Episode Overview:
Looking back on 2024, what were some of the biggest, most notable events in the world of data and analytics?
Join the host of the CDO Matters Podcast, Malcolm Hawker, and he discussed the death of the data mesh, the growth of the fabric and AI, data products, and many other key data trends that were top of mind for many CDO’s over the past year.
Episode Links & Resources:
Good morning.
Good afternoon.
Good evening. Good whatever time it is, wherever you are. I’m Malcolm Hawker. I’m the host of the CDO Matters podcast.
Welcome to episode sixty six.
When you listen to this, if you are subscribed and you are getting updates, notifications of new podcasts, and you are listening to this as it is coming out of the podcast oven, if we stick to our schedule, then you’re probably consuming this either on December twenty sixth or maybe the week after. The week after Christmas or perhaps on the twenty sixth, which Canadians like me like to call Boxing Day.
Interesting history around Boxing Day. Happy Boxing Day, which is, by the way, for Canadians is their Black Friday.
So, you know, us Americans love to go shopping the day after Thanksgiving.
And in Canada, the Black Friday is really Boxing Day. It’s the day after Christmas. So Merry Christmas.
Happy New Year, to come.
I hope you if this is after Christmas, which it will be, I suspect, it’ll be after Christmas. I hope you had a wonderful Christmas holiday or whatever celebration brings you and your friends and family together.
Maybe that’s Hanukkah. Maybe that’s Kwanzaa. Maybe that’s something else. Whatever it is that brings you and your family and your friends all together to celebrate and to be in enjoy around each other, I hope you had a wonderful time. I hope you had a great Christmas.
I suspect I will have at this point. I’m not entirely sure where I’m gonna be at Christmas this year. My wife and I are still trying to figure it out. The time machine that is this podcast will, will obviously the truth will reveal itself, I think, probably Texas, maybe.
But I’m in Florida today recording this, and it’s Friday. So I’m in a good mood.
I’m always in a good mood on Friday afternoons. Today, we are going to talk about twenty twenty four, the year that has just passed.
I love doing these retrospectives. I also look look I I enjoy looking forward. And based on some of the traffic to the podcast, so do you. You enjoy the retrospectives, and you enjoy the looking forward and top trends and talking about those things because those are some of our most listened to and most downloaded podcasts. So, I’m excited to be talking about the year that was twenty twenty twenty four.
I hope you had a good year. I’ve had an amazing year. All of my years are amazing.
This one was particularly good for a few reasons.
A lot of professional development, a lot of travel. Oh my goodness.
I traveled so much.
I went to a lot of different places, got to talk with a lot of amazing data leaders, that are doing some great things in the space. So it’s been a great year for me, and I hope sincerely that it’s been a great year for you. What we’re gonna do on this podcast today is we’re gonna look back to my predictions from January of twenty twenty four, and see if they came true.
So I’m all about accountability. I’m all about a growth mindset as, as you will see in a book that I’m releasing next year through Wiley Publishing. I’m all about a growth mindset. And a growth mindset would say that you need to learn, and you need to seek feedback, and you need to do retrospectaries.
And what did you get right, and what did you get wrong, and how do you improve the next time around?
I don’t know because that was a lot of prognostication, but we’re gonna do a retrospective. So if you look back, episode forty two of the CDO Matters podcast, you can go back and see what I said. See what I had to say about what’s gonna happen in twenty twenty four. I’ll review that today.
But if you wanna hold my feet to the fire as it were, and check the things that I was saying, there is a record of everything that I said, and it was published in episode number forty two of CDO Matters.
So, that’s what the topic is gonna be today. We’re gonna look back in twenty twenty four. It’s ups, it’s downs, it’s sideways, it’s everything’s.
But we’re gonna start with, did I get it right?
Did I did my prognostications?
Did my, soothsaying, as it were, did my future telling, from January of twenty twenty four, did it come correct?
Let’s start on that, shall we, as we continue to digest our Christmas dinners.
Number one, I started really high level, talking about what’s gonna come. And I I in January twenty twenty four, I predicted a slow start to an economic recovery.
I think I largely got that wrong, but I’m not alone. So the good news is that a lot of smart people thought that the macro economy would start turning around a lot sooner than it actually did. A lot of people believe the US Federal Reserve would start lowering interest rates in the spring, and they didn’t. Because as it turns out, the inflation problem was, I think, much much deeper and much stronger. It had a stronger hold on the economy than a lot of people originally thought. And, many people still believe this to be true, by the way. Many people believe that inflation will be higher, for much more, for more prolonged periods of time than it has been in the past.
That remains to be seen. But I did predict that we would start to come out of kind of an economic stagnation in twenty twenty three and in twenty twenty four that we would kind of round the corner.
I don’t think that’s happened. In fact, it’s quite the opposite.
As, you know, interest rates remained high, economic activity remained relatively stagnant, I would say, through the year. What we ended up seeing was into the summer months.
I think a lot of those extremely restrictive financial policies and economic policies coming from Western governments around the world.
This isn’t just a US phenomenon. This is all kind of, you know, developed nations.
We’re we’re doing quantitative tightening. Right? We’re trying to take money out of these money supply because everybody injected tons of it post COVID or enduring COVID.
And there so there was a lot of belt tightening across, across the world. And that really, I think, started to take its toll towards the summer of this month.
So, you know, whether we’re talking about MDM software sales, data quality software sales, data management software sales, I think we saw a significant slowdown towards the middle of the year. Consulting services, I have a lot of dear friends of mine who are consultants, who make a living on consulting. I said that you were gonna have a good year this year, and I got it wrong.
By the summertime, I think things really, really started to slow down for a lot of my consultant friends and a lot of folks in the software business.
I’m very lucky that I work for a great company in Profisee, and we’re still growing. And we’re still we’re still growing very, you know, great numbers, but we slowed. So last year, we had around a forty percent year over year growth, and I think this year, we’ll be closer to around twenty, which is still phenomenal.
Phenomenal, especially in a year of slowdown when a lot of companies, struggled to have any sort of growth whatsoever.
So I’m very lucky to work for a company that has got a great product and a great team, where there’s great demand and we’ve got great partnerships with with Microsoft. But I would consider Profisee, in the MDM space and writ large the data management space to largely be kind of one of the outliers.
I would argue maybe the only space that we saw some some potentially strong growth in the data management world was in and around what we will call data governance solutions, a k a metadata management, a k a data catalogs.
There’s There’s a lot of blur there. Catalogs, metadata management, governance.
But I used all three of them because it’s like if you’re a CDO and you’re looking and you’re saying, okay.
Well, there’s this thing called a metadata management platform, and there’s this thing called a governance platform. There’s this thing called a data catalog. Well, you know, what gives? They’re the same. They’re all largely the same. They all kind of fall into the same genre. I would argue that maybe the one shining star of the data management software space this year is data catalogs, which I find interesting, because arguably arguably, data catalogs are one of the data management software solutions that are the most difficult to tie to tangible business value.
Data catalogs play a librarian role. They play an inventory management role as it were. Yes. Some have, you know, marketplace type capabilities where if you’re building data products, you can expose those in a catalog. So arguably, there’s a little bit of end user benefit there.
But when I was an analyst for three years, I did not hear a lot of CDOs saying, yeah, we implemented a catalog and now my customers are ecstatic.
Doesn’t happen. Right? Like a lot of people are saying, hey, we need to get data catalog so we can democratize access to data.
Yeah. Okay. Maybe.
Maybe, but not a ton of value there. Honestly, you know, I I just don’t hear people in procurement, supply chain, sales and marketing, finance saying, you know, if only I had a data catalog. Some of them may be saying I want better access to data. I get that. But we’re not talking about transformational value here, which is largely what a lot of companies expect their CDOs to deliver as transformational value. So there is a slight bit of irony in that, data catalogs. You know, I did did see a lot of growth of data catalogs this year, and that started in twenty twenty three.
I noted this last year when I was talking about kind of some of the things that I was seeing a lot of interesting conversations in twenty twenty three was, like, just this wellspring of data catalogs popping up everywhere. Half of the vendors at one event that I went to, the CDOIQ event in twenty twenty three, were data catalog vendors, most of which I’d never heard of.
You know, I think the market will kinda call through a lot of those. But still, data catalogs, one of the maybe outliers in the space that I would argue in a year economically from a software and consulting perspective has been not a great year. Not a great year. So I got that one wrong.
What else did I prognosticate?
Well, one thing that I said, which is a pretty bold prediction, I said that I think that I thought that in twenty twenty four, we would see a net decline in the total number of private sector CDOs for the first time.
So for years, the growth of the CDO has been going up up up up up up, and I said, hey.
I didn’t wanna say it, by the way. I this making this prediction did not bring me any joy.
But then I said that I thought that we would see a decline for the first year, and I think I was right.
There is no data necessary.
I couldn’t find any data that’s that separates public sector from private sector.
But Randy Bean, NuVantage partner, his annual CDO survey showed that, from twenty twenty three to twenty twenty four, there was a point six percent point six percent growth in the number of CDOs, which is basically flatline.
However, I know for a fact there is significant significant growth in CDO roles in the public sector, and I’ve I’ve seen this every year for the last three years going back to a federal mandate coming out of what was it? Twenty twenty two, That all departments all federal departments need to have a CDO. And a lot of those departments were still staffing up. We’re still hiring out, and they still are to this day.
So the the fact that I know the growth in the public sector is meteoric and the growth overall was totally flat.
That would suggest perhaps that I was right.
One other data point that the new vantage survey, found was that the number of companies reporting that the CDO role has totally failed went from two point seven percent to six point three percent. So three and a half percent of companies have said the CDO role publicly has failed. The role has failed. Did it’s failed.
They didn’t go so far to say those roles have gone away, but I suspect many of them have. So sadly, folks, I think I was right. At the very least, what we have maybe seen is that the CDO role has plateaued.
Around eighty two percent ish of companies reporting CDO and CDO equivalents. I think that was the last number.
I should know that one given my podcast is called CDO matters. But I think we’ve seen a bit of a plateau here. And a lot of that has to do with what I was talking about in January twenty twenty four at episode forty two was, frankly, a lot of c suite executives getting frustrated with a lack of delivery from CDOs. And I think this is something that will continue in twenty twenty five. We’ll talk about that a little bit more.
I do think that we’ll see CDOs losing more of AI functions to CFOs, believe it or not. Yes.
I said that CFO, and CTO, where people are, you know, where c suites are getting a little bit frustrated with a lack of traction in and around AI. But I think well, I’ll talk about that a little bit more in a couple weeks when I do a look forward in twenty twenty five, but I think that that will be something that we will see more of.
And I think that we will actually see, again, a decline of CDOs next year. I think we plateaued this year. I think we did.
And that’s, you know, good growth. We’re there.
I think you could say the CDO role is now a widely accepted, arguably increasingly mature role, but there are dark clouds in the horizon.
We’re gonna talk about those in a couple of weeks.
What else did I say? I’m looking at some notes here. So if I’m not looking straight at you, it’s because I’m looking at some notes. Forgive me. And people who are listening to this are like, what the heck is he talking about?
What else did I predict? Well, I predicted that the data mesh will evaporate.
Ding ding ding ding. I got it. I was correct.
Again, not one that I take a lot of glee in necessarily, because I know a lot of people who literally, pivoted their entire career towards the data mesh.
There are people who’ve literally pivoted their entire data career to the data mesh. So it gives me no glee to say that it has gone away, but it has most certainly gone away.
There are some things I have said consistently over the last two years when I have been publicly outspoken about the data mesh. There are some things that I like about the mesh. I actually do like decentralization. I like that notion.
I like domain centricity. I like giving our business customers flexibility around their analytical programs. Right. I like that.
However, it’s not an either or. You also need to manage cross domain uses of data and and you also must manage and govern and optimize analytical and and operational uses of data. This is one of the biggest weaknesses of the data mesh. I would argue one of the reasons why it has gone the way of the dodo, which is an extinct bird, by the way, because it didn’t support some of the operational uses. Beyond that, though, it’s entirely conceptual.
And I had yet to see anybody who could build a business case that could could, in dollars and cents, say with certainty or even even a reasonable amount of of like, give me seventy percent probability is fine. Give me seventy five percent. Who could say I’m seventy percent confident that the ROI of a data mesh will be net positive. I I mean, the idea that you could go from a centralized network to a fully, completely decentralized peer to peer network, that there wouldn’t be significant cost there and that the benefit of doing that that the benefit of doing that would significantly exceed the cost. Nobody could tell me that. Nobody could show me that. It was a thought exercise, a conceptual thought exercise where you’re betting the farm.
And fright guys, I know I know companies that got rid of their data warehouses because they went all in on it in mesh. I know companies that did this.
Like, holy cow. Like, we no no more centralized.
That’s an anti pattern according to the mesh.
Centralized data warehouse, anti pattern, gotta go. Like, wow.
I know a lot of companies have pivoted to this model, and I I know a lot of people that rushed to this from a career perspective, consulting perspective, built consulting practices around the data mesh.
Wow. But meanwhile, the underlying premise of the mesh is that a decentralized a decentralized approach to managing data and analytics.
Let’s just say analytics, a decentralized approach to managing, governing, optimizing the processes that companies use to drive analytical insights is inherently more cost effective and more user centric, than than any form of centralized pattern.
Right? Like, that’s the underlying assumption is that going to this decentralized pattern, you’ll have fantastic, excited users that are driving way more value for their organizations that are making better decisions and have better, more timely, accurate insights. And money will fall from the sky.
And, yay, that is the underlying premise of the mesh that is entirely and completely unproven, and it is why a lot of C suite executives it is partially why a lot of C suite executives are frustrated with CDOs because we keep chasing these unproven models, and we’re not building business cases.
We can’t say with any any sort of certainty, yes. This is gonna drive economic business value. We can’t do that.
And this is just another example of that, which leads me to number seven, the data fabric. I prognosticated the data fabric will rise in popularity and and become far more prominent.
And you should rightfully be saying right now, hey, Malcolm.
Isn’t this just another data mesh?
It might be. It it might be. I don’t think it is, but it might be.
You could rightfully be saying, hey. You know, isn’t this another situation where you’d be betting the farm on an unproven technology and an unproven operating model? Because that’s really what the data mesh was. It’s an entirely unproven operating model.
Aren’t you just doing that again? And aren’t you, Malcolm, the guy who is out there saying data fabric is gonna be transformational, and I am saying that?
Am I helping to drive us towards another cliff? A data mesh like cliff where there are people that are investing in the fabric, moving to the fabric, embedding the farm in the fabric.
Well, I hope I’m not, and I hope that you approach things with caution, but there are certainly things to suggest that the data fabric is different, especially because starting on a data fabric, like like starting on it, implementing some maybe some technology like Microsoft. I’m not here to shield Microsoft per se, but implementing their v one fabric, and I would call it a v one.
There’s value there. I absolutely see the value there. And I I see the value of having a single operating plane, a single UI for a data person to do data engineering, to do their ETL work, to do their analytics work, to do transformations, to do the data quality stuff, to do MDM stuff, all one place.
That includes data scientists, by the way.
So having one place to do all of that work in a place where you could have arguably all of the data governance rules visible, you know, transparent, managed from maybe within a single plane where you don’t have to do one off ETL jobs. You don’t have to do one off pipeline jobs. You don’t have to have MDM sitting over here completely separate from everything else and your data quality hub sitting over here completely separate from everything else. Having one place to do all of that vis a vis fabric, that I get.
That I see some value in, and it doesn’t require you to throw out your data warehouse, by the way. And it doesn’t require you to do anything differently necessarily, by the way, because you’re already doing all this stuff. You’re building your pipelines. You’re building master data records. You’re doing data quality. You’re doing all this stuff today, but bringing that under one envelope, what Gartner would call a data and analytics governance platform.
I people are calling it a fabric. You could call it a governance platform. You could call it something.
But this one place to do all of that, that makes sense to me.
There because there are economies of scale here that will be exposed in taking that approach. Now people could be saying, well, that is entirely centralized, and centralization is bad.
No. It’s not.
No. It is not. Hubs and spokes exist for a reason. Talk to a network architect.
Right? Physical layer one architect that will tell you there are economies of scale in networks from having hubs.
Talk to United Airlines.
Talk to any other highly distributed network architecture architect, and they’ll tell you that there are economies of scale to be gleaned by having hubs.
I do think somewhere down the road, maybe blockchain will allow us to do both.
Right? Have a trustless entirely decentralized point to point network where you could have an overlay of some idea of a governance and data management function, right, that allows decentralization, but with oversight somehow, someway. Maybe blockchain can do that. I think it can.
But this is a long winded way of saying, I think the data fabric is here to stay.
I said that last year, and I will say it again this year, and I think that I’ve largely been proven right.
Argue with me if you would like in the comments below.
Okay. What else did I say?
I said that AI readiness would be the topic that you would hear from consultants in the year.
If you were a consultant, pitching a you know, your services at an industry event in twenty twenty four, I would I was saying you were gonna be talking mostly about AI readiness. I think I’m right.
I’m mostly right. I would argue AI readiness kind of pivoted towards the end of twenty twenty four, because we finally two years later after the launch of Chat g p two, we finally started to figure some stuff out, stuff that I’ve been talking about for two years. But we as an industry finally started to figure some stuff out. I’ll talk about that in a bit.
But AI readiness was the topic at the beginning of the year.
I would argue one of the topics, especially if you’re more of a consultant. Right? But vendors are saying this as well. Profisee, we were talking about it.
Data quality vendors are talking about it. All loosely tied to this idea of get your foundation straight. Right? And if you’re putting in garbage data into, an AI based solution, well, then what what, you know, what are you gonna get out the other end?
Kind of, like, around that very simplistic deterministic view of the world of, you know, how can you trust your AI if you can’t trust your data, which is a very simplistic, honestly, not really actionable way of looking at things.
The story that I tell is, you know, if you’re out there as a vendor, software consulting, whatever, and you’re still out there saying, you know, how can you trust if you’ve got if you don’t trust your data, how are you gonna trust your AI?
If you’re still saying that in twenty twenty five, I think we’re now to the point where people are like, okay.
As a buzzword, as a buzz phrase, as a platitude, because that’s what it is. It’s a platitude.
I get it.
And you can’t argue it. That’s one of the inherent qualities of a platitude. It just makes abundant sense. You can’t argue it.
It’s what platitudes are.
As a platitude, it’s fine. The idea of you can’t trust your your data, then, you know, how do you trust your AI?
Fine.
But I need a little more meat on that bone.
If I’m a CDO and I’m investing, I’m investing in software, I’m investing in consulting services, I’m investing in people, we’re gonna need to take it a a couple layers deeper than just that. For two years, that level of platitudinous nessness, the the the platitude level was enough.
I would argue it it sent many of us, many, far too many of us on a path that said it put many of us on a path that that actually didn’t help at all. Didn’t help at all because many of us heard that.
Many of us heard, oh, well, if you can’t trust your data, you gotta double down your foundations.
Gotta double down your foundation. Data quality, MDM, you gotta double down to to to all that stuff. Your data governance program, if you don’t have one, you need one. Absolutely, you need one, and you need to double down, triple down, quadruple down in your foundations because that’s the only way you’re gonna get any money or value or benefit out of AI. And if you try to do AI without the foundations, well, then you’re gonna be the laughing stock of the CDL community.
We got away for two years of saying that stuff when largely, I would argue, not valuable, not insightful, not actionable, and largely untrue at a detailed level.
What do I mean by that? Well, GenAI based solutions are built on and optimized by structured data.
I have been saying this over and over and over and over and over and over, and I will continue to say it. Unstructured data, AKA text, Word docs, PDFs sitting on SharePoint servers. Unstructured data is how these Gen AI based solutions are built and optimized.
I don’t know a ton of governance programs that at least ones that are kind of struggling, the governance programs that you could argue need to be buttressed.
They need to be improved. I don’t know many of those that have a deep and robust focus on unstructured data.
That many. I talked to literally hundreds and hundreds of CDOs when I was a Gartner analyst.
Unstructured data is largely the kind of it’s it’s it’s out there.
It’s it’s out there. Right? It’s not even in the scope of my governance program.
If it is, maybe I’ve got some loose access controls around vis a vis Microsoft or or so other other providers like a Microsoft where I’ve got some basic access access controls, access controls on docs.
But do I know what’s in those docs?
Like, you know, Word docs, PDFs, HTML files. Do I know what’s in them? Do I know what they say? Do I know if they’re accurate? Do I know when they were last updated?
Do I know if they conform to any sort of governance? No. No. No. No. I don’t.
That’s kind of where we are. So my point here, guys, is that many of us were told, go double down in your foundations.
Hard to argue. Who’s gonna argue that eating better isn’t a good thing? Who’s gonna argue that getting more exercise isn’t a good thing? Who’s gonna argue that doubling down on your data management, data governance foundations isn’t a good thing?
Nobody’s gonna argue that.
But when it comes to GenAI, doubling down on the status quo ain’t gonna move the needle.
Not moving the needle.
It’s not.
Long winded way of saying what people started to figure out by the middle of the year, by the middle of twenty twenty four was that the platitudes aren’t helping and AI ready.
The idea of being AI ready and the idea of doubling down on foundations, well, okay.
I get it, but I’m going to need to start focusing a little bit more on some areas that I’ve never focused on before.
And that’s kinda scary, and I really don’t know what to do. What does that mean? What does it actually mean to start focusing on structured data? Unstructured data. So we’re gonna be talking a lot about that in twenty twenty five. I’m here to help.
Starts with things like tagging data, right, profiling, discovering all this data, what’s out there. My goodness.
So AI readiness. I said that it would be the the topic of conversation or one of the topics of conversation in twenty twenty four. It certainly was, but the conversation started to pivot towards the middle of the year as we slowly figured out that being Gen AI ready didn’t necessarily mean I need to go buy a data catalog, and it didn’t necessarily mean I need to go, you know, double my data governance staff.
What it actually really, really means is that we need to manage our businesses differently, folks. We need to manage our businesses differently, and we need to figure this stuff out quickly quickly quickly.
What else did I say? I said in twenty twenty four that the topic of AI governance would be front of mind for everybody. Bing, bing, bing. Got that one right as well. So this does kind of dovetail off the idea of data AI readiness. These things are intrinsically intertwined.
But we’ve got a lot of work to do here.
We have got a lot of work to do here. I have been to, I don’t know, twenty conferences this year or conference and conference like.
Round tables, brown bags, smaller conferences, user meetings, twenty easily.
Easily.
The topic of AI governance is at the top of the list and was at the top of the list and will continue to be at the top of the list at many of those.
But what I saw, again, what I heard, and I think we will continue sadly, we will continue to hear in twenty twenty five, is a lot of focus on platitudes.
You need to focus on AI governance.
I’m a CDO. What does that mean?
What does that mean?
There are an increasing number of frameworks.
Okay. Helpful. But how do I operationalize, though, those?
How do I actually implement an AI ethics framework?
What does that actually even mean?
What is ethical AI?
There are people who are talking about it at a diff kind of at a definitional level, but I don’t see enough of us.
And what I mean by us is thought leaders, consultants, you name it, others who are here to help and to teach and to guide. I don’t see a lot of us talking about how to operationalize AI governance.
I hear a lot of us talking about and saying it’s important. I hear a lot of us talking about how there’s a lot of regulations we need to be aware of. I’ve I’ve been to session after session where people put up the slide, and it’s like this shock and awe of, like, this long list of post and or existing regulation around AI where people are saying, look at all this. Isn’t this scary? It is daunting. Yeah. It sure is.
But what do I do? Like, how do I okay. Thanks for the framework. That’s that’s great.
But how do how do I build an AI model? Or maybe I’m not even building models. Maybe I’m just trying to build some chatbots. What does it actually mean to have an ethical chatbot?
Like, wait. How do I operationalize this? How do I measure this?
We need to do more of that. We need to do more of that. These are difficult problems to solve, and frankly, I don’t think we know the answers is part of the problem.
I I I don’t think we know the answers because, you know, like, how do you codify ethics? How do you turn that into a mathematical equation? Right? How do you turn that into a set of conditions?
I I honestly don’t know.
I don’t. I need to learn. Maybe somebody’s figured this out.
But if we don’t know, we just need to say that.
We need to say more of that.
But I I just need individually.
I desperately need to learn more about how to operationalize these processes and these policies because I know what it takes to define a governance framework and implement a governance framework when we’re talking about the same old same old. But when we’re talking about these technologies, when we’re talking about uses of data in ways that, honestly, folks, we don’t know how they work.
The transformer technologies, we don’t know how they work.
Fact.
Sam Altman will tell you he doesn’t know how ChatGPT is able to code.
I’ve talked about this in other podcasts, but think about this.
Computer languages are never part of the training except for ChatGPT. Never. Sam Altman will tell you that they weren’t, but they can code.
They can they can write COBOL. How?
They don’t know how. So if we’re dealing with a technology, we fundamentally don’t know how it works. And maybe maybe we will figure it out.
But how in the world do we write rules to control, and I hate to use that word, but in the case of AI, I think it’s an appropriate one, to control the behavior of a system that we fundamentally don’t know how it works and can’t necessarily even predict how it works.
If we don’t know, and I don’t think we do, but if we don’t know, let’s just say that instead of instead of telling all of our CDO customers and CIO customers and VPs of data and analytics, instead of telling them, go do AI ethics.
Go do it as a part of an AI governance program. But if we don’t really know how to make that real, we should be saying that.
Or maybe you do, and maybe you’re listening to this podcast and you’re screaming at your your computer screen or you’re yelling at me through your earbuds.
Of course, you you you you dummy.
We’ve got this figured out.
If you have, let me know.
DM me, send me an email, hit me on LinkedIn.
I’m I’m out there. You can. Because I need to learn. Because I need to learn because I don’t I I’m not seeing it.
I’m not seeing it. It’s not even just an ethical issue, by the way. It’s it’s it’s also it’s it’s a data curation and data management issue. Like, how how do we how do we enforce ethical concerns for lack of which is this is a bit of a catchall. Right? This this could even get into things like sustainability and ethics and stereotypes and bias.
How do how do we control these things? How do we put in rules to ensure the data we are using, the data is unbiased when it inherently is because we created it.
So these are these are really big questions, and I don’t think we have any idea what the answers are.
But we’re out there telling people to go do it and how important it is and how critical it is. And, oh, and by the way, here’s all these regulations that are gonna make you do it.
Huge opportunity here in twenty twenty five to go from platitudes to here’s how you actually put a shovel in the ground, I would invite anybody who is a thought leader in data governance, who’s a thought leader in AI, who is a thought leader in data management and anything else who knows a little bit about these things. I would invite you and all of us to find a way to make more actionable content and insights for CDOs in twenty twenty five to go from platitude to okay.
We are implementing an AI governance program that we believe is fundamentally different and is tailored for this very unique and transformational technology.
Do that, and you will be, on my Christmas card list for twenty twenty six, or you’ll be added to my favorite person list. I don’t know. If you do that, you’re gonna be a star because there’s a there I can tell you there’s a thirst for that content out there because I just don’t see it. I just see a lot of AI governance is important, but a lot of people just not even not not providing a ton of insight of actually how to make that happen.
So AI governance will continue to be front of mind in in next year, but I’m gonna I’m getting ahead of myself.
What else did I say? I said sustainability is gonna gain some steam. Boy, I was wrong there. I’ve been saying sustainability will gain steam every year for the last three years. Maybe this is wishful thinking, but I’m not hearing a ton of it.
Just not hearing a ton of it. I firmly believe that CDOs will be given sustainability targets at some point at some point. In the short term, I suspect most will just buy their way out, which is what most companies are doing today. They are buying carbon credits and saying they are carbon neutral.
When at the same time, they’re spewing a lot of carbon into the sky.
That’s only getting worse because of our focus on AI.
So maybe again, probably gonna make my list for twenty twenty five.
Then maybe it’s gonna be wishful thinking one more year, but it’s it’s we we we we we gotta figure it out.
Like, we just gotta stop hoarding data. We we’ve got we’ve gotta figure out folks.
Just just if we’re not using it, do we really need it?
If just sitting there collecting digital dust, do we really, really need it? This does start to get into kind of some basic blocking and tackling around managing a data function and and how do we make decisions around what we store and what we don’t store.
I know for a fact. I’ve been in that position. I know for a fact there’s not a ton of rigor there and generally it’s FOMO and it’s I’ll store it just because I may need it one day, which pretty much describes half of what you would find in my garage.
So I get it, but we we we we we can do better.
We can do better. And, just because you might need it one day doesn’t cut it in a world of rapidly depleting energy resources, at a time when we need them more than ever before.
So, Andrew, Anton, sustainability.
I said in episode forty two in January twenty twenty four that data literacy will lose some steam.
I got that wrong.
I definitely got that wrong.
Data literacy is, apparently, you know, taking deep hold within the organization or within within most organizations or many, and certainly in the world of data and analytics. And y’all should know my perspective on data literacy by now, but I do believe that data literacy is in very many ways, representative of a negative mindset because because the underlying premise of a data literacy program is one that says the reason why companies are not getting value from data and analytics is because people consuming data and analytics lack skill.
They may lack skill, but I can tell you with one hundred percent certainty that is not the number one reason why data functions are struggling to show their value. I know that with one hundred percent conviction.
We need to do a better job of building products that people want and people get value from and that people want to use and find easy to use.
We need to do a better job at building great and amazing data products. If we do that, if we are focused on customer success, if we are focused on relentlessly focused on driving value for our customers, if we are focused on ease of use, if we are focused on building, in essence, the next iPhone for our customers, then you could argue that training may be needed, but it is a secondary concern at best.
Right?
That if you build a great product that people wanna use and they find value from and is easy to use and is well designed, will you still need to train on it?
Maybe.
Maybe. But it’s you don’t lead with it. You don’t start with it. You don’t say, okay, I’m not even going to focus on the product stuff. I’m not even going to focus on all that other stuff. I’m just going to go and dove straight into a literacy program because our users lack skill.
That is the wrong approach. That is the wrong approach. So my hope really, this was an optimistic thing, not because I don’t wanna see the demise of data literacy. I know a lot of people who make their living on this, including a friend of mine, Jordan Morrow, who’s been incredibly supportive to me, incredibly supportive to this channel.
I don’t want to see them lose their incomes or their vocations focused on data literacy because there’s training is required. Training is necessary. Training is needed.
But what I would like to see is more of a holistic approach to building great products, to solving customer needs, to being rapidly focused on customer needs instead of saying they are the problem. Because trust me, folks, they’re not the problem.
My last or I had two more predictions.
My second to last prediction was that I we were gonna see more and more people come into the space. That is absolutely true. Every time I go to Dataverse events thank you, Tony Shaw, for having me.
Oh, and by the way, by the time you see this late, late December, I would have given a keynote speech the second week of December in DC at the DGI Q East event. Every time I go to a diversity event, Tony always asks who’s new and half the room, more than half of the room put up their hands.
And I I think that will continue to be true.
This is a good thing for data and analytics because our space is growing.
Our companies do get it. Our c suites get it.
They know the data is the new oil, and it’s also the new refinery, by the way. It’s both, and our companies get it.
So the opportunity is ours to seize on that, to seize on the zeitgeist of data being important because it is. We all know it is.
We all know it is.
So the opportunity is is there for us to take that take that feeling, that spirit, that truth of data being important and find ways to deliver incredible value from it. So that’s why people continue to flood into this space. So I was right about that, and I think I’m gonna that’ll be another prognosis case for next year. Lastly well, last was a bit of a bonus prediction.
It was a little bit of a bonus prediction. I was talking about AGI and the combination of artificial generalized intelligence. This is, by the way, the point when AI is able to solve problems that were never part of the training set.
Meaning, I’m solving a novel problem in a novel way or maybe it’s an old problem, but it’s a novel way. It’s it’s I’m going beyond the training set to develop solve a problem in a way that is entirely and completely novel that we’ve never done before.
That’s AGI.
My prognostication was that in combination with quantum computing, could take us to a place where encryption becomes irrelevant.
Turns out, I think I’m prob based on everything I saw on the news, I’m probably about ten years ahead of my time on this one because Sam Altman says that he needs needs seven trillion dollars worth of chips in order to build AGI. I take him at his word. And he also needs, more electrical power than we could ever even imagine, and I take him at his word for that. And the fact that Microsoft and Amazon are buying and resuscitating old nuclear plants suggests that for the time being for the time being, a lack of access to energy will be the the gate the the biggest gating factor in operationalizing AGI at scale, which frankly folks is a really good thing, because I don’t want encryption to break and I don’t want the machines to be smarter than me. I want us to be the smartest things on the planet for the time being, at least for the time that I’m on it.
So those are my prognostications for twenty twenty four. I think I was at about a seventy percent ish hit rate, give or take. What else did we see in twenty twenty four that that in my last few minutes that we didn’t actually predict. What are some of the things that we saw? Well, I’ve already mentioned some of these. I we saw kind of a negative and less less than stellar financial performance, at at a at a macro level, inflation lasting longer, lackluster performance in some of the markets that are that are near and dear to my heart, particularly software and and and consulting. We certainly saw that.
I mean, twenty twenty four was was an interesting year in that I I really kind of feel like we’re in a time of transition.
You know? And and I think that we’re still kind of catching up to the the post pandemic.
But I’m optimistic that twenty twenty five, we will go from this kind of frozen state and this kind of this arguably, this transitional state out of a time of turmoil, twenty twenty two, turmoil, uncertainty.
I think times leading up to the US election, there was a lot of uncertainty with that, kind of turmoil around economic and inflation. And it was just really it was causing a lot of uncertainty.
And and for most, whether you’re talking a company or whether you’re talking a financial market, uncertainty is the is the kind of the the case of death when it comes to growth and it’s the case of death when it comes to investment and and and making bold moves. Uncertainty just kinda has this freezing effect as it were on markets. And I think that’s what we saw.
I think that was what we saw in twenty twenty four was was was kind of a lot of this stuck.
You know, AI, of course, is still the topic of discussion.
It is still the bell of the ball. It is still something we’re gonna see folks focus on. So, you know, I think it would be a yes. We saw a lot of that in twenty twenty four, but of course we did. I mean, that was I think that was well expected and I think we’re gonna continue to see that. I think we’re gonna continue to see more discussion around the growth of, you know, AI as a as a lever to automate and augment data management processes. Again, I think that’s like a of course, we will.
So that is twenty twenty four. That is twenty twenty four. It it has been a good year. It has been an interesting year for many. It’s been a challenging year.
I hope you had a good twenty twenty four. I’m committed to helping you, data leaders, CDOs, VPs of data and analytics, data governors, data stewards, data analysts, data engineers. It’s my job to make your job easier.
If there are topics you would like me to discuss, if there are issues that you want me to bring to the front of mind for listeners or viewers of this podcast, please connect with me on LinkedIn. Please give me some feedback. I want to make this content better. I want to make this content more insightful. My whole rant about the whole AI governance thing, I hope you can see that I come at this sincerely.
We can do better in a lot of key areas. I think AI governance, it most certainly is one of them, and I’m committed to doing that. So if you’ve got suggestions, if you’ve got feedback, if you’ve got a complaint, connect with me on LinkedIn, please, and send me a message and say, hey, would really love to see more of x or may have more of y in twenty twenty five and I will most certainly take that to heart.
Happy New Year.
It should be right around the corner. I hope you have a wonderful New Year. I hope you’re having a wonderful day, a wonderful holiday season. Thank you for listening. Thank you for subscribing. Thank you for supporting. Thank you for downloading, liking, subscribing.
All of it, I live an incredibly charmed life and it’s because people support my content and listen to my content. I’m thrilled that you’re here, and I hope to see you again in twenty twenty five. Happy new year, everybody. We’ll see you next year. Bye for now.
ABOUT THE SHOW
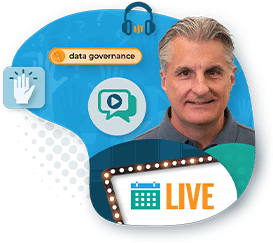