Episode Overview:
The truth isn’t always black and white. Sometimes, it requires more context and background when attributed to different scenarios and situations.
The same can be said about your data and whether a “single version of the truth” can be properly applied to multiple use cases for your business.
In this episode, Malcolm interviews Jeff Jonas, the Founder, and CEO of Senzing — a software company on the leading edge of developing “entity resolution” solutions — which solve the growing challenge of uniquely identifying people or objects across multiple systems of duplicate, low-quality data. Malcolm and Jeff discuss how advances in technology are fueling more modern forms of entity resolution, where companies are now able to implement more context-centric approaches to complex matching, particularly within their master data management (MDM) programs.
As technical as “entity resolution” may sound, the two uncover the global effect this technology has on people each day — including the job of the Chief Data Officer (CDO). Also known as “disambiguation” or “fuzzy matching,” effective entity resolution allows software systems or data stewards to decipher whether records for Richard Smith and Dick Smith may represent the same person, even when it is not overtly suggested by the data.
Jeff describes how entity resolution sits as a foundational component of data within MDM, customer relationship management (CRM), know your customer (KYC), supply chain and every other major business process that relies on accurate, trustworthy data.
Jeff correctly notes that aside from horrible customer experiences that may arise from a lack of effective entity resolution, “it creates a lot of waste for companies to think you are two or three people instead of one.” Citing a person’s example of having their name represented three distinct times in a hotel loyalty club database, he emphasizes the toxicity that comes with a lack of focus on entity resolution for companies who are trying to be both customer and data-centric.
While many companies — particularly those already investing in AI/ML — may be attracted to implementing DIY solutions for entity resolution, Jeff notes that it’s “super expensive to build”, especially given the complexity and diversity of data, and even language itself. The ability to understand meaning across objects, cultures, languages and even alphabets is at the core of reliable entity resolution and building bespoke solutions for tackling these complex problems — at scale — is beyond the capabilities or budgets of an overwhelming number of companies.
When considering a “single version of the truth”, Malcolm unpacks the 30-year history of large, monolithic enterprise resource planning (ERP) suites that created the mindset of master data only living in a singular place within the organization. Thanks to the democratization of IT, the “single version” mindset is shifting both as a practicality and as a business need. Today, master data can be sourced from a single location while supporting multiple versions of truth based on the use case of that data.
In talking about the evolution of large-scale entity resolution, its use in MDM to enable multiple versions of the truth and the legacy requirement to have data stewards manually review records, Jeff notes, “There are definitely times…when you want a human to take a look and make an adjudication. But, I will tell you, in large-scale systems, you don’t have enough humans.”
Rather than adding more people into data stewardship roles to support higher confidence matching, Jeff advocates the approach of widening the pool of data used by entity resolution processes — beyond just name and address — to make match decisions, including the possible use of third-party data sources.
The last few minutes of the conversation go deep into AI/ML, and how these new technologies are used to augment human data stewardship processes. Jeff makes a great case to suggest that most stewardship tasks could be mostly automated, but that many companies are unable to duplicate pure human judgment.
Throughout the conversation, Jeff and Malcolm take extremely complex technical issues and make them digestible and relatable to CDOs — consistently refocusing on how using entity resolution is critical to establish truth in an organization, especially when using MDM systems to manage said truth. CDOs who want to have more informed conversations with their technical staff about the role of entity resolution going beyond just “fuzzy matching” will find this episode of CDO Matters highly insightful.
Key Moments
- [3:42] Entity Resolution Defined
- [7:50] The Impact of Poor Data Quality
- [11:23] The Death of the ‘Single Version of the Truth’
- [15:45] Entity Resolution Failures
- [24:03] Understanding Unique Entities
- [26:13] The Cost of Being Wrong
- [29:10] Human Intervention vs. Trust in the Algorithm
- [32:05] Gaining New Insights with MDM
- [35:09] Valuing Human Judgment
- [40:03] The Future of Entity Resolution in Digital Transformation
Key Takeaways
What Is Entity Resolution? (1:58)
“Entity resolution is recognizing when two things are the same…it’s [also] called ‘fuzzy record matching’, ‘link detection’, ‘disambiguation’, ‘match/merge’ and lots of names. It’s been congealing into this term ‘entity resolution’ and is being more used. And really, the definition that I would have for it is recognizing when two identities are the same despite being described as different.” — Jeff Jonas
The Cost and Complexity of Efficient Entity Resolution (5:15)
“This problem [with poor entity resolution] is ubiquitous, and it turns out, is super expensive to build. People think you can hire a little team and do some AI/ML and think you are going to match well. And I am telling you, you cannot create something competitive in five years for twenty million.” — Jeff Jonas
Is the ‘Single Version of the Truth’ Dead in 2023? (13:07)
“There are still many people saying you need a single version of the truth…At an operational level [within a company], there are multiple versions of the truth. The way a marketer would define a customer is different than the way somebody in finance may define a customer, particularly B2B, where one would be a ‘sell to’ and the other is a ‘bill to,’ and they are both correct. A lot of people still think that is what MDM is, and it can be that if you want it to be that, but it doesn’t have to be.” — Malcolm Hawker
Working with Multiple Versions of the Truth (14:12)
“I think the ‘single version of the truth’…those are the dark ages, the dark days. The truth is you really want systems that can present truth to the eye of the beholder. It’s about who the recipient is. But there are two forms of truth: One is about separating ‘who is who?’ from which attribute is the best attribute…and how many entities does the organization have? Do you really want marketing to have a different [data] account than finance?” — Jeff Jonas
Human vs. Software: The Role of Human Judgment (29:38)
“There are definitely times/cases in data when you want a human to take a look and make an adjudication. But I will tell you, in large-scale systems, you don’t have enough humans. Second, I will tell you, ‘How does the human do it?’ The human is using additional data. It’s either data stuck in their head or they’re searching it up somewhere…but a lot of times, you have to actually do research…so making these decisions on records with some human intervention is about adding data. And one of the things that we propose is that there are kinds of data that is the initial data needed.” — Jeff Jonas
About the Guest
Jeff Jonas is not only the CEO and Founder of Senzing but also the Chief Scientist. Since 2016, the organization has provided fast and easy API for accurate data matching. For more than 30 years in the field, he has been at the forefront of solving big data problems for both companies and governments. National Geographic recognized Jeff for his talents in the data space, referring to him as the “Wizard of Big Data.”
Episode Links & Resources:
Malcolm Hawker
Hi, I’m Malcolm Hawker, and this is the CDO Matters podcast. The show where I dig deep into the strategic insights, best practices and practical recommendations that modern data leaders need to help their organizations become truly data-driven. TuneIn for thought provoking discussions with data. IT and business leaders to learn about the CDO matters that are top of mind for today’s chief data officers. Good morning, afternoon or evening, everybody. I am Malcolm Hawker. I’m your host of the CDO Matters Podcast. I’m honored today to be joined by Mr. Jeff Jonas, who is the CEO and founder of sensing. We’re going to learn a little bit more in a minute here about what sensing does for a living, but I’m excited to talk to Jeff. I’ve known. Jeff 2-3 years ish somewhere in in there. Through my travels in, you know, obviously a gardener and through kind of the data handlers community at large and through another community, we’re going to talk about this this this this subgroup of of folks like me and Jeff, who are fascinated with this thing called entity resolution. We’re going to talk about that a little bit more and how it relates to what CDO? It do day in and day. Out, but I’ll leave it at that, just why? Don’t you introduce yourself instead of me doing all the kind of the flowering intros and and tell us about? Yourself and about your company.
Jeff Jonas
So you know, as you said, I’m the founder and CEO sensing we’re a spin out from IBM. IBM bought a company that I built called SRD. They bought my company in 2005 and that was my fifth generation entity resolution and I built 5 engines over the years and that Fifth Engine was a goodie. And IBM has a product in the market today called Identity Insights. Great product. And while at IBM I said, you know, for $50 million, roughly plus or minus, if I start from scratch. I could build a radically different entity resolution engine. And and so we did. We got commissioned to do that. They’re hard to build these things. Or maybe we should decode what this word entity resolution. Let let.
Malcolm Hawker
Let’s let’s do that and and and. Then tell us why it is. But I would yeah, I’d love to hear from the expert I could I could find. All day on. Any resolution, but I’d love to hear your perspective.
Speaker 3
Well, first of all.
Jeff Jonas
Comes by a lot of names like everybody this affects everybody’s life. Everybody experiences it. When you get duplicates in the mail, that’s an entity resolution problem. When you have duplicates in your address book, that’s not. You’re the only curator.
Speaker 3
Putting things in.
Jeff Jonas
Your own address book. And now you’ve got two or three copies. Imagine if you’re a bank and you have like thousands of people that enter things. Imagine how many. Duplicates they have. So entity resolution is. Recognizing when two things are the same and in in healthcare might be called patient record matching. The first version I wrote for TransUnion credit bureaus was called debtor match. So you can make one phone call for three does not call somebody three times. It’s called fuzzy record matching link detection, disambiguation, match merge, lots of. Things, but I mean. Like it’s been congealing into this term entity resolution. Maybe it’s being more used and really. The definition that I would have for it is recognizing when two identities you could call it a person or a company or a plane are the same despite being described different. One will be Jeffrey Jonas. One will be Jeff. Jonas will be PO B versus PO B without the, you know with. Right, but as well, recognizing that 2 records are different even though they’re so similar like you have two records that are exactly the same, But one’s Jr. And Sr. yeah. And then we’ll build. A graph, while we do it too, it turns. Out you having been in this my. Whole life if you if you don’t remember who’s. Related to who and adjacencies? You can’t match as well. So that’s what entity resolution is and it’s in it sits behind master data management. See arms, KYC’s, AML’s vendor, supply chain, patient systems.
Malcolm Hawker
Right, I mean. It’s it’s. It’s one of those things where. It could touch. Everybody, right like? We’ve all had horrible customer experiences right where we check into a hotel that we stayed in before and they don’t know who you are right and and they treat they treat you like a net new customer or or conversely. And you get that piece of snail. You know that is directed to you, and it’s very clearly not for you. I used.
Jeff Jonas
To get a lot.
Malcolm Hawker
Of emails or snail mail coming to me in Spanish? For some reason I I don’t know. Why but somewhere? Somehow somebody thought that. I was a native Spanish speaker that actually happened. Recently somebody came to my door only speaking Spanish and they were they. Were looking to. You know, explain the benefits of of becoming a Jehovah’s Witness and and they had sent their Spanish speaker to my door because they thought that I was a Spanish speaker. For whatever reason, those. Are classic examples of yeah. Right, not doing what you just talked about well.
Jeff Jonas
It creates a lot of waste for companies to. Think you are? Two or three people instead of 1 by. The way I’m able I. I go to check in. This isn’t so long ago into one of these major hotel chains. They go. I’ll give a little card they go. It’s not. Already on there, my travel people didn’t put it on. Oh, they’ll look. It up they go. Which one are you they? Name 3 they’re all me. If I’m in. Their loyalty club three times. I’m like, hey, can you just put those all together? It’s all me that, oh, no, we you might go earlier, remember?
Speaker 3
And I’m like well.
Jeff Jonas
OK, which one has the most points I? Want to be? That Jeff Jonas, but this problem. Is ubiquitous, and it turns out it’s super expensive to build like people think.
Malcolm Hawker
Right?
Jeff Jonas
You can hire a little team and do some AI ML and think you’re going to match well and. I’m telling you, man, you you cannot create something competitive in five years. For 20 million, like literally, right? Well I.
Speaker
Used to have this.
Malcolm Hawker
Conversation to Gartner all the time and related to kind of build, make or buy around NDM when I would talk to didn’t matter, I mean like it could just any any very. Very large company that has. Robust development, presence and they’ve got engineers and they’ve got architects and they’ve got really, really smart people. And and I had a number of conversations with Gardner where people were like, OK, we can just build this thing right this this MDM thing or data quality tool didn’t matter, right? Like we can. Just build this. And I said, well, you know. Dragons here. Because companies been. Trying to figure this out for decades and. There are, there’s. Major major major investments that you need to make and why would you remake the wheel? American companies like Sensing and other. That do this for a living in. Heaven for a long time.
Jeff Jonas
A quote that. I heard from a friend that is an exec technical exec at Deloitte Center for any resolution excellence. He says, you know why it’s? So hard, I’m like why it’s so hard to. Explain to people because because there’s 1000 edge cases. As soon as you talk about doing people data company data, then different geographies, then different cultures and different languages and then this one’s got these features and that one’s got those features. It just piles on, just becomes very, very complicated right away that gets down to my purpose you. Know all my. Company’s purpose is to just make this make. World class any resolution available to everybody. Right, so we have a developer tool. People plug it in. It’s like why would you write your own spell check and grammar check or imagine going to a company. Go hey, what’s this team? Do they go? Oh, they’re working on sentence. Parsing, building spelling, grammar checking, you’re like.
Speaker 3
Right, right, but you still gonna.
Jeff Jonas
Library and so that’s what we are. It’s you can do any resolution in this Q3 API calls. And developers just compile us into the Java, Python or C. It all runs. Local lives in their stack. You don’t have to. Inherit our stack. It’s a link library, you know. It a dynamic link library or shared. Good old fashioned.
Malcolm Hawker
Lol yeah. It’s it’s interesting. I was at a conference this week. The data governance and Information Quality Conference in Washington DC and I was able to hook up with an old friend. I’ve lived in DC for a decade prior to to to living where I live. I hope that with an old friend who works for the federal government, I won’t say which branch, but a branch that would be very, very concerned about people and the movement of people globally. And this is a huge issue. And so it’s not. Just a matter. Of you know, convenience and does the hotel give you foam or feather pillows? But in the case of national governments? We’re talking about national security here. For example.
Jeff Jonas
Yeah, what’s that? I missed that again.
Malcolm Hawker
Oh well, in the case of airlines. Right like yeah.
Speaker
Yeah, soon as you.
Speaker 3
Have a a list of people that you.
Jeff Jonas
Maybe wouldn’t want to let into the US. By the way, that Boston shoe bomber was on the.
Speaker 3
List, but when when that person? Came to the US.
Jeff Jonas
They had one extra consonant in their last name, and so the matching software missed it. And they let the Boston shoe bomber in when they which they already knew not to let them in. I know I worked with this bank in Asia and they they fired a money launderer you know it’s like, hey, you’re no good your money’s no good here. Your money launderer the same person shows up in I1 letter different their last name and a different passport. And they thought like yay, we have a great customer. It’s just a a A. Wolf in sheep. ‘s clothing you know just sneaking back in.
Speaker 3
By the way, it turns out an.
Jeff Jonas
Interview resolution if you’re trying to catch clever bad people. Clever bad people. Don’t use the same name and address. In every record, you can’t even do record matching because they’re. Their purpose is to deny you they’re trying to separate their channels. They don’t want you to figure out this. Person and that person the same, so they’re using.
Speaker 3
Their middle name.
Jeff Jonas
They transpose the month and day and the date of birth. They use the post office box set of the address. And so you. You can’t even use record matching. You know record matching is a record you’re. Trying to find another. Record and what we spent years figuring how to do at scale. In real time is entity centric learning. What that means is as as two or three records become known to be the same person, maybe you have an address on this record and on that record get a middle name and on this one you had a date of birth that they used. And by the way, this gets to like why there’s no such thing as a single version of truth. Is they used? Date of birth they’ve never used before, but that’s. Their brothers and they just. It’s easy to remember, you know, right? And so, now and any sense of learning as a record comes in and you’re matching it to this Union. And if you’re trying to catch clever bad people, that’s essential. And then it turns out if you’re just trying to do, you know marketing and householding and eccentric learning makes. Makes magic.
Malcolm Hawker
So so. Just to kind of wrap. On the. Kind of the idea of entity resolution. I, I think a lot of. CEO’s could say, well that’s really kind of not relevant to me, or that sounds like an. Edge case, but I would. I would argue that that that no one who your. Customers or your suppliers or your vendors or your assets, or your employees or your locations. Doesn’t matter is it’s kind. Of the kernel with the K.
Speaker 3
It’s it’s sitting. It’s sitting at the.
Malcolm Hawker
Beating heart of your data and if you don’t have control over that process, and if you can’t tell the difference between Jeff. Jeffrey, everything else you tried to do. Whether that’s analytics, whether that is any. Sort of operational task will be will be compromised. So that’s the importance of entity resolution now.
Speaker 3
And can I add though in machine?
Jeff Jonas
Learning if you use machine. Learning to find fraudulent behavior and you’re training it on a system that has 4% or 8% duplicates.
Speaker
Like how how?
Jeff Jonas
Good those models. Going to be right, yeah right yeah I’m.
Malcolm Hawker
Totally, totally agree. Whether it’s machine learning, whether yeah, absolutely again, kind of the the the beating heart of of your data as it. You had mentioned a few. Minutes ago this this idea of a, you know, the death of the. Single version of the truth and and and let’s. Kind of wind back the clock just. Just a little bit here. And I would say. Maybe 20 years ago, ISH when the world was still largely dominated by large ERP. Jacks, the siebels of the world and that ilk. Where ERP and CRM kind of game? Prominence this idea of a single. Version of the truth kind of came along with that. I would say it was kind of tagged on and appended into kind of the proliferation of ERP and then subsequently the proliferation of of CRM. But where organizations believed that they needed to have a single customer record 11 to rule them all and it was managed in one single place. And it had very, very tight controls over it and it had tight governance and only certain people could update it, right? And this is kind of the old legacy model of ERP’s where there was a single customer table and a single supplier table, and that one table said everything. It said procurement. It said manufacturing fed. Logistics, it said. Rev Rec you name it. That one table was was again kind of the beating heart of an ERP stack and and that worked for a while and that worked for a while and then along comes CRM and then and and thank you Mark Benioff and and now you can go and deploy a CRM system anywhere and put it on your credit card and sales and marketing. People can manage their own. Customer records, and lo and behold. You start to have a. Drift between what’s in an ERP and. What’s in a CRM? Where that now? Is has been magnified a hundredfold, thanks to the democratization of IT. You’ve got processes. We can just pick on customer because that’s an. Easy one, you’ve got positive customer data. All over the place. It’s and there are still many people that are saying, well, single version of the truth. You need a single version of of the truth, when in reality at an operational level at an operational level there are multiple versions of the truth, the way that the A marketer would define a customer is different than the way somebody in finance may define a customer particularly. B2B let’s so B2C, but particularly B2B or one would be a sell to and the other. Is a bill to. And they’re both. Correct, and they’re both and they’re both correct so. What we’ve been seeing within the last few years is. And I think. Challenging the notion of this idea of 1 version of the truth to to to rule them all. But a lot of people still think that’s what MDM is and it can be that if you want it to be that it doesn’t have to be that. But I love your your. Thoughts Jeff. I just kind of gave what I. Thought was like the evolution of how we got. To this this place where a lot of people. I think that there needs to be one to rule them all and. And when you hear me say that, what do you think?
Jeff Jonas
First of all, I’m thinking it’s really fun to geek out with people that know the. Business, you know that’s my first.
Speaker 3
Yeah, this is fun. You know there’s a there’s.
Jeff Jonas
Two, I think of of the. Single version of Truth is well. Those are the dark ages. OK, the dark dark days.
Speaker 3
The truth. Is you really want?
Jeff Jonas
Systems where you can present truth to. The eye of the beholder. It’s about who’s the recipient, but there’s two forms of truth. One is who’s who? There’s a I want to separate from moment the truth about who’s who from which attributes. The best attribute which date of birth, which address is the best address? What’s the best name and you know address film and whatnot? When communicating right to create the golden record. One is a lot of some a number of systems are have loss E processes, so when they make a decision to bring things together they keep the longest name you know they got Richard and ****. They throw **** out. They keep Richard or they keep Elizabeth over Beth. Bad news there is. If you ever change your mind because they’re sisters or it’s a junior senior, you can’t take them apart. So that’s one. Reason to keep every variation of truth. I’ll, I’ll stay on the attribute side for a minute, then maybe at the end. If you remind me, we’ll go back to the how many entities does the organization have, and do you really want marketing to have a different count than finance when you’re trying to? Answer to the street. OK, because because I think there’s. A there’s a a better way. To do that, but when? It comes to these features. I want to go back to this story where I. I used to try to go. Get it I tried. Once it was my biggest customer ever at the time and. I was going. To to define single version of truth and we. Were going to pick. Which address was going to be? The address for the enterprise so. I’m going to tell you. The story OK because.
Speaker 3
It really it was a face plant.
Jeff Jonas
OK, just hold on. This is my generation 3 entity resolution. The company doesn’t exist now. As a parent company, it’s called Cendant. It’s the NDA. NT doesn’t exist now.
Malcolm Hawker
Yep, remember it.
Jeff Jonas
But they owned 23% of all the hotels Ramada Howard Johnson Super Super 8 Days Inn, Travel, Travelodge Wingate. They owned Avis and Budget Rent-a-car they owned ERA Century 21 and Coldwell Banker and Jackson Hewitt. They owned a lot of and they wanted to take a billion records. No common key and he resolved to figure out.
Speaker 3
Who’s who to get?
Jeff Jonas
A A 360 profile and they use it.
Speaker 3
For market, the.
Jeff Jonas
Goal was to predict who was going to go to Orlando the second week of May.
Malcolm Hawker
And if you really believe they.
Jeff Jonas
Were going to go is this the? Kind of person, easier to influence three days before or three weeks before. So I’m built. This is before the word big data. By the way. OK, right? This was like. Billion record, 100 million person entity resolution system. I go to this meeting with. The heads of marketing. And I March in and I go look, we’re. Here today. Because we’re going to choose. What’s the best address? Let me tell you. What happens next? One of the hotels goes well if our customer told us to use this address on the loyalty club statement. I don’t care. What else this organization has? Maybe they’re having an affair with somebody. It’s not on me to change that address.
Speaker 3
And I’m like ohh.
Jeff Jonas
That was like the clue you know, and the other one was like if somebody just gave me a hotel reservation address as a chip. You know over the phone where an operator. Checked it in or online and it’s super messy. I’d rather have an address off of a license or a loyalty club, so I’ll take your address and. You can tell, right? There the definition of the best feature was particular to the recipient. And that’s where I really. We had to rethink this single version of truth and it led us to something called full attribution systems where we keep every variation of every feature that we get from every record source. And rules of visibility, which happens after us. It’s actually. Can be trivial. Think the longest thing like the longest name. Take the most recent address or you can make it more sophisticated, but rules of visibility is like a down downstream thing, because what we now present is like entity ID. 57 has 16 things in it. There’s four addresses, three days of birth. Now just one more thing while I’m on. A roll.
Speaker 3
My son has.
Jeff Jonas
Count them two dates of birth. It’s a bad daddy story and it’s very embarrassing. My son was born on September 2nd. OK.
Malcolm Hawker
4th go virgos.
Jeff Jonas
Well I. Hey, that’s awesome moment he was older than you and for a moment he was younger. Than you because we’re on September 2nd.
Speaker
Speaker 3
For some reason, I thought he was born on September 5th and I convinced mom and the grandparents. And we celebrated his birthday on the 5th for years.
Jeff Jonas
We used the 5th for every doctor’s visit. Every you know sign up for Ricky Rick News, you know, whatever.
Speaker 3
Imagine that date of birth being.
Jeff Jonas
In 50 or 100 databases, September 5th, I get ready to take him to Mexico I order. His birth certificate, and to my surprise.
Speaker 3
It’s September 2nd.
Malcolm Hawker
How about that?
Jeff Jonas
And you got to tell your kid. You you got your birthday.
Speaker 3
But I want you to imagine.
Jeff Jonas
This from a single version of true. Imagine having evidence of one date.
Speaker 3
Of birth over and over and over, and.
Jeff Jonas
Over and over for years. September 5th and now suddenly September. Second, shows.
Speaker
Up, yeah.
Jeff Jonas
Really upon you to say, well, because I have so much evidence, I’m going to suppress it. I’m going to snuff that little piece out that bad data. If you do that, you can’t let. Of diversity, you have to let diversity.
Speaker 3
Faster and you can’t. If the the the the the dissenting. Voice would never add up you. Would never get to where the.
Jeff Jonas
New date of birth is more predominant.
Speaker 3
If you couldn’t keep.
Jeff Jonas
The old so you have to have systems that support multiple dates of birth. If you want to do this well anyway, right?
Malcolm Hawker
Yeah, I. I love the story, so I’ve I’ve got a virgin I’ve got. I’ve got a version so this was about ohh maybe even 15 years ago, maybe even more. So 20 years ago, I think that’s accurate, bright eyed, bushy tail. I’m I’m. I’m running this MDM program in an IT shop and we’re we’ve been tasked to come up with the single version of the truth and I had the meeting after meeting after meeting after meeting with marketing and finance and we bring them all in together in a room and we would have the you know, the the the kind of the. The working session. The the. To to figure out you know who wins, right? And and and we couldn’t get them to agree, so that that’s that. I mean that that’s standard stuff. We’re right, you’re right, you’re right, you’re right. You’re right and and. I was just finally. Given an edict by our by our CTO. ‘s like just go. Clear out hey it just go figure it. Out, it’s like OK. So we we. The the the the technical people sat down. And we figured out, OK. We we’re smart, we we can do this and what we did is we were looking at need to be customers and we started to build all of these rules. These cascading rules all these themes but last update day whether it was null or not null. And all of these fancy rules and. What what was? It structured correct? And we looked across all these sources of data, and this was a multi week. It’s not a month long plus exercise because we actually made multiple iterations of it as well. So we put. The rules into an MDM. It was an. IBM, by the way. This thing. Called initiate, Oh yeah, you probably.
Speaker 3
Remember, I was in IBM and to encourage.
Jeff Jonas
Them to buy that. The company.
Speaker 3
Yeah, and and so we and.
Malcolm Hawker
We do the rules and we run multiple iterations multiple. Iterations Yep. Yep, Yep, Yep. So to figure out and make sure. What came out of the other end of the sausage grinder actually fully reflected the the business. Rules that we had. We had defined up front. We thought we were the smartest people on the. Planet we start to. Engage some of the end users and kind of unit. Testing of. Stuff and and what they. Said is what the hell is this? I only I don’t. Even recognize this this this. Makes no sense. This record can’t even. Exist in real space and time? Because what you’ve done here is, is we. We have patched together a customer. Record at an attribute level. Where we were kind of plucking this this address this address. This address we had prioritized sources right we said oh we don’t trust our sales people so we’ll prioritize Salesforce lower. We’ll prioritize the ERP higher because you know there’s more rigor that goes into that that data. So that’s going to probably be better. Quality data and on and. On and on and. We created something called Franken record. That actually exist in real time in space, because there were these buildings and ships that were completely different. We had a we had a shipped to addresses for for companies that weren’t even reflective of and Acme Incorporated address, we’d say Acme Incorporated and then we have this address and the address is for some sort of. Warehouse or a? Loading Bay where Acme was doing business. But it didn’t actually conduct business there. And that was months of chasing our. Tail on that by by figuring out by thinking that we could could, could could congeal these multiple versions of the truth into something that look truthful and ended up being a disaster.
Jeff Jonas
Those were the dark Days man this. Whole thing, I think that there’s. Only there can be one of something when there’s a plurality of things and to have to then choose one is. You you might need to choose one. At the moment you go to mail something to somebody, but ask that team what is their precedence and and somebody else has to pick something when you report something to the IRS with a with a tax ID, you’re going to have to. Pick one if there’s two not getting. Somebody to find out or validate it.
Malcolm Hawker
Yeah, so that’s the good news. The good. News is is we have evolved to a place now where as data leaders we can say everybody wins. At least at an operating level, at least at an operating level, I would argue as. You go from. Kind of functional to cross functional to enterprise wide. Those these those differences need to be. Resolved somehow some. Way because if you’re CEO, asked if. You ask your team how many customers do. We have this. There can only be one answer at your level. Of the organization.
Jeff Jonas
Well, that’s I was going to say to me there’s two different things about single versions of truth. One is which attributes are the winners? You know which name and address are you going to pick if you? Only pick one that. You have a list, but the other one is how many? Unique entities are there. And and that I really think it’s most fitting. I hate to generalize, but I’m going to say in the 95 percentile, you’re best off having a pessimistic view. That you use for the organization and if you and I’ll give you an example. One thing might be a possible match. It’s possible when that’s presented to the marketing department, they become optimistic and move possible matches in to do a single mailing because it’s just, you know, a a campaign oriented. But that way you get. An exact entity account across an exact entity account across the enterprise. Yet I will call it through a lens of optimism. Or in the moment, doing dynamic entity resolution taking a portion. Of the graph, so to speak. And then in that moment, using something or optimistic or householding, even if that entity is now a surname and an address. And and then using that in the. You know for the different. For example, marketing group or somebody? But you can really have. It’s easier to have a pessimistic view and optimistically roll things in as you service the enterprise, then have an optimistic graph. And then try to. Figure out how to bust things out. Downstream for for really precision things like, hey, we’re going to cancel their account or call it a fraud alert when you’re sitting there.
Speaker
Right?
Malcolm Hawker
I used to explain this at Gartner. Often when I had conversations trying to explain the kind. Of this concept of. Fit for purpose, right? What what’s what’s? What’s the right fit for purpose here and and and the phrase that I often used was what’s the? Cost of being wrong. Right and in the marketing world, the cost of being wrong is generally fairly low. I think that’s kind of the way that I was expressing what you. Would call an optimistic. Reading meaning meaning we would err for. It’s OK to have more false positives. I think it’s what you. What that’s that’s what you’re using. The word optimistic is. Is it’s OK if there’s? Some false positive work their way in because. Oh, we give the wrong offer. They get the. Wrong piece of mail. It’s not optimal, but we’re not going to get sued. Probably right not, but on the on the kind of the finance fraud, compliance, national security level. That’s where you need to be more pessimistic and where you need to be buttoned down on the false positives, because that could be. The difference between well. A lot, a lot of different dire. Consequences the the least of which being, you know, running afoul with regulatory constraints. You you agree.
Jeff Jonas
Yeah, and and falsely too many false positives in systems where you’re you’re looking for risk and bad actors, you know.
Malcolm Hawker
Are they saying you said?
Jeff Jonas
Bad guy hunting is you’re stopping the wrong people. You’re infringing on people’s rights and freedoms. There’s that way back when. TSA used to do its matching. I used to. Get a lot of calls from. People before they introduced date of birth. Because so many people were being stopped and questioned because of the false positives. You know it needed some additional attributes.
Malcolm Hawker
Yeah, I remember that after 911. Where, where you would hear all the time that. They would like like. Senators and Congress people were finding their way their their way under the No fly list, right? And that was a big deal, right? After 911, I remember that. Yeah, so so is is it safe to? Assume then that within. Your solution they’re potentially leveraging an NDA for this. I’m not sure whether. It would matter, but with. That those views, the pessimistic versus optimistic view, could be expressed by consuming application or consumer.
Jeff Jonas
Oh yeah, OK, yeah, what what? You do with a sensing. Is you likely? You can run a version pessimistic and a version optimistic. But if you’re doing enterprise and data fabric around this, you’d likely have a pessimistic view and in the moment as you’re publishing data, you’d roll things up. You’d roll in possible matches. It’s a super simple transform. We’re JSON and JSON out like we’re a developer tool, right? Like we’re an API, so the output that we produce, though, produces the evidence that you would need if possible matches something else kind. Ambiguous that you can just easily roll it, right? Up and combine it and get that you know. Single optimistic view and then I accidentally get sent to hair care product, you know?
Malcolm Hawker
Hey, you never know you never know. You you could. You could you could want to soften that skin maybe a little bit, who knows. So interesting in in kind of old school MDM. World, there’s always been an assumption that the algorithms can only get us so far right, and the and that. You need to have some sort of. Capability and this is still a. Critical capability for MDM.
Speaker
Like if you if you.
Malcolm Hawker
Look at, you know. Gardening magic quadrant. They will say Gardner will say you need to have a capability for data stewardship. Because the algorithms are only so smart.
Jeff Jonas
Right?
Malcolm Hawker
First of all, do you agree with?
Jeff Jonas
That assertion, there are definitely times, cases and data where you want a human to take a look to make an adjudication. But I will tell you in large scale systems you don’t have enough humans. Second, I will tell you how does. The human do it. The human is using additional data, it’s either data stuck in their head. OK, or they’re Googling it or searching up somewhere or calling the patient, but a lot of times. You got to actually do research, OK? I remember testing. Some data from Venice Beach, California business data and I lived there so I knew things about the locality. And there were two there’s. Lincoln Blvd. Goes right through, right, right through it. There was a locksmith store here and here. I thought my software did it wrong and then I googled it and realized no. I thought my software did it, which. Way was it anyway, I Googled. It and realized my. Software was wrong and then I called them and it turned out they just moved to the software was right. So so making these decisions. About records with some human intervention is about adding data. And one of the most. Exciting things that we propose. Is there are kinds of data? Often the organization has in a different data set over there. That is, the additional data needed, right? Wouldn’t it not be magical if 30% of your maybes got resolved just by widening the observation space? And because our software is sequence neutral meaning did you? Did you leave record a first then? B then C or C first. Then B, then a because it doesn’t matter, it fixes it no? Matter what way it’s loaded. You can load a few data sets and go well. We got. We got quite a few possible matches there. We’re going to need. 70 people to review those for a year. Or then you’ll ask yourself, hey, maybe we can buy some corporate registry data from Donna Bradstreet, or get it from open corporates, your Vandyke. Or maybe we have an extra set of data in. The enterprise and then you. Just load that and it cleans them up. It zippers some together and moves them into matches, and it moves the others over here into relationships. So you have to have govern. That’s these are things that we don’t do. We just do the matching and then people build this into things that would have governance and visualization.
Malcolm Hawker
Right, well what you describe. I I I kind. Of at least through. The lens of MDM use cases what you described. I kind of posited as a bit of a paradox when I was a gardener and I actually wrote about this at a certain amount when I was a gardener, which is this idea that with the technology that we have today. With with knowledge graphs with. AI and ML in theory. You could keep adding. More and more and more data to a an evaluation set. Shall we say I don’t know? A better word, but the candidate data source data, some sort of data. That’s being evaluated. The more and more data. That you add to that process, the more insights you could drive from an Indian perspective. As a matter. Of fact, if your graphs are good. Enough you could be you. Could figure out some of the unknown notes right? You could you could. You could start to understand relationships that are relevant from an MDM perspective that you didn’t even know. Well in the Oh yeah. However, historically, historically, when you start throwing a lot of data into this process, it becomes very compute intense and things start to really, really slow down. Is that what you’re seeing now or or are things changing?
Jeff Jonas
Well, I will tell you. Our fifth generation engine was scaling to. You know a. 100 billion input records, and we’ve learned a few things. And when we started the 6th Gen. engine and I said I gave the $50 million budget, we literally spent one year on the schema. The structure of the of the data store. Structure governs function. I can look at the schema of a database or data modelers can and you can tell them what the behavioral be. You can tell them when you see the structure and the indexes. You can tell them how that, what, what it can do and.
Speaker 3
Can’t do we? Spent a year just modeling the schema because if you get the schema wrong.
Jeff Jonas
You can’t scale. You have a full rewrite, right? And I’ll tell you what the hardest thing. To do in this space. We’re transactional entity resolution, which means while you’re loading a bulk batch of 100 million records while you’re doing user queries while you’re onboarding new customers Wednesday, Tuesday, and you’re doing 10,000 GDPR deletes all at the. Time no AV system, no reload. Now you’ve you’ve ingested hundreds of millions of let’s just say, a billion records and our big customers have 10 billion records. You have a billion records. You get one record in not only you have to figure out is it a new entity or. A known entity. You have to ask yourself. Had I known at the beginning.
Speaker 3
Over the building decisions.
Jeff Jonas
That I’ve made already.
Speaker 3
When I’ve made any differently.
Speaker
Right? Right, yeah?
Jeff Jonas
Yeah, would this be the evidence that that’s a junior senior and should take them apart? Or is that the evidence that this PAT is really that Patricia? Not that Patrick. Doing that in real time at thousands of transactions a second and fixing the past. Is is honestly? That and and simple you don’t have to train it and tune it anymore. Self tuning and self self learning out-of-the-box is what makes something the difference between you know 10 million to build and 50.
Malcolm Hawker
Right, exactly that’s the that’s. The you don’t want to try to make this. On your own argument. One thing that I find. A little a little interesting here is. Maybe I’m a little old, I’m a. Little old school and I’m. Kind of getting back to the question about is. There going to always be a role. For for data. Stewards and adjudication, particularly in those pessimistic use cases. That you were talking about. Where where you need to button things down. Historically, I’ve kind of always. Kind of leaned towards what we’ll always need humans.
Speaker 3
But I’ll tell you.
Malcolm Hawker
One of one of the more kind. Of lasting conversations that I ever had as a Gartner analyst and and I can’t. I can’t say who it was with, but it was with a vendor that was very active in the kind of the AIML space. And I said. Well, we will always need data stewards. We’ll always need human beings in this. In this process flow. Because the this. The machines can only be so smart and and the answer to that from this person, which really still sticks in my head. To this day, the answer was, well, that assumes that the people are smarter than the machines. And and what this person was saying. Is that is. That they had run test after test. After test where the machine was outperforming consistently outperforming the human and in a more predictable, explainable way.
Speaker 3
Well for a.
Jeff Jonas
We see that when we’re we’re producing better than human results out of. The box and the and. The way that you can easily observe. That is, if humans look at the same data set, just try to sort it. Out by themselves they. Might find a few things we miss. We’ll find more things they missed, and even if it comes down to exhaustion, you know just there’s only so long you just you’re looking at messy addresses. You don’t realize the part of the address that matching kind. Of over here. Tucked in and. So you know, we’re consistently outperformed. That, but I will say this is that. Humans have a lot of orthogonal data and judges. And very few AI ML domains have a sufficient amount. A sufficiently wide and diverse observation space to have human judgment, and the more significant those decisions are. And let’s say in an MDM context. Where maybe you have more maybes than you would want to task A-Team for. You would triage it to the ones that your top 500 customers, for example where it’s a bigger consequence to treat, you know in in Vegas. We call them a whale you you do not. Want to take a whale and send them a promotion that says if you come in on a Saturday you can have $3 off? May right? And so it you really can use your data. To triage it, but I think. It’s going to be a long time before. In some domains where. The judgment of humans you can just.
Malcolm Hawker
Ignore right I. I think half the battle. At least this was true for me when I was responsible for entity resolution and responsible for everything. Half the battle was explained. Ability and defensive.
Speaker
Right could could.
Malcolm Hawker
You explain why somebody was looking at what they were looking at and and could you. Defend it, right? Do you? Do you have a like standing strong enough to stand on and and often when it came down to human judgment it was OK? Human did the best with the information. That they had. That’s it. I mean and again if it’s. If it’s not something that is a compliance use case where it’s like OK, well, the human error and now we’re getting sued, not good. They’re just talking about the use case. That you just described right which? Is like a reward program or you know you. Know making sure somebody gets the. Right offer the right content. To me it was. I was able to. Say OK, human made this call. They may have made the wrong call, but with. The information that they. Had available it. Was the best at the. Time and and and for my stakeholders. The time that was that was good enough, and I think what we’re seeing now is with machines. It was OK. Well, we should have known this right and wrong answer these bad machines and and what I just heard you say is we’ll never have a training set big enough. I’m I’m paraphrasing you now.
Speaker 3
Most most are.
Jeff Jonas
Organizations won’t have a training set big enough. And big enough not as just vertically deep. Right? Really wide right? Most don’t, unless you’re the biggest companies in the world and you have a representation. Of all of the cultures. Just a simple example. Arabic names Ben and Hodge isn’t. Really part of the. Name just the way you think about matching names needs to be different.
Malcolm Hawker
Right?
Jeff Jonas
And you’re not going to match a Cyrillic name that’s in Russian, but the way you’re going to match a Chinese name. In you know a Mandarin name. And are you going to have a sufficient amount of data to train all of that and? The answer to the 99 percentile is.
Malcolm Hawker
No right exactly yeah, so in our. Last few minutes here, I I’d love to to. Hear your thoughts. On where you kind of see things are going right like like let’s let’s wind forward the clock. Five years ish maybe? We we continue on a path of digital transformation and digital acceleration and we kind. Of the world continues. Going where it’s going. From the. Perspective of of entity. Resolution and and where that fits in the modern data ecosystem and where that fits from. The overall data strategy perspective. Where do you see things heading? What’s different and and? What would a CEO? You just start thinking about. Now to respond to this future state that you described.
Jeff Jonas
I think it’s going to become more. And more obvious that high quality and into resolution sitting in between raw data and decisions is essential. If you’re making any decisions and you’re not accurately counting, is it three people or one? All downstream decisions all downstream learning are going to be very materially effective, but that is going to be very difficult to compete, and so in the you know, in the big elephant big animal picture, right? It’s data sources are gonna are gonna take performance context I’ve done over the years. A lot of presentations around the use of puzzles. You know the difference. Between a pile of puzzle pieces. And a puzzle assembled. And ending resolution is just one of those processes that is organizing the entities and graphing them for downstream consumption. I think the second thing is making better decisions. Faster is going to be essential. To be competitive. So people running batch processes, whether you’re reloading once a day or once a month. Or once 1/4. Where you have the right information, but you can’t see it. It’s like trying to cross across the. Street when you can only see how it. Looked 5 minutes ago like it is not competitive and So what? I think we’re going to see is more. You can call it a new resolution, expertly counting your entities right expert? It’s a form of expert counting. You’re going to see. Real real time counting of who’s who and who’s related who and using that to to drive decisions while they’re happening. Not after the fact, not like oh we already gave them loan. Oh we already gave them the car. They already let. Him in the country or we already used that. We already missed that opportunity to market to them in in moment when they were logged into the portal, and so I think the most competitive organizations are going to. Be able to make sense of what they know. While that they’re. Observing it so they can do something about it. So I think we’re going to see a collapse. In latency. To do real time decisioning?
Malcolm Hawker
So it’s it’s interesting historically, any resolution. Well, not always, but to. A certain degree, at least from my perspective I I tend to be a little kind. Of IT centric has has. Lived in the world of data management with data quality. MDM which has always been. And the data work back office right. And and that’s why I think you know it’s it’s batch right? Like we we do. Our report runs once every 24 hours. And if we. Can keep it up daily that that’s good enough. Whether that is a a load for BI or whether that is a load for data quality or whether even it’s just an ETL process that is supporting some sort of operational. Use case downstream. What you’re describing seems to be a world where those data management processes, which would arguably include entity resolution and potentially even others, including data quality, MDM lots of other where those things are no longer over there on the other side of the fence where they’re no longer. Kind of in this. Other world where they are deeply intertwined with application. Processes with CRM processes with the ERP processes you agree.
Jeff Jonas
Yeah, in fact. I mean, you’re I’m thinking. Out loud here, but. Whether it’s 3070 or 50. 50 or 7030. There is any resolution processes that get built into etls and MDMS. People have been building. I’ll call them third world. Sorry, but you know minimum viable products. First base attempts at any resolution.
Speaker 3
But those those live in.
Jeff Jonas
All kinds like CRMS and Kycc and amls. All of these systems vendor supply chains. All these things where you’re trying to not onboard duplicates. You’re trying to find connections between these customers and those customers. It it’s a.
Speaker 3
It’s a different.
Jeff Jonas
Family use of entity resolution, but it’s the. Same thing you know. And I think what we’ll see is. I mean, MDM products is really entity resolution with governance and synchronization, right?
Speaker 3
When you do that, I.
Jeff Jonas
Think we’re going to see the future where I’ll call on MDM Systems, but they’re really entity 360 systems with governance. The sink. There’s a chance, especially if they can service more elements of the enterprise. You know they can service larger scales. They can service real time. I think we’re going to see them used as components in these other workflows where the the group that’s got the custom AML software inside the bank is just going to use. The Enterprise entity resolution or entity 360. And benefit from the governance in the sink and the. Things that come. With it so.
Malcolm Hawker
Any resolution as a service?
Jeff Jonas
Was going to say, you know.
Speaker
Any resolution as.
Malcolm Hawker
A service you got to add the as a service to it right in in DC where I was advocating governance as a service which of course is gas so that one made us stick as well. But that’s basically what I’m hearing you say. Right, which is which is it’s just. The service that. You call and maybe it’s a shared service enterprise wide that it manages and maintains, but that is is going to be deeply embedded into the into the. Applications that need it.
Jeff Jonas
Yeah, and I I think. Less, I think a big trend is as the terminology and the commonality of it becomes more known and how ubiquitous the problem is. I think it’s going to become more commoditized. Yeah, you know where? Just people can get access to it with and not have to toil on it and have a team at 10. Or twenty of their best. Just work on that. They can work on something else.
Malcolm Hawker
Right? Or where what I was even talking about in DC is potentially some of these use cases become. Cross company or intra or Inter company right where it is is if if you are part of a complex value chain, for example a supply chain where every every participant in that chain has access to some of that. Capability right where you have access to that capability and where the answers are. Consistent across or. Relatively consistent, or at least predictable across. The value chain.
Jeff Jonas
That’s that is companies that are really engaged in digital transformation are going to put in any services in their data fabric. It will be a tier one player, right? Yeah, and it’s going to service enterprise needs.
Speaker 3
Although I’ll tell you on the.
Jeff Jonas
Theory sometimes when you have a really big project and it’s going to be really important. If people realize how important it’s going to be, there’s a lot of committees and subcommittees formed. I would argue that if we knew how important Internet was going to be in the beginning, we might have still been working on the subcommittees around it, you know? And I say this because there’s a tip for a CEO is if you have a really big horizontal vision, you might get one running first. And then promote it up. Start letting it take on a horizontal role. If you’re a big complex company and you announce to because maybe there’s other CEO’s and other groups. Or maybe you’re just herding cats because you’re CEO of all CEO. Those to tell somebody there’s going to be one in service everybody. You’ll spend you could. You could spend years bringing it together instead. It’s best to have a totally held conspiracy to do good just. Pull one up. Make it great and start making it available horizontally. That’s I think a better way with less friction to have a bigger company impact.
Malcolm Hawker
I I couldn’t agree more this. Is going to be worse for that right like Scout strategy? Or something, right? Because I’ve absolutely seen this, which is I’m going to build a shared service. Whatever the shared service is, I’m going to build, I’m going to build it and you’re going to. Use it. No, I’m not.
Speaker
Well, yeah.
Jeff Jonas
Everyone comes.
Malcolm Hawker
No, I’m not. Why am I going?
Speaker 3
To do why?
Malcolm Hawker
Am I going to do this right I’m. Not, I’m not doing. This I’m not. I’m not taking the edit versus the. I’m going to build this and I’m going to show some value and then you’re going. To want to use it. Because you’re going to see how valuable it is. So certainly some some truth. Here maybe that’s just touching a little bit more on human nature and. You know we we need to figure things out on our own data. People, kind of IT people we certainly do. That’s that’s true. So I love that. Right, that’s a great. Way to end the conversation. I think that the the last nugget of wisdom. There for aspiring or. Current CEO’s and and and how to be stealthy and executing their data strategy. I I love it. Jeff, thanks so much for your time. As always, good to see you are you home.
Speaker
By the way.
Jeff Jonas
I called Vegas home, but I I’m dramatic so I’m out and about.
Malcolm Hawker
Well, I know because every time. I talk to you. You’re somewhere else like you’re well I’m in Lisbon or I’m. In London or I’m. I’m somewhere else you’re traveling the world, doing your marathons and and and.
Jeff Jonas
Triathlons, triathlons, triathlons, marathon. At the end, it’s still a marathon. That’s just the last part.
Malcolm Hawker
Yeah, yeah, yeah, yeah, you’re you’re. You’re you’re making the marathoners. Look, you know like underachievers. Is what you’re saying with your focus on the on the triathlon so?
Jeff Jonas
Not not at my speed. Not at my speed.
Malcolm Hawker
So good to see you enjoying our chat. Look forward to doing it again. Sometime in the near future and so.
Jeff Jonas
Yeah, awesome, thanks for having me join your.
Malcolm Hawker
Thank you and for our listeners. Thank you again for tuning into CDO matters. I look forward to another episode with you sometime soon.
ABOUT THE SHOW
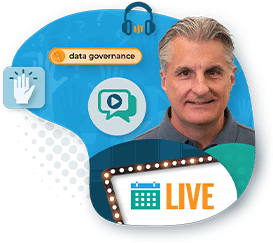
Malcolm Hawker
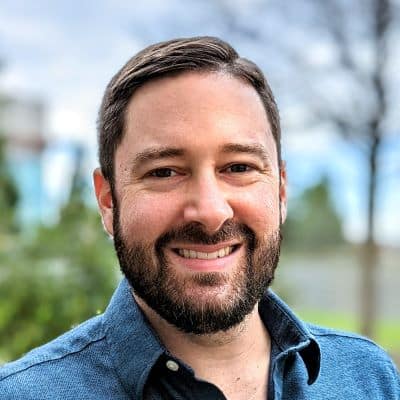
Benjamin Bourgeois
Ben Bourgeois is the Head of Product and Customer Marketing at Profisee, where he leads the strategy for market positioning, messaging and go-to-market execution. He oversees a team of senior product marketing leaders responsible for competitive intelligence, analyst relations, sales enablement and product launches. He has experience managing teams across the B2B SaaS, healthcare, global energy and manufacturing industries.