Hello CDO Matters Community!
Given we’re slightly more than a year into the explosion of gen AI, I’ve decided to devote an entire newsletter to give my “State of the AI Nation” update for data and analytics teams. I hope you enjoy it. Author’s note: This entire newsletter was created by yours truly, and was not generated in whole, or in part, by any form of AI.Hype is Fading, Knowledge Gaps Remain
As of early March 2024, it appears that much of the initial hype around generative AI and large language models (LLMs) is fading. For many, the hype is taking a back seat to all the hard work required to meaningfully and responsibly integrate these new technologies.
Based on my travels and my many ongoing conversations with data leaders across the globe, about half of all companies are using some form of gen AI (with most aspiring to do more) while another half is still trying to figure things out.
For this latter population, many still have significant concerns about their overall AI readiness as well as the quality of their data to support AI use cases. I spoke with a senior leader at a big company recently who specifically said they aspire to create gen AI models using internal data sitting in their data lake, even though most of that data is in a structure not suitable for training gen AI models.
While concerns about the quality of enterprise data are often valid, it’s still surprising that many of us do not recognize that text data is the preferred format for training gen AI models — and more importantly — that text data is usually completely ungoverned in most companies. This means that concerns about the suitability or quality of using existing data for training gen AI models are valid — but not for the reasons many cite.
The Fastest Path to AI Value Realization
Over the last year, it’s become abundantly obvious to me that the fastest path to utilize gen AI technologies in most organizations is through the adoption of chatbot and copilot-enabled smart agents. These agents essentially act as gen AI enabled apps, which mediate exchanges between the user and an LLM.
While it’s still important for users to understand how to optimally engineer prompts, in time these agents will increasingly become more context-aware and context-specific, not unlike using a steak knife to cut meat. You can use any knife you want to try to cut meat, but one is particularly well suited for the job.
The same will be true with copilots and smart agents, where agents will increasingly be built specifically to support specific use cases. This is already happening. As an example, look no further than the OpenAI copilots being deployed within Microsoft applications, such as the Power BI copilot. In many ways, it’s not incorrect to view these agents as rather powerful query writing bots, which will enable just about anyone to become a data analyst in your organization.
Operationalizing Chatbots and the Air Canada Debacle
Using copilots and smart agents will not negate the need to ensure the data they are consuming is accurate, consistent and trustworthy. (Although I do suspect that they will increasingly leverage capabilities — like using RAG patterns or intermediary queries to graph or vector databases — to reduce hallucinations and improve response accuracy.) But again, the reliability of what the copilots tell users will very much depend on the data they consume.
Defining internal policies for the proper use and configuration of these copilots, be they internally or externally facing, will be a critical dependency for their successful deployment.
Look no further than the recent news story citing an Air Canada chatbot that gave incorrect information about the company’s bereavement policy to a grieving customer. In a rather shocking twist, rather than admit their mistake and simply credit the customer for a ticket, Air Canada took the issue to court, attempting to claim that their AI smart agent wasn’t an agent of the company. Huh?
Thankfully, the grieving customer won their claim and Canada’s worst airline was forced to do the right thing.
When I first read about this issue, I naturally concluded that the chatbot had hallucinated, but based on the news stories I read, it’s unclear if that’s the case — even though that’s exactly how most media outlets framed the narrative. It’s equally possible that the information the chatbot cited was incorrect at the source, or it was incorrect when it was consumed during a training or fine-tuning exercise.
Put another way, the root of the problem highlighted in the Air Canada story could very well have been a software engineering or data governance problem and not a problem with the underlying LLM. My previous point about text data being “ungoverned” (or very poorly managed) could very well help explain what many have been prompted to believe is a problem with the AI.
The same is equally true with the behavior of the chatbot, which may have been a custom bot built on top of an opensource LLM that was incorrectly engineered.
The Air Canada chatbot has since been decommissioned, and the company left with a major PR headache. As nebulous as the cause of the issue may be, it certainly highlights the many issues that companies must navigate on their road to AI value realization.
This navigation must necessarily include the creation of acceptable use policies for AI models within companies (at a use case level), and it must also include policies that define how software engineers must interact with data scientists in the creation and deployment of any custom built or configured/fined tuned gen AI solution.
Data scientists building or configuring gen AI models can no longer operate in a vacuum and must work closely with their peers who are building applications that interact with their models. This is especially the case during both model development and model testing.
The same is true with people using gen AI internally, such as a software engineer using a copilot to automate some portions of code writing or testing. Changes to existing software development lifecycle policies must be made which ensure that any code generated by a copilot goes through the same level of rigor (or maybe even more) as a human would.
Are these policies “data governance” polices as we’ve historically defined them? I don’t think they are, although many data practitioners seem to be suggesting they are and that any use of AI requires “AI governance.”
DATA INSIGHTS,
DIRECT TO YOUR INBOX
Data and Analytics vs. Software Engineering
There is clearly an evolution afoot in the world of gen AI where software engineering functions will have increasing responsibility for the enforcement of AI governance in many companies. The behavior of gen AI models, and the data and processes used to create them, will be governed by data and analytics teams. This much seems clear.
Software engineers will write the apps that interact with AI models, and those engineers will ensure their software behaves within specific parameters — at least in situations where the software is built in-house and where out-of-the-box solutions from gen AI vendors are not the tool of choice.
This helps to explain why some companies are increasingly looking at their CTO teams to take more ownership over AI capabilities in their organizations. Some see this trend because of CDOs not moving quickly enough to deploy AI, while I think it can be easily explained when you consider that most companies will likely not be in the business of building AI models — but they will most certainly widely integrate them into business applications.
AI Governance
There are many people talking these days about “AI governance,” but to me, its definition is entirely murky. This isn’t a complete surprise given we are very early in the evolution of AI, but it’s something that certainly needs more attention. This dividing point for accountability of the behavior of AI-based solutions between those who build (and training) models, and those who deploy them within a complex internal application ecosystem, is evolving.
The division of accountability for the behavior of AI tools seems clearer, however, when what’s being used is a commercial out-of-the-box solution (like Microsoft Copilot), where accountability for model performance and accuracy would more clearly fall to those who are using the model within a user-facing application.
In this situation, it’s not unreasonable that data science teams could be involved in some form of a certification process for those vendor-provided solutions. This could become a valuable role for any AI governance committee within a data function, where experts who know how LLM technologies work would be in the best position to assess their suitability in a given company.
However, we must remember that as long as the gen AI model is consuming data from internal systems, data teams should remain accountable for the accuracy of that data. The interdependencies of the consumers of gen AI models and their builders (or certifiers) will probably give rise to more talk of “AI Ops.”
Let the hype begin!
Final Thoughts
For any of you who follow me on LinkedIn, you’ll know by now that I’m a big believer in the potential value of AI and that I believe any company not already doing some form of AI POC or limited-scope deployment is quickly falling behind.
Yes, we need to move with caution, and we need to ensure we’re not doing anything dumb, but I believe the best way to get ready for AI is to learn by doing. And through the use of commercial chatbots or copilots, there are plenty of opportunities to do that.
If you’ve made it this far, I applaud you!
Thank you for your support and for being a member of this ever-growing community of data professionals. My goal is to help you on your journey and to grow your career, so if there is anything I can do to help, I would love to hear from you.
Happy spring!
Malcolm Hawker
Head of Data Strategy @ Profisee
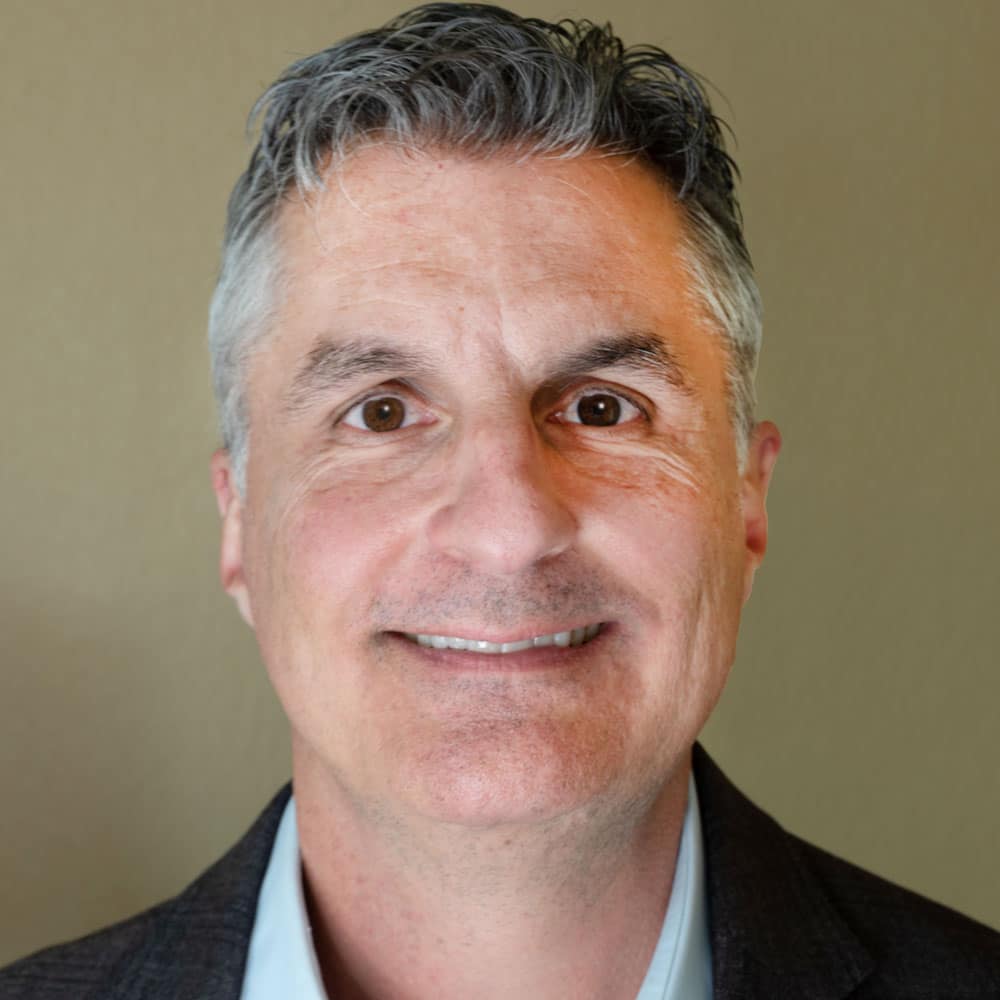
Malcolm Hawker
Malcolm Hawker is a former Gartner analyst and the Chief Data Officer at Profisee. Follow him on LinkedIn.