Episode Overview:
Are you looking to learn more about Knowledge Graphs, and the role they will increasingly play in a modern data ecosystem? If yes, then you need to check out the latest episode of the CDO Matters Podcast. Sumit Pal, ex Gartner analyst and Strategic Technology Director at Ontotext, shines a light on the world of knowledge graphs, and the important ways they differ from, and complement, more traditional data analysis and persistence methods. If you’re a data leader and you’re not yet embracing knowledge graphs, then this episode is for you!
Episode Links & Resources:
Good morning. Good afternoon. Good evening. Good whatever time it is, wherever you are in this amazing world of ours.
I’m Malcolm Hawker. I’m the host of the CDO Matters podcast. Welcome to episode what episode are we on now? We’re this is number sixty five.
And today, we are gonna be talking about knowledge graphs, the magic of knowledge graphs. We are going to demystify knowledge graphs. If you are active in, you know, data and analytics conferences, if you are on LinkedIn, if you’re talking to other data professionals, chances are pretty good. You’ve heard about knowledge graphs.
Maybe you’re not using them. Maybe you are. Maybe you’ve been using them for a long time. I still hope there’s gonna be ton of value in this conversation because we’re gonna talk about how knowledge graphs are being used today and how they are going to help operationalize AI at scale within your organization.
So with that, I’m thrilled to be joined by my fellow ex Gartner, employee, Sumit Powell. Sumit, how are you today?
Hi. I’m doing very very well. Thank you, Malcolm. Thank you for inviting me.
Awesome. So Sumit and I worked at Gartner together.
We were in slightly different groups. Sumit was in the, GTP, Gartner for technical professionals, and I was in IT leaders group, but we were both talking about data and analytics all day, every day, data management, data governance, fun stuff.
And everybody that I’ve ever worked with at Gartner, I I they’re always really incredibly smart people, and they have lots to share. So, hopefully, that isn’t me being too self aggrandizing, but I I assume it when he was at Gartner as as a wise and knowledgeable person. So I I’m I’m I’m happy that you are here hap having this conversation. I think you are, I think, the second ex Gartner guest I’ve had. So I had Doug Laney.
Oh, I see.
Yeah. And he’s ex Gartner.
Yeah.
And if this if I’m forgetting anybody, boy, I’m gonna look really silly.
I’m looking at my list of guest speakers, but there are people I need to have, for sure, from the ex Gartner community. No. No. Sanjeev. Of course.
Sanjeev. Must must be Sanjeev. Yeah.
I I almost forgot Sanjeev.
If he’s listening to this, he’s like he was probably screaming at his computers like, beep beep beep beep beep.
Anyway, Sumit, let’s let’s talk about knowledge graphs. Now let’s assume that I and Sumit, by the way, is with knowledge graph company OntoText. I’ve had a long relationship with folks, at OntoText, And, I thank them for giving you an hour of our time today. Let’s let’s talk let’s assume that I don’t really know much of anything.
Right? And that maybe I’m new to knowledge graphs. Maybe I am a business centric CDO who’s come from the business side, and now I’m hearing all this stuff about knowledge graphs. Sumit, how do you how do you describe knowledge graphs, what they are, the value they provide, and why should me as a CDO care about this?
Yeah. That’s a pretty overloaded question, Malcolm, but let me break it down. Right? Let’s first understand, what is a knowledge graph. But even, before we try to answer the question, what is a knowledge graph, let’s understand what is a graph. Right?
A graph structured database or a graph structured data is sort of ubiquitous for most of us across most in most domains. We use graphs every day. We just don’t realize it. And graphs are everywhere.
The moment we use any social media, social media is all about connections. Right? How one thing is connected, one entity is connected to the other. When you use GPS, under the cover, graph data structures or graphs are being used to take you from point a to b to figure out the route, the route.
When we are using cell phones, for example, we as we move, as the cell phone is moving, we are driving and we are having a cell phone, the handover happens across different cell phone towers. That uses craft technology.
Supply chain, transportation, the whole Internet, if you think about it, is a graph of connected web pages. Right? And one of the things I would I’ll highly recommend all of you to look at is Netflix has a very good documentary called Connected, which shows that we live in a world which is so well connected. Right? Now knowledge graphs are one layer built on top of these graphs. Right? They are they are like graph plus plus you can think of, where they include what is called as a knowledge model that adds lot more semantics, descriptions of the things that is being connected, and the semantic metadata around it.
Now with knowledge graphs, the good aspect of knowledge of the good aspect of knowledge graphs is the data and the metadata are together.
That is the very good aspect of you don’t have to let’s say you have your instance data in one data store. You have your metadata in another. You have to go and query it and and then sort of link it together. But with knowledge graphs, the date instance data and the model, the instance data and the metadata, which is the model, can be all together.
And that gives lot of power in terms of bringing your schema, the different terminologies associated with your domain, which could be in terms of very domain specific taxonomies, ontologies, vocabularies.
And this sort of a structured representation of knowledge in a knowledge graph gives you power in terms of reasoning and inferencing where you can deduce newer facts.
For example, if I say Socrates is a man, all men are mortal.
We as human beings can quickly decipher that Socrates is mortal, but a machine may not be able to do that. But when you represent this knowledge graph as a graph, when you represent these two statements as a graph where you can say Socrates is one entity, is a man, is an end is a man is another entity, is a is a relationship, these kind of inferencing becomes much more easier.
Now knowledge graphs also help you to organize and connect data semantically from different data sources. Right?
Where we know the whole data integration space is a huge, huge space. So many vendors, so many solutions.
But knowledge graphs, one thing we always miss in the data integration is the semantic aspects. Where is the semantics?
Right? What are you connecting? Why are you connect it’s sort of embedded in the scripts or the ETL code that we write. Right?
Whereas in knowledge graph, it can be much more implicit through metadata specification when you bring in these ontologies.
Let me explain it to you a little bit more. Let’s say, and you can actually go to our website and take a look at some of our documents or some of our blogs where let’s say there is a ecommerce site that’s selling shoes. Right? And you have different types of shoes.
Shoes could be celebrity type of shoes. Shoes could be running shoes, party shoes, sneakers. Right? And they have different colors.
That’s your instance data.
Now if you overlay if you bring in the shoe ontology, which describes the shoes, A shoe can be like a boot, a sports shoe. Boots can be high boots. They can be different other types of boots.
Sport shoes could be sneakers, running shoes, these kind of things. When you bring in that and then you bring in what is called the color taxonomy, where you say color x is a warm color, a cold color, and then you overlay that on top of your instance data, suddenly your data becomes very enriched.
And now if you want if you are you if you are this structure is powering your website and someone comes and searches for one colored shoe because it’s all linked together as a graph, you can do graph traversals to quickly get your answers.
What is a one type of issue? You don’t have to write ETL code to say only these colors are subsets of warm colored shoes, and only if this happens, you show the results. Otherwise, you don’t show. Right?
So with knowledge graphs, we can think of knowledge graphs as concepts that that whole conceptual aspect becomes very useful. Right?
We, again, everyday use knowledge graphs we don’t realize. For example, when you are doing and when any of us do Google search or Bing search, right, we use knowledge graphs. Let me give you another example. Let’s say you search for Albert Einstein. Right? Google will give you a list of results. But on the right hand side, you will see more information about him.
Where was he born? Where is that place located? It’s in which country, which city, which county, where was he educated?
Right? Which is a it’s a university, a college.
Those we are moving away from this whole idea about string based searching to more conceptual or semantic searching. And this is where knowledge graphs come in.
Link LinkedIn, for example, we use LinkedIn all the time. LinkedIn powers its skill graph with the knowledge graph where different skills are sort of, you know, available as ontologies or taxonomies.
Hope that helps, Malcolm to Yeah.
Well, so, like, a a lot a lot to chew on there.
I’ll I’ll try to unpack a a a few core concepts, but what I what I heard you say is that it is it is both a way of storing data. So graph is a way to persist data. Correct?
Correct.
Okay. It’s a it’s a way to persist data, but it’s also, to a certain degree, a way of analyzing data and allowing you to see data in a different way. For example, you know, historical SQL databases, rows and columns, you would have a customer table that was joined to a product table. You could infer that customers and products are somehow related, but that’s about as far as you can take it. You wouldn’t be able to understand additional metadata or additional insights that would even take it farther than that secondary relationship, third degree.
Person is related to product, which is related to location, which is located to transaction.
All these things can be tied together in a graph. Let me ask you another maybe maybe a kind of a stupid question. You know, I’ve I’ve got a very basic data estate today.
We haven’t we haven’t actually really implemented knowledge graphs because we haven’t thought that we really needed them. Do they replace my my my SQL estate or do they sit on top of it? What how like, as as an investment, how does this typically work?
Yeah. Not at all. Not at all. They are not a replacement of your operational data stores. Right?
Even they are not a replacement of your analytical data stores where we are doing all app type queries, right, where you are doing aggregations, pivoting, drill downs, drill across, those type of things. Right? Graphs are more meant to complement your existing existing structures with the capability of better data management in terms of data integration.
Knowledge graphs help you to do a lot of good things around data quality, where as you ingest data, you can have rules specified which can quickly detect bad data coming in and prevent it from getting into the knowledge graph. Right? So, yes, when I was at Gartner in twenty nineteen, I first published the first paper on graphs in Gartner. And, after that, this was the most, most asked question for me after a lot of our of Gartner clients read it. Are they replacing knowledge are they replacing relational databases? Right?
Yeah. Alright. I didn’t ask a dumb question then. That’s good.
Not at all.
That that that’s good. So I think the number one thing that I’m hearing over and over and over again is what well, a couple of things. One is I’m hearing context.
And and absolutely critical, and we’re gonna talk about context a little bit more within the context of of of AI.
But another thing that I’m hearing you say is, well, which is and and I will just use the phrase unknown knowns.
Right? We know there are complex relationships that probably exist within our across our customers or our products or our suppliers or our whatever.
Right? There are complex relationships in everything that we do, but we just may not know what they are. Right? Or we have a hard time inferring that there’s a relationship between this payer and this provider or this location or this treatment plan. We’d have to we’d have a hard time inferring those with other graph, but what a graph can provide you is insight on what those unknown knowns are. Is that a reasonable way of looking at things?
That’s definitely one of the ways of looking at it at it, Malcolm, and you got it. If you look at the the the financial institutions, right, where they are struggling with frauds, right, fraud detection, fraud mitigation, and understanding discovery fraud, discovering fraud. Now the the previously, a lot of the fraud based, identification, fraud identification was happening through plain machine learning, right, where you took your transactional data, where you had merchants, you had different transactions, different customers. Right? Took the transactional data, did some feature engineering.
Right? And then try to figure out anomalies, outliers, or figure out frauds.
Now what happens is we know that we are always few steps behind the fraudsters. Right? Fraudsters are way too smart. Right?
What the financial institutions or the financial industry discovered was, as you said, unknown unknowns. Right? When you bring in when you model all these transactions, take a merchant as, as a node, you, me, others who are customers of that merchant or customers of a particular credit card company or a bank are another node. And we as we make transactions, these are the links that connect us to a merchant to a transaction. Right?
Now fraudsters typically work as cohorts. Right? They create bots. They create fake accounts. They do some minor small transactions to make sure that things are working before they, you know, before they do bigger transactional, frauds.
Right? Once you overlay a graph based approach of these transactions, you get lot more insights. That’s why you will see lot of the anti money laundering related use cases, fraud detection use cases, use graphs because they help you get another dimension to what is happening in your operational data. So you are spot on, Malcolm.
So I just heard a I just heard a third thing. So we had talked earlier about graph as a as a means of persistence. We talked about graph as a way to help analyze.
And what I just heard you say is a graph as a way to visualize as well. Because you can you can export and visualize things in in a way in a graphical way that to our eye, you could show some of these ontologies or some of these relationships via these nodes in ways that could just kinda to our eye make a lot of sense. Is that correct?
Exactly. Exactly.
And that that’s that’s right. The visualization aspect. But as datasets become bigger, right, visualization becomes much more difficult. Then you need to drill down into particular areas which are more suspect or these kind of things. Right? So you have to do subgraph visualization to get a picture to get to get the picture of, you know, where you think frauds could be happening, things like that.
Right? Yeah. So I I love the fact I mean, thank you, by the way, for focusing on actual, like, business use cases. And and and, you know, we talked about fraud and e commerce, and there’s this whole I I would throw out kind of, like, health care and patient and provider and location and treatment plan.
I love all of that stuff. We could also talk about, kind of, data management use cases. You talked about data quality. I suspect it would be relatively easy, I’m air quoting here, to see, like, anomalies in in a in a in a graph, like, where an anomaly would pop up where you’ve got these relationships where there shouldn’t be a relationship.
So not only is this something that’s gonna help, you know, your business run better or tell you things about your business you may not have otherwise known, but the same it sounds like the same is true, from the data management perspective, and these these can be tools that can be deployed internally facing to understand the the the state of your data or who’s accessing the data and under what condition. Yeah?
Exactly. Exactly. And that’s the reason why when especially if you know, like, there are two, basically, two different graph models. Right?
One is called LPG. It’s called the labeled property graph. That’s one way of modeling graphs. The other is called the RDF, which is where onto text comes from.
Right? Resource description framework. Now resource description framework has a lot of standards behind it. It’s built on very strong mathematical foundations on in terms of semantics.
It derives most of its standards from Web three standards from right? This was originally the concept conception that doctor Tim Berners Lee, the inventor of the inter Internet. Right? He had in mind to build a semantic web, right, where it’s not just about strings anymore, but it’s more about the concepts and how they are interlinked.
Right? When we talk about ontologies and taxonomies, taxonomies taxonomy is sort of a hierarchical classification. Right? And you can think of it as a graph.
Right? Same with ontology. Ontologies are also modeled as as graphs. So all of them fit together.
Now with an RDF based graph model, which is what OntoText has, there we have what is called as a reasoning engine, the inferencing part that I was talking about when I gave the example of Socrates as a man, all men are mortal, and and right?
What happens is when you bring in data to a knowledge graph, into the graph, this kind of inferencing kicks in to ensure there is no inconsistent data that is coming in.
That’s why also the other thing with RDF is they have every entity has a unique identifier.
Right? And that helps to prevent bringing in duplicate data. So that in when you bring in data to a knowledge graph, there is no need to do deduplication. And you coming you and me coming from a database background, we know no company spends so much of money doing data deduplication data deduplication.
Right? There are so much of code with knowledge graphs because of that capability, especially when it’s built with RDF technology.
You don’t need deduplication.
Data quality is inherently built into the system.
I hope that answers Let’s drill on that a little bit.
Let’s give the example that I provided, the the health care example. You know, patient, provider, payer.
Three three entities, three objects, three three things. Patient, provider, payer. What role does RDF play in saying this is a patient, this is a provider, this is a payer? It’s not gonna define the rules of how I define those things, is it? It’s gonna just provide more of a framework of where they exist.
It does provide a framework, but we can all think about it that somewhere or the other, they are all linked as they are all like, one is an organization.
The patient or the provider. Right? Provider at a doctor level is a human being. The patient is also a human being.
And that is the conceptual connection that comes in. Right? And that’s why each each entity, whether it’s a provider or an organization or a pair, has a unique identifier. Just like think about it as just like each website has a URL.
Right? When you go to CNN dot com, right, it has a URL. Right? That is unique.
Same way with every entity that comes into the knowledge graph, there is a you are a unified resource identified associated with it. Right.
Typically, if your data is modeled with RDF, the the useful part is if data is getting is if you need to merge data, right, or integrate two different datasets, which have the same entity, because if they’re modeled with RDF, they will have the same ID.
And merging it becomes much more easier, much more much more seamless as compared to. That’s why semantic data integration is one of the major areas where knowledge graphs can help.
So you use the example of organization. So RDF has a shared semantic definition around organization. That is a universal construct organization, universal construct location, universal construct person. Is that am I getting close?
Exactly. Exactly. You’re you’re you’re you’re getting close.
Okay. So so here in lies the difference between what an RDF would do and what a semantic layer would do and what MDM would do. Right? And, sir, I’m bringing things back to MDM because I’m an MDM nerd.
Sure.
Sure. But but but MDM in many ways, that object is is also a function of all of its attributes of how I define an organization, address, ZIP code, what what whatever, that would be part of the metadata that this in in a graph describes that that resource. Correct?
Exactly. That would be part of it. So an organization has a address.
Right? Now address, you can break it down into components name of a street, name of a building, a county, a state. The state, the country, each have their own ID. So now the the the power of reasoning comes in RDF because you we model it.
It’s the model is done in a very granular way, right, in a very granular way where address is not one entity by itself. It’s decomposed into street, building. I’m just giving an example here. Yeah.
County, state. Right? And the country has a unique ID. Right?
The state will have a unique ID. So there’ll be lot of if there are multiple, you know, multiple organizations in a state, they will have all pointers to going to state as an entity.
Does that does that make sense?
Yeah. No. It it it it absolutely does. I think I think there’s a lot of really interesting use cases here from a data management perspective, data quality.
Right? From an MDM perspective, I think that there are, interesting use cases here to apply graph from an entity resolution perspective, AKA matching perspective.
Because historically, matching you know, algorithmically driven matching was just not very efficient because you spend resources to evaluate every potential pair against each other. And when you’re talking billions and billions of rows, that can be very compute intensive. But a graph could tell you, in theory, if you run a graph first, it can tell you where there are relationships between nodes and where there aren’t any at all. Exactly. And if there’s no relationships at all, well, then why would you even burn the compute power to go to try to match them?
Exactly. We do entity disambiguation, entity linking all all the time to make sure that we are talking about the same entity and how that entity is linked to its to to to to the other ones. Yeah.
Okay. I I love it. We we went a little deep there, but that’s okay. We’re demystifying knowledge graphs.
Let’s let’s pull back up, and let’s let’s make a transition into talking about AI in our last the last half of our conversation here. So what I love about knowledge graphs is is, again, the idea of of context and being able to take things that don’t have context, meaning, you know, intersection of a row and a column. Right? Get a traditional database management approaches where there isn’t necessarily a lot of context there, and it’s tough to infer or derive context.
I love the knowledge graphs because it starts to bring us a little bit closer to the way that humans speak.
It’s not I mean but but what you’re talking about is these graphs are representing triples. Like, there are these basic subject, predicate type sentences that say, you know, Sumit podcast Malcolm. I mean, I think you could you could infer, okay, Sumit and Malcolm did a podcast together. Right? Like, there’s there’s there’s ways that you can start in making inferences here using things that start to look a little bit and sound a little bit more like human speech, which is exactly how Gen AI based solutions work. So, Sumit, how much today are you seeing, you know, your product and knowledge graphs at writ large be leveraged to help extract value out of structured data when using Gen AI based solutions?
Yeah. We are seeing a lot of traction around this, not just with structured data, but as well with unstructured data. Right? The name OntoText the the name OntoText, for example, comes from two things, ontology and text. We have been very strong with, you know, building knowledge graphs with ontologies and also a lot of capabilities around NLP and text analytics, right, where text can be parsed out into different entities and then linking linking the different entities together. Right?
If you look at Gartner’s, impact radar for twenty twenty four, right, knowledge graph is at the center with, you know, with adoption having accelerated with use of AI and LLMs, especially in a couple of very areas around few things. One is you must have heard about this term about the rag. The rag, I think, rag is the rage. Right now, it’s like the retrieval augmented generation.
That works purely on on, basically, on on on vector databases where you’re doing chunking and all that. What we found in our our r and d team found was we advocate this whole idea about using graph RAG, which is one step over on top of plain RAG.
What happens with RAG is let’s say this is my document. Right? And I chunk it into my these four fingers. Right?
These four fingers, I chunk the data and store it and embed it and create and create the embedding and store it into the vector database. Now if there are relationship between the chunks, often the RAG based approach is not able to figure that out. Right? Especially when it returns the results and the ranking is not correct, it may not give the right context to the user query to get an answer from the LLM.
Right? What we have seen is once you apply a graph based approach to RAG, where you take the knowledge graph, you convert it to text.
You know, there are knowledge graph to text converters, and then you chunk it. You get much better results in terms of accuracy when you do the RAG pattern. And we tried it with an example, I’ll give you, where we developed a knowledge graph, which is based on our website content. Right?
Our website has a lot of has a link called knowledge hub, where there are white papers, case studies, different blog posts, articles, all that. We took all that and created a knowledge graph out of it. Now when we we also built a natural language interface to it, where you can use natural language queries to query that graph now. You don’t need to do graph based specific query lang query, language to query the graph.
When we searched for show me the show me government agencies in US that are onto text customers. Right? NASA is one of our customers. It when we used plain rag, it did not give NASA as an answer because the plain rag did not know that NASA is a government agency.
Because the knowledge graph, as I was saying earlier, the instance data is, yeah, NASA is our customer.
But it is not overlaid. It’s not enriched with that NASA is a government agency.
Right? It comes from that ontology. Right? This organization is this type of an agency. It’s this in this type of an industry. Right?
So that’s where you see the power of knowledge graphs even in the AI space, in the RAG space. One of the other things we also did was, you know, with most LLM LLMs don’t give you provenance where they got that answer from. Right?
When we developed our knowledge graph, we when you give the answer, we also provide you links to the source from where that answer came from. So we are providing these kind of, you know, additional things on top of AI, or or making AI better.
The other aspect is also this is a huge area, which is around explainable AI, where knowledge graphs is are very used. One of the first steps is the provenance aspect where you can understand where it’s getting.
So those are the areas where we are doing a lot of work to, help enhance AI.
I I love it. So if you are a CDO and you have not yet found a way to dip your toe into the AI pool, strongly recommend taking a look at how to leverage potentially, tools like this is a Microsoft tool, but it doesn’t have to be a Microsoft tool. There’s a lot of tools out there like OpenAI Studio or other tools to help you build co pilots or chat bots where you could use a knowledge graph on top of our vector database to start using your internal data to what we call ground the behavior of these LLMs, where you could do things like maybe deploy a chatbot on top of your I’m just spitballing here.
On top of your HR manual or your your your employee policies or some other internally facing use case. If you’re concerned about hallucinations, if you’re concerned about these things getting out, deploy it on an internal use case. Right? So there’s there’s, you know, Randy Bean, New Vantage Partners did a study, earlier, in December that said only five percent of companies and five percent of data and analytics teams are actually doing this type of stuff, like custom rag patterns and vector databases and and using knowledge graphs to to help inform and control, as it were, the behavior of Gen AI based solutions.
So I would if you haven’t done it, I would certainly take a look and I’ve got a strong feeling that knowledge graphs will help significantly improve the performance, of your solution. Last kinda topic here.
I’m really intrigued by the the kind of the bringing together of of the worlds of data management and knowledge management. And I’ve kinda I spoke about this at a conference a couple of weeks ago in Germany, but I’d love to get your perspective, Sumit, on these worlds of data management, like what, you know, what what we do in data quality, MDM, data integration, data governance. Like, that’s this whole separate world. There’s this whole other world of knowledge management that has existed for a long time, like taxonomies, ontologies, even arguably like the world of search engines that you had mentioned, talking about searches, the use case, that for a lot of companies, it’s just a completely different world.
How do how do we bring these worlds together? Because it sounds like graph may be one of the ways that we can help bring these worlds together. Do you agree?
Oh, absolutely. And that’s what gets me so excited about, about knowledge graphs. Right? Because, you know, data is still in a mess in most organizations irrespective of, you know, the match talks landscape.
Right? So many different products, vendors out there. Why haven’t we solved the data problem yet? What is that missing piece?
Right?
There are so many tools. There are so many experts, thought leaders like us. Right? But why is data organizations not able to leverage the the capabilities of the data?
Right? And I think the reason is, as I think, also, Juan from data dot world, he he and me had a conversation a few weeks ago on on on one of these podcasts was we’re we’re conquering on the fact that the missing piece is the semantics piece. Right? And I call it for most organizations, we talk about semantics, semantic layer.
What happens is semantic layer becomes semantic later.
Right? We we do it. Let’s forget it. Let’s go for the buzzword, the shiny thing.
Right? The lake house, this, that. Right? This and I mean, until and unless we bring in semantics and the context, we we cannot be data driven without knowledge driven.
Right? You have to be knowledge driven, the context of the data. Right? It could be user context.
It could be process context. It could be content context. Right?
And context is all about metadata. And what better place to store your metadata than knowledge graphs where you can, you know, closely link the the metadata along with the data? I think that’s the most important missing piece that most organizations don’t yet get. We are trying our best to help convince the leadership to think about that aspect because just chasing technology will really not bring in all the the value add of the data and the technology by itself.
I I couldn’t agree more. I I love the idea of semantic layers being kind of the missing ingredient to to bring these worlds together. I would argue historically in the world of data management, we’ve kinda relied on business applications to provide all of the semantics in the context and to help provide you know, understand what the context are. Because if you’re a salesperson working in a CRM, you know what the context is.
Right? I’m trying to execute contracts. I’m trying to keep customers happy. But when that data just lands in a database and you just look at it, what the heck is the context?
That’s what we really, really struggle with. We can infer that this is related to a sales process or a marketing process, but that’s about as far as as as as we can take it.
And it’s funny. It’s it’s it’s AI that has just kinda broken that old world and said, this is not enough. Right? Like, have having stuff without context and just good old rows and columns as as great as that is for driving analytics at scale, right, and and data warehousing and all the things we do.
What it said is is that, hey. We don’t have enough of this context. We need context. That’s where a knowledge graph would come in.
That’s where the idea of a semantic layer would come in. I would argue MDM has some role to play here. It is it is a semantic layer by definition. It handles the definition of things.
But I do submit, like, see all of these things starting to come together. Love that conversation. Love it. Love what you’re working on.
With that, I think that that’s a great place to tie off.
Where can where can listeners who wanna know more and learn more about knowledge graphs and and and and, you know, seek more advice from a knowledgeable person like you, where could they go to get that information?
Yeah. They should go to Ontatext. They should connect with me on LinkedIn. Ontatext has a very rich website with lot of information around how knowledge graphs work, the fundamentals aspects of it.
Because we have seen, you know, historically, it has been a challenge to build knowledge graphs. But with the coming of LLMs, there is synergy happening in both sides. LLMs making building process of knowledge graphs easier, though no nowhere near close to automated completely, but it’s a step in the right direction. And knowledge graphs helping LLMs with graph rank, explainability, the provenance aspects as well.
So, yeah, reach out to us with, at at, you know, with, info at ontertext dot com or, you know, reach reach out to me on LinkedIn. I’ll be more than happy to help you understand the power of knowledge graphs, new demonstrations. We have a lot of demonstrators that are available to showcase the value of knowledge graphs.
Love it. Alright. Let’s leave it at that. Thanks, everybody, for tuning in to another episode of the CDO Matters podcast. I’m Malcolm, your humble host.
I hope if you’ve listened this long that you are already subscribed to the podcast or maybe you like us or rung the bell on YouTube or whatever it is you need to do to make sure that I show up high on the algorithm.
I would be thrilled if you continue to listen or check out our previous episodes. We do this every other week. If you aren’t already, please connect with me as well. Connect with Sumit on LinkedIn.
Connect with me on LinkedIn. I produce content every day that I hope that you will find valuable. If you are in the world of data, data governance, data quality, data management, data and analytics, BI, you name it, I would be thrilled if you could connect with me. Alright.
On that, thanks everybody for checking into this episode, and look for another one in another two weeks. Thanks everybody. Bye for now.
Thank you. Welcome. Thank you so much. Appreciate it, mate.
ABOUT THE SHOW
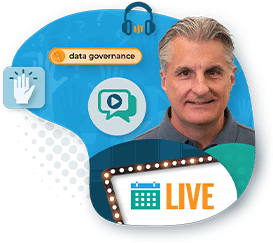