Nearly two years since the meteoric explosion of ChatGPT and other generative AI solutions on to the consumer market, we have learned much about the impact of this transformational technology on the discipline of data management. While many longer-term adjustments will remain unknown for some time, the modifications needed for data management and governance programs to support the operationalization of gen AI based solutions at scale is becoming clearer.
What we’re learning — based on experiences coming out of the pandemic — is that companies that can quickly adapt to changing markets and technical dynamics will differentiate themselves from their competitors. This is most certainly true in an era of gen AI, and data practitioners able to understand the impacts of AI on data management and governance and modify their practices accordingly will be at a significant advantage.
AI Augmented Data Management
When I was an analyst at Gartner, I published research in 2020 outlining how augmented data management was impacting the discipline of master data management (MDM), and those perspectives are still relevant today. Augmented data management is an approach to data management in which advanced technologies and analytical platforms, including AI, provide added layers of insight and automation to traditional data management practices.
Broadly speaking, AI augmented processes are ones where AI and similar solutions make recommendations on how to optimize a given data management process, but where a human remains in the loop. Augmentation is an intermediate step in a progression towards process automation, where humans will still have ultimate accountability but are out of the decision loop. This progression is visualized in the graphic below.
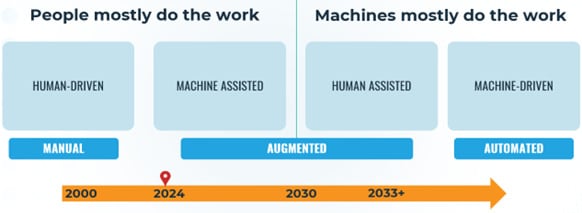
As you can see, we’re still very early into the augmentation phase of this progression. This doesn’t mean that some data management functions will not be automated by AI anytime sooner — many already are. It just means that in general, AI and advanced analytical methods (such as graph analytics) are focused primarily on improving human-driven processes.
We’ll touch more on the types of augmentation and the disciplines they impact in the next section.
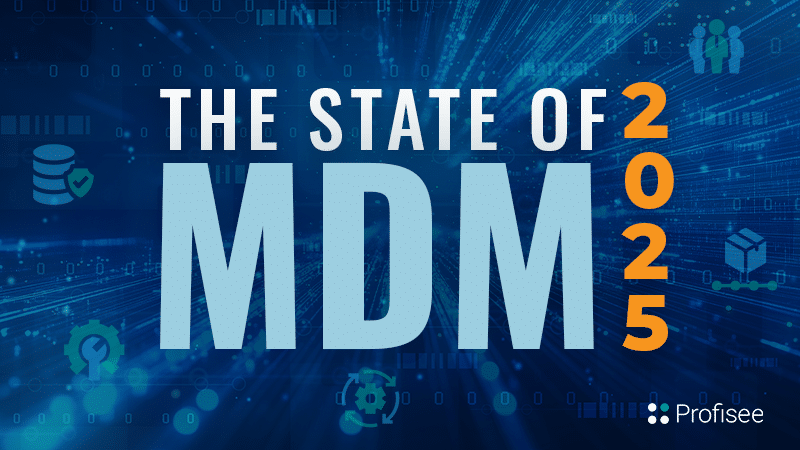
The State of Master Data Management (MDM) 2025
Learnings to Date and Current State — Market-Wide
Looking more broadly at the entire data management market — which includes the competencies of business intelligence (BI)/analytics, data integration, data quality, data governance, and master data management (MDM) — you’ll notice a few notable shifts are impacting the way organizations approach data management.
Gen AI Is How Most Companies Will Operationalize AI at Scale
Earlier this year I had the pleasure of giving a presentation at a Gartner Data and Analytics event with Piethein Strengholt, author of Data Management at Scale, where we shared our perspective on how gen AI-enabled chatbots and smart agents will be the primary way that most organizations operationalize AI in the short term. (He and I shared many of our perspectives in an episode of my CDO Matters Podcast, available here.)
I am confident that most companies — especially those without established data science functions — won’t be in the business of building or training large language models (LLMs). Major concerns for how to govern and manage data used to train AI models will be for the minority of companies willing to make significant investments in a data science function and supporting data management and governance processes.
For the rest, the biggest data management issues surrounding the use of smart agents and chatbots — including those using tools like Azure OpenAI Studio to build their own chatbots — is the use of RAG (retrieval augmented generation) patterns within complex prompts to mitigate hallucinations of out-of-the-box LLMs. Given generative AI solutions are built on and optimized with unstructured data, the use of internal data from structured sources within RAG patterns is limited, but slowly expanding.
Using knowledge graphs in RAG patterns to provide the necessary context about structured data so it can be consumed in a complex prompt holds great potential. This means knowledge graphs should be on any company’s radar that’s looking to bridge the world of structured data and the data chatbots consume in fine tuning processes, or complex prompting.
Focus on the Right Foundations: Unstructured Data and AI Governance
When ChatGPT burst on to the market, many pundits and consultants told data professionals that they needed to “focus on the foundations” of data management to ensure they were best positioned to build or use an AI-based solution. While this is true, exactly what foundations you focus on is extremely important, as are the types of AI you intend to use.
Generative AI solutions consume unstructured data. The reality of most data governance programs today is that most unstructured data is entirely un-governed — a reality I highlighted in an article I wrote for Forbes. If you intend to build or fine tune your own language models — or use unstructured data on complex prompts to “ground” the behavior of LLMs — then bringing your unstructured data into your data governance program is critical.
Another consideration is that it’s typical at companies with established data science functions for data scientists to not use the output of more traditional data management processes, such as data quality and master data management. Instead, data scientists extract data straight from source systems. What this means is that a “focus on foundations” through more traditional methods like data quality, governance and MDM processes will not benefit data scientists.
To realize the economies of scale a governance process provides, it’s important for these companies to find ways to integrate their data science functions into more traditional data management operating models. A great starting point for many companies here is the use of a single integrated cloud data management platform, such as Microsoft Fabric running in the Azure ecosystem.
AI Requires a Different Mindset
Another impact that AI is having on data management is that data leaders need to think differently about how they manage and govern data for AI use cases. AI-based solutions are inherently probabilistic, which means that applying legacy deterministic approaches to how you manage data designed for AI-based solutions is a bad fit.
One example is having the same data quality expectations for both AI and more classic analytics. In many situations, it’s fully expected that data used to build AI models will have a certain degree of variability since the goal of the model is to ensure the data used is representative of the broader dataset. Data used for analytics is held to a different standard, where the expectation is that data represents the most accurate expression of a business process.
In other words, the quality of data used by AI-based systems should model reality (the way things are), while the quality of data used for analytics should model an ideal reality (the way things should be).
The probabilistic nature of AI-based systems also means that applying highly rigid, broad-based quality standards to data used to build or optimize AI models is a bad fit. The quality rules should ultimately be defined not by enterprise-wide standards used by analytical tools but by the specific quality rules needed to support the AI use case.
Context Is King
The ability to use AI to start augmenting traditional data management processes (like data quality rules) requires additional insights into the context of how data is used across the organization. For many, this added context comes from the use of graph technologies to reveal previously unknown relationships in data.
The combination of knowledge graphs and AI to analyze large amounts of transactional data and metadata allows for the creation of AI models and what Gartner calls “recommendation engines” that will eventually enable the automation of many data management processes, including data governance. I wrote about this four years ago at Gartner, and significant progress has been made since then.
This combination of AI and advanced analytics is a foundational component of a data fabric, the capabilities of which go well beyond a data access and virtualization layer. My CDO Matters Podcast episode Demystifying the Data Fabric provides much more insights into the significant benefits of a data fabric, particularly around the automation of data management.
Examples here include:
- Using a combination of graph insights and some form of machine learning to start augmenting the process of entity resolution (matching) used in master data management systems — which vendors are increasingly offering
- Using graph-based insights to help augment or automate the process of modeling data and data hierarchies
- Using AI to recommend specific data quality rules designed to optimize the efficiency of a given business process
Vendors will increasingly enable these capabilities, but data teams with skilled resources in both graph and data science will be able to build their own.
Metadata as a First-Class Citizen: The Growth of Data Catalogs
Another impact of a heightened focus on AI in data management is the increased importance of metadata management. For many, this starts with the implementation of some form of a data catalog, which allows organizations to create robust inventories of their data estates.
With a growing focus on governing unstructured data, data teams are increasingly relying on catalogs to be the primary repository of information around the acceptable use of data within an organization — particularly for use by AI based systems. Rising popularity of data products is also fueling the growth of data catalogs — which, for those with a data science function, includes AI-based data models and products.
Data Management Software Systems Are Converging on the Cloud
The proliferation of data management and governance silos in organizations — including those which today separate data science from traditional analytics — creates the need for vendors to provide more holistic environments that combine multiple data workloads, pipelines and management applications into a single cohesive, interoperable cloud-based infrastructure.
Microsoft Fabric is a great example of this, as it combines data science, data warehousing and classic analytics into a single management platform. Other vendors like Snowflake and Databricks offer similar solutions. The ongoing explosion of data volumes creates even more motivation to move data workloads to the cloud, as does the expansion of computing power needed to build and test AI-based models.
These forces are converging, motivating data leaders to consolidate on a single IaaS service provider.
DATA INSIGHTS,
DIRECT TO YOUR INBOX
Use-Case Specific Impacts
Now let’s look more specifically at generative AI and consider some examples of how it’s impacting each data management discipline or use case.
Data Quality
- Data Anomaly Detection and Cleansing: Gen AI can be used to detect anomalies in data and suggest fixes to the data.
- Data Enrichment: Gen AI can be used to enrich data by analyzing and providing additional insights about data.
- Automated Data Classification and Standardization: Gen AI can be used to analyze data and suggest ways to standardize and classify it.
- NLP for Data Quality: Gen AI can be used to scan large amounts of unstructured data to help apply data quality and governance policies against that data.
- Data Observability: Gen AI solutions can be used to detect anomalies in data pipelines and — when coupled with machine learning-based systems — can be used to predict when pipelines will break.
Data Governance and Data Catalogs
- Data Discovery and Profiling: Gen AI is increasingly being used to discover and profile data, including complex relationships and data hierarchies. Identifying these relationships will play an increasingly important role in helping to provide the context needed to optimize the behavior of gen AI-based systems used in a business environment.
- Automated Metadata Generation and Enrichment: Gen AI can be used to automatically capture metadata and enrich it for publication into a data catalog.
- Data Governance Policy Augmentation/Augmentation: Generative AI solutions can be used to make recommendations on basic governance policies and flag data which may be out of compliance with those policies.
- Improved Data Lineage: Gen AI solutions can be used to automate the process of understanding the lineage of data in a complex ecosystem.
Data Integration
- Automated Data Mapping: Gen AI systems can be used to make recommendations on the optimal mapping between source and target environments.
- Automated Data Transformations: Gen AI systems can be used to automate the normalization or transformation of data needed during an integration process. This includes identifying data quality issues within an integration process and automating their resolution.
- Semantic Linking: Gen AI solutions can be used to help better understand the context of data, which can add capabilities to an integration process akin to what a semantic layer would offer.
Master Data Management
- Entity Resolution/Deduplication/Matching: Generative AI solutions can be used to help augment the processes used in entity resolution and can make recommendations for attributes of data to be included in a match process, or the optimal weightings of a given attribute in the match process.
- Hierarchy Management: Gen AI solutions can make recommendations about optimal hierarchies and can highlight previously unknown relationships in data.
- Data Stewardship: Gen AI solutions and chatbots can be used to help improve the productivity of data stewards, including processes like data validation, enrichment and classification.
- Data Modelling: Gen AI solutions can be used to help make recommendations for optimal master data models.
This Is Just the Beginning
The impacts of AI on the various disciplines of data management are nascent yet significant. Considering we’re only in the earliest stage of a progression from the augmentation of data management processes to the automation of them, there is still significant process to be made and value to be realized.
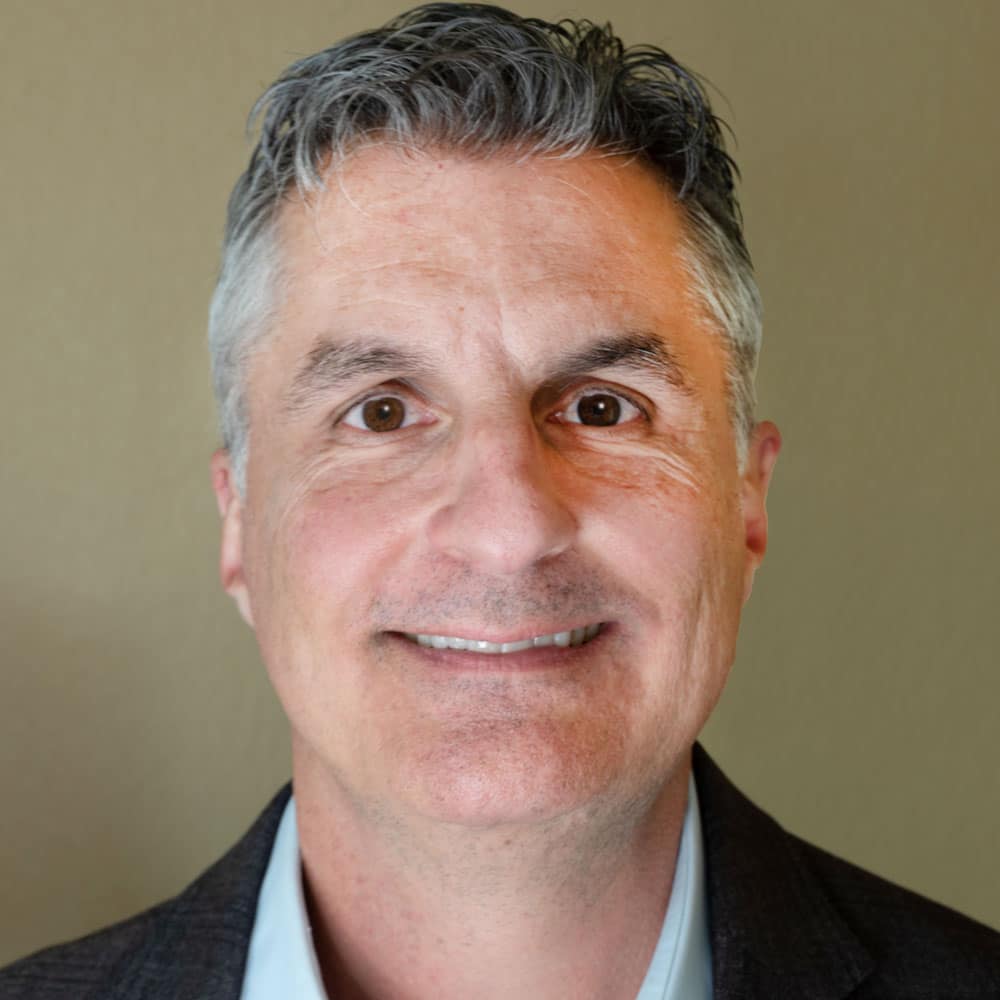
Malcolm Hawker
Malcolm Hawker is a former Gartner analyst and the Chief Data Officer at Profisee. Follow him on LinkedIn.