Episode Overview:
New technologies never exist in a vacuum, and getting value from any new innovation requires the right combination of multiple enabling competencies, including people and processes.
In this episode of the CDO Matters Podcast, Dr. Michael Proksch, author of the Wiley book ‘AI Value Creation’, shares insights on how some of the biggest global brands are generating value from AI-based solutions.
Episode Links & Resources:
Hi. I’m Malcolm Hawker, and this is the CDO Matters podcast.
The show where I dig deep into the strategic insights, best practices, and practical recommendations that modern data leaders need to help their organizations become truly data driven.
Tune in for thought provoking discussions with data, IT, and business leaders to learn about the CDO matters that are top of mind for today’s chief data officers.
Good morning. Good afternoon. Good evening. Good. Whatever time it is, wherever you are in the world. I am Malcolm Hawker. I am the host of the CDO Matters podcast.
Thank you for joining us today. We are going to talk about AI value creation.
I’m thrilled to be joined today by doctor Michael Broxcher, who is the chief scientist of Accelerid. And you are the Accelerid is the technology provider to University of Maryland. Correct?
University of Maryland Global Campus. Yes. But, it’s it’s not just University of Maryland Global Campus. We serve various universities. Mhmm.
Okay.
So you are your your background is economics, I believe, but you have re recently coauthored a a widely published book, The Secrets of AI Value Creation. Correct?
That’s true. Yeah. So, apparently, you saw it.
Well okay. So so I met you two weeks ago. I just it’s two weeks ago, two and a half weeks ago at the CDOIQ, event in Boston, and I was lucky enough to stick around to the end. Michael gave the last presentation of the conference, and it was most certainly one of the best, if not the best.
It was one of the best presentations of the conference, be because it it gave some kind of fresh perspectives about AI, particularly related to AI value creation. And we’re gonna we’re gonna talk about that today because there’s a lot of people Michael, I’m I’m sure you’ve been hearing this. There’s a lot of people talking about, oh, there’s no ROI. Right?
It’s the last number I heard was it’s six hundred billion being spent on Gen AI and, like, maybe a hundred billion in value. And a lot of people saying, hey. Is this is this is this worth it? Have you have you heard some of this the same rumors floating around recently?
I I haven’t read any numbers. Oh. But, I’m with you on it.
Mean meaning meaning are you dubious of the benefit?
Or or are are you are you optimistic the benefits will be there eventually?
Well, I mean, it’s gonna be eventually. As soon as we, let’s say, rephrase our expectations towards the dictante towards the technology.
We’re gonna if we after we see what we can actually expect, what are the real capabilities of the technology, we can actually make, money with it.
So are you saying that there is a knowledge gap then with a lot of practitioners, a lot of CDOs today who really don’t necessarily understand the capabilities of the technology? Or is it more about, you know, modifying our existing operating models, modifying our existing perspectives in in order to extract the value from the technology? What what is what are some of the real challenges here when it comes to to value realization?
I would say both, but it’s not necessarily the CDOs or and that are struggling to understand the technology. It’s more about, you know, businesses in total, to not go after the shiny object and really have realistic expectations and not, you know, there’s so much hype around it. And that that definitely creates a movement, which is good thing.
The challenge is that, you know, you need to you need to take the learnings to really get to value creation. And then, you know, Gen AI is just one piece of AI. Right? So when we talk about AI, it’s way, way bigger. Right? It’s it’s the it’s the newest one, which is cool and looks great and, you know, you can create a green cat.
But I haven’t seen the big value from it yet from green cats.
But, besides that, there there is certainly value and there’s certainly money to be made. The question is, how? And, you know, the newest, I like to follow Sequoia and, you know, they’re the big investors in the field. Right?
And they’re, you know, talking about the same same same issue. Right? And it’s, a the technology is there now, but how do you actually create value from it? And then as you said, the the value creation is way bigger.
Right? So this is just the technology piece. And now you need a and that’s, you know, we talked about at the conference and we talked about it in the book. It’s about there’s, you know, many factors that create you know, that are relevant for value creation.
Right? And we need to take a step back and not just talk about technology because that’s not the issue.
Well, what you rightfully point out in in the book and the presentation is that AI is not new. Right? I think sometimes I I fall into this trap of thinking, oh, well, this is we’re in an early adopter phase.
Right? And it’s in an in an early adopter phase, it’s normal for there to be a disparity between the amount of investment going into something and the amount of value coming out of it. Like, a lot of people are applying the Internet as as an analog and saying, well, in the early days of the Internet, there was a lot of investment in infrastructure, and and systems, and a lot of the returns weren’t there.
But what you rightfully point out is this is not new, and this is not an early adopter phase we’re in right now. Arguably, maybe more of a mature life cycle. Correct?
I would say mature.
But Oh, no.
No. You you you’re right. Like, we we we are pretty mature. I would say after, you know, how you how you define AI, you know, when you say it started in nine fifties, right, it’s seventy five years ago.
Right? Almost. So it’s like, no. It’s not you. Right? It’s way older than me. And, I consider myself old.
But it’s like, you know, we the the the the the challenges here because we start to we constantly give it new names.
We’re always you know, every time we we we see some hype, we seem to throw everything off a board that we learned before and say, hey. You know, in the times of AI now, everything’s different. Right? You can forget everything you learned. If you don’t know about AI I know you don’t see that on on on, you know, on x and and all the social media things. If if you don’t know about AI this year, you’re gonna be gone next year.
Oh, okay. So, probably the last forty years that I learned in my life are worth nothing.
But that’s that’s exactly the problem that, you know, when we started and I started thinking about the book, and I talked to my mentors and now coauthors, and I was very lucky to have very experienced coauthors with me, managing vice president of Capital One, Nishant Paliwal, CEO of Premier Tech, doctor William Baylard, and we have the the CDO of Hewlett Packard and the and the CDO of, CBS and the CDO of Starbucks and so many others, that were so kind and become industry contributors to the book and help us writing it because they like the concept.
We it’s not like they they started yesterday. You know? They are achievers in that field because they have been doing it for a long time, And, they have done something right, apparently, because they wouldn’t be where they are. So I was very lucky through the book and, you know, through my last decades of experience in industry and to, you know, separate between the successful AI achievers and the less successful ones and, you know, go through that journey. And then we were actually you know, most of the things that we that we learned was that somehow everybody seems to be alone on that journey.
Mhmm. We don’t really share the experiences, number one.
Number two, is this partially right? Because we only share the successful experiences.
And that’s where we have the hype created. Right? We have ten cases where we tried projects and POCs and things, and one of them was successful. And we share that one, but we don’t talk about the nine failures.
So if we don’t know about the negatives, how can we learn to do it better? Right? And that’s something that we realized that there was no book that that has that, you know, that points out all the mistakes on the on the on on the journey. Right?
And then when we talk about those AI achievers, most of them, you know, when you really look at them, they started decades ago.
They started last year and are an achiever this year.
So it’s like we talk about John Deere and Coca Cola and others. Right? But they started early two thousands or end of the nineties. Right? John Deere collected GPS data for a long time, over two decades, and now they have self driving tractors.
But they started that journey a long time ago. Right? And that’s like, what we seem to forget is, like, there is a path to success. And it’s a marathon. It’s not a sprint.
And so you’re gonna you have to be you know, you have to stay focused beyond that marathon. What we also learned is that that marathon is nonlinear.
It goes up and up and down. And so many of those many of the successful people today, they had their failures and experiences, including myself. I had many of them, like, many failures, and I failed many times, but I always learn from it. I always analyze and figure out, okay.
What did I do wrong? And what we figured out eventually throughout that book and by looking at it from a holistic perspective that it’s not the technology that drives the value. It’s a piece of it that drives the value, but there’s more elements to it. And when you think about the different elements, when you when you ask your engineer, you know, what is important to create value, you know, to have a stable pipeline, right, or to have an API that is, you know, serving the answers in, you know, low latency.
Right? You go to the data scientist, highly accurate models. You go to the business person.
You know, you have the right KPIs.
You go to your data engineer.
Right? And it’s like, yes. You have the, you know, the right data. And then you go to, you know, to their chief transformation officer.
You have everybody on board.
And, you know, and you you focus on, you know, people love the technology.
And all of them are in their individual areas are all correct, and they optimize towards it.
But the problem is if you don’t have a holistic optimization, it it’s gonna fail. And that’s when but that’s what you what many learn, and I learned on that on that path the same way. Right?
Another way of looking at it, if if I give you five million dollars and say, hey. That’s that’s for funding of your AI initiative, and you take five million dollars and give it to AWS or, you know, Azure, you know, the value created is exactly zero.
There’s nothing done with it. You just invested in the technology, which is cool. You need it. Right?
But then you learn, wait. Something’s missing. Right? I I need a I need to focus a little bit on the algorithms.
Right? Yeah. Okay. Cool. Now you invest, you know, maybe only four million dollars in the technology and one million in algorithms.
You still fail, and then you realize, wait. I I need to I need to invest a little bit maybe in in, business, you know, business domain knowledge, some, say, five AI business consultant that understands how to apply to business and will actually find the opportunities.
And then you keep going. Right? You invest then another million in data and data management, especially, and then you invest another million in transformation. And then you realize, wait. Everything is now a million dollars, all of those five, and you seem to create value. So what does it tell us? Like, this example that this formula we’re talking about is not additive.
You cannot compensate one with the other.
So you cannot throw more money at, you know, at technology and expect that you don’t need to do anything in transformation or change management or organizing our psychology, however you wanna call it. Right?
So it is the that you need it all.
And that’s, I think, that’s that’s the mistake we make because because it’s so broad. Right? We we we we we we are technologists, and and we’re proud of it, which is good. Right? I’m I’m I my background is in business, so I really like the business part, and I had to learn the technology part. I’m not passionate about it.
I’m honest. I I really love the the the application to business and creating value.
And I’m I I have to do that technology. I love statistics, but I’m not the the the best friend of, you know, the data management. Right? But I I know I need to do that too. Right?
And there’s, like, but I have my favorites. Right? I have my prioritization, and everybody does because they are so far apart. Right? They also, like, sometimes require different personalities.
Right? So I come from a let’s say I’m a mathematician. Right?
I’m maybe more introverted. Right? And now I have to do transformation and talk to a lot of people that might not be part of my comfort zone. Right? And that’s why we we we we focus on one or two.
And organizations big organizations usually have when we talk about silos, we talk about silos for many, many years. Right? Usually, you know, data silos. But those silos are around departments as well. Right? You have somebody that, you know, does you have the CAO, does analytics, then you have the CDO, does the data, And you have the chief transformation officer, the chief technology officer, and then you have all your BU unit heads, SVP of something.
So and then taking it altogether, you have them all.
So if the relationship for value creation would be additive, everybody would make money if they hire somebody in all those fields.
So let’s so I I love to this idea of the the holistic piece, and I and I wanna come back to those four key pillars.
And and I would really wanna drill down on kind of the Venn diagram that you shared in your presentation, which are the four key pillars that you’re saying you need all four. And if you take one away, something will happen. But let’s let’s double back to one thing that you you talked about, which is kind of some of the things that that define these achievers, these these AI achievers. And one thing I kept hearing over and over again was, okay. This has been a journey. There’s been ups and downs. It’s it’s a it’s not it’s nonlinear.
Right? It’s it’s not like everything is keep going up into the right. There’s been failures. There’s been problems. There’s been more failures than successes, which is natural in an r and d function. Yeah. Very natural in an r and d function.
So that suggests to me that perseverance is is a is a big key here, and it and it also suggests that a really, really short term focus on immediate returns could do more harm than good.
So did these achievers how do these achievers go go from I need returns in six months, I need ROI in six months to how do they get over that into this this idea of this being a multiyear journey where you probably have more failures and successes? What’s how do they do that?
I mean, it seems like there is a common goal.
When we look at all the AI pioneers and how they talk about AI, and they have done done that for many years. Right?
And we bring that up in the book. They seem to have a common vision. Right? There’s an overlap. They all describe AI very human like.
So they you know, Elon Musk produces robot robots that that look like humans. Right? It’s like that there is some, you know, similarity.
We also talk about, robots in a way that they they make human like decisions or behave human like or they have human like capabilities.
Right? So there seems to be a common final goal, number one. And number two, I think that many of those, AI achievers have achieved value on the way. Mhmm.
So although they had something they were striving towards to some aspiration, they seem to have, incremental value creation on many steps on that way, and that keeps them going. So that keeps them motivated. And we compare it to a marathon. Right?
So we have the final, you know, finish line in in in in in mind, but there there’s no we keep running because we have little little wins on the way. Right?
And I think that’s that’s a good way a good way of looking at it and, and and a and a good learning from, you know, many of those AI pioneers and achievers, and, you know, and they have they they go through kind of the same steps. Right? Many of them go through a first phase of data driven decision making. Right? Getting their getting their data together, and understanding the value, what they can do with data, to the point that they augment human, decision making. Right? They support human decision making, right, through the relevant insights.
And then the next thing is they go towards exceeding human capabilities.
Right?
Where it’s, you know, where AI does something that humans can’t do anymore. But it it’s the it’s the journey, and there there’s different steps in between and so on. Right? But just looking at those three steps, they all seem to have gone through those.
Interesting. So it’s not just a top down. It’s not just this grandiose vision, and it’s not just a bottom up. It’s both. You’ve you’ve you’ve got you’ve got kind of coming at it from the bottom, short term wins, grand vision, heaven both. That make that makes total sense to me. Let let let’s get oh, go ahead.
And then let me analyze the, the the potential of AI value creation. Right? And, we we talked about it at the conference, and people always ask me, k. Where do I start?
Right? We Yeah. Good question. I start I start with a use case catalog.
Right? I started with my industry use case catalog and looked at the use cases and tried to figure out what’s best for us. The interesting part here is that we talked about that we seem to forget everything that we learned. Right? So when you go back in the eighties, right, we learned from Michael Porter in in economics. And everybody you studied economics and business and heard of Michael Porter. Right?
And one of the things he taught was that, a competitive advantage is critical for value creation. And competitive advantage, by definition, is you have to do something better in, in your industry. Right? You go against your industry to have a an advantage either through differentiation or pricing or right?
But you you you do something different. So when we look at use case catalogs, industry use case catalogs, they they’re they’re really helping us to catch up and understand what the industry is is doing and where we are right now and what they can do or what they’re thinking about. And that most of those cases, you can already buy. You know, you go to Salesforce or you go to, you know, startups, and they, you know, they they they follow those those use case, you know, use cases for an industry because there’s definitely big audience.
Right? It definitely makes sense. It may be even cheaper to buy it than building it. Right?
And on the other hand, if you start building something that’s unique and gives you a competitive advantage, right, through out your organization or whatever your strategy is, right, then you’re gonna you’re gonna realize that this is where you actually create value for your organization, especially when you built it. Right? And then there’s when we talk about those, you know, how how where do we start? Which one do we pick?
Right? We need to understand what AI can actually do for us. And and then how do we evaluate those use cases? Right?
If if we now find something within our organization, there’s there’s two sides to it. First, I need to evaluate if it’s actually value creation. Can can that create value? And second, where do I look?
Right? So if we say, you know, there there’s no way which one we start with. But let’s say we start with the evaluation. Right? Figuring out if there’s actually value behind something. Because having an idea everybody has an idea. Or we need a chatbot.
What do you need the chatbot for? Johnny. That’s exactly where we always talk about, like, the this technology hype and you know? So but if we start analyzing it and look at three dimensions right? And those three dimensions are business opportunity, and that’s already very hard to do and way more complex than people think. The second one is and you talked about RI at the beginning. The second one is feasibility.
Can I actually do the and achieve that opportunity, that business opportunity, what I just thought of? And number three, everybody seems to forget, or at least many seem to forget. It’s the adoption and adoption risk that, you usually have. And then that’s, like, where because that comes at the end. We we always seem to forget that piece. But how many dashboards have you built in in as a data scientist or, you know, now you’re a CDO or CDAO, but you started usually somewhere, on the lower end. How many dashboards have you built that nobody used?
Right? And how many how many things have you built that that just went into the nowhere?
Right? And that’s like we need to learn from that part because that’s value.
It’s diminishing the value. Right? You can be and I always thought it. You know, I made a PhD and MBAs and all the degrees and coming from academia, right, going to industry. I was like, I’m the smartest guy in the room, but I failed so many times.
Wait. Wait. Wait. Maybe I’m not the smartest guy in the room. So what what do I do wrong? And that’s where I realized it’s not about how smart I am.
Certainly not. It’s about how do you build something that has a business opportunity that I can actually achieve with the feasibility?
And number three, how can I make it that actually people want it? How can I give people what they want?
And and I think from thinking from that perspective, you you quickly realize that there are many things we we have done. Maybe we focus on one or two of those, but seem to forget number three.
And so and and we can talk about examples here. Right? We can talk about, like, business opportunity. I would really like to bring, bring up in the in the insurance industry, an example, and we write about in the book.
That’s, insurance churn. Right? So leaving the insurance company going somewhere else. You know?
And let’s go to car insurance. Right? They have, like, twenty percent churn rate. That means on average, every, you know, five years, people leave the insurance company.
Now if you think about it, you have a billion dollars in premium, twenty percent churn rate. You know, you think you can save two hundred million dollars with an AI that does churn for you. Right? And it sounds logic. Right?
The guess is the churn, you mean? Like like, says this person’s at risk of churning.
Yeah. So, but the but that’s not where it ends. Now first of all, the AI is not gonna be a hundred percent correct. Let’s say it’s between eighty and ninety. Let’s say it’s ninety. So you’re already down to a hundred eighty million opportunity. But what do you do next?
You you have to do something about it. You know? Just knowing who’s gonna leave is not helping you, so you have to take action. What did the what is the action you would take?
Well, I’d probably deploy some sort of, you know, save type campaign, maybe hire a bunch of call center agents, maybe push it down to the individual, you know, whoever the agents are in that market and say, hey. Your customer is about to churn out. Call them.
Yeah. And so let’s say you have a call center. Right? Yep. You call your, your customer, and how many are gonna pick up?
Today? Probably very few.
Let’s say a third. When you’re lucky, it’s a third.
Oh, they would beat me lucky. Yeah.
And it’s already down from a hundred eighty to sixty million.
So and what happens next? They pick up and you say who you are.
How many are gonna talk to you if they already know they’re leaving or You know, you you are dissatisfied.
They’re dissatisfied with the product. Yeah.
Yeah. So they let’s say a third is actually talking to you. So from sixty, you’re down to twenty million. So and then what are the two they they actually wanna talk about the problem they’re having. So what are the two problems they’re usually having?
It’s the product or the price.
Right? Yeah. So you can’t change the product because you only have one product as an insurance company usually. And number two is the price, and it’s a regulated price pricing market.
Right? You can’t just give them a thirty percent discount, at least not here in the US. And, you know, you can give them a toaster for thirty bucks, but it doesn’t really make up for the three thousand dollars you pay in insurance. And so taking all that into account, there’s nothing you can do about it.
And the project’s gonna cost you two million dollars because you have to call them, you have to build it, and all those other things. Right? You you just calculated two hundred million, and now I we get to two million dollars in loss.
How could that happen?
Right? And that’s like the business opportunity is not always how it looks. Right? I have barely seen you see that very often that what we think it is because we don’t think it end to end.
And, really, like, the actions we take and all the risks on the way to really make it a business opportunity, it usually turns out to be way lower. So that’s that’s number one. It’s the business opportunity side. And then the next one is the feasibility side.
Right? Now I actually have to build what I just promised. Right? And I my favorite example here is always Siri.
Right? So you you look at Siri, and I always ask people, okay. How many of you have Apple? Yeah.
Their hands go up. Right? How many use Siri on a daily basis? You know? You see two out of fifty people still say something, right, or five.
And how many people use it as the best friend, as your assistant that you use, and you don’t need anybody else, and then everybody hand everybody’s hands go down. So but that’s what it that was what promised. That was the business opportunity that was created. Right?
And it doesn’t, you know, doesn’t catch up to what what was the expectation that that were created. But on the other hand, you have, like, Amazon that sells Amazon Echo, and you have thirty percent penetration rate in the US. And many people use Echo, but none as their best friend, you know, to say, hey.
Please switch on the light here and, you know, you know, go on Spotify and play song b. Right? But this is, like, where what’s feasible. Right?
So it they never promised it to be your best friend and replace everybody else. Right? I mean, I I’m exaggerating. Right?
But it’s like it’s it’s the it’s the alignment of what is actually feasible, to what what, you know, what the business opportunity is. And number three is how many people do you impact in in which way? Right? If I have to if I implement a use case within an organization that is supposed to implement a thousand people and it changed dramatically how everybody’s working, there’s a huge risk.
Right? And that’s that’s number three is where we always seem to forget. You know? You have to change how people work.
Right? Because you want them to use whatever you built. Right? The the the challenge is we need to think about it from a different perspective.
Why would they want to use it? Yep. Right? And that’s where where we also need to think about what we always forget.
It’s like there’s three stakeholders we usually have, and they they can be more. I mean, I just you know, there’s a couple others. But in general, you have the organization itself and their shareholders. Right?
And we always try to focus on that. You know, that’s the business opportunity part. Right? And they should be happy if I make them money.
And then you have the you have the customers of the organization. You try to, you know, create value for them or at least not impact them negatively. And then we always forget the employees. And we’re always saying, okay.
Yeah. We just tell them they have to do it.
That’s not usually how it works. Right? You you don’t go and say, yeah. Okay. Here, marketing department, I just built your solution.
Everybody has to use it now. Good luck. How many times has that worked?
Especially when we think about, you know, dashboard technology. Right? We have adoption rate of less than thirty percent according to the numbers. Right? But it’s, like, seventy percent is done for nothing.
So how can that be after twenty years, right, since we have that technology?
So and then you think about those three factors, and realize, wait. There is more to it. Right? And then, k.
Good. Now we know how to evaluate those use cases, and then the next step is, like, where do we find them? Where do we find use cases? How do we start?
Right? And, in general, like, there’s, let’s say, four areas we categorize roughly in the book, and there’s certainly more or less how we wanna define it. But four that seem to be good to look at because they have a different profile on those three value factors. Right?
So number one is, like, business process optimization, which is usually very simple low hanging fruit if you have business processes mapped and you have some standardization in your organization.
Then, you know, it there’s a process.
You have steps. You have actions, and you just try to optimize them a little bit.
But in general, it’s there’s some some value. Right? Some business opportunity, low risk. Right? And then you go and you do decision making. And first, you start with decision making augmentation before you get to automation.
And augmentation can have a huge opportunity already because you give something insights to people that they haven’t known. But with that, the risk increases, the feasibility, and the adoption risk increases because you change what people do. And then everybody’s scared of automation.
Right?
So you need to make really clear that it’s augmentation and not automation, and you need to make sure that people actually, you know, want to work with you. And you need to address that elephant in the room. Right? It it’s really hard to do, and we usually don’t.
Right? So we’re just like, okay. We build something, and then we expect people to to to use it. Right?
And that’s the that’s the other thing. Right? How many times I you know, when I thought I’m the smartest in the room, I built you a solution, you know, fulfills all your needs. And I have them never asked them before if they actually want it or how it should look like or you know?
Just go there and say that here it is. Right? So that usually doesn’t work. And then if you try to automate things, and that becomes more complex and complicated, especially from a feasibility and adoption perspective.
Right? Let’s say, from a feasibility perspective, before, I just give you an an insight that needs to be correct, but an insight and you make the decision. But here, I take decisions. I make the decisions on behalf of humans, which has, you know, way more risk to it.
And plus, what do you do with the humans then? And so as soon as and, you know, people always ask me, yeah. But what do we do then? And he said, yeah.
Don’t fire them.
Why why not? And he’s like, because then nobody else wants to work with you anymore on the topic of AI. You just fired fifty people, and you have a ten thousand people organization. You’re kind of dead in the water after that, right, because everybody is scared.
So it’s like you need to find a way of how do you partner with the technology in the long run.
Right? And you need to create trust. Trust is one of the most important topics for for adoption. And then last but not least, AI products and services.
And everybody wants to build products and services, but that’s the hardest thing to do because it needs to be scalable. You need to have a product that actually fits the market needs perfectly that you get, you know, interest from the market. And then you need to build more around it. You need to build the marketing, sales, and so many things that if if you’re not familiar with it, you you’re gonna struggle.
Right? And there are more pieces to it. Right? Talking about security and then, you know, legal and other things that, you know, you need to think of if you build AI AI in products and services.
But in general, I would say those are the things when we talk about, the potential of value creation. So I know it was a very long answer.
Well, it well, it was long enough to support a book. So, I mean, you know, obviously.
I it’s interesting. What you just described, from from the perspective of the feasibility of of marketing and taking a product to, you know, taking your product to market, I would argue is why most data monetization efforts fail is because data people don’t do that stuff very well. They don’t know marketing. They don’t know they don’t know product management.
They they don’t know competitive analysis. They don’t know a lot of these things that are required to take a product to market, and it makes it a lot harder. So in our last few minutes, I wanna get back to the the four legs of of the value creation stool where what what you say in the book and and the case that you make is if you’re not doing all of these four things, you do you failed to do one of them, you’ll fail to do all of them. So in our last few minutes here, go into detail on what those kind of four pillars value creation are.
Right. We they all line up, and you can find them if you think about it in those three higher categories of business opportunity, feasibility, and adoption. They all go in the same direction. Right?
So you have, number one is business. Right? You need to be business focused. Right? And you need to understand the business business domain and where you can where AI has the capacity and the capabilities in order to support business.
And there, AI comes in, and that’s the, usually, the algorithmic, right, the algorithmic part. And you need to support it with the technology. So that’s number three. And then you need to have the right data and the data management, and data needs to have the right characteristics.
And number five, that’s the that’s the organizational psychology or the the closely related to adoption. Right? Building trust and understanding stakeholder motivation.
And if you build a tool, that is wonderful, but it actually reduces the salary, the variable part of the salary of the people that are supposed to use it, there’s no way that thing’s gonna be successful. So you really need to think about why should somebody actually use the tool, and you cannot use the features. You cannot go and say because it’s the smartest chatbot, because I just don’t care.
Because how does that help me? So how does it help me to do my job, and how does it impact the outcome? Do I have, Do I save time?
Do I have a higher salary? How does it all come together? But eventually, it’s those four or five factors that we all need to focus on in order to drive value, and all of them are important. So now you can play the game and always take one out.
You know? You have a lot of fight. You know? And you realize, okay. You’re gonna fail.
And you, you know, either you don’t have you don’t have business applications or you don’t understand the full business, so the tools are gonna fail. Right?
Or you you don’t you don’t do, the the psychological part. Right? So the the the change management and work on work on that part, you know, you’re gonna fail too. We just talked about it.
Right? Because nobody wants to use it. Or from a feasibility side, right, you you don’t know how to do technology and that thing, you know, fails to open and load and, you know, all the time because you were not prepared, then that’s gonna fail. You know?
The algorithmics, right, it it’s not accurate. It doesn’t really work how we promised it.
It doesn’t fail. You know? It’s gonna fail, and then, you know, you don’t have the data. You don’t have any data, or you don’t have the data at the right moment, or your data is not accurate, you know, then you’re gonna fail too. Either way, you’re gonna fail. So it you need it you need it all. And that and that’s a challenge where, where we have to, you know, kind of anticipate the the problems on on the way to to value creation.
And when when we start and the the book is really not about the the how to do Right.
You know, how that’s how you have to do it. It’s it’s really more about, okay. Those are the failures we’ve seen and we’ve made ourselves.
Just try not to repeat them. And then, you know, the less failures you and errors you you make, the more successful you become over time.
Right? Then, you know, we talk way more. We have we have a whole chapter, a whole part of the book, part two, about overcoming those challenges. And then we have, a part three, so where you have in a part two, you overcome all the challenges of one use case.
So how do you integrate it and scale it through a organization that has, ten thousand, fifty, hundred, two hundred thousand people? How do you scale an AI initiative? What what do you need in order to make it scalable within an organization? And last but not least, and that’s usually what we’re talking about, it’s about, you know, the capabilities, the required capabilities.
And when we talk about those, we always focus on technology. But there’s two more, and that one is data management, which we usually don’t like to touch.
And then yeah. I know. But, you know, the and then, number three is the talent. Right?
So we usually talk about data scientists and and the skills of data engineers and or data science, you know, software developers, but we forget the other skills that are necessary. Right? The the, you know, the change management skills, the project management skills, the, you know, the the the management skills of that initiative, the the business skills, and how do they and and, you know, how do they become like, to which roles do they transition. Right?
And that can be totally different between organizations and especially, at the beginning to the middle to the end of an AI journey or, let’s say, the maturity of an organization. Well, at the beginning, you need really full stack of developers that can go end to end and, you know, you try to find that golden egg that the people that can do everything, right, to the point that when you go and look at the big achievers, they have a thousand people. They are in in small little buckets and and and are linked to each other. Right?
One has a very specific narrow job which we try to optimize. And is that the best approach? I don’t know either. So it’s like, it must be something in the middle because it at the beginning, it it’s hard to get those golden eggs, and it’s it’s really hard with that very specialized and narrow, system that’s created when organizations are more mature to to adjust or to be flexible.
So there’s has its, you know, advantages and disadvantages.
Well, so you heard it here first, CDOs.
Not only do you have to be good at, you know, business and understanding the business, understanding, you know, potential value you’re gonna deliver, you also have to be good at technology. We already knew that. But you also have to be good at kind of some of these kind of interpersonal issues. And that, to me, Michael, that’s the really the the big takeaway for me is is understanding kind of some of the political environment that you operate in, understanding some of the kind of the interpersonal issues.
Power dynamics are, like, are are even are even relevant here of, like, what will building this AI based solution do from the perspective of me being perceived as a threat? Like, if this is if this is meant as a way to automate jobs. Right? Will I be perceived as a threat?
And if I’m perceived as a threat, it could be the you’re what you’re saying is it could be the greatest AI ever made, and it doesn’t matter. It’s not gonna be adopted, and it’ll just sit on the shelf and and rot. So I really appreciate these these perspectives. Thank you so much for sharing them with our community.
If you are not already subscribed to the podcast, please consider doing so. Michael, thank you. Really appreciate you coming out.
Yeah. It was wonderful. Thank you very much. And, Nisha, Will, and me and, all the contributors, we we really like to learn.
We’re very curious. So if somebody has a different opinion or a different examples or, has a different experience that they would love to share with us. We we would love to learn, and see you know, it’s a moving. It’s a dynamic field.
So we’re very excited about the feedback and people that come and, you know, we’re you can reach us on LinkedIn, and, there’s a website to the book and, you know, be welcome to, you know, contact us and tell us what you think and help us to improve.
Check out Secrets of AI Value Creation available through Wiley on it’s available through Amazon dot com. I definitely recommend it. Again, thank you so much, Michael, and I hope to see everybody on another episode of the CDO Matters podcast sometime very soon. Thanks all. Bye for now.
ABOUT THE SHOW
How can today’s Chief Data Officers help their organizations become more data-driven? Join former Gartner analyst Malcolm Hawker as he interviews thought leaders on all things data management – ranging from data fabrics to blockchain and more — and learns why they matter to today’s CDOs. If you want to dig deep into the CDO Matters that are top-of-mind for today’s modern data leaders, this show is for you.
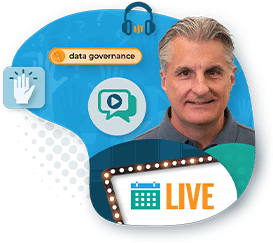
Malcolm Hawker
Malcolm Hawker is an experienced thought leader in data management and governance and has consulted on thousands of software implementations in his years as a Gartner analyst, architect at Dun & Bradstreet and more. Now as an evangelist for helping companies become truly data-driven, he’s here to help CDOs understand how data can be a competitive advantage.
Facebook
Twitter
LinkedIn