Episode Overview:
Are you confused about all of the hype around data products? Are you interested in understanding what the benefits of data products are and how you would implement them?
If yes, you need to check out the latest episode of the CDO Matters Podcast as Malcolm cuts through all of the hype around data products to provide clarity on what all data leaders should be conserving around data products, and why.
Listen as Malcolm demystifies the swirls surrounding data products, providing actionable and understandable insights on this popular trend in data.
Episode Links & Resources:
Good morning, good afternoon, good evening, good whatever time it is wherever you are in the world.
I’m Malcolm Hawker. I’m your host here at the CDO Matters podcast, and thank you for joining today.
We are going to talk about data products. You saw the title. Maybe you were intrigued.
Data products demystified.
Fied. Fied. Fied. Yeah. I’m excited to talk a little bit more about data products. We have spoken of them in the past.
I have going all the way back to episode twenty eight. This is episode sixty two. So a while ago, well over a year ago, I was talking about data products, Early you know? What what what time of the year is that?
What is that? Like, early twenty twenty three, late twenty twenty two? Because I was bullish on them them, and I’m bullish on them now.
But as you would have heard me talk about in previous podcasts, I’m bullish about the discipline of product management being applied to data and analytics.
Simply slapping a label of data product on something where you haven’t really fundamentally changed how you operate as a CDO or a leader of a data and analytics function, that’s not really that innovative.
It looks like oh, okay. I’m just checking my audio. Seems like everything’s okay. Since I’m the only one here, I just can’t anybody’s gonna tell me, hey. Your audio is not working. I think we’re okay.
Just checking. We’re recording on a, on a, platform that has just recently cape gone through some UI updates, so I don’t even know where all my buttons are. I don’t know what’s going on. I’m fumbling around in the dark as it were. Anyway, to fit quickly finish my thought. Yes. I’ve always been bullish on data products, but I’m bullish on data product management and the integration of product management as a discipline into the world of data and analytics.
Still, we’re gonna talk about data products today. I had a a post a couple of, I think it was last week.
By the time you listen to this, that post will have been out on LinkedIn for probably three months.
But it generated a little bit of stir, a little bit of buzz. It’s been one of my one of my more successful posts in the last couple of months because I built this graphic. I’m gonna share that with you, and I fully acknowledge that many of you are driving in your car or, you know, maybe working out on a treadmill. Hello, Catherine. Others.
And you can’t see what I will be sharing on the video version of the podcast. So I’ll walk you through the graphic, but I’m gonna I’m gonna share that that graphic. I’m gonna share some thoughts that I have around what I believe to be is a spectrum of where data products exist. I think they exist on the spectrum. We’re gonna talk about that a little bit more.
Some housekeeping items. By the time you listen to this or download this or watch this, I think it will actually be Halloween. If we stick to my production schedule, the day this launches will be October thirty first, Halloween. So happy Halloween. Happy scary Halloween. If we don’t stick to our production schedule and you’re watching this some other time or you watch this a year later and you’re like, what do you mean happy Halloween? It’s the middle of August.
It might be.
But as this is released, I think it will be Halloween twenty twenty four. So there you go.
If yes, when you listen to this or watch this, I will be in Edmonton, Alberta, Canada. Edmonton, Alberta, Canada, where I was born and raised, where all of my family still most of not all. Most of my family still lives, including my parents and my sister.
I’ll be in Edmonton, giving a keynote speech at a DAMA annual event. So the great fine folks at the the Edmonton chapter of DAMA invited me up there to to speak, so I’m excited about that. The prodigal son returns.
I went to undergraduate school, University of Alberta in Edmonton. I went to high school there. This I spent my first twenty five years there. I left, to go grab the brass ring, as it were.
I left to go to graduate school in the US, and then I’ve never kind of been back. I’ve stayed in the US the entire time. So, yes, all my family’s still there, but I’m really excited to return in a professional function. I’ve I’ve never gone to Edmonds in my hometown in any sort of professional way.
So that’s cool. Looking forward to that.
I will have also recently returned from Munich, Germany, where I was, giving a keynote speech at the Symantec Summit, sponsored by my good friends at Enterprise Knowledge. So talking about knowledge management, the transition to knowledge management, talking about next generation semantic layers. So I hope that they will be posting some of the, the conference presentations. I’m not surely sure how that’s actually gonna work.
But I think that’s gonna be something I’m talking about at more events in twenty twenty five, next gen semantic layers. I’m an expert in MDM, and MDM is a semantic layer, always has been. It it it’s it’s a decoder ring. It allows you to manage definitions and how you define things and how you define metadata and how you define master data.
So, yeah, MDM is a semantic layer. So I come at my expertise here honestly. But when you kinda look at the future, which I love to do, thinking about, okay. Wait.
Where does MDM fit within a modern data ecosystem in the year twenty thirty? What’s that gonna look like? When you layer in AI, when you layer in kind of legacy capabilities like MDM and metadata management, and you layer in data governance, and you layer in knowledge graphs, you layer in some of these evolving technologies, things start to get really interesting, really interesting. And I’m gonna be talking about that.
Well, I will have talked about that in Munich a couple weeks ago, which for me today is in a couple weeks from now. Time bending.
But like I said, I think I’m excited to talk more about that at events in twenty twenty five because I think it’s something that we need to start steering towards now, particularly what I will call this transition away from data management to more knowledge management.
There is a very useful framework called the DIKW framework, data information knowledge wisdom. You can think of it maybe like a pyramid with data at the bottom and wisdom at the top of the pyramid.
And, you know, data is what we’ve historically managed always, and it exists without context, particularly, you know, the intersection of a row and a column. There’s just a field. Right? There’s something.
There’s an entry. And what the heck is that? You know? There’s very little context. It’s difficult for AI based systems or generative AI based systems to to run off of those things because GenAI systems run like we think.
Right? It’s natural language, how those things are you know, GenAI systems are built on and optimized by natural language.
The intersection of a role and column is anything but natural language. So I think where we need to get to, particularly in the context of this next gen semantic layer, is getting to a place where we are managing knowledge less so data. We’ll we’ll always manage data.
I think there’s just a natural evolution that’s gonna start to happen here where we are widely using graphs. We are widely using AI, other systems to understand at a deeper level the context of business. Maybe a a useful way to think about this would be the narrative of business. Imagine if what we were storing instead of the intersection of rows and columns and I know this is structured data. It’s not always rows and columns, but this this you just work with my metaphor here because this is a metaphor.
Instead of the intersection of a role in college, call column, imagine that what we were storing was a story.
Restoring a story.
We’d be we’d be databasing a story.
A narrative, right, where instead of, you know, rows at the intersection of rows and columns, what we’re storing is Malcolm clicks on button. Malcolm scans items. Right? Malcolm buys item. Item is shipped.
Revenue recognized. Right? Like, a basic form of this is the triples that naturally exist within graph. Right? Subjects and predicates where it’s a very basic form of a of a sentence.
Right? But where in the future, we really blow that out, and there’s far more context and there’s far more knowledge than we ever have before.
AI will process that knowledge. I suspect Gen AI based systems will be processing that knowledge and give us levels of insight to tomorrow that we really just do not have today. Because we we have these blinders that exist within the world of data that we manage today and in the world of knowledge management in the future, I think some really, really exciting things happen. But what do we do?
What do we need to do to start moving towards that now? Right? There are a few people in our world like my dear friend Juan Cakeda, who is advocating the use of graph as a way to improve the performance of LLMs. I think this is just like a baby step, like one little wee baby step towards this world of knowledge management.
So I will have given a keynote speech on this two weeks ago in Munich, Germany. Will have been my first time to Munich. I’m stoked about that.
So I can’t really pitch the Germany event because it would have already happened.
So it’s not like, hey. Go sign up.
But, yeah, hopefully, there will be some, you know the presentation will be posted. And if not, I’m gonna be talking about that a lot in twenty twenty five, probably at a few different events. That could be a good one for Data Universe, actually. I haven’t really submitted my speaking for that yet, so we’ll see. Anyway, other housekeeping items, I will have also just returned from Big Data London. I’m going there. I’m getting on a plane in two days to go to Big Data London.
Man. I’m I’m excited, but also a little, what’s what’s the word? Tense? I don’t I don’t I don’t know.
But, like, I’m gonna be working the prophecy booth, two days straight. And, apparently, there’s, like, twenty five thousand people at this time. So it’s like, hopefully, all twenty five thousand don’t show up at the booth at the same time. Of course, they won’t.
But you get my point. There’s gonna be a lot of people, and I think it’s gonna be pretty chaotic, and I think it’s gonna be pretty intense, and I think it’s gonna be pretty awesome. But it’s it’s like me and, hey, folks. You might you might find this hard to believe. I am a Myers Briggs introvert. Right? It’s, I I am an INTJ.
I forget what all the other ones are. Maybe you remember this stuff. But but, anyway, being around people draws energy from me. That’s what introvert in Myers Briggs parlance means.
Right? It draws energy from me. I can talk all day long. I can I can I can go to industry events?
I can be literally standing in front of hundreds, if not thousands, of people talking. It’s what I do. Right? I actually enjoy that.
However, it that those experiences through the day cumulatively draw energy from me. So at the end of the day, maybe you’re a little bit like me. Maybe you need to retire to your ice cave, to your, you know, your fortress of solitude. Maybe you need downtime. Maybe you just need no interaction, right, to allow your batteries, your social batteries to recharge. That’s me.
That’s me. And an event like Big Data London where it’s just, like, full on, full frontal, like, go, go, go, you know, it’s gonna be great. Looking forward to seeing a lot of people. Looking forward to seeing a lot of friends.
But I just know at the end of that, it’ll I’ll feel like I’ve been run over by a tank just because of I know my social tank will be empty.
But on the bright side, I’ll have a ten hour flight home, where I can decompress, right, and just kinda turn things off. That’s that’s one of the joys of of joys.
Are there any joys of travel? For me, that’s one of them. Traveling actually allows me to decompress after those types of events. So, I I’ve been around a lot of extroverts where, you know, they they not being around people is is like, anxiety causing for them. And in the case of this, they would they would go to a big day to London, and then on the plane home, they’d wanna sit next to somebody they know so they could talk to them the entire way. For me, it’s like no.
Leave me alone.
Leave me alone.
Also causes, like, awkwardness sometimes when you’re, you know, at work events and whatnot, and, you know, you you’re at you’re you’re doing work all day long, and then somebody’s like, hey. Let’s go. Let’s get together and do and I’m the guy that’s, no. I gotta, Yeah. I got the thing with the guy at the place that I gotta I gotta be yeah. No.
Kinda coming up with slightly creative excuses for me to go decompress and and turn off.
So so that’s me. Probably a little bit of two TMI, but I have a really busy September and October.
I will share all of my insights, of of course, as I always do. I’ll share them on LinkedIn. I’ll be sharing them here on the podcast, of course.
Probably LinkedIn is a better venue for that. I’ll be sharing that in my live events. My my monthly CDL matters live, the third Friday of every month. I do a live event.
That’s a perfect topic for live events. Right? It’s just to talk about the stuff that I heard at at all these great events that I get to go to. I live a a a blessed life.
I have people who listen to me. Like, so thank you. That’s that’s amazing. I have people who support me and who give me great feedback on the content that I create and the insights that I create, and we’ve got this growing community here through the CDM Matters brand.
It’s it’s all fantastic. I just live I just live such a blessed life. Thank you for tuning in. Thank you for supporting, the content we create.
My goal is to help. My goal is to extend the tenures of CDOs, and, I I hope that we’re doing that. And I hope it doesn’t feel like I’m I’m complaining about getting to go to these amazing events around the globe because I’m not. But I do know I do know that just just mentally and physically, they can’t can be training for me.
And and it just is what it is, and that’s fine.
And I love these events, and I love going to see people. I love to go see old friends. I’ve I’ve met so many friends through LinkedIn and through through the conference circuit. Like like, I’ve always been friends with Scott Taylor, but, you know, like, Juan Cicada, wonderful, wonderful human, and who’s somebody who I now consider a friend. Sameer Sharma, somebody I also consider a friend now who who I’ve met kinda just through LinkedIn, and and it’s just it’s just so I’m just so blessed. It’s so awesome.
Let’s talk about data products.
Let’s demystify data products.
Where to start? I’m not gonna share the graphic just yet because I I need to be mindful here that a lot of people are are not watching on YouTube, that they’re that they’re list they’re only listening. So I’m gonna explain the graphic, then I will share it for people on on YouTube who are who are watching. So I think the most useful thing for me, and it took me a while to to figure all of this out, was was coming to the conclusion that the concept of data products within the world of data and analytics really exists on a spectrum.
On one hand of the spectrum, let’s just call it the shift left side of the spectrum, you have data products that are built for, I would argue, internal analytical consumers, people who are using those products to create analytical insights.
If you follow kind of the data mesh paradigm, shifting left gets you as close to the source as possible. That’s what that’s what the shift, I think, metaphorically means, at least through a data mesh paradigm.
On that end of the spectrum, the shift left, a useful way to think about data products maybe is more of a, what I would call, a raw material and not an actual finished product. Not an actual finished product, but a but a a processed curated, ready for consumption raw material.
Right? Something that is gonna go into something else to create value downstream.
That’s one end of the spectrum, the ship the left. The extreme other end of the spectrum, where I kind of live and breathe and where I’ve always lived and breathed, at least through the idea of data products, let’s just call it shift right, is a world that I see where data products are serving and solving a specific customer need. They are finished products. They are being consumed by the end customer.
Right? That end customer generally is going to be out not exclusively, but generally is going to be outside the data and analytics function. There will be people in marketing. There’ll be people in procurement.
There’ll be people maybe even external people. Maybe that data product is something that you’re monetizing. Maybe it’s a dataset that you are monetizing to somebody outside your four walls, And that’s valid too. But over on that right hand side of this spectrum, we’re talking about finished products, finished goods.
Here’s how I define the products at that end of the spectrum, the data products at that end end of the spectrum. They are things that are built by data and analytics function that solve a specific customer need, that address a specific customer need, or solve a specific customer problem that the customer would otherwise be willing to pay for.
That last bit is really, really important, and it’s something we need more of in the world of data and analytics.
This is a bit of a thought exercise, and I and I have this dream.
I have a dream that one day what I’m about to share with you is not a thought exercise, that it’s actually how our data functions run.
But let’s think about a thought exercise. That thought exercise is, can you imagine a world where in a world where data and analytics professionals, CDOs, VPs data analytics, directors data analytics, had to charge for every one of their products? If you had to put a price tag on every product that you offered to your customer, could you? And if you did that, would you be able to operate as a successful p and l?
Would you be able to operate as a successful business?
Would you bring in more money than you put out?
That’s the thought exercise that I think we need more of. That’s what I you know what? As a north star as a north star, I love that idea.
Right? I love that idea to say this is my north star. As a data and analyst leader, as a CDO, this is the north star that I want to get to.
Right? And you can articulate this. Absolutely, you can articulate this through a data strategy. Most certainly, you could.
But that’s that’s a I think that’s a useful lower start. I think it’s a useful thought exercise, and it does align nicely to this idea of a shift right product mentality when applied in the world of data and analytics. If I if I’m building products that are being consumed by customers, would they charge for them? Would they pay for them, I should say?
Would they pay for them? So this necessarily means that over on the right hand side of the spectrum, this necessarily means you need to understand how much your stuff costs, right, and how much customers would be willing to pay for it. Right? It’s two sides of one coin.
Right? Cost benefit.
As a formula, that’s ROI.
Right? But how much does it cost and what benefit is it driving? The benefit could be articulated through the price that a customer would be willing to pay.
The delta is profit, quite clearly.
So that’s a useful exercise. That’s a useful perspective.
And if we’re on the right end of that spectrum, and if we’re really and truly and honestly embracing product management as a discipline, we would necessarily need to also embrace what I would call value engineering, pricing.
Right? I’ve run a product function. I have been a chief product officer.
I managed large teams who were defining and implementing and selling. In my case, it was project management software.
We had a team that had to figure out how much are we gonna charge for this thing.
What is it worth in the market for this thing, for this product, for these capabilities?
What does it cost us?
Right? That’s part of a product function. And if you’re serious about data products, I would argue I would argue that you need to be serious about value engineering pricing. You need to be serious about product management.
You need to hire product management. But, again, that’s my perspective. On this spectrum, all the way left, data is a raw material where the customer is largely people in an analytics function, where the end goal is efficiency and scale, and that’s okay. It’s okay.
It’s not how I would do it. It’s not how I would recommend, but it’s okay.
Right? So you’re driving efficiency and scale. You’re driving reuse.
That’s okay on the left hand side. But on the right hand side, that’s where I think the transformational value is.
I think if you do that stuff on the right hand side, if you apply product management, if you are doing pricing, if you if you understand the value you’re delivering, if you are just relentlessly focused on user centric design, right, if you understand your customer needs, you understand what what their biggest problems are, what their biggest challenges are, if you understand their business processes as well as they understand their business processes, if that’s the world you are going to, that’s where the transformational value is. That’s like, holy cow. That’s like you’re building stuff that customers want to use.
And it doesn’t necessarily need to be about reuse.
So it’s it’s it’s, you know, it’s interesting. There’s there’s a lot of people who just automatically naturally assume the right way to do data products is to build things that are built for scale and reuse.
Right? And this is what p ten Strangle’s book, data management to scale, I would argue is largely about.
Extremely insightful, extremely valuable, and, yes, we should be aiming for efficiencies and scale.
Absolutely, we should.
However, if you take a product management mindset, not everything needs to be about efficiency and skill and scale. Doesn’t necessarily need to be.
This piece of clothing that I’m wearing is just is woven from the same cloth that a female, you know, a shirt designed for somebody of a different gender would pay often pay more for. Or maybe this goes into an extremely expensive this fabric goes into an extremely expensive suit jacket or something else. Anyway, you get my point. Or maybe you don’t. I haven’t quite made it yet.
But but my point is is that for some products, right, extremely high value products, it doesn’t necessarily need to about be about efficiency and scale. They only make Rolls Royce only makes a few cars every year, but they charge a premium for those cars. And they have handmade components, hand machine components, where the building of a brake system for a Rolls Royce or a Ferrari or a Lambo is not about scale. It’s about precision.
It’s about quality because there are customers who would be willing to pay for that. So if you take a product management perspective on these things, you could see a world where maybe I’m gonna build a highly customized AI model for something to solve some problem, and it’s a single use enterprise. It is a single use undertaking, one model, but it’s so valuable, and it solves such big problems. The customer is willing to pay so much for it that I take a highly bespoke approach to building it, a customized approach to building it.
Do I need to be worried about efficiency and scale for that model that is gonna drive that much value? I I would argue less so.
Less so. So not everything needs to always be about efficiency and scale. Of course, we need to run efficiently. Of course, we need to scale when we need to scale. I’m just saying if you are focused on product management, the market dynamics and customer needs will drive how you fulfill those needs.
It will necessarily determine how you fulfill those needs.
The flip side of the highly bespoke, highly customized product that is solving a very, very high value problem, the flip side of that is maybe self-service analytics, where part of that product mix and, yes, again, this is more product management where you’ve got a mix of products. Maybe you’ve got some expensive products. Maybe you’ve got some cheap products. On the cheaper end of that product mix, maybe you’ve got self-service analytics, where you have things that are focused specifically on efficiency and scale, where you have more self-service from a help or customer support perspective.
I think think it’s some of the things that that befuddled us in the world of data and analytics is that we try to apply these one size fits all models.
Right? And maybe sometimes they’re appropriate for some use cases and for some customers, but other use cases and other customers, they’re not appropriate. And that’s I think that’s the trap that we fall into. We always assume it always necessarily must be about scale.
It always necessarily must be about efficiency. Those things are important. Don’t get me wrong. But again, if you knew how much customers were willing to pay and if you knew how much things cost to build, you’d be able to have a rich assortment of products.
Some were expensive, some are cheap depending on the product needs, depending on your market needs.
That’s what a product management approach is going to do for us. It’s going to help us understand the go to market for all of these things.
Sometimes, if you’re taking a very kind of low end approach where it is all about efficiency and scale, Right? Maybe maybe there is a higher aspect for very, you know, very little customized training.
Right?
Data literacy as it were. Y’all know my peer my my my perspective about data literacy, but you get my point. There’s a support function. There’s a go to market function. Sometimes things will be highly customized. Sometimes things will be bespoke for that individual product, and other times there’ll be more mass market.
We need more of this thinking in the world of data and analytics. We need to get away from this one size fits all approach to everything because it’s not how the world works. It’s not what our customers want necessarily. Maybe some do.
Maybe some do, but others most certainly do not. They need more hand holding maybe because the products or the problems they’ve got, the challenges they’ve got are higher value.
So back to the spectrum. Shifting right shifting right is all about building products that end consumers like. The benefit is the goes to the customer. Right? On the left hand side of the spectrum, I would argue the benefit largely goes to the data and analytics team.
If you are relentlessly focused on efficiency and scale, that’s a part of a shift left paradigm, if that’s what you’re relentlessly focused on, I think you could argue maybe the end consumer would benefit a little bit, maybe, but, really, the benefits are about data analytics. They accrue to the data and analytics team because you’re operating more efficiently. You’re getting better, bigger, faster, stronger. Your operations are more efficient. And those are good things. They’re good things.
But, again, folks, I don’t think they’re transformational.
Right? I when when you deploy a data catalog and you start to expose some stuff in the data catalog and say, hey. Bing, bing, bing. We’ve got data products.
I think our customers and I think our business people or friends in the business see that, and they’re like, like, what?
Like, it’s just another label and and and data people keep doing this, throwing new labels on stuff or thinking things are new and things are different and they’re actually really not.
Is there value to exposing master data records, for example, in a data catalog? Of course, there is.
Is there value in having a single place to go to access data, to look at who has permissions and data, to look at descriptions, to look at glossary terms for all of my data? Of course, there’s value there. Not saying there’s not. I’m just saying that’s not gonna be transformational.
It’s gonna be something. It’s gonna be something, but it’s not something that you’re gonna want your c level people knowing about. It’s not something you’re gonna be ringing a bell about. It’s not something that you’re get is gonna be like, hey. Look at us. We’re amazing. We deploy data products.
No. Not at least at the left hand side. Again, there’s value there. There’s benefit there. I’m supportive of those efforts.
However, if you’re serious about using data products to help transform the way you interact with your customers, the way you manage a data and analytics function, the way you value your function, I would argue you need to be on the right hand side of that spectrum.
Now something that was raised to me during some of the banter that was, that was experienced in in LinkedIn and, man, you know, people you can knock LinkedIn all day, but the quality of the interactions that I that I have on there are just so good. And the feedback and the comments that I get are so good.
Somebody mentioned it. It was actually my friend, Brian t O’Neil, past guest of the podcast and also a data products guru who happens to think very, very, very much like me. So maybe that’s why we get along.
But Brian O’Neil, in a comment, mentioned, hey, these things don’t necessarily need to in essence, I’m paraphrasing him, forgive me, Brian.
But in essence in the comments said, hey, these things are not necessarily mutually exclusive.
If you wanted to take a shift right, right, if you wanted to have that relentless focus on customer success, you could argue that you need to do all the things in the left as well. And I think that’s true. I think that’s actually true as long as we don’t get too hung up on a one size fits all. Because that’s what I happen to see on the left hand side is, generally, I see this kinda one size fits all because I think we are we as data people are prone to highly deterministic thinking. Right?
So, yes, shifting left and being efficient in how we operate and doing things like building data catalogs and doing things like, you know, having transparency on lineage and having business glossaries and terms defined and all that stuff.
That’s great. You know, reusable, discoverable I forget what the you know, on the left hand side, what what some of the aspects of the data product are. That’s another way they differ, by the way. When you ask people who are kind of of a shift left mindset, you know, how do you define a product? They will define it through the attributes.
It’s governed. It is discoverable. It is consistent.
You know?
It it it it it is defined through the attributes of the product. If you ask somebody in the right hand side what the product is, typically, they will tend to define the products through the benefits that it gives to the business. Another fundamental shift. I would argue we need to get to that world more as data people and define and articulate and express data products through the benefits of the problems the benefits they deliver, the problems they solve.
Right? And I think we need more of that.
Is it functionally correct to describe a data product through its attributes? Sure.
Sure. You can do that. I can describe this this this shirt that I’m wearing. I can describe that through all of its raw materials. Right? In the manufacturing world, that’s called the BOM, a bill of materials.
I can define a shirt as being, you know, thread and dye. Right? And what else is on here? I don’t even know.
But you get my point. Right? Like, you can you can define things through their component pieces. It’s not incorrect to do that.
But if you think about things like maybe, through a manufacturing lens, does is that the way that the customer describes it?
No. Right? I’ll probably just call it a shirt. But, ultimately, I think a better way to describe it is, you know, an apparel item, a piece of clothing. Right? It’s winter or it’s summer or it’s sport apparel and on and on. So it’s clothing specifically designed for this purpose.
Right? That’s that’s the world I would say we need to get to. Instead of defining things through a bill of materials, through the component pieces, which is what we we are data people. We’re librarians at heart, and we like our our ontologies and our hierarchies, and that’s kinda how we kinda look at the world. That’s okay.
But we also need more of this product centric view of the world. Alright. So for those of you who are watching on YouTube, Let me bring up this slide.
Hopefully, this works. I’m hoping it works.
So here you go, YouTube. And, again, for my friends who are only listening, I’m just basically sharing a graphic that that is very, very basic. Left hand side, shift left, data products as a raw material.
Right? Data products defined by their attributes, data products that are built to drive efficiency in a data team, typically distributed through data catalogs. That’s the left hand side of this diagram.
The right hand side is one where data products are benefiting end consumers, typically outside of data and analytics function, where these are finished goods. I would call them finished goods. The distribution may vary. Right?
It doesn’t have to be through a data catalog. It could be be through an API. It could be through a a file feed. It could who knows?
Right? Could could be delivered in multiple ways. The distribution method will be determined by the product, would be determined by the need, it would be determined by the customer. How does the customer want to consume that data product?
Maybe that will be in a dashboard sometimes. That’s okay. But maybe sometimes it won’t.
So, again, getting away from this one size fits all where all we do is distribute through a catalog or we only distribute through dashboards. We could distribute insights in a lot of different ways in a lot of different ways, and it all depends on what the customer wants in the problem they’re trying to solve, their use case that it that is that is that is being solved for.
So right hand side, I would call that more of a product management mindset, and it’s all about value. It’s all about value where you are relentlessly focused on delivering customer value and where you’re quantifying that value where you know what it costs to make it and you know what the value is that’s being delivered through that product.
You’re operating optimally.
At some point in the future, you’re operating actually as a p and l, or if you had to, you could.
You could justify every dollar invested in a data and analytics function. Every dollar, you could justify it. That’s the right hand side.
Where, ultimately, the efficiency gains here are for the business. They’re not necessarily for us, you know, per se. Yes. We need to operate more efficiently, but the goal here is customer satisfaction. The goal here is customer retention and adoption.
The goal our success as data people would be measured through our customer success.
That’s the world that we are trying to get to. On this spectrum, there’s a bunch of different labels.
Data products, data as a product, data product management.
We get so hung up on these def definitions.
You know? I I think the key takeaway, at least from this graphic and what I’m trying to share with you, is there are multiple valid approaches. I’m not trying to suggest that shifting left doesn’t have value. It certainly does have value. It certainly does.
I would think, though, that if you are only shifting left, you really, really need to start thinking about how do you go upstream.
How do you work your way from the left over to the right? That’s what you need to be thinking about. How do we get over to the right? Because, again, that’s where the transformational value is going to be delivered.
It’s not gonna be over in the left hand side. And if you are looking for budget, you’re looking for resources, you’re looking for people to get excited within your organization because you wanna do data products, And if all you’re doing is exposing some stuff in a data catalog, I think you’re gonna have a hard time getting that support. I think you’re gonna have a hard time getting excited. You might get budget for it because I do think data catalogs are incredibly important.
They’re foundational. Again, they’re foundational. Don’t get me wrong.
I like data catalogs. We all need data catalogs.
But my point is is that you’re if you’re out there waving this flag that says we are gonna transform how we operate, we are going to deliver unbelievable value for our customers, You need to be leaning right and not left.
So, hopefully, that makes sense. I’m gonna stop sharing.
This is available through, the graphic that I just shared where I’ve been talking about. It is on my LinkedIn post. It’s on my LinkedIn feed. Right? So if you’re searching for me through LinkedIn and you go back to when when did I post that? First week of September.
In my post in the first week of September, you’ll you’ll find it. Or you know what? If you just DM me, send me a direct message on LinkedIn. I’ll send you the graphic.
I do wanna talk about this a little bit more in twenty twenty five as well. I talked about next gen semantic layers and its transition to knowledge management that I think is important for us as data leaders. And I think this data product focus is also important for us, particularly data product management.
So with that, my friends, I will bid you ado for this episode, episode, I believe, sixty two of the CDO Matters podcast. Happy Halloween. If it is in fact when you are consuming this, you may be consuming this way into the future, in which case, hey. It’s not Halloween, but I hope you had a a great Halloween twenty twenty four.
Thank you for tuning in. If you made it this far, please consider subscribing through your podcast provider of choice. Please consider maybe throwing a little like on this if you’re watching it on YouTube that would be awesome please consider subscribing to the prophecy channel we do this every two weeks my goal here is to help if you’ve got any questions about anything that I talked about send me a message on LinkedIn. And by the way, if we are not already connected on LinkedIn, please let’s connect.
I talk with people on LinkedIn every day. They’ll just send me these random questions. They’re like, hey, we’re wrestling with this, and we’re wrestling with that, and I happily answer them because that is my job. I’m here to help.
I’m here to extend the tenures of CDOs. So with that, thank you so much for listening. I will see everybody, and I will hope to see you again in another episode of the CDM Matters podcast sometime very, very soon. Thanks, everybody.
Bye for now.
ABOUT THE SHOW
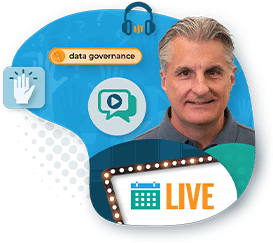