Table of Contents
- Data Governance vs. Master Data Management: Key Differences
- Should You Implement Data Governance or MDM First?
- Governing and Mastering the Most Critical Business Data
- Determining Data Quality Rules and a Conflict Resolution Process
- Data Governance: An Application or a Set of Principles?
- Coordinating Governance and MDM: Monitoring, Execution and More
- MDM And Data Governance: What Comes First, the Chicken or the Egg?
- Frequently Asked Questions
Updated: October 10, 2024
In the years that I covered data governance and master data management (MDM) as a Gartner analyst, data architects and CIOs frequently asked, “Why is our data such a mess?”
Though I have written at length about how we got into this mess, I want to share how data governance and MDM are key to digital transformation and, more importantly, getting real business value from your data.
I often hear from companies that implementing MDM and DG presents a bit of a “chicken or egg” problem. They ask, “Should I first define my data governance and quality standards or work match, merge and clean my business data from disparate systems across the enterprise?”
Read on to learn how to best coordinate and implement these critical programs. But first, I need to put them both in the right business context.
Data Governance vs. Master Data Management: Key Differences
Master data management and data governance are closely related programs that share some similarities, so it’s important to understand how exactly they differ — and relate — to one another.
- Function and Focus
- Data Governance: Data governance acts as the business function of MDM, controlling how data is created, collected and used. It focuses on policies, procedures and standards to ensure data quality and compliance.
- MDM: MDM is primarily a technical function that involves the management of master data entities and attributes to ensure consistency and accuracy across the organization. Critically, MDM enforces the policies defined by data governance to ensure data remains of high quality.
- Organizational Placement
- Data Governance: DG should optimally reside within the business side of the organization — not within IT — to achieve its full strategic value.
- MDM: Conversely, MDM typically resides within the IT department, focusing on the technical aspects of data management, like de-duplication, data enrichment and standardization.
- Scope of Responsibility:
- Data Governance: Data governance is responsible for all categories of data, including master data, transactional data and metadata.
- MDM: Master data management specifically focuses on master data, which is state-driven, slowly changing and widely shared across multiple sources.
- Implementation and Coordination:
- Data Governance: DG involves business stakeholders in defining and managing data policies, resolving definitional conflicts and ensuring data quality.
- MDM: MDM involves IT in creating and maintaining data models, integrating data sources and managing master data entities.
- Role of Business Stakeholders:
- Data Governance: DG requires active participation and ownership from business stakeholders, who may serve as data stewards or members of the data governance council.
- MDM: While business input is important, the primary responsibility lies with IT to implement and maintain the MDM system.
- Goals and Outcomes:
- Data Governance: Data governance aims to ensure data is accurate, consistent, and used appropriately to support business decisions and compliance.
- MDM: MDM aims to create a single, authoritative source of master data that can be used across the organization to improve data consistency and accuracy.
Should You Implement Data Governance or MDM First?
I argue that data governance and MDM are co-dependent disciplines. That is, companies cannot successfully implement one program without a commensurate focus on the other.
In this relationship, data governance is the “business function” of MDM, controlling how data is created, collected and used. For a governance program to reach its full potential of optimizing existing processes while generating new business opportunities, it needs to reside within the business as opposed to IT.
Once companies view their data governance initiative in this proper business context, they soon recognize the need for executive participation and stakeholder engagement. Then, this fully engaged group of stakeholders can formulate and ratify a set of business outcomes and engage IT to engineer a data model that services those outcomes.
Governing and Mastering the Most Critical Business Data
By first placing a data governance initiative in the proper business context, companies can focus on which data is most critical to their success and scope an implementation plan from there.
MDM implementers refer to this most critical data as master data, the core data within the enterprise that describes objects around which business is conducted. Master data is usually state-driven, slowly changing and shared widely across multiple source systems.
Just as key as having the business drive both governance and MDM, it is critical that that organizations properly scope their initiatives to avoid “boiling the ocean.” Governance, then, describes only the data entities and attributes that helps reach the previously defined business goals.
The key step here in coordinating MDM and data governance is to separate the target master data logically and physically from the non-master data in the model, as only the master data will be managed within the MDM system.
Determining Data Quality Rules and a Conflict Resolution Process
At this point, business stakeholders begin earnestly participating in data governance at all levels of the enterprise. At most organizations, leaders have formal meetings with their IT partners and begin the important work of governance, including:
- Resolving definitional conflicts between sources for each data element
- Recording any subsequent technical and business definitions, lineage and mapping or transformation rules
- Creating and documenting data quality rules
- Consolidating the number of definitions that are actually the same
- Adjusting the data model to reflect these governance rules.
At this stage, it is critical that business and IT stakeholders thoroughly examine their business data and co-author data definitions that help meet their organization’s goals.
Data Governance: An Application or a Set of Principles?
The most basic definition of data governance is that it is a set of principles and practices that ensure high quality through the complete lifecycle of an organization’s data.
Though it technically does not require a dedicated technology platform, data governance solutions have made it easier for organizations to scale their governance efforts and leverage their accumulated data assets in support of new business processes and analytics.
Some organizations acquire dedicated data governance or data catalog technology to track metadata, while others treat metadata as an additional master data domain and manage it within their MDM system.
Regardless of the technological approach, organizations need to walk through this “policy creation and management” phase of data governance to later track progress and resolve any further issues.
Once all conflicts have been resolved, the remaining metadata described previously can be captured, and the data quality rules also created and recorded.
Coordinating Governance and MDM: Monitoring, Execution and More
Policy creation and management is only the first step in implementing a data governance program and identifying the means to enforce its rules through an MDM implementation.
To learn more about the “monitoring and execution” phase of data governance — as well as tips on how to configure, load and implement the MDM data model — read the full article: “MDM And Data Governance: What Comes First, the Chicken or the Egg?”
MDM And Data Governance: What Comes First, the Chicken or the Egg?
Frequently Asked Questions
What Is Data Governance?
Data governance is the business function responsible for managing how data is created, collected and used within an organization. It involves establishing policies, procedures and standards to ensure data quality, consistency and compliance.
By residing within the business side rather than IT, data governance aims to maximize strategic value and prevent data from being seen as mere IT overhead. Effective data governance requires active participation from business stakeholders, who help define data policies, resolve conflicts and ensure data quality.
This approach ensures that data governance aligns with business goals, enhances decision-making, and prevents the creation of additional data silos. Effective data governance transforms data into a strategic asset, driving business value and operational efficiency.
What Is Master Data Management?
Master data management (MDM) is the process of managing an organization’s critical master data to provide a single, consistent and accurate view of key business entities. MDM involves the identification, consolidation and maintenance of master data, ensuring it is reliable and accessible across various systems and departments.
Typically managed by the IT department, MDM includes creating data models, integrating disparate data sources and implementing data quality measures. By centralizing master data, MDM supports better decision-making, operational efficiency and data consistency.
MDM and data governance work together, with MDM handling the technical aspects and data governance ensuring proper data stewardship and compliance. In other words, data governance establishes policies for acceptable data quality while master data management enforces those rules to cleanse and standardize data so that it is consumable by downstream systems.
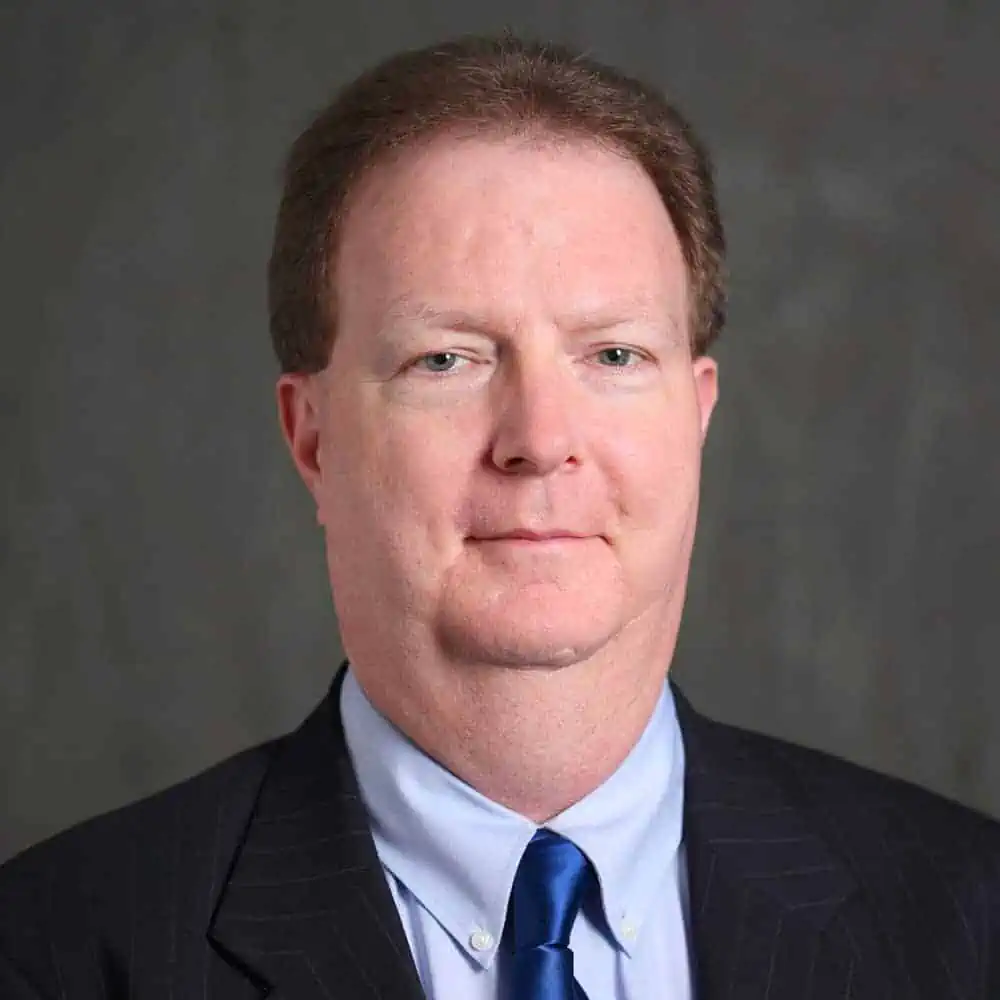
Bill O'Kane
A former Gartner analyst, Bill O'Kane was the VP & MDM Strategist at Profisee.