Past experience has taught most data managers that the number of big data projects on their lists will only continue to grow. It seems always to be the case that one business executive or another has a job for them to do, and possibly
in a very tight timeframe.
It’s exciting, of course, when they get to play a role in strategic corporate initiatives. For example, knowing that their work preparing data to help the company execute a customer growth plan affirms their value to the organization. While they may not have the right tools to be able to quickly and permanently clear up all big data inconsistencies that exist across various production systems, their input can at least improve company leaders’ ability to understand how many clients the business actually has at that point in time, where those customers shop and what they are buying for analytics purposes.
Protip: Making that data as consistent as possible under the circumstances can help with web content personalization, targeted upselling and other opportunities.
But their time also may be taken up by various one-off projects, such as manually troubleshooting data for a product line manager who must come up with accurate profitability calculations before his meeting with the boss – which is scheduled to take place the next week. Or they might be responsible for seemingly small but always-on tasks – compiling data from different systems and then updating and maintaining it in spreadsheets for various department heads so that they can perform complex calculations and analyze scenarios and outcomes, for instance.
Harness the Big Data Project Tornado
How to juggle all these responsibilities without missing incremental and final deadlines? The ideal approach is to implement master data management (MDM) technology. This will be the foundation for centralizing data management by creating master copies of data items. It will enable modeling, integrating and managing entities across multiple domains to ensure ongoing conformity among all the “nouns” that describe a business, such as customer, item number, unit price, distributor and so on.
Doing so is key to breaking the cycle of chaos that occurs when business units build their own applications and processes and create disparity in non-transactional data entities. These practices result in there being no single, cross-domain view of the current version of any and every entity. An MDM platform that enables a clean data foundation – one common definition of each entity with relevant reference data to help describe it – is designed to create trust in these definitions that will be shared across business units and multiple IT systems.
Business stakeholders often have to be convinced that there is value in implementing an MDM solution. Department heads may believe that chaos actually erupts when their systems and data definitions and practices are disturbed. So, higher-up data leaders – ideally a CDO – must make the case that the effort will result in sustaining data quality and delivering data dynamism across their own departments as well as the entire company, and lower costs with advanced management processes, too. But once buy-in is secured, the data managers who do the actual work of creating cleansed, verified, matched and de-duplicated data now have a holistic way to help the business accelerate analytics.
That won’t necessarily shorten the number of big data projects on their lists, but it will certainly make tasks easier and quicker to accomplish.
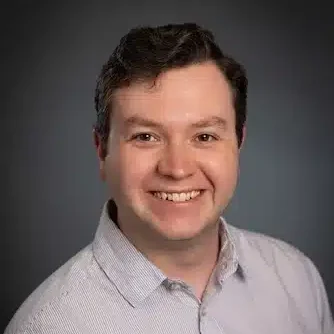
Forrest Brown
Forrest Brown is the Content Marketing Manager at Profisee and has been writing about B2B tech for eight years, spanning software categories like project management, enterprise resource planning (ERP) and now master data management (MDM). When he's not at work, Forrest enjoys playing music, writing and exploring the Atlanta food scene.