Key Takeaways
Data governance frameworks define rules, processes and ownership of data for the entire organization.
When implemented and followed, data governance frameworks put reliable data into the hands of all employees.
Data governance frameworks require buy-in across the enterprise for maximum success.
As companies take on new data sources faster than you can down a sleeve of Thin Mints and everyone wants to experiment with the latest AI tool using company data, data professionals might be forgiven for their Pepcid habits. A data governance framework that defines how the enterprise consumes and uses data may just prevent your data leader’s next ulcer. This article explains what a data governance framework is, how it’s useful to your team and what it might look like for your company.
What Is a Data Governance Framework?
A data governance framework is the set of rules, processes and ownership assignments that regulate data quality and usage across an organization.
The framework dictates how information enters, moves through, gets used by and deleted from the corporate network. A data governance framework simplifies data decisions and builds reference materials so companies can move faster and use their data more efficiently.
How Do Data Governance Frameworks Work?
Data governance frameworks are living documents that change and grow with the company’s data landscape and its data maturity level. They should also work as a cycle that the organization can repeat and expand upon as it brings more departments under governance.
The data framework of a single location’s ERP will look much different than the framework for that same company after an acquisition or merger. But at the simplest level, all data governance frameworks will define:
Total Data Governance
For the data governance project to succeed, the framework must cover every data source and use case across the organization. Framework rules and processes dictate:
- How new data sources will enter the system
- What shape the data will take upon cleansing
- How to identify reliable and usable data
- How to discover and integrate previously unknown sources
- What technology the company uses and for what purposes
- When processes must change
Ideal governance blankets the entire organization. The framework reduces the risk of malformed or unusable data that throws off the reliability consensus of the data currently in use.
Individual Accountability
In the ideal data situation, every person at the company takes responsibility for the accuracy and usability of the company’s data. Accountability requires:
- Frank communication between individual contributors (ICs), managers and leadership on how data does and will inform daily work
- Education and training to shift to a data-informed environment
- Stakeholders from every business unit to guide communication and decision making
- Scheduled audits followed by training, where needed
Data stewards or governance offices need to audit the existing data structures, update the documentation and guide training. Without individual accountability, data professionals will spend their days enforcing and reinforcing rather than improving processes.
Full Company Buy-In
The company shows its support for the data governance program by building governance into everyday behaviors. This requires proper training and documentation of processes within the departments and roles in addition to adherence to company-wide rules and processes as defined in the documentation.
Components of Data Governance Frameworks
Data governance frameworks answer the major questions that occur during data transformation. The resulting framework aligns data decisions to business priorities and company goals. The following breakdown is derived from the Data Governance Insititute’s data governance framework.
- Why: The mission of data governance. An ideal mission statement will serve all parts of the company.
- What: The goals that define the data governance program, informed by success metrics, funding and capacity. The goals should be prioritized from most to least impact on lines of business.
- How: The rules that regulate how data is collected, accessed, used and destroyed. These rules and processes ensure that data rules and business actions align and receive stakeholder support. The “How” of the framework should cover:
- Focus areas
- Data rules and definitions
- Decision rights and responsibilities
- Who: The people that support consistent adherence to data governance rules, continuously measure success and investigate shortfalls and communicate results and next actions to stakeholders and the company at large. The framework should outline:
- Accountability assignments for reporting and analysis
- Data stakeholders, including governance officers and stewards
- When: The training, technology and communication plans that support a successful data governance roll-out, upkeep and further projects.
Data Governance Framework Examples
There are five major data governance frameworks, but we’ll focus on those from the Data Governance Institute and McKinsey, as they sit at two ends of the spectrum when it comes to level of detail. Other frameworks include those by PwC, Data Management Body of Knowledge (DAMA-DMBoK), Deloitte and the Enterprise Data Management Council (EDM Council). These frameworks have varying levels of complexity and different forms (circles or layers instead of flowcharts), but they all have the same aim — to help the company conceptualize and implement the data governance framework.
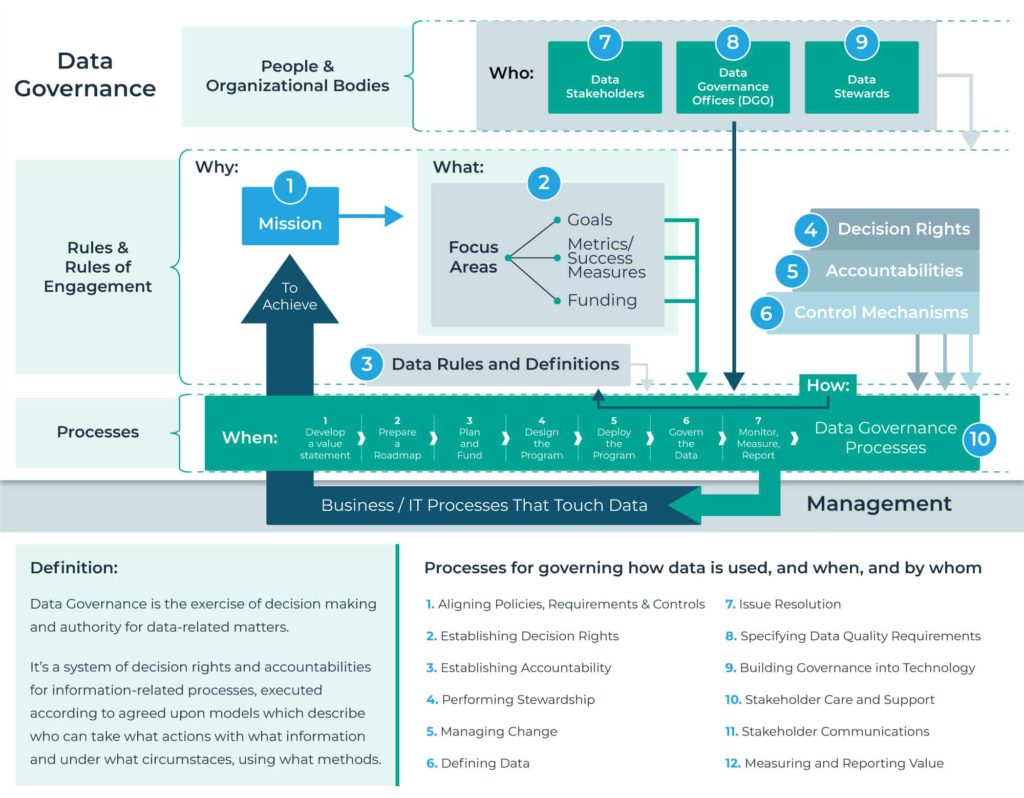
Data Governance Institute (DGI)
The DGI framework is highly detailed, with ten major components that cover the Why, What, How, Who and When of governance as outlined in our description of components above. The DGI framework also lists 12 governance processes that companies should address in the How stage. The DGI framework’s detail makes it helpful — if a bit overwhelming — for the broader enterprise’s reference and understanding of the goals and execution of the project.
McKinsey
McKinsey’s data governance framework takes an organizational stance, assigning rule, process and accountability duties to three different groups:
- Data management office (DMO)
- Data council
- Data leadership by domain
What this framework lacks in specificity, it makes up in flexibility. And don’t worry about finding enough people to make up all three groups — the DMO and the data leadership by domain collaborate as members of the data council.
Benefits of an Enterprise Data Governance Framework
A data governance framework will set the team up for success in current and future data projects. Here’s how.
More Reliable Data
More reliable data is more useful data. When the full team agrees upon and adheres to rules and processes for the data, everyone can feel more secure knowing the information they’re using to make decisions and drive the business is consistently accurate for all departments. Software tools with capabilities like fuzzy matching and reference data management help with data discrepancies.
Better Data Communication
When all parts of the enterprise can agree on the reliability of the data they use to make decisions, they can more easily communicate and collaborate on how to achieve the company’s goals. From master data like customer, location and product data to sales and revenue figures, a data governance framework assures all team members that their data is accurate and consistent.
Faster Decision Making
Data governance helps companies build stores of usable data, which enables faster decision-making by cutting down the amount of digging and cross-referencing one needs to do to feel confident in those decisions. Whereas departments may have spent hours or days finding the right metrics within disorganized data sources, well-governed data is available and ready for analysis. Reliable data is even more important when companies introduce AI through augmented data management, machine learning and AI assistants.
Data Democratization
A data-aware company educates and trains staff members of all experience and access levels to understand the data they can use. Well-informed teams can pull reports and analyze their data, reducing ticket numbers and report generation strain on data professionals. Teams that expect data literacy of its team members and support reliable data move faster and pivot quicker because they don’t have to write a request ticket for every report.
Regulatory Compliance
Data governance frameworks take regulatory compliance into account when planning processes and rules. Adherence to the data governance processes means compliance is built in. While companies will still need to audit and monitor data for security and compliance breaches, the data team can work by exception, moving the team from a reactive to a preventative stance.
How to Benchmark Your Data Governance Framework
Understanding the maturity of your data governance framework will take a mix of reflection on project success and company awareness of data. You can find maturity and benchmarking models from several well-known organizations including Gartner, Oracle, IBM and Stanford. Each of these needs to be adapted to the real-life work environment of your company.
Most maturity models have six levels that chart a company’s progress from least to most sophisticated:
- Unaware: No knowledge of data governance strategy or tactics.
- Aware: Leaders, data professionals and some major stakeholders understand the importance of data governance. They may or may not understand execution.
- Planning: Strategy, frameworks, use cases, technology and primary projects are identified and mapped.
- Project-Based: One or two projects successfully launched and company awareness of benefits of data governance is growing.
- Expansion: Data projects and education spread across the organization following the revised framework and strategy following the initial real-world implementation.
- Cohesion: The organization is data-literate, able to manage role or departmental data with little intervention and able to follow data governance mandates within daily workflows.
While it helps to be aware of these levels, they all indicate growth toward the ultimate goal. Use benchmarking to drive the next steps in your data governance journey, and keep looking toward that cohesive, democratic, and data-literate workplace.
Guide: The What, Why and How of Data Governance
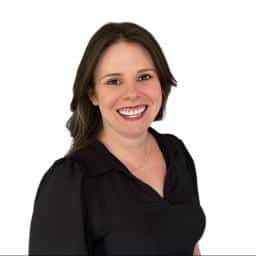
Tamara Scott
Tamara Scott is a writer, editor and content strategist with over a decade of experience located in Nashville, TN. Tamara holds a Master's in English from Belmont University, formerly served as Director of Content for TechRepublic, and her work has appeared in ServerWatch and EPI-USE.com, among others. When she's not crafting SEO-informed and conversion-ready content for SaaS and IT service companies, she's probably at home on her pottery wheel. Connect with her on LinkedIn.