Episode Overview:
Unleashing the power of Data Products, and transforming CDOs into Product Visionaries.
In this the 28th episode of the CDO Matters podcast, Malcolm explores the topic of Data Products and what CDO’s should know about them. Malcolm taps his expertise as a prior Chief Product Officer to explore the differences between data products as they exist within a Data Mesh architecture, versus the more general concept of data products outside a Mesh. Malcolm takes issue with the idea of a ‘bottoms up’ approach to defining data products, and instead advocates that CDOs take a more practical view of a data product as anything that a customer is willing to pay for.
Malcolm urges CDOs who are curious about data products to ensure they first establish a business justification for their efforts – one which focuses more holistically on the benefits that can come from the integration of the discipline of product management into a data and analytics function. Malcolm shares his insights that data products should be treated as an output of a product management focus, and the many benefits that are realized when CDO’s embrace the discipline of product management.
Lastly, Malcolm dives into the role of a data product manager, sharing details on the core responsibilities for these experts and how they would differ from the concept of a data ‘owner’. Along the way, Malcolm touches on the misguided idea of data literacy, and how taking more a product-centric approach to building and supporting data solutions could enable CDOs to completely transform their customer relationships.
Episode Links & Resources:
Hi. I’m Malcolm Hawker, and this is the CDO Matters podcast, The show where I dig deep into the strategic insights, best practices, and practical recommendations that modern data leaders need to help their organizations become truly data driven.
Tune in for thought provoking discussions with data, IT, and business leaders to learn about the CDO matters that are top of mind for today’s chief data officers.
Good morning, afternoon, evening, whatever time it is, wherever you are.
I’m Malcolm Hawker. I’m the host of the CDO Matters podcast. I’m thrilled that everybody can be joining me today, whether that is through your podcast provider of choice, the Spotifys, the Apples, the Googles, the unite the you name it, or on YouTube or through prophecy dot com or any of the other channels we use to distribute this content.
I really appreciate you taking a listen or taking a watch. I don’t do this much, on the on the channel. Don’t really kind of advertise. But, if you do appreciate the content, if you like it, feel free to subscribe.
It’s free content, and I’m doing this every other week. My mission, in case it wasn’t abundantly obvious by the title, my mission is to extend the tenure of chief data officers to support chief data officers and anybody that wants to be a chief day data officer.
There’s lots of data out there to suggest that CDO tenures are pretty short, at least by comparison to their CIO peers. CIO tenures are ranging around five years, and CDO tenures are ranging around two and a half years. A lot of theories for why that is. Chief data officer is still a relatively new title.
The roles are generally poorly defined.
There’s a lot of them.
A lot of government agencies have now required actual chief data officers. So there’s a lot of titles out there, and there’s a lot of people that are moving into those titles that don’t have a ton of experience in the space. So there’s a lot of different reasons why, but it’s it’s really my goal here, guys, to to help provide information, best practices, insights, practical, actionable insights the CDOs can use to improve the way that they manage their data. I am an ex Gartner analyst.
I’m an f g ex, head of a of an IT function within an organization. I’ve implemented a data governance program. I’ve implemented master data management. I’ve helped define data strategies.
You name it in the data space. I’ve done it. I’ve been a consultant. I’ve been a vendor. I’ve been an analyst. I’ve been a leader, a practitioner.
So about every hat imaginable, I’ve worn it. And now through my current employer, Prophecy, it’s my mission to help CDOs. We at Prophecy believe that the more that I’m doing that, the more content I’m providing, the more insights that I’m providing, the more I’m helping create and support a community, the more that you will view my company positively. And if you should ever happen to need MDM software, we happen to make a pretty good solution. We think it’s, we think it’s excellent. So, that’s the win win.
What am I talking about today? So that’s an end of advertising.
I just just thought I would, I would throw that out there. It’s probably good to repeat that every now and then, kind of my mission and my reason and my why. That’s actually been one of my LinkedIn posts recently. I talked about your your north star because I think it’s important to have one. We all need to have a mission. We all need to have some goals. And when it comes to this podcast, my goal is to help chief data officers.
So that’s that.
Today, I’m flying solo.
Usually, I have guests. That’s my preferred approach. Today, it’s not because I didn’t want to have a guest. I’ve had other guests on. We’ve talked about the topic, today, which is gonna be data products.
I have an episode dating back a few weeks ago now with a gentleman named, Salim Khan, who’s the chief product officer for a company that sells data. So a combination of chief product officer and chief data officer are all rolled into one. Love that conversation with Celine. Such a super, super smart guy.
We’ll be having more conversations with product leaders, with data leaders. But for today, I wanted to focus on on on on data products and and have it be me because I think that I actually do bring a little bit of a unique perspective here.
Tracing back before I went way down the data and analytics rabbit hole, I was actually chief product officer. I I, I was working in a product related function, going back as far as nineteen ninety five. Yes, folks. This is real real gray hair.
I held many product related, roles at a little Internet startup called America Online, AOL, believe it or not.
Kinda worked my way up the product ranks there. I was, I I read I led a p and l for one of AOL’s products, including, I was the lead product manager for their all of their dating products, including, at the time, something called love dot com. Wow.
Yes. Believe it or not, I was the product manager for for a dating site, which was, one of the earliest dating sites by by the way, which was a was a blast. Kinda worked my way up through the ranks today. Well, was recruited to run a product organization for a company out of Austin called hoo called Hoovers.
Led a product team there, was recruited out of Hoovers to to be a chief product officer at a small start up, in Austin that is since long gone got bought up by a company called NetSuite, but I ran a product function. I hired and retained product managers. I had product PNL.
So I come by my insights in the product world honestly, although I have been, faithful, as it were, to the data world for for at least the last, whatever that math is. Good grief. I’m getting old.
Fifteen, twenty years.
But but my first kind of love, my first corporate love as it were, is is is in and around product management.
And I actually think that data people make can make really good product managers because at the core, product management is about problem solving. It’s about understanding customer needs and then translating those needs into products.
And I think that if you’re really good at, business analysis, if you’re really good at product management, I think you can actually translate that fairly well into the data world, and vice versa. But, gonna take a bit of a different approach today.
My apologies for anybody who may be just listening, today. I’m gonna share a few slides. So if you are on YouTube, you’re obviously going to see them, assuming my scribe my slide share works.
But I’ll be reading a lot of that content. So my apologies to my viewers on YouTube, who are watching me read things to them and you’re like, dude, I can read, just remember that a lot of the folks that are taking in this content are doing it while they’re driving their cars, or mowing the lawn or whatever, and they’ll have the, the benefit of visual aids here. So why don’t I try to share my screen? Hopefully, this works. And, hopefully, everybody is seeing, a large version of the PowerPoint.
And if not, well, then you get to see all of the the menu bars and everything else in it. So let’s talk today about data products. Why am I talking about data products?
Well, frankly, because I’m hearing a lot about data products out there. I’ve been to a lot of events the last year. I’ve spoken at multiple events. Data products have been a common topic at at those events, and Data leaders are asking a lot of questions about it.
If the indication if the traffic on LinkedIn in LinkedIn related to data products is any indication, there’s a ton of hype around data products. And what does it mean? What does it not mean? Is data as data products, is that the same thing as data as a product?
Is that the same thing as data product management? What’s different? What do I need to know? If I’m a CDO, I’m hearing a lot of buzz about this, and a lot of people saying they’re doing it.
I’ve certainly talked to a lot of companies who are saying they’re doing data products.
And if I’m doing it, why?
What does it mean to me? Do I need to build out a team? Do I do I need to buy some new technology? Do I need to adapt my processes? As a CEO, why are so many people talking about this, and why is it important? That’s why we’re talking about it today.
So let’s step into it. So when it comes to anything that we do in the data and analytics world, we need to know why. Right? Why?
What problem are we trying to solve? The time anytime we are in the data world or doing anything that is just for anything’s sake. We’re doing governance for governance sake. We’re doing quality for quality’s sake.
Red flag. Don’t do data products for data product’s sake because you’ve heard about it or because a lot of people are talking about it. That’s not why. So first and foremost, as a CDO, you need to ask yourself, okay. Why am I actually thinking about this? Why am I having folks on my team investigate data data products or data as a product?
Why? Well, you know, how does that so so you gotta figure out why.
And how do what you’re trying to achieve here, how does that actually fit to your overall data strategy? How does that fit to your overall business strategy?
And and the why here, we need to be really careful and and separate why from how.
K? We’re gonna talk about how, but the why would be things like increasing your customer satisfaction, improving your operating efficiencies, lowering your business risk, better internal decision making.
The list of why here, it it it could be very, very long, but let’s keep in mind here, folks, that the why needs to align to your strategy and the why needs to be articulated in a way that the business can measure.
Right? That that there are business KPIs generally, roughly aligned to more money, lower cost, or lower risk where there are business KPIs associated to one of those three top high level buckets. Right? That’s the why, and that why should tie to some overall strategy, business strategy, which should tie to your data strategy, which should tie to some of the road map items that obviously you’re gonna be having here, that you’re gonna execute against.
So then we get can get into kinda some of the how. Right? And we can start talking about, okay. Well, so what’s your goal?
We wanna drive new revenue. And and we think that a way to do that would be to maybe monetize some of our data. Maybe we need to implement something that looks like a like a data marketplace.
Maybe, we believe that we can be more efficient as a data and analytics organization, and we can drive down some of our costs or increase our user satisfaction or increase our adoption of our data and analytics solutions, maybe we’re gonna do that through something called the data mesh. And we’ll talk about that a little bit more. It’s rather unique, because a core operating principle of a data mesh are something called data products.
And on and on. Right? You could use something, like, related to we want to share data because we think we’ll drive more efficiencies in in within a very complex ecosystem that we exist in, a supply chain that we exist in. Maybe we need to have data products as part of a broader kind of data and analytics self-service initiative. So these are all some of the tactics that you would use to implement data products.
We will talk a little bit later that that the actual implementation of data product management, I would believe I would, I’m confident could actually help fulfill, some of these some of these goals that we’re talking about here. But but, lastly, once you figured out the kind of the high level why, the high level how, then the very next thing you need to do is to figure out, okay. How am I gonna measure this? Right?
This needs to tie back to those k p those business KPIs that I was talking about. More money, lower cost, less risk. These KPIs that that your chief product off your chief revenue officer, your chief procurement officer, your chief operating officer is going to be measured around. You need to tie this initiative to those KPIs.
So if you’ve got those three things under identified, fantastic. You’re ahead of the game. You’re probably in a pretty good place to start asking questions about, okay. What types of data products?
How are we gonna do this? How are we gonna implement or execute against the strategy of this data marketplace or data sharing or maybe even the data mesh? Right? But you absolutely, positively need to have a why.
Do not be implementing or talking about implementing data products just because everybody else is talking about it. If you don’t have a why, then it shouldn’t be on your priority list.
So then the next question, I think, at least for now and this has become increasingly relevant over the last year, year and a half as the data mesh has risen to prominence. And it is at the top of the hype cycle without a doubt. Everybody’s talking about the data mesh. And if you’re not, you’re probably talking to people who are talking about it.
Every every conference that I’ve been going to, yes. Recently, of course, it’s AI. But topic number two, is is often data mesh or number three, data fabric, which kind of doesn’t closely align, but it it reasonably aligns. And and I’ve got separate podcasts talking about the data fabric, by the way. I will be interviewing Zhamak Daghani, the author of the book, the data mesh, in a in a podcast that will be coming out very, very soon, so look forward to that. But when talking about data products, I think you need to ask, okay. Are you seriously considering a data mesh architecture?
There are a lot of pros and cons for that, separate topic. But the reason why this is this kind of represents a bit of a branch.
If this is a decision tree, the top of the tree is the, hey. I’m thinking about something that could use a data product or or needs a data product.
Then there’s a bit of a branch in the tree here. One of the branches would be, is this are you implementing data products specifically to support a data mesh, or is this something else? Because if it’s a data mesh, what we’re talking about here, folks, is a very specific thing. Data products insofar as the data mesh is concerned. As an overall data management architecture, yes, I know it’s a socio technical approach, people, process, and technology. But we’re talking about a data mesh. A data product within a data mesh is a very specific thing that Zhamak goes to very, very low levels of detail to describe in her book, which you have if you haven’t read, I would absolutely recommend you read it.
There are some very interesting concepts that are shared within that book. It’s a very dense read, so it’s not something that would be kind of, maybe that you could you think you could digest in a single plane ride, unless maybe you were going from New York to Singapore.
He might even need more time than that because, again, it’s a pretty dense read, but I do recommend it at least to know, you know, kinda what’s going on there. But when it comes to a data mesh, data products are very specific.
A few things to think about, from the what makes a data product for a data mesh kind of different than other data products? Well, first and foremost, within the data mesh, it’s analytical only. It’s an analytical product. It’s some sort of dashboard or other insight.
May maybe it’s a schema, maybe it’s a table, but it doesn’t matter. It’s for an analytical use case because the data mesh is for analytical use cases only. Anybody that says otherwise has not read the first paragraph of Zevak’s book. This is about an analytical paradigm here, folks.
Second thing, it’s it’s the the data mesh is all about domain centricity.
Right? A a specific business function or even a subfunction within a specific given like a business function like marketing or sales or finance.
Domain, again, has a specific con connotation within the data mesh, but the domain has control over, has ownership, has management, has authority over these data products.
Highly the the data mesh is a highly, highly decentralized approach here. Right? So so the data products for data mesh are also highly decentralized. You could conceivably have in a data mesh, you could conceivably have the same product replicated four, five, six times over across a a data mesh because, again, it’s highly decentralized, and the domain decides what it wants to do from a product perspective.
In In a data mesh, you would have something called a data product owner, which in the data mesh to me, and I would love to some mock opinion on this, but to me, a data product owner within the data mesh actually fairly closely aligns to a traditional product management role. And we’ll talk about that a little bit more, in a few minutes here. But, at a high level, this is a bit of a combination of a governance and product management role. So that is one area where it would be different is is that a data product owner and a data mesh would be defining governance policies for this product.
Another key attribute here is that it’s it’s it’s kind of self-service provision. So there is this underlying notion of a of a enterprise wide data platform that would be supporting a data mesh, and these these products would be provisioned available through it. I I guess you could kinda call it a marketplace, but it’s it’s not. It’s much more than that in a data mesh. It’s it’s an enterprise wide data management platform that is that is supporting the data mesh.
Governance. This is a big one.
The governance for data products in the data mesh is what Zhamak would call enabled through federated computational governance. This is a this is a complicated way of saying, automated governance.
Right? So here’s where things start to get fairly complicated for a data product in a data mesh.
The the governance would ideally and optimally be automated, where the where the rules of use, where, access controls and and everything else would be defined as a as an attribute of the product.
And those attributes would be enforced through data contracts. This is another bullet on my list. Right? So data contracts is is a key operating tenant of a data product within a data mesh. So those two things together right there, data contracts and and federated computational governance are something that are rather unique in in the mesh world, but they’re also pretty complex, requiring fairly high levels of data governance maturity, also requiring fairly high levels of technical maturity to do this.
Data products are not necessarily for one domain alone. They can be shared, should your your your meet your needs and your requirements for individual domain centric data product also support those of another.
So it doesn’t necessarily mean that it’s only bound to a specific domain, but that’s another area where things can get kinda complicated if you’re sharing these products across across domains. So if you put all these things together, in my opinion in my opinion, data products for a data mesh are pretty complex.
They’re fairly specific. They need to have these attributes, meaning they need to be for analytical use cases. They need to be under domain control, not any sort of centralized control. Domain, defined requirements, domain defined governance, automated governance, provisioned over a data management platform, supported by data contracts.
These things together kind of define what data product would be in a data mesh.
And I’ve had a lot of conversation with data leaders over the last year and a half since the data mesh book came out, and a lot of people are really attracted to this. And and I kinda get it from the perspective of that domain centricity.
You can make a very valid case that doing this is the way to be the most customer centric within an organization. Right? And making and you could make that claim. Right?
Because you’ve got the analytics happening as close as possible to the to the to the creation and consumption of the data within an organization. So that’s a good thing, but they’re complex. And even would would I’ve heard her say this many times. These things are complex.
What that means is that I have yet, in my year and a half of kind of tracking all of this and talking with data leaders, I’ve yet to see anybody implement one of these from top to bottom.
And these meaning a a fully realized kind of, data product for data mesh that has all of these all of these bullets checked. Right? Everything that I’ve got on the screen now or everything that I just mentioned, previously, data contracts, automated governance, domain centricity, analytical use cases. I’ve yet to see anybody actually check all those boxes.
What I do generally see is that organizations say, yes. We are mesh curious, perhaps. Are we looking at a mesh? We we’re we’re slowly phasing in what we believe will eventually be some form of a data mesh. And a phase one of that or maybe even a phase point five of that is we now have data owners.
We now have data product owners within specific domains, but that’s about it. Like, that’s all I’m generally seeing is is that we have given somebody this title of data product owner, and it’s bound by a domain. And that’s where things kinda end typically that I’ve seen in the last year and a half. Now I’m sure there are organizations, that have gone more than that. I’m sure there are organizations that have actually implemented everything that I just described, and I’m sharing with you on screen yet. I just have yet to talk to any of those data leaders, because, again, the levels of maturity, the levels of complexity here are pretty high.
So I just haven’t seen it. But this is this is I’m covering this because it’s it’s important to realize that when a lot of if you’re on LinkedIn, if you’re talking to somebody at a cocktail party at a at a at an industry event or some sort of conference and they’re saying, I’m doing data products, Well, then drill down a little bit. Right? What does that actually mean to you?
First of all, why are you doing it? What do you think the business benefits are gonna be? And you can you measure those business benefits? Okay.
If you can, those things are great. Oh, and then why are you doing it? Is it in support of a data mesh, or is it in support of something else? Because if it’s in support of a data mesh, it’s a very specific thing.
It’s everything that I’ve just described in the on this last slide. Else could be something else. We’ll talk about here very, very shortly. But, again, a bit of a branch in the tree here in data products for data mesh.
It means a very specific thing that I would argue is slightly different than how I would do it as a chief data officer. If I was looking at the implementation rollout of data products, I’d probably be looking at things a little bit differently. And you may ask, well, what would that be, Malcolm? How would you do it differently?
I’m so glad you asked.
Well, for everywhere else outside of the data mesh, when it comes to data products, things are right now are fairly poorly defined in the market. And, honestly, that’s okay, because I see a lot of people just spending a lot of energy trying to kind of reverse engineer what a data product would be.
Right? Like, as we are want to do in the data and analytics space, we often start this stuff from the bottoms up.
It’s our first inclination almost always.
Not not always, but often, I should say, more appropriately. It’s often our inclination because we’re analysts. Right? Because we’re data people, because we’re problem solvers, we like to look at things as their component parts and component pieces. Right? So what we often do in the data and analytics space and this is true, by the way, whether you’re talking about governance or data quality or MDM, and you’ve been given a problem to solve, often what I see data leaders do is they’ll start at the bottom.
Meaning, they’ll start at the the the lowest kind of atomic level, within their ecosystem. They try to understand, okay. What’s the smallest piece of something that could be could be a product? Right?
Like, what’s what’s the lowest atomic atomic level here? Because I tend to look at things hierarchically, and maybe I could find the lowest level then I could group things together, and I could group things and and and and and group other things and branch and and create this ontology of all the things that I could be offering, and that’ll be my data catalog. But I’ll start at the bottom, right, at the very lowest level, and then I’ll find a way to group all these things together. I’ll find a way to define all of that stuff, and then I’ll lo and behold, ultimately, I’ll come up with some sort of catalog that represents all of my skews.
Well, that could be a very, very, very long process.
And, ultimately, I would argue, probably not that beneficial.
I I suspect, yes. As a part of that, you could maybe work on or develop or adapt some sort of an overall kind of framework to define what a product is or isn’t. But, ultimately, I see a lot of those exercises being massive wastes of time.
Because, honestly, if you asked me, and I’ve I’ve led a product organization. I’ve been responsible for hiring, retaining, recruiting product managers and and and and managing that team and and having a p and l and keeping customers happy and keeping our support rates low and on and on. If you ask me what’s a data product, it’s pretty simple, folks. It’s any solution provided by a data team that solves a specific business problem that a stakeholder or user would otherwise be willing to pay for.
It’s that simple. That’s it. No more. No less.
If you’re provisioning something, developing something, creating something, selling something that solves a specific problem that somebody would be otherwise willing to pay for, that’s a product.
Right? So we don’t have to get into these kind of long drawn out arguments. Is it is it a schema? Is it a table?
Is it an attribute? Is it metadata? Is it an API? What is it? Or is it a group of tables?
Is it is it a is you know, like, is it a query? Oh, for heaven’s sakes.
If it solves a problem and somebody would be willing to pay for it, then yeah. But, otherwise, do we need to spend so much energy splitting hairs about what is and what isn’t a data product?
And maybe I’m maybe I’m oversimplifying, but I but I don’t think I am. Because if you take that need driven approach, if you take that kind of that problem centric approach, what problem are you trying to solve?
Dear stakeholder, what is your problem? How can I help you? Right? Well, we wanna increase our revenues, or we wanna increase our customer service or customer satisfaction, our retention, or whatever it is.
And if we had better analytics, we had better insights, if we had some AI, we could do that and on and on and on. And then maybe data products would kind of fit into all of that. And then you’ve got a reason. Then you’ve got a use case, and then you’ve got something you can go build and you can go execute on.
But starting from the bottom up and looking at data or building out a data catalog as a means to understand what Coder couldn’t be in, you know, your list of data products, I I I just don’t see that as as a as a fruitful way to spend your precious time in the data organization. So another thing. I hear often, well, data is a product versus data products. Are these two different things?
Well, no.
Data is a product. Data products, they’re the same thing. I don’t unless you’re talking about the mesh, which I just talked about. Right? So a data product for data mesh is a very specific thing, but data as a product, data products, it’s same thing.
Now I hear many of you perhaps thinking out there as you’re listening to me speak, but, Malcolm, you know, these really aren’t products. They’re actually services. Okay.
Fine.
Again, fine.
We we could have another academic discussion about the difference between a product and a service between something that you own, a tangible asset.
And, yeah, sometimes data products can be that, right, where you can sell data and I own the data. You’re selling me an asset.
Right?
That’s that closer aligns to what what a product is at least from a accounting perspective and a perspective versus a service where you’re delivering something over over time. Right? Where you there is not necessarily an asset that you own. Maybe you’re only buying access to something.
Right? CapEx, OPEX may be another way potentially to look at this. Right? If you own something and you can depreciate it, over time, if you own a capital asset, sometimes that will be a data product. Yes. But often not.
I worked for a company that sold data products. I worked for a long time called for a company called Dun and Bradstreet. They sold products, but what they were selling was access to data. You didn’t actually technically own it. So in that model, yes, it’s a service.
Okay. It’s a service because you don’t own it, and it’s something that you would it’s OPEX, and you would you would, you know, depreciate the cost and not depreciate. You would realize the cost of that if you were buying it over time. Or if you were on the other side, you were selling it, then you would realize the the the revenue over time instead of in one lump shot lump one lump sum.
But, again, product versus service, okay. Now if we wanna spend a lot of time going into how that’s relevant from a product management perspective, we’re starting to get into the weeds. Right? If you’re a CDO and you’re having a lot of discussions about I mean, it’s a rev it’s it’s it’s relevant from, like, from a rev rec perspective.
You’re actually literally selling something to somebody else outside your organization. If you’re literally selling data via some sort of data marketplace, then, yeah, you need to have a conversation about the revenue, what the model looks like, and what what kind of, like what impacts are in from a GL, rev rec perspective. Yes. Of course, these things are are germane.
But if you’re at eighty thousand feet and you’re just talking about data products, you’re thinking about why, you’re trying to understand how this fits into your overall data strategy, don’t get too hung up right now on product versus service because it it’s I I don’t think for somebody who is in an early stages of evaluating if this is something that they should be looking at, if that’s really entirely relevant. It will eventually be relevant, but at at a high level, I don’t think it really is.
So what is this what what is the when thinking about data products, honestly, if you ask me, you can’t do data products without a data product manager.
Like, these things go hand in hand. Because if you ask me, the real benefit here beyond enabling certain capabilities, Right? Like, maybe a a data marketplace or data monetization.
Right? Beyond enabling some of these things that require you to treat data like a product, I think the the greatest benefit here to organizations is the implementation of product management as a discipline within data and analytics organizations.
And I would argue, guys, that this could be beneficial in and of itself, that your why here, getting back to the first slide that I’ve talked about, your north star, why are you talking about this? That the why could actually be enabled simply by implementing product management disciplines into your data and analytics functions.
Right? So let’s take data management. Right? Let’s take kind of classic data management use cases, data quality, MDM, integration, data science use cases. Right?
Can product management as a discipline help make those things better?
Can you, for you know, keep have happier customers? Can you increase your adoption? Can you increase your usage of data? Can you make more data informed decisions within your business units by implementing product management as a discipline? And as a part of that, you would necessarily have data products because it’s just a way of looking at things. It’s a it’s a lexicon, and it’s a lens through which you would apply, a lens in which you would use to improve your processes as a chief data officer. I think the answer here to this question of could product management in and of itself as a way of managing data improve the services or products that you offer, absolutely.
Absolutely.
So I would I would I would even go so far as to say, you know, you don’t necessarily even need to be talking about a data mesh. You don’t need to be talking about data marketplaces, data self-service, data monetization.
Any of these others kind of, you know, initiatives that would involve data products, you don’t even necessarily need to talk about that unless unless you want to, could could align your strategy.
But I would argue that you could be talking about how do I take some of the good stuff of product management as a discipline and implement it into my data management processes. And I and I think that’s what you should really be asking yourself.
Yes. There are other questions about, do we need a data marketplace? Do we need data to sell service? Do we need do we need to sell our data? Do we need to share our data across a complex, you know, value chain, like, across our supply chain? There’s there’s other reasons to be talking about data as a product for sure. But I think the number one reason outside of data mesh that you should be talking about data products is because of product management.
And and what does that actually mean?
Well, data product managers, I’ve I’ve got on the screen now, and and I’ll walk through this for for folks who were just listening.
Data product management and data product managers, I would say, have very specific role within the organization. And I’m starting to lose my voice. I’m gonna have a little sip of water.
Oh, and by the way, if you ever see me with this bottle, this is my favorite water bottle, and it has a piece of tape on it because there’s a vendor name on there. But it’s not my company name, so that’s why I’m hiding it. I actually hate it because I this I this goes back to my days as a Gartner analyst, and we were so concerned about not showing any favoritism that that that I covered a vendor name on my water bottle, but it’s just stayed that way.
Anyway, data product manager. What does that mean? What does the role of a data product manager look like? Well, let’s talk about governance first and foremost.
This starts to get into to to data ownership, which is a pet peeve of mine if you didn’t already know that.
But data product managers would not typically be defining governance policies or implementing or managing governance policies. So data product managers are not data owners, at least insofar as most people define ownership today, the kind of the DAMA definition of data ownership. Data product managers are not data owners, and they’re not data stewards. They’re neither.
Right? The way to look at this is that they’re consumers of data.
Right? You could look at data through a more of a like a supply chain lens. Right? A data owner would be part of the data supply chain.
Right? They would be making sure that the product is of high quality, and it’s consistent, and it’s well well defined, and it’s and it’s accessible, and that there’s policies to ensure PII and all the other stuff that you would need as the manager of a data supply chain. So what is a great way to think about it. Right?
Because product managers take raw ingredients, and in this case, our raw ingredients are is data.
They take those raw ingredients, and then they make stuff out of it. Right? The data product managers are not the ones that are gonna be defining governance policies, although they will most certainly influence them.
K? So a data product manager will have a seat in a governance committee. They’ll have influence over governance policies.
Right? Whatever those policies are that are needed in order to allow data product manager to monetize data, to distribute data, to do whatever she or he needs to do in order to fulfill their mission. They’ll influence the governance policies, but they will not be accountable for it. So So there’s a bit of a church and state thing going on here.
Right? But, again, best metaphor that you can use to think about this is that classic data management and data governance, that’s the supply chain. Right? So data stewards, data owners, others will be creating the data.
The product managers will be productizing the data.
So they are consumers. Product data product managers are optimally consumers of the data from data management process perspective, not the creators.
Now where do they sit? Well, they certainly can be in a CDO organization, and we’re seeing more and more of this. Right? We’re seeing now I think the last time I was at Gartner, we had, in the in the last CDO survey that I was involved in, which I think would have been the sixth annual CDO survey, which is twenty twenty one. I think in that survey, they showed that twenty five percent of CDOs now have p and l, responsibility and accountability. And often that p and l comes in the form of some sort of data products.
So more and more you are seeing data product managers be within a CDO organization where they are deploying data products to support a digital transformation or an ERP transformation or data mesh or other stuff.
Right? Data monetization, perhaps even. Right? Standing up a data marketplace, perhaps. So often, these data product managers are sitting in a CTO organization, but they don’t necessarily have to be.
They can they can be anywhere. They can actually be in a classic product function, right, in in some sort of product organization. They could even they could probably even be within a domain, like, what the mesh would call a domain or business function. They can be anywhere in the organization.
They’re consumers of data that are productizing data.
They would be doing things like defining a product data life cycle. This is this is one of the unique value props that I think product managers can help with often in data and analytics teams.
People who are creating products tend to be fairly transactional kind of and and and kind of more of a service desk approach where it’s like, hey. I need report x, then then they go build report x, and they’re on to the next thing.
Product management takes a different view of that. Product management takes more of a holistic product line centric view and more of a product strategy view. So you could have a completely separate product strategy that would align to a data strategy, of course, but where there is a freestanding strategy that is defined by that product manager and all the things that she or he needs to do in order to fulfill their product related goals. Right?
So that also includes life cycle management. So all the way from product launch to product sunset, and, yes, sunset even. In the data and analytics world, we have a problem with data hoarding. We never shut anything off.
We never turn anything down because we’re we’re petrified that maybe someday might somebody might use that dashboard.
Right? We we or that data may someday one day be actually be used. Well, wouldn’t it be great to have a product manager that had a life cycle defined and they’d say, okay. You know what?
We we’re we’re past maturity on this product. Nobody’s using it. Nobody’s getting a little value out of it. We’re not generating revenue out of this. It’s time to sunset because our scarce resources could be deployed somewhere else.
We need more of this in data and analytics, life cycle management, classic product management. It’s part of what they would do.
Also, kind of value positioning. Right? Value statements.
Just positioning in general. What problem are you trying to solve? This starts to get into storytelling.
Right? So if you see the as a CDO, if you see the value of storytelling, and maybe you would even realize the value of storytelling by telling stories to your CEO or your CFO or your COO, well, that can that can that can cascade down into a product management function, and that’s what product managers are actually really good at, telling stories around data, which is part of a classic marketing function.
So I would also argue that most data and analytics teams need to be better at marketing. You need to be promoting your stuff better. You need to have value stories. You need to have data’s be able to tell stories across the organization of the problems that you’re solving. That would be part of a product management role.
So, yes, CDO should be kind of the chief data storyteller for sure, but she or he should also have some form of data storytellers in the in the form of these product managers because they’re good at it. It’s what they do.
Of course, any product manager would need to be defining product requirements as well, product capabilities.
This starts to get into a a little bit of a mindset shift here because product managers increasingly, not always, but increasingly are looking through the at requirements through the lens of user experience and user design, taking more design centric approaches to defining requirements. Instead of capabilities driven approaches or technology driven approaches, How do you want that user to experience this data?
Not just the data, but the problems that the data would solve. Right? So that’s where we start to get into more design centric approaches. What are the problems that you would use that you will solve with with using this data? So it’s not just about the dashboard.
It’s about how you take the dashboard and actually operationalize it. So these design centric and and user centric ways of looking at product requirements definition and product management is, again, a bit of a unique value prop that I think we absolutely need more of in the data analytics space.
Here’s one that may be sounding a little controversial, but I will I this this is a big one. P and L pricing. Now this gets back to the definition of what is a product. It’s something that’s something that that people will pay for. K?
Well, that means that you should, in theory, if you’re doing product management within a data and analytics function, in theory, you should be pricing your stuff.
Yes. All of it in theory. Now I understand that that would be a massive leap for a lot of organizations right now, but this is something this is where you start to need to walk towards. This is something you you need to be thinking more about. If you could charge for this, what would that be? Maybe even in your organization, you have some sort of charging mechanism or some sort of a chargeback function from IT to the rest of the organization or from the the CD organization to the back of the organization to the rest of the organization. Or maybe it’s based instead of a chargeback, maybe it’s more of an a yearly allocation.
But getting to a place where those chargebacks are rationalized against prices being paid for specific solutions or allocations are a function, a roll up function of what your business partners would be willing to pay for your services.
I know there’s a lot of work here, guys. I know you’re a long way from where where you know, kind of this future state that I’m talking about, but it’s something that we need to be thinking about.
How much is this actually worth? Because what this is, in essence, is doing cost benefit analysis for all of your large initiatives. So maybe maybe you could partner with more of a PMO function if you’re in a larger organization that has that type of function, product man project management organization, when it comes to the allocations, any sort of chargebacks.
But figuring out what organizations would pay for the stuff that you provide, I think, could be just truly and honestly transformational.
Because wouldn’t it be great for you to actually know exactly how much value you drove to the organization instead of guessing? Wouldn’t it be great to quantify it? A great way to quantify that would be through pricing what you sell. Right? So, again, I know there’s a lot of work here, but absolutely, positively something to start thinking about. And I think that a lot of the mechanisms are already there within large organizations. We just need to find a way to exploit them and just be better across the board in terms of, you know, how we actually value and and align what we do, to to providing products to our to our, to our to our stakeholders, to our customers.
So another thing, another kind of unique value prop that that’s another pricing. It’s something that product managers know how to do. They do.
Whether that is from the bottom up, cost up, or from demand down, right, from what people would be willing to pay or from what it costs to make. Either approach. Two sides of the same coin. Product managers are good at that stuff. And if you start hiring product managers to manage these functions, to manage data products, they’ll start asking these questions. Right? And they’ll help you to navigate a path to be closer to this world of being able to actually charge.
I’m air quoting charge because I I doubt there will ever be a situation where it’s like a vending machine and you put your money in and then you get your your analytics out.
But to have mechanisms to charge and to value. Not just charge, but to value what you do for the organization.
Another unique value prop here is the second to last bullet on the slide, which is, you know, owning kind of product launch.
This one is so huge, guys.
What a product manager would call go to market.
Right? If you have done a robust job of user centric design, if you understand your customer’s needs intimately, if you understand how they’re using the data and the analytics or the insights or the or the the the AI models or whatever it is, if you know how they’re using those to drive value within the organization and you’ve done your best to define all those requirements and make them highly user centric and make them very user friendly, very easy to use, all those things that product managers do, kind of innately. If you’ve done that, then you need to launch your product, and you need to support your users.
Right? And they do need to be trained in how to use those products. Of course, we all need to be trained on how to use products.
But I would argue that if you’ve nailed it from a product management perspective, if you have nailed it from a requirements perspective, you’ve nailed it from a design perspective, you’ve nailed it from the perspective of understanding how your customers leverage your solutions day in and day out, that training component will be a fraction.
Tomorrow, that training component component will be a fraction of what it is today.
Fraction. It may not even be required because if you do this the right way and you and your products are so intuitive and so easy to use and so so well designed, maybe they’re ease they they can be used right out of the box. You don’t even have to do anything. People just get it.
They understand it. They understand how to use things. Now I I I know that’s probably you know, we’re a long way from that for a lot of organizations, but my whole point here is is that instead of assuming that your users will lack skill and and lack the ability to drive value from data because they lack skill, turn that around. And by the way, that is the underlying premise behind any data literacy program, which I fundamentally disagree with.
I don’t think our users lack skills. I think we’re poor product management.
I’ll say that again. I don’t think our users lack skills. I think we are generally poor at product management. If we do a better job at product management, the need for what people keep calling data literacy, which I I I that phrase, I vehemently disagree with.
But I because I disagree with with the premise that that that’s that our users are are incapable of driving value from from our solutions. I just think that our solutions are are are not sufficient. They’re not well designed. They’re not intuitive. They’re not easy enough. That’s where we need to be focused, and that’s the biggest benefit I think that product management can bring to a data and analytics function.
That’s a separate topic altogether, data literacy. I I’ll probably record another kind of solo episode here because I because I think that I think data literacy is is toxic.
Now that doesn’t mean that I think training people is a dumb idea. Of course, it’s not. It’s a great idea. You need to. And you need to people need to know how to to use the tools that you’re providing. But I think the notion of data literacy is toxic because there’s an underlying premise there that assumes that your users lack skills.
And and and maybe they do, but the story that I tell over and over and over, guys, is that if I was making a product, a widget let’s say I was making this phone, and there’s a phone in my hand. I’m waving it around if you’re listening to just listening to me. Let’s assume I’m a product manager for a cell phone for an iPhone. Wouldn’t that be a fun job, product manager of an iPhone?
And And nobody used it. Everybody hated it. The user reviews were horrible. Right? The return rates were high. Everybody was sending it back, or maybe even somebody went so far as to go buy another phone from another provider, even when yours was free.
You’re building a phone and you’re making it for internal consumption. If you’re building a product and it’s largely free, I don’t have to pay for it, but I’m still willing to go buy it from somebody else and actually pay for it. Right? Like, that’s that’s these are massive product management red flags.
Like like, if I was that product manager of of the phone that everybody was returning and complaining about online and everybody hated and said it was difficult to use, and they said, I don’t wanna use the phone because they don’t understand it. Right? If I was the product manager for that, I’d be like, man, I blew it.
Like, I blew it. I I missed some requirements. My design sucked.
I really didn’t understand how are they they were using the product. I didn’t I didn’t know I didn’t understand the the the value they were gonna get from it. I was way too complex.
Any supporting documentation was weak. We didn’t do any sort of broader go to market. There was no communication plan. Like, all those things, I’d say, man, I missed it as a product manager. If that was happening for me, I’d say I I missed it. I missed the mark. I blew it.
But in the data and analytics world, we say, oh, well, nobody wants our products. Nobody can use them.
Everybody’s complaining.
It must be it must be them.
Man, I that’s a head scratcher to me. Like, don’t get me wrong. You need trading, and you you need post launch support. You need FAQs.
You need all of this stuff. I I I get it. But, like, having that underlying assumption, when there are so many things that can go wrong in a product launch, when we know as data leaders, we’re just not that good at product management. We know we’re not, but I because I can tell you we’re not because I was chief product officer, and I I just know we’re these are things that we don’t really kinda focus on.
When we realize that we’re just not that good at it, yet we still say there must be something missing in the skills of our users, I mean, that’s that is that is just a short circuit. Right? And I and I think we can do better than that. So I think that’s all the content that I wanted to share. That’s it. I’ll stop sharing my screen so you can go back to my giant mug.
At eye level, I I am the most excited about product management as as a tool, right, as a vehicle to improve the way chief data officers manage a data and analytics function.
Right? Because I because I think there are transformational benefits there that would go beyond more tactical approaches for data products, like data monetization or data sharing or some of the other examples that I gave. And there may be some pieces of your road map that that absolutely require you to treat data more like a product and assign a skew to it and assign a price to it and maybe even distribute it to external users. There are certainly use cases there that that would require product product kind of approaches.
But I think the more transformational transformational thing here is to act like product managers, right, to implement product management as a discipline into your organization because I can see only goodness when you do that. Product managers know how to understand customer needs. They know how to define requirements. They know how to interact with engineers.
They know how to be agile.
They know how to build roadmaps. They know how to build strategies. So a lot of the things that I think we need in the data and analytics function, product managers can bring to it. So that’s today’s episode of CDO matters.
I’m bullish on product management in case you couldn’t already tell. I think you should take a harder look. If you were if there’s something about data products and the conversations that you’re having that is attractive to you, ask yourself why. What problems are you trying to solve? And I’d be willing to bet that many of those product problems could be solved by applying more product management as a discipline into your data and analytics function. So with that, I will let you go. Thank you so much for tuning into this episode.
Look for another episode here very, very shortly. I think this one is our twenty ninth. Goodness gracious. Wow.
Look for episodes shortly coming from, the dean of big data, Bill Schmarzo. He’s gonna be talking to us. If you don’t know Bill, you should. One of the smartest humans, I’ve ever interacted with. We’re gonna talk about ethical AI.
That should be interesting. I already mentioned that we’ll be talking to, Zhamak Dehghani about the data mesh. We’re gonna be talking to Alison Sawgraves about some of the problems that we’re seeing day in and day out, in in managing data and analytics functions. So there’s so much more to come from a c from the perspective of CDO matters.
I’m excited about, how far we’ve come from the podcast. Please consider subscribing. Again, I would be honored if you did. And with that, I’ll let everybody go.
Thank you for tuning in. Thank you for listening, and we will see you on another episode of CDO Matters sometime very soon. Bye
ABOUT THE SHOW
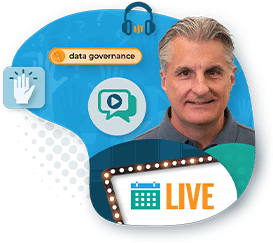
Malcolm Hawker
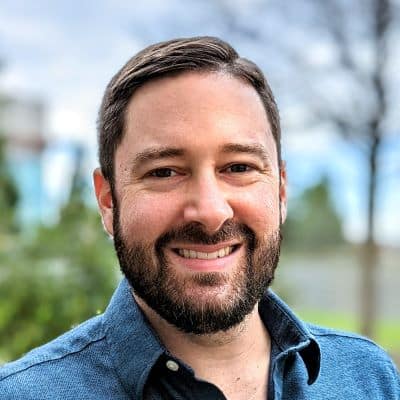
Benjamin Bourgeois
Ben Bourgeois is the Head of Product and Customer Marketing at Profisee, where he leads the strategy for market positioning, messaging and go-to-market execution. He oversees a team of senior product marketing leaders responsible for competitive intelligence, analyst relations, sales enablement and product launches. He has experience managing teams across the B2B SaaS, healthcare, global energy and manufacturing industries.