With the many different business intelligence (BI) and analytics solutions available to organizations today, they can directly benefit from streamlined data access and simplified control. But despite this development, many organizations continue to struggle to maintain trusted data. And when business owners or customers question the trustworthiness of this critical data, businesses are at increased risk.
Although data governance helps define data ownership and quality standards throughout the organization, enterprises often have a very limited, monolithic view and understanding of the implementation process when optimizing analytics capabilities and business outcomes.
As a result, organizations mistakenly attempt to treat all their enterprise data as master data – which places an unintended burden of more stringent data governance. By mischaracterizing and misjudging their data, its needs and the responsibilities involved, they overburden their data governance initiative and set it up for failure.
The 4 Critical Data Types for Data Governance
When taking a measured approach to governance implementation, it is important to understand the four broad categories of enterprise data involved to optimize results and impact across the organization. Effective data governance means applying it appropriately to each individual data type.
Here are the four broad data categories to understand for data governance (as shown in the graphic below):
1. Master Data
When determining what qualifies as master data, the two biggest factors are that it is slowly changing and widely shared throughout the enterprise across multiple data sources. Examples of this include customer name and address, product SKU number/categories, supplier locations and more.
This critical information must remain consistent across every business system for the data to remain trustworthy, thus requiring the most stringent level of governance. However, this is not to say that this process does not apply to multiple data domains.
In some instances, data governance technology may determine that a singular definition for a data attribute is not plausible throughout the organization even if the definition of a customer frequently does not match across operational source systems. If these definitions cannot be mapped to each other, then multidomain master data management (MDM) technology allows the data model to be constructed accordingly.
2. Application Data
Based on its definition alone, application data is the opposite of master data. This information is rapidly changing (event-driven) and is far less shared across business operations. Despite these huge differences, there is still confusion between the two because application systems often contain master data attributes (e.g., customer’s name). This confusion may prevent a successful digital transformation.
Governance is also affected by this data confusion. For this reason, it is important to create separate governance policies for each data category.
For example, if policies are set and enforced for a customer’s name, this will include instances of the name across every data source. Once master data attributes are recognized, the same level of master data governance can be excluded from application systems, provided it is being enforced by the MDM system.
When applying this initiative to the event-driven information that comes with application data (e.g., an issue billing a customer), urgent attention is usually required by default. Inaccurate application data frequently causes costly operational issues that need immediate attention and are fixed quickly while residing in operational and analytical data silos. Less-stringent governance is required for application systems because these attributes tend to be more fit for purpose due to their limited scope (as opposed to master data).
3. External/Purchased Data
External/purchased data — although possessing certain qualities of master or application data — is purchased or acquired to supplement internal data. This certainly changes things when it comes to data governance.
These values cannot be traditionally governed because they are determined by an external entity, often a data broker. This data comes into play when there is no internal counterpart or if an enterprise is updating internal data attributes, believing the external data source has better attribute values.
in either instance, the eventual internal record of this data needs to be governed under the policies and processes described for master and application systems. This level of governance operates more from a business perspective as opposed to a technical one.e.
When data is purchased, often the most valuable function performed is the analysis and consolidation of multiple licenses for the same data in the organization. This situation often arises when multiple departments each address a specific need at a point in time without concentrating on benefiting the larger organization. Functions for purchased/acquired data are more concerned with the specific operational/analytical environments in which the data is used. External governance does not require the same expertise level necessary for internally sourced data.
4. Internet of Things (IoT)/Big Data
The level of data governance required for big data from Internet of Things (IoT) goes hand-in-hand with that of external/purchased data. Oftentimes, these two data categories experience significant overlap. This is primarily because the inability to govern individual data attribute values inherently results in more sophisticated aggregate data quality checks.
The lighter governance level — like the external/purchased category — often requires fewer total data stewards with only one steward often handling both the IoT/big data and external/purchased realms. Although the steward’s experience and background determine their efficiency in handling both categories, combining the two drastically simplifies the governance process.
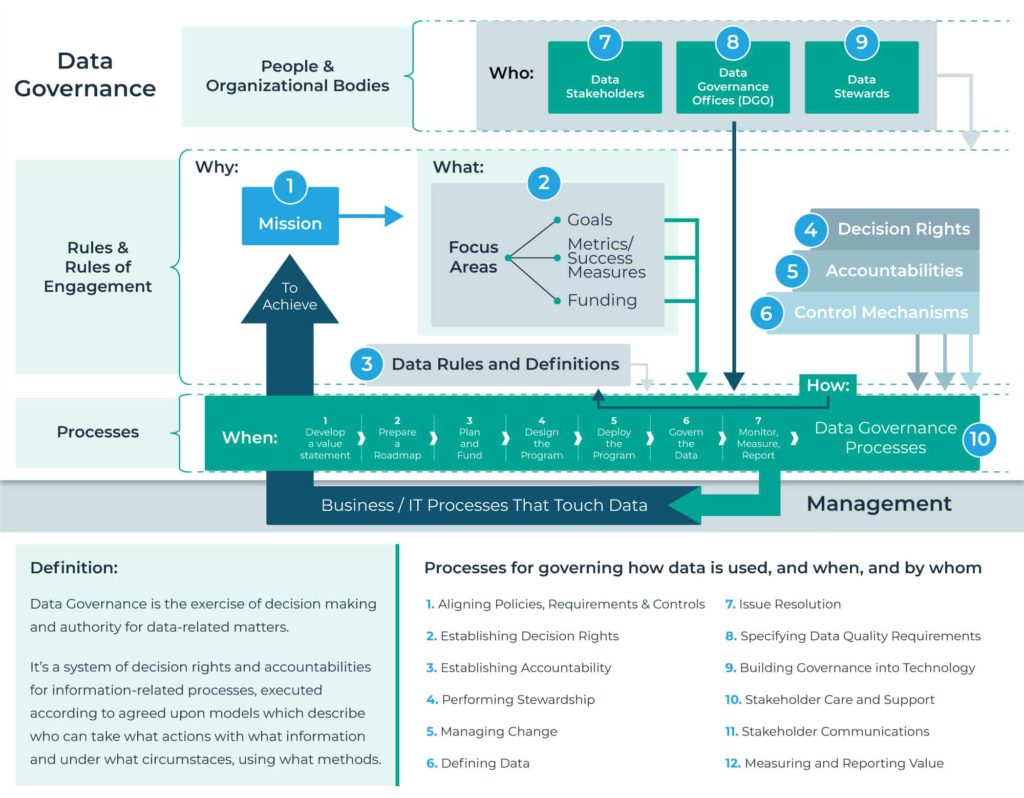
Data Governance Implementation Best Practices
After gaining a better understanding of each of these broad categories and the various levels of governance involved, the implementation process feels far less tedious and intimidating. No longer does all data appear as if it needs to be governed at the level of master data.
To successfully leverage the requirements of these categories, some best practices during governance implementation include:
1. Starting with master data, but not all of it
- Create an analytics implementation road map for better data prioritization or a data model only pertaining to the master data attributes required to deliver the initial set of identified use cases.
2. Avoiding over-governance of the application data category
- Once required master data attributes are governed, focus on the remaining application data required to deliver prioritized BI and analytics use cases, obtain agreements from stakeholders, and conform those values to the standard with minimal technology.
3. Applying the lighter touch of governance required for the external/purchased and IoT/big data categories
- While it is impossible to govern the attribute values for these categories, it is important to aim your governance efforts at defining their proper use of data sources within the organization, focusing on metadata rather than actual data while also minimizing stewardship.
Data Governance for Maximum Business Impact
By understanding the significance of data governance and how it can best be leveraged for each of your enterprise data categories, you can exponentially grow your business with a solid foundation built on trustworthy data.
Each type of data requires varying levels of governance which plays into your overall implementation initiative. Once governance levels are established, best practices ensure stronger data systems across your entire organization. With governed and trusted data, anything can happen.
To learn more about how data governance can optimize your BI and analytics impact, read my more in-depth guide and download your copy below.
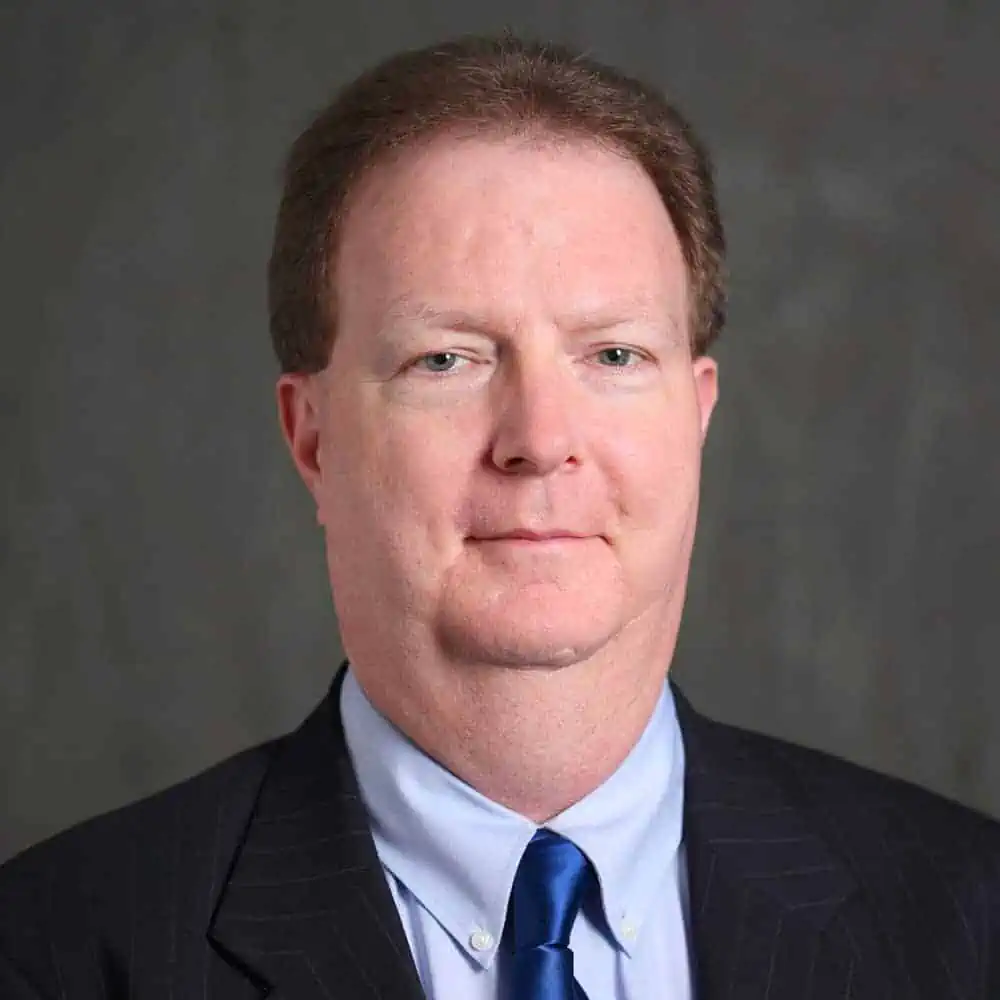
Bill O'Kane
A former Gartner analyst, Bill O'Kane was the VP & MDM Strategist at Profisee.