
I think the next one I would mention would be data quality cannot just be a one off exercise.
So many times, I’ve come across organizations that are trying to bring in consultants for a fixed period of time to clean up the data. When I first became a leader in data, actually, my predecessor was about to employ an organization, large consulting organization with an offshore team who were just gonna take a look at our supplier data and fix it. That was without talking to anyone within the organization to understand what was really important within that supplier data.
And and, you know, I’m sure they would have made improvements. They would have found VAT numbers and, company IDs and things like that, but they wouldn’t have found the things that really mattered to the organization. At the time, the organization was trying to work on spend analysis and understand how much money was being spent with certain groups of suppliers. So what they really needed was was vendor parentage hierarchy information.
So, you know, there’s no I’m I’m sure that the third party wouldn’t have focused on that. So it it can’t just be solved by throwing money at it for a short term period, bringing consultants in and fixing the problems. It has to be embedded in the organization, and it needs to be repeated over time. And you need to be constantly monitoring it. It doesn’t stay fixed, basically.
And then a cup a couple more things.
So I actually think that for a CDO starting a new data organisation, data quality work can be a really great place to start because it does deliver those tangible results. Obviously, it’s easier if you’ve already got a data governance initiative up and running because you’ve got data stewards. You’ve probably got data definitions telling you what good looks like for your data, things like that. But if you don’t have any of that up and running, the quickest way you can deliver value, I think, is to start to identify the key things that are wrong with the data, linking that again back to the strategy, providing some quick wins.
So, I mean, I I I fell into that discovery at an organization that I joined to I I joined an organization to start the data team from scratch. And when I joined, it was easy, actually, from a from a certain perspective because I was pushing on open doors. I’d been used to trying to persuade people to get excited about data quality, but the quality was so bad in this organization that they were desperate for somebody to come in and do something about it. So I was straightaway monthly going to the board to report on data quality. And, actually, it was very easy to move the dial for them quickly because they could again, back to being practical, you see the tangible results and you make a difference and they can see that improvement in the numbers. So so I do think that’s a great place to start.
And I I think those are the main things, I would say, for the time being.
So to to to summarize you need to you need to focus on outcomes.
Number two, you’d need to, align to a business strategy.
Avoid random acts of data quality.
But we can talk about this some more because there’s plenty of random acts of data quality happening out there.
Number three is it’s a program. It’s not a project.
And and and number four is it’s a great place to start. It’s a great it’s a natural kind of prioritization thing or way to prioritize, way to galvanize the organization around making improvements to your data management.
Absolutely. Absolutely.
So let’s press on the on the on the let’s we’ll press on all of them, but let’s press on the first one first. The first one first.
Let’s press on number one.
And and what I heard you say, I’m paraphrasing you now, is is that you will be able to establish benchmarks of what good looks like, and you’ll be able to measure against those.
And you could be able to maybe put put together some metrics that estimate what the impact of that would be, at least at least on at least on the data side of the house. An example would be you you have something that consistently breaks data pipelines, and if you didn’t break the data pipeline anymore, you’d be able to do a b c or some something else. Or you have some companies are still sending snail mail. I actually had a conversation with a company last week that is still sending, like, Royal Mail snail mail.
Wow.
Maybe your return rates are ten percent, and that costs you a certain amount of money based on local but you can come up with some estimates. But what I did hear you say, though, is that it’s gonna be really difficult, at least in the short term, to come up with very robust metrics of what the improvements are going to drive from a business outcome perspective. Did I did I did I paraphrase you correctly?
Yeah. A hundred percent. So let me try and give an example. So one organization that I worked for, was really struggling with a huge number of queries coming in from suppliers.
So the suppliers were questioning money that they’d received in their bank accounts, because they were not receiving remittance advices. So they the remittance advice provides you with a breakdown of, hey. I’ve just sent you ten thousand dollars, and that ten thousand dollars goes across these three invoices that you sent. And they can then allocate it in their systems and and close all the invoices down.
But the company that I was working for was struggling with the data quality, and one of the things it didn’t have was the correct remittance advice email address details. So most suppliers weren’t receiving a a remittance advice.
And they were then coming to the accounts payable team and asking them, what’s this money for, which invoices that get allocated. And the the number of queries coming in far exceeded the capacity of the team to deliver it. Now, you know, it the they could you could count the number of queries that you were getting, and you could count eventually the number of the number of suppliers that were missing the email addresses, and then you could you could fix those email addresses and create some kind of estimate of how much the the queries were gonna drop by. And then you could you could think of you could try and come up with a cost per query, and then turn that into a an FTE number. That’s that’s that’s a real example of how you’d come up with a business case. But remember, in a data quality program, you’re you’re usually talking about hundreds of rules, hundreds of things that need to be right in the data.
And so that estimate I’ve just roughly outlined, would probably take a few hours to think about and validate and get all the assumptions in place. And you’ve got to multiply that few hours by, say, three hundred rules that you want to develop.
And you’ve got to come up with clever ways of estimating how much the benefit is going to be of each of those three hundred rules. It’s just not economical to do. You could spend just as long coming up with the estimates of the benefits as you could actually resolving the data problem if you’re not careful. So the the the challenge is you’re going in and you’re saying, I need to spend some money to build a data quality program, but I can’t really tell you completely what those benefits are gonna be yet.
I can give you some examples of what the benefits will be. I can pick on five rules, tell you precisely what those benefits will be, but you’re gonna have to take my word for it that the other two hundred and ninety five rules are gonna add some kind of equivalent value. You know? So I do spend quite a bit of time in my book talking about how to make that compelling, that case.
Well, you just touched on it, which is to me, in my experience, have having been in these positions before, the one or the two cases that are, like, the glaring, in in this case, you’re causing a lot of headaches to your your suppliers, unhappy suppliers. Right? Yeah.
Souring those relationships. But in that one case or the or the case I gave, the snail mail. Right? Maybe sound sounds antiquated, but it’s still true. It still still happens. But there’s there’s in any given company, I would argue there’s probably tens, if not hundreds, of of major well known relatively high profile where the one one thing where you could come up with some estimate, where that one thing in and of itself could justify pretty significance in pretty significant investments in data quality. Do you agree?
I do. I completely agree. And and and, I mean, I I always think of three different approaches to this. So number one is you cherry pick those rules where you know there’s a lot of value. You calculate the the business case for those rules specifically, and, usually, you can deliver enough benefit that it’s you can justify the investment. You can get to a point where the rate of return is high enough just from a handful of those rules, and treat the other rules then as a bonus, if you like.
You can then Right. You could you can then do the the second approach you can take is to calculate again for a handful of rules and extrapolate across the others. So you can and and you can say, well, we think we’re gonna deliver fifty thousand dollars of benefit for these three rules. So if we’re gonna deliver three hundred, we can times that by a hundred, but you you don’t be as simplistic as that.
You you would you would say, for us to pay for the project, we would only need the other two hun the other two hundred ninety seven rules to deliver a further fifty k of benefit. So you say, I guarantee you fifty k of benefit, and we think there’s a lot more than fifty k in these two hundred and ninety seven. But even if there isn’t, even if there’s only fifty k, you still get your money back. You know, that’s that’s the second approach, that extrapolation approach.
And the third the third approach is to go top down. So you start looking at benchmarks. So you can go to organizations like Hackett, for example, and you can say, organizations typically in in my sector are able to do supplier, management with a team of three FTEs for my size of company and my my industry and all those kinds of things. We’ve currently got fifteen FTEs.
So and we we attribute five FTEs of that problem to data being an issue. So we believe we can move towards that benchmark, and that will save us this amount of money. That’s that’s, you know, a third way of doing it. And I think it really depends on the culture of your organization as to which of those is gonna work best.
That’s that’s great advice. And and something I would say and and really stress to our listeners and viewers, One of the things that I really enjoyed the most about Robert’s book, not the fact that it was written by a hawker. I do enjoy that.
One of the things that I’m I really enjoyed the most is that a lot of these stories, a lot of the narratives, a lot of the kind of the anecdotes that you use always tie back to op what I would call operational uses of data.
And I think we have a little bit of a problem in the in the data and analytics world where so many people align data quality issues to analytical problems. And and and don’t get me wrong. Having reports that are incorrect is a problem. Right?
Having incorrect reports, giving data to your CEO that is patently wrong is is a problem. But I think that in many ways, talking about data quality, especially using a very broad definition of it in the analytics world, can often lead to really academic undertakings and academic conversations that really maybe not don’t have anything today to do with data quality at all. Yeah. I’ll give you an example, and I love your perspective on this.
There there there have been multiple sound bites published over the years talking about how much time data scientists lose lose to data quality issues.
Yeah.
And when you peel that onion, what you find is that what the data scientists are doing is what I would call data wrangling. Yeah. Right? They’re they’re they’re creating pipelines.
They’re creating ETL processes. They’re doing they’re transforming data. They’re normalizing data to get it in a in a form where it can be used to build models or can be consumed by a Python script or or something, but it has to it has to go from being optimized for operational use to optimize for an analytical use. And I fundamentally disagree that that’s a data quality process.
What do you what would be your response to that?
I I would agree with you completely. I think that is just a natural consequence of having multiple different datasets that you need to bring together to get the result that you want. So the data in each individual system can be perfect, from that system’s perspective. But when you start to bring the systems together, then it becomes a problem. So, again, to give a real example of exactly this, a manufacturing organization that I worked for had an SAP system where everything was driven by batch number. So, you know, this particular batch has gone through these processes on these dates and things like that, and we managed to get the yield of x, you know, coming from SAP.
But the manufacturing system had all the really interesting data in it that you could use in multivariate analysis, for example, to see what was going on and what was driving yield. So things like temperatures in every work, every space in the in the manufacturing site, humidity, all of those kinds of things were very important to the process.
And that manufacturing system didn’t have the batch number from SAP. There was no way of tying together the manufacturing data and the batch data in SAP from the two different systems.
You could only tie it together using dates and times. So you could say, we’ve got these readings between this date and time and this date and time, and that seems to tie roughly to what was in SAP for that batch at that date and time and that date and time. And you could tie the two things together that way, and data scientists were doing that. But, again, there was nothing wrong with the data in the manufacturing system. There was nothing wrong with the data in the SAP system. They just they needed wrangling to get them together. So that’s a fortuitous example that that fits exactly what you were saying there, definitely.
I’ve had interesting conversations with people online who who vehemently argue that data quality is both a noun and a verb.
Okay.
Me meaning it’s a it’s a state of data, and it’s also a process.
And it’s true that it is both. Yeah. Right? Data quality can is a discipline. It could be a process. But in the case of the use case that that that I just described, I I just don’t think to your point, if the data is perfectly fit for purpose in the operational system and we in the data world have to do something to it to make it easily consumable in an analytical world, that’s I think that’s just that’s just the way it is. I don’t think that’s data quality, but that that’s just me.
No. No. But I I I think, actually, interestingly, you’re making me think now, it does then fall within my definition of bad data in my book. So what the way I define bad data in my book is I say that basically, data is kept in an organ you only bring data into an organization for kind of four purposes, really. One is to run your processes.
Two is to make good decisions.
Three is to enable you to be compliant with laws and regulations.
And four is is to actually sometimes use data as a product or or a revenue stream. That’s not applicable to every organization, but it’s becoming more and more applicable. I mean, very quick example on this. One of my previous organizations started to take the data from their their customers of stock levels of their product, and they started to manage the inventory and and the stock from their customers as a service. And it made them quite sticky. Exactly. It’s so they’re they’re combining the real product, the physical product with a data product, and they’re making themselves quite sticky then as a supplier for those companies because it’s quite hard then if you if you give that service up, you you’re buying a different product and you you suddenly need to manage all your inventory again.
So for me, my definition of bad data coming back to my point was if you’re if if the data is intended for one of those four purposes and it doesn’t meet one of those four purposes, then it’s bad data. So, actually actually, my my example of the SAP system and the manufacturing system, it has become bad data because from a system silo perspective, it’s okay. But when you actually bring it together for the purpose that it’s intended for, it’s it’s not meeting that purpose. So it is, from my definition, bad data.
But then it’s not actually correcting the data in the operational system. It’s adding an enhancement to that operational system. So the enhancement in this case would be to add a batch number field into that manufacturing system so that whenever a particular batch was running through a process, you’re tying the humidity and the and the temperature to that batch at that time.
And then I think so so for me, it’s a it’s a really interesting one. I’ve I’ve accidentally flip flopped between the two. Well, there you go.
We we won’t tell your publisher. Oh, well, you’re no. Well, you’re consistent with your book. It’s good.
Yeah. It’s fine. But, I mean, I think it’s okay I think it’s okay, because we in the data world, we’re librarians. Right?
We need to label everything. We need to define everything. We need to classify everything. It’s it’s just how our brains work in the data world.
That’s okay.
I think the problem arises that was when we start complaining about it to the business. Yes.
I think that’s when the problem arises because the business is like, hey. What the heck?
Yeah.
Everything’s working here. I’m hitting my SLAs. We’re pushing product on time. We’re we’re exceeding customer expectations, and you’re telling me my data’s bad.
Yeah. Absolutely. And and, again, this is another thing I I can’t say enough about in the book. It’s, you have to make that link between the peep the data producers.
So this is my name for the people that actually put data into systems. You know? So it could be in my old world, it’s the manufacturing staff who are putting the putting the data in about that batch and what’s going on with that batch. They’re data producers.
They’re producing data in the SAP system or whatever. And then you’ve got the data consumers. So the people that use reports, the people that benefit from those processes, the people that work with the regulator, all that kind of stuff, They are consuming the data, and the data stewards and owners need to bring those two two groups together, the producers and the consumers.
And and they need to educate the producers on what is important for everyone that consumes that data. And so in in you know, going back to our example, those data producers would need to understand we’re gonna enhance that manufacturing system to include the batch number, and they must include the batch number in every time they run a process in that manufacturing world.
You know, that’s that that if you don’t tell them, then, of course, they’re gonna hold their hands up and say, what you know, I’m doing everything you’ve ever told me to do, and you’re still saying the data’s bad. It’s all about that communication facilitating that.
Indeed. So so getting back to the four things that CDOs need to know about data quality and to kind of focus on, your your number two was and I’m this is my phrase, not yours, but it’s avoiding the random acts of data quality.
I think I think that one that one to me makes sense. Right? Because in top three deliverable for any CDO, generally in the top three, number one is almost always gonna be data strategy. And if you’ve got a data strategy, you know what’s important, the data strategies, the life of the business strategy.
You’ve got your top five things that you need to execute against. And if one of them is better customer or improving your customer relationships, then maybe focusing on your employee data or doing an employee data cleanup just doesn’t align to your strategy, then don’t don’t bother doing it. I think that’s that’s basically what you’re saying. Yes.
Your number three about a program. Let’s let’s talk let’s talk about that more because and I and I already know the answer here, but I wanna I well, I think I already know the answer here, which annoys my wife because I almost always think I know the answer.
But I’d love your perspective. When I was at Gartner, you know, data quality and MDM were were kind of going at it, you know, neck and neck for the award for the program that loses steam most often.
Yes.
Right. Right? Like, the one and done.
You know, we did MBM and then we lost our funding, or we did data quality and then we lost our funding.
To a CDO that may be facing that or concerned about that, what would you what would you say?
Interesting question. I mean, I think first thing, I’ve I’ve been an MDM person as well. So, you I implemented SAP NetWeaver MDM, which is an older product.
Long lost brothers here. Long lost brothers. Yeah. Keep it going.
And then implemented MDG as well, which is SAP’s more recent product.
And, you know, we we created centralized processes for creating catalogs of product data, and we brought down some of the SLAs for that for kind of thirty days all the way down to kind of a few hours, things like that. So we you know, I’ve been there and done it with MDM. I think for me, over time, m the business case for MDM has become a little bit less easy to get across the line.
So especially if you think about customer and vendor in particular, those were traditional areas where you do a lot of MDM work. And I think, you know, when I first started in this this area, you were typically using spreadsheets. You know, you’d get a supplier to fill out a spreadsheet. You’d send out that data to your procurement teams and the finance teams and your master data teams and get everybody to approve and contribute and things like that.
And but these days, you’ve got, you know, supplier portals online where the supplier will provide their details, and then an automated workflow kicks off and collects all the information you need. And then eventually, APIs will post it into your ERP system or something like that. And the role of MDM gets a bit lost in that process. I mean, it can still be really important for consolidating and harmonizing data, but the the kind of central MDM process is less tangible these days. And I I found it really hard to get that off the ground, when I was leading a data and analytics team.
I think coming back to the real question, how do we avoid losing our data quality initiatives? I think it’s it’s, starting small, first of all. So just picking that really fundamentally important area. So, you know, when I joined the business I talked about before, suppliers were actually pulling the plug on arrangements with that organization. They were we even had a risk of the electricity going off in a manufacturing site, which would have been absolutely horrendous.
So the board had their eyes firmly on that issue. So, clearly, we just needed to sort out supplier data in, you know, a very short period of time and move the needle. And to be honest, just by achieving that, we we then gained ourselves a great deal of grace for a a long period of time to invest in making that sustainable and building other other data types into it. So I think start small, deliver quickly, is an obvious piece of advice.
I think the next piece of advice is to produce really tangible numbers on your data quality as quickly as you can. So be able to say, look. We we are getting a score of x percent on our product master data at the moment based on these rules, and we’ve shifted that from x percent to y percent. And that means this for the business.
Here’s some of the wins we’ve achieved to this this this period. You know? Those are the things that that sustain your data quality program.
I think the other part of it is is thinking ahead. You know, quite often the programme focuses on that first critical issue and then and you’ve got all your staff all aligned and and you’ve you’ve got people really working well as a team. And then that initiative ends and there’s a gap, And then you have to start all over again and get the teams back on and get everyone interested again. So it’s like keeping that consistent push going and planning ahead and making sure you’ve got the next area in the next area gradually interested.
So Love it.
Yeah. Yeah.
So so so so what I heard hear you say, start small. Yeah. Deliver value in bite sized increments.
Celebrate your successes. So it’s something that I I I heard you say. Like, shout it from the mountaintops. Put put something over the water cooler. Make a T shirt.
Whatever it is, but shout it from the top.
But but still, get the business folks who you helped to shout for you.
I think I love that.
Yeah.
Yeah. I’m not I’m not the the greatest at shouting about my own successes, to be honest. I I prefer to get someone else to do it for me. And if it’s the business person you helped, it’s just so much more authentic. You know?
Yep. And and do that, wash, rinse, and repeat, and just keep doing it over and over.
I I love I love that advice. That is very, very sage advice. Thank you.
However I’m playing devil’s advocate here.
It’s allowed. No problem.
Okay.
But I did hear you say earlier that, you know, a big a big part of this is defining rules. Right? How do I define data quality rules? Right? How do I know when something is is accurate? That sounds like governance to me.
And so what you’re saying is is that I’ve gotta define my governance framework before I can start on data quality, or or or is there a different way? Because because No. I mean made a covenant sounds hard, and that sounds like a lot of work.
Yeah. I I think that, ideally, it’s easier for you in a data quality program if somebody has defined data definitions. So the definition is the description of what a particular field or fields is for in in your records.
And, usually, when you define a a data field, you pretty you include a little bit about what good looks like. You know, you say this field typically should contain x.
And that helps that gets you halfway there, and that’s really helpful. But, again, when I joined the organization I talked about earlier as the first employee in data and analytics and establishing it from scratch, we didn’t have any of that, and we started first with data quality. And that did mean we had to do some rule rule definitions as we went along, but it it’s not as hard as it sounds. I mean, you you’re basically looking at what is the business problem that we’re trying to solve, what is the cause of that business problem, and it’s usually a series of causes.
It’s it’s always the, you know, small things chipping away at your success. And then you ident you you get yourself a spreadsheet list of all the fields that don’t seem to be correct and seem to be causing problems. And then you say, well, what what should be in that field then? If it at the moment, we’re seeing that’s empty fifty percent of the time.
Is that okay?
And you speak to your business people that know that data and know what it does, and they’ll say, no, that’s not okay. That should be one hundred percent. That field should be, always containing numbers, and half the time it’s got numbers and alpha characters in it. And, you know, very quickly you can derive a reasonable set of rules and start measuring the data against those rules. So it it definitely helps if someone’s come along and done that first, but, you you know, you can do both in in parallel and and deliver.
And and then you’re not just doing the kind of theoretical data governance stuff. You’re doing theoretical data governance stuff. At the same time you’re delivering practical results and improving things with data quality.
Love it. I mean, the the what you said is figure out what problem you’re trying to solve and then define the rules needed to solve the problem.
Yes. Correct.
So so I would call that an MVP, minimum viable product or a minimum viable what whatever is whatever is needed just to solve that problem.
I I you know, we we are kind of prone to want to implement frameworks. Right? And I I heard this often when I was at Gartner. It’s like, you know, do you have a good framework for data governance?
I was like, yeah. There’s lots of them. We have one. Donna has a beautiful spinning wheel.
Yep.
That I think you you quote actually in the in the book that you made reference to the to the Dama wheel.
And it’s great. It’s great. But you don’t have to figure everything out on the wheel. You just need to figure out what’s needed to solve that problem.
That is Correct.
That is wonderful advice.
Yeah.
Alright. Our last question. Of course, we can’t have a podcast these days without talking about AI.
No.
What what do you see what do you see are the short term benefits of of of AI, longer term benefits of AI when it comes to data quality?
And and maybe if I’m a data quality professional, net net is is is is AI a a force for good or something I should be concerned about?
Okay.
I I’ve thought about this fairly long and hard, and I think that, actually, AI is gonna be really valuable to data quality professionals.
I think that one of the challenges of a data quality program is that those of us that instigate one are usually business focused people. I mean, my original career was accounting. I was a financial accountant.
And so we we tend to come from those business backgrounds and end up in data, and we don’t tend to be coders and developers.
So something what what you always end up doing is you define those rules, and then you hire, often from a consultancy, a third party, people that can actually codify those rules into a data quality tool.
And I think that AI is gonna be built into all of those tools over time so that you’ll be able to write in natural language what your rule is and and link it to certain fields in your dataset, and then the AI will generate the code. So I’m not saying you won’t need any developers anymore. Clearly, you’re gonna want developers to check over that that code and make sure it’s doing the right thing. I mean, these days, I’m a Power BI geek, writing code regularly.
And, and I I I I use GPTs to help me generate code when I’m stuck, but I still need to understand that code well enough to know that I’ve done a a a good job. So you there’s still a role for those developers. But where your program might have had five developers, they’ll probably be able to have one. And so going back to this really challenging business case that we talked about earlier, suddenly the business case becomes a lot more compelling because the costs go right down.
So I think you’ll be able to get that. You’ll also be able to go the other way around. So when you’ve written a data quality rule that gets turned into code, you’ll be able to then get something like AI to write a data definition, which will speed up your data governance program. So if you have started down the data quality path without doing data governance first, then I think you’ll be able to retrofit some of the work you’ve done and get the benefits for data governance quite rapidly.
So I can see plenty of benefits there. And I think, also, the other the other benefit really is a kind of a, a side benefit. But remember that your whole organization at the moment is thinking a little bit about how it can use AI to drive revenues, reduce costs. You know, every every every company is doing this right now. It’s thinking, how can we jump on this Agreed. Bandwagon.
And the one thing I’ve I’ve I’ve not heard as much consensus on data quality until now, really. Every organization is not just saying we want to do AI, but they’re also saying, how are we gonna be successful with AI when our data’s so bad? Our data quality needs to be improved so we can get the best out of AI. So guess what? Suddenly, a lot of doors have just opened for you data quality professionals because people have recognized that they need they need, the data quality to get to a level to get the real value from AI.
And I would argue, as long as we have functional distinctions in our organizations, as long as we have sales versus marketing versus procure versus isn’t the right word, but sales, marketing, procurement, HR, finance, you name it. As long as those functional silos exist, there will be a need for something to resolve differences that naturally occur because those people speak different languages, they use different tools, they have different different perspectives, different goals. Yeah. And that means job security for people who do MDM, who do data quality, who data data integration, everything that you just spoke to.
Hundred percent. I put in agreement. Cool.
Alright, Robert. This has been awesome. I’ve I’ve really enjoyed the conversation. I really I I, again, I really love the focus on operational uses, real world examples of what does it mean to improve your data quality.
Thank you.
Practical data quality by my long lost brother, Robert Hawker. Please pick up your copy now. The publisher is packed, p I c k t.
Enjoy your evening, Robert. Thank you so much for joining.
Thank you. Thanks for having me. It was brilliant. Thanks.
Wonderful. And to our listeners, to our subscribers, to our growing CDO matters community, thank you for tuning into another podcast. I truly hope you got value from this. I hope you will join us on another episode sometime very soon. Thanks all. Bye for now.
ABOUT THE SHOW
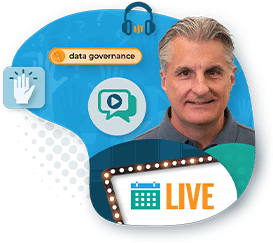