Episode Overview:
In this 38th episode of the CDO Matters Podcast, Malcolm interviews Mark Stouse, the Founder and CEO of Proof Analytics. An accomplished business leader with decades of experience in leading data science initiatives, Mark shares his perspectives on the growing frustration that many organizations are experiencing because of an inability to realize significant benefits from investments in data management and data science.
The risks of CDOs saying they aren’t ‘ready’ to provide business value from AI and data science are real and increasingly unwelcome from companies looking for more immediate returns from their data teams. This is producing an immediate need for CDOs to be unabashedly lean, with an unrelenting focus on a very limited number of specific business needs and outcomes.
Episode Links & Resources:
Hi. I’m Malcolm Hawker, and this is the CDO Matters podcast, The show where I dig deep into the strategic insights, best practices, and practical recommendations that modern data leaders need to help their organizations become truly data driven.
Tune in for thought provoking discussions with data, IT, and business leaders to learn about the CDO matters that are top of mind for today’s chief data officers.
You are. We are live and recording now, by the way, Mark. So that that was the, hey. Go let’s go live on stage and, so we’re we’ll we’ll we’ll we’ll learn as we go here. But No.
That’s awesome. Yeah. Let’s let’s keep it down. Let’s keep it maximum spontaneity. Right? That’s it’s Friday.
And That’s right. We’re we’re being fully agile here. We we just did the MVP of prep for the podcast recording, which which was talking about, you know, Texas and the oasis in Austin and Mexican food and the weather in Florida and the weather in Phoenix.
But, anyway, without without further ado, hello, everybody. I’m Malcolm Hawker, the head of the head of data strategy with Prophecy Software and the host, the head and the host, of the, CDO Matters podcast. I’m thrilled that you are joining, us today in whatever way that you you do that, whether that is through one of the podcast providers or whether it’s through YouTube or whether it’s through prophecy dot com, you name it.
Thank you for listening. Thank you for subscribing, and thank you for supporting this growing community of data leaders and chief data officers. My goal is to extend the tenure of senior data leaders, extend the tenure of few data officers, and my sincerest hope is that you get value out of these conversations.
Absolutely.
Today, I’m joined by Mark Stause. Mark is the CEO and founder of Proof Analytics.
I’m looking forward to learning more about Proof. I’m looking forward to learning more about what you do. I’m looking forward to picking your brain.
You So so let me let me just say this. Let me just say this. Yeah. This is where I screwed up by pushing the red button too soon. Right? So it’s Stuus.
Stuus. My apologies, Mark.
No. Not at all. Not at all. Yeah. I just, I’ve yeah.
I’m just Well, here’s the here’s the thing here’s the thing about, like, making friends online.
And and and we have made a friendship online, and I I’ve I’ve probably traded I don’t know how many how many exchanges with you on LinkedIn over the last year and a half.
And we’re into the point where I kind of know that guy. I know but but I can’t even pronounce your name because I never had to.
So No. I think, hey, I think that’s totally where we all are, you know, and and that’s just a that’s reality. And for whatever it’s worth, okay, my last name is Alsatian.
And so if you’re on the German side, it actually is Staus. And if you’re on the French side of the border, it’s Stus.
Interesting.
Like, how like, how many generations removed? Like, is that is that relatively recent? Are you second or third generation? Or is it go way back?
So the Stu’s family, my ancestors, from that branch, anyway, came came from France in the eighteen fifties Alright. Okay. To Nauls and New Orleans or well, but I say Nauls. And and, you know, then I have two other branches of my family that came much earlier, seventeen twenty ish.
Right? That kind of thing. Right? So, I mean, yeah. Alright.
It’s so it’s it’s it’s not, like, immediate. But No.
Definitely not. Not recent.
Fair enough. Doesn’t have an awful lot to do with data, but we’re getting to know you, Mark Stu. So so thank you for correcting me, and thank you for for for the story.
No problem. Really have much of a set agenda today because I know your your depth of experience is broad. I know your subject matter expertise is broad. I know your opinions are broad, and I wanna stay fairly broad. But we’ll see where the conversation goes today. Why why don’t we just kinda start with some of the basics? Because I was doing a little bit of homework.
I I would like to know more about proof. I’d I’d like to know more about MRAM, marketing relationship management.
I consider myself a Resource management. Yeah. Yeah.
I I consider myself a pseudo expert on CRM, and and have been supporting and living and breathing in that world, but would love to hear what makes things different, in the MRM world. But, of course, more specifically, I I’d love to deep dive in and around analytics, in and around data science, in and around some of the things that you’re that you’re working on, conversations you’re having with marketing leaders day in and day out. I I think our viewers and our listeners would be extremely interested to hear kind of the voice of marketing and what’s going on in that world. We’d love to we’d love to poke on that a little bit more. So does that sound good?
Yeah. No. Absolutely.
Alright. MRM, tell me more.
Yeah. So the proof is a startup, a scale up now, that is, all about operationalizing analytics at the speed of the business, which my personal experience as a analytics led decision maker in large companies and a champion of that for better part of twenty years, That’s one of the big takeaways that I have is that people don’t see the value of data science and analytics a lot, because of this issue. Right? The the latency issue is a real problem on data science.
It’s not decision support at all because it’s pretty much a day late and a dollar short every time to the party.
Scalability, cost, understandability.
Right? All of this kind of stuff is, not to mention a rather large cultural difference between kind of a cult of precision on one side and a cult of pragmatism on the other.
So when we we were so I’m still very much like a CMO of Honeywell Aerospace, that kind of role.
And we’re working on all this. And we had already gotten past, you know, a lot of the issues around, like, math and things like that. Right?
And so this became much more you you know, you get to a point where you start to really understand that the the questions or the problems that you were seeking to answer or seeking to solve really weren’t there was a whole layer of problems and questions below that that were kind of, like, far more existential.
And that was what I just said, right, about latency and scalability and and operationalizing analytics and all this kind of stuff. So we had to do it at Honeywell. This is ten years ago. We had to do it by brute force.
Right? So particularly to get the latency down on the re the model recalculation so that it was it stayed relevant, we had to overhire massively on the on the data science, data analyst side to the point where we were spending eight, nine, ten million dollars a year just on marketing or go to market analytics.
You you know, nobody ever complained about that expense at all because the value was so recognizable and so real.
But you don’t have to be a rocket scientist to figure out that the number of companies, even large companies on the face of the earth that are willing to spend that kind of money just in one part of the business, right, is thin.
And, and also, you know, I mean, we were we we did great, but we were absolutely at the outer limits of what you can accomplish by brute force.
So coming out of the software industry before Honeywell, You know, again, it was pretty obvious that automation was a big part of the answer and that certain kinds of targeted AI also could really help the situation immeasurably. And so we built a largely, substantially, but not totally, automated causal AI platform tool that is is predominantly, the all the different kinds of regression. Right?
There was a time, when I don’t think regression was considered to be AI. It was you know, and then and then it kind of got sucked into being part of machine learning, which kind of de facto makes it AI, you know. And and then as things got more and more automated in certain areas and self learning in certain areas and all this kind of stuff, right, that, it it really I think it legitimately is today.
Causal AI, you could argue with its proto or whatever. Right? But, I mean, ultimately, who cares? Because that’s we’re solving big problems with this software just like you would with the math itself.
So we built that and and it’s, you know, it’s agnostic, on data source. We put it on AWS for that reason.
Later, we had the opportunity to buy a, a really, really super contemporary, very, very cool marketing resource management tool, which is essentially an ERP for marketing. So if you’re familiar with ERPs, it does whatever ERP does for particular functions. So we’re talking about planning and budgeting and compliance and asset management and all that kind of stuff. Right? And in our case, it is uniquely, native on Salesforce.
So that part of our business is has a heavy, in fact, even overwhelming bias for Salesforce.
If you’re not a major Salesforce user already, you’re unlikely to become one just to get our MRM tool.
But if you are a major Salesforce customer and you want an MRM tool, you’re very likely to buy ours.
So we then, ported the two together, so that gave everybody a full life cycle closed loop, planning, budgeting, etcetera, analytics tool where the analytics then comes around and informs your next planning and budgeting cycle and, you know, it creates a virtuous circle that thoroughly optimizes everything. And, you know, with the stipulation that, hey, there’s always a hard deck. You’re never gonna drive all waste out. In marketing or go to market, that’s about a hard deck is about five points.
Usually, you so you start out with thirty points of wastage or maybe even more than that. Right?
So spend efficiency of spend on a campaign?
Yeah. Sub optimized or, you know, underperforming, nonperforming, stem, things like that.
This is the, you know, fifty percent of my marketing is extremely valuable. I just don’t know which fifty percent?
Yeah. Pretty much. Yeah. Exactly. But this addresses that.
Or or this this this this helps address with that. Okay.
Yeah. And it helps you because of the the speed of the platform.
Right? It operates exactly like a GPS.
So it is I’m I’m being very conversational in the way I’ve been slinging these terms around here. Okay?
But It is It’s effective for me, by the way. So yes.
Keep the conversation. Thank you.
Right. It’s, it’s it’s kind of self optimizing in that sense. Right? Because we just kind of take classic regression. Right? So it’s looking at the history. It’s giving you a stack rank of essentially the greatest contributions against a particular dependent variable.
Right?
It’s like giving sales or, like, something moving through a a marketing funnel or a conversion. It would only be anything. Anything. Anything. Anything.
Actually, our we focus it a lot on the go to market space as a business, but it’s a completely agnostic platform and some of our customers have figured that out. So they’re using it for all kinds of things. Right? In fact, when we do a major product update on the analytics side, we use health care data with a known outcome.
To me to test.
To test. Yeah. So, so we are, and one of the reasons why we do that is that, there’s no bias, right, in that as far as the system is concerned because it’s such a major shift. Right?
And so, you know, the whole goal is it’s got a, you know, post update.
Right? It’s got to generate the same answer that the other guys got, right, out to three or four decimal places and, you know, at that point, it’s beyond good. Right?
Well, I mean, we’re not using it in life and death kinds of circumstances here. So, so, I mean, it it, it it really keeps everybody in the know, about what’s working, what’s not working, the effects of time lag, which are, you know, routinely ignored, in in business, but just because they’re not really understood and nobody has really systematized that.
You know, two thirds, roughly seventy percent of any given model, situation is is externalities. So that’s really emphasizing that. That is part of what AI, does in the from a prompting perspective is, hey. You know, mister modeler, don’t don’t forget, you know, the externalities, and have you thought about this one? Have you thought about this one? Have you thought about that one? Right?
And so it it, it keeps it you know, it it keeps them on an even keel.
And then the last thing I’d say is that you’re able to we stick with the GPS analogy. Right? So you’re cruising along on the route that you’ve chosen, and all of a sudden, externalities intrude, things have changed, lots of volatility, maybe there’s a wreck ahead, you know, whatever. Right? And there and so what you were doing, which was right and which was optimal at the time, is now not.
And so just like you your GPS would reroute you, give you different choices on rerouting, proof will show you, hey. Here’s some different ways you can war game this, statistically to get back on track and do whatever you need to to do. And so you can do that, look at different scenarios, find the one that works best for you, find the one that, has the business’s support from a funding perspective if that’s necessary, and then lock it in and go. And so that is, that’s really it. I mean, it we’re not doing anything that a normal data scientist doesn’t totally understand and do on a daily basis and, like, state it or whatever.
We just do it a lot faster, a lot more scalably, a lot less expensively.
You know, this is sort of like, you know, a long time ago I don’t know. This is, like, almost twenty years ago.
When I was involved with data center automation, there was a lot going on in a data center that was very necessary, very unsexy, but had to happen.
Right? And we automated that entire layer of stuff, allowing everybody in the data center to kinda take a big step up in terms of what they were focused on and add more value. And, actually, very few people lost jobs to DCA.
This is sort of very, very similar, in in the analytics space.
You know, everybody wants to do all the cool analytics, and, and I get that totally.
But there’s a reason why regression in all of its forms is still eighty percent of the cornerstone of DS, particularly in business.
Well, that makes sense to me. I mean, if you’re if you’re establishing causality, I mean, that’s that’s that’s that’s easily half the ballad the battle of predicting future behaviors. Right? Like, no the past is not always indicative of the future, but if you establish causality with with with relatively decent statistical probability, I think you could you could roll the tape forward. Right? That’s basically why we’re too worried.
I mean, and and and I think we all have to just remind ourselves all the time. Okay? We live in largely a probabilistic world. Right? Outside of certain, natural laws like gravity, which was totally deterministic.
Most of what we encounter on a daily basis is about odds. Right? And which sort of brings us to the next big thing, I think, and that is why what is the freaking problem between business people and data science people?
Right? And and Continue.
I I I don’t know.
Well, I is that a rhetorical question?
Because because I’d love I’d love to poke a little bit more. Yeah. No.
I I deserves serious poking.
Right?
You you you used the phrase earlier, the cult of the pragmatic versus the cult of the of the perfect.
What for a second?
Siri wants to help. Yeah. Siri wants hey. Siri’s got an answer. Maybe we should ask Siri.
What’s what’s what’s the what’s what’s the problem with this I messed up or happened to me.
That’s hilarious. That is. That’s weird. Me.
Why would I say it?
I don’t think it Yeah.
Yeah.
So her up all the time, actually, inadvertently.
I don’t know why. Maybe I got a list or something. I don’t know. Anyways, you were you were saying the the problem between data science and business people.
So if we look at the way that data scientists are taught and acculturated, it’s a very academic perspective.
The the ninety five percent confidence score, okay, is a great example of this.
The problem the problem is is that as soon as you start dealing with data that represents predominantly human behavior, which is most of business, the ninety five percent becomes a moot point.
Right? Like, if I mean, you’re gonna be lucky a lot of times to be in the fifties. Yeah. Right? So most data scientists will do one of two things. They will either say, well, that’s worthless, or they will overfit the model to get to the ninety five, thus introducing their own set of bias into the outcome, Right? Which is non transparent to the business user.
So you’ve you’ve got that kind of whole thing. You’ve got you know, it’s manifested also in an obsession with complexity, an obsession with, really, you know, stuff that doesn’t really matter to the business at all.
You know, parenthetically, an example of this is eighteen months or so ago when the world melted. Right?
A lot of c suites that I’ve interviewed on this have, you know, they turn around to their DS teams and they said, okay, guys. Guess what? We know that you’ve been spending all kinds of money and all doing cool stuff, and now we you know, this is your time, and we need your help to help us understand what’s going on. And the answer that many of them got back was still working on the data management thing.
Right? And that that just really pissed off c suites all over the place. Right? And so what is happening now, more and more and more DS teams are being pulled under finance, not because finance believes that it can teach DS anything about DS, But because they’re going to do to data science what they did to enterprise IT teams twenty years ago, they are going to compel them into being t shaped. They are going to teach them what’s important to the business and what’s not important to the business.
Right? So what is important and I actually learned this the same way the hard way. Right?
So you gotta understand that from the business leader perspective, they are used to making all kinds of decisions that if you were to model them after the fact, and I have, I did that at Honeywell, They’re routinely in the high teens, low twenties.
Meaning meaning of driving desired outcomes?
Of confidence.
Okay. Okay. So meaning, I’m gonna make a decision, but I’m only I’m only twenty percent confident that it’ll actually do what I wanted to do?
Yeah. But they don’t even think about it that way. Okay.
Oh, okay. The twenty percent is the the ex post facto.
You Well, I mean, it’s not modeling it at all anyway most of the time.
So Okay. No. They’re not. It’s a gut decision. Right? Yes. But that’s where that’s where it comes out.
And so when you expose them to analytics, they’re like saying, man, if you can get me in the forties, I’m a hero. Yeah. I mean, I mean and and and so why is that? It’s because business is all about the law of compounding.
This is something that Bill Schmarzo and I talk about all the time.
Right? It’s all about the laws of compounding. And if you know, a classic example, this is actually a bill example of this, right, is that if you are a business leader somewhere in bowels of some company and you have to make the same decision every morning for three hundred and sixty five mornings, and all you’re capable of doing with the analytics is being one half of one percent better each day than the day before.
The compound value annualized on that is bumping two thousand percent.
It’s huge.
Okay? That’s all they care about. That’s it. Right? They’re not try they don’t want DS, which they would classify as decision support, not data science.
Right? They would they they don’t want DS to say, with great precision, but lots of caveats, right, that this is going to happen nine months, three weeks, and two days from now with ninety five percent confidence. Right?
They don’t want that. They’re not interested in that. That’s not what they’re getting.
So so the the cult of perfection in this case, in your metaphor, was the data science team looking for this this nirvana that arguably doesn’t even exist of of perfect data management and perfect data quality and no wrangling ever, and all data is naturally structured and semantically consistent and this this nirvana that that will never exist, that’s the perfection, the pragmatic that you that you that you’re talking about is that, hey. Just give me one or two percent.
Yeah.
I mean and I think we also have to really say with great clarity, right, that we live in a world full of numerator denominator relationships, which obviously the denominator API was.
Yep.
Right? And data science will never, in a business context, be the denominator.
So data science is gonna have to adapt and move to meet the business where it is because the business is not going to meet is not gonna change to meet you. It’s not happening. Right?
So if that is your big expectation, get out of business, go back into some sort of scientific or academic data science kind of application, and leave business behind because that’s just not you’re gonna be frustrated forever. You you will hate your career.
So the numerator being cost of units sold, number of units sold, something.
Right?
What you’re basically saying is is that business KPIs are are are the creative endeavor.
And and and you as a data scientist aren’t going to create them, and you’re not gonna you’re not gonna convince the business that there’s some other yet un unknown or, you know, to be known KPI that they need to be driving the business off of. You’re not gonna do that. You just need to understand. As a data scientist, you need to help understand and predict causal relationships that exist that are maybe known or or or even more valuable unknown, and and do that in a way that is fast and scalable and doesn’t take three months in order to produce a model to generate. That’s what you’re saying.
Yeah. No. And I and I think, you know, what I try to do assiduously, but, obviously, I’m still human, is I don’t I try really hard these days not to speculate.
Right?
So what you’re hearing from me on this is the product of a lot of conversations, a lot of personal observation, a lot of personal interaction over a course of my career.
Right? It’s it’s as close to a representation of reality as I can get right now.
It is so I’m not I’m not not I’m not engaging in thought leadership here. I’m I’m just more like a reporter.
These are qualitative insights.
But so so so I get it, as as are mine, frankly.
Yeah. But you are talking to a lot of CEOs. You’re talking to a lot of CMOs, as am I.
And you kinda put all this stuff together and you start to triangulate. I mean, we do have to be mindful of of of thought bubbles and, you know, kind of self reinforcing thought silos, which certainly exists out there. They certainly exist in social media. But something that you said earlier, you know, about moving data science functions under CFOs, I just saw a post earlier today from, a friend of mine, Carl Winterbottom, over in the UK where where he’s seeing and talking to people where they are seeing data science functions being moved, under CIOs and taken away from CDOs, which would which would naturally kind of lead one to conclude that CDOs are increasingly getting penned into, you know, classic data and analytics and data governance corner and the arguably potentially higher value add and more transformational stuff, is is getting moved in in other places.
And I think what you describe and and and what seems to be happening based on the conversations I’m having and others are having is is really kind of a a I hate to use this kinda this sounds a little bit trite. But kind of a put up or shut up moment for CDOs when it comes to a lot of this stuff.
Like Oh, I I think that that’s totally true.
Okay.
I think that I think that is, that’s actually exceptionally well well said. Right? I mean, it it it it it is. It’s it’s both an acknowledgment of the power that data science can bring to a c suite, even if they don’t understand it.
Right? They they get it. Right? There’s they they are constantly surrounded by messages about that from their own peers.
Right? So they get that, and then they that’s held up in contrast to what they see from their data science team, which is and from, again, this is from their perspective, from the business perspective. They see data science as a giant boondoggle of a science experiment.
Right? They see data management systems and all their complexity as roads to nowhere if when they say, hey. Can you help me with this, analytical problem understanding the situation better decision? And the response is, well, no.
We’re not ready to do that yet. Right? We we’re still working working on data flows and data supply chain, right, issues. And they’re like, the hell you say?
Right?
So, I mean, this is this is a, not the first time that a function has behaved like this and thus gone you know, been drawn through the eye of the needle, as a result.
The if you know, regardless of where they’re putting data science organizations today, whether it’s finance or IT or, you know, ops of some kind or, you know, it’s it’s I guarantee you one thing is the great commonality.
So I’m having a Hadoop flashback moment here. Like Yeah. I’m I’m I’m old enough to remember and actually have been in an IT leadership function where we were involved in spending an awful lot of money on Hadoop.
And at the time, it was it was interesting because a lot of companies were were making that shift to get off of commodity based systems. Like, they’re they were they were getting off a very expensive kind of, you know, infrastructure stacks, like the like the like the Oracles of the world, the Terradatas of the world, and the others of the world in getting on to more commodity kind of, you know, back end systems. And that was part of the driver. But another part of the driver was this dream of Hadoop and and big data. Right? And I’m seeing a lot of very, very similar things. I’m seeing a lot of companies invest in a lot of money in answering questions that nobody’s asking.
And and and that that was the to me, that was the biggest knock at the time. It’s like, yeah, some of the insights are unbelievable, but does anybody really care that there’s a strong correlation between eye color and career longevity?
Right? Like or whatever. I mean, you you you pick pick anecdotal insight that was being generated at times. So it it seems like we may not necessarily have learned our lesson there, or maybe we are.
From your perspective, obviously, you think one of the one of the things we need to be focused on as as data leaders, and I think this would be true for data science function or just a general data and analytics function, is to be more what you’re calling t shaped. And and for those who may who may not know, this is kind of like depth of expertise. So so is is it are you broad or are you deep? Right?
Broad, it means you’re a generalist, and deep means you’re a specialist. Right? And and what Mark is saying is is that you need to be a bit of a generalist when it comes to business knowledge. I’m putting words in your mouth now, Mark.
You need to be a generalist when it comes to business knowledge and the business drivers and what the business cares about. You need to be very, very shallow and broad, but you need to be able to go deep into your area of expertise, which in this case would be a data scientist or maybe you’re a data steward or maybe you’re a business analyst, whatever. It doesn’t matter. But advocating for more t shaped people instead of what arguably are upside down t shaped people maybe.
Right? Like, where they start narrow and and maybe broaden out at the bottom. Yeah.
The only thing I would add to that, right, is that, a hallmark of a of a t shaped person is that when so sticking with this specific example. Right?
A t shaped, CDO, when they’re with the board or the rest of the c suite, they’re going to talk about analytics and data science using business language.
They’re not going to use any data science language really to speak of because that’s not the language of the of the people they’re speaking to.
Likewise, when they’re with their data science team and they’re passing along business perspective and requests and all that kind of stuff, right, they’re going to interpret all the business stuff into data science speak while still exposing them to the business principles.
Right? So this is as much about being a middleware layer, right, as anything else.
Right.
Particularly for, for business leaders. Right?
Being a facilitator and an enabler. Yeah.
Yeah. So so I wanna run something by you because, obviously, you you you you think a lot about this stuff.
And in in in my world of more, shall we say, traditional data and analytics, which I would argue should also encompass data science, but I I think there’s a general consensus that data science has historically lived in a little bit of an operating silo on its own off over to the side. You you agree? I see you’re nodding your head. Okay.
Totally. Yeah.
One of the the key kind of rallying cries, for lack of a better phrase right now, around AI and around within the broader data community is, hey.
We gotta get our hands around our data, and we need to better manage our data, better govern our data, and do all of the things so we can realize value from AI.
And it sounds awfully like the thing that you’ve got a bit of a concern with in the data science world, which is, hey. I can’t give you value because I I gotta get my hands around all the stuff that leads to eighty percent of my time being wasted every day.
Mhmm.
When I’m I’m struggling a little bit with with with that that premise, not because it it’s kind of like mom and apple pie. Right? Like, to say you you need better if you have better data, your AI is gonna be better. Well, of course it is.
Right? Like like it’s a fortune cookie. Right? Like, of course it is. Better data means better AI. Of of course.
But the the vast majority of people are the vast majority of companies aren’t gonna be building their own models. So so let’s just put that aside. Right? Most of them will be using, I suspect, in the short term commercial LLMs, and they’ll be doing things on top of commercial LLMs.
But if data science teams have always kind of lived off on their own, using Python and and R for data quality and for and for MDM and for for doing entity resolution, kind of hand rolling entity resolution solutions and doing all of this stuff on their own outside of traditional data management, and they’ve been doing it on their own forever and ever, when we say, hey. We need to get our data house in order. Is that I mean, does does that really mean we need to all run out and go buy a data quality tool? Because data scientists seems to have been working for a long time without one.
Do you know I I don’t know if this is I don’t know if this is clear on this.
Yeah. So I think, again, if we’re looking at this from a mathematical perspective, a data analytics process perspective, it all makes total sense.
Right? The fact the fact that you really over rotate on all the key dependencies in the data supply chain and all this kind of stuff. Right? Because, obviously, you know that all that stuff matters to the end game. Right?
The problem is is that in business, you have to earn your way to deeper and deeper and deeper investments, And that means you have to give people more immediate value as the price for being able to ultimately generate other kinds of value that maybe they don’t have yet.
So, actually, the whole thing is inverted.
The the a lot of data science teams have been playing a scalability game instead of a they’ve been playing again, I’m gonna go back to Shmarzo. Alright. He has some great insights. Right?
They’ve been playing an economics of scale kind of game, not an an economy of value, game, or insight or or understanding or fill in the blank, whatever word you like better. Right?
And that’s that’s now proving to be a real super big problem, for data science culturally and and kind of as a profession.
I think we also have to ask ourself you know, we every time I ask this on LinkedIn, I get a bunch of messages saying, man, that that totally nailed it. And yet, I don’t see any changes at all, and that is there’s a huge question about the private, use of AI.
And that is, if you just look at data stocks in fortune one thousand companies, It’s almost all time series. It’s almost all, lean, meaning the data depth is maybe two to three years max. Okay?
What they are measuring, the kinds of data that they have do not fit in any way, shape, or form the definition of big data.
Right. Right? So where is this big data cache coming from to drive these private LLMs, private use of AI? I mean, hey. Let’s just go to the horse’s mouth here. Right? IBM Watson.
Okay? Technically, a tour de force.
Commercially, a total bust.
Right? They’re good.
Why was it a total bust?
It’s because they couldn’t feed the beast outside of academic and medical circles and things like that. Right? So the more regulated the industry, more life and death oriented the the the industry, the bigger the stocks of big data are, and so it works. Right?
But, still, even in those companies, the stocks of data of big data regarding the business of the business are not there.
It’s not there. Right?
So now we’re back to lean data approaches like regression.
So, I mean, this is this is an extremely practical aspect to this, and I realize it’s not sexy or cool or fun or all that kind of stuff. Right? But the reality is is that if you can’t feed it, it isn’t happening. Right? I mean, you can have awesome software, and if and if you don’t have the data to feed it, you have a gun with no bullets.
Well, but yeah. So I think to to paraphrase you, what what I’ve been hearing you say over the last hour is that if you run a data and analytics function or if you’re a data scientist, VP of data and analytics doesn’t matter, and you are telling the business right now, like now, you’re telling the business, I can’t give you value because the house isn’t in order, that that represents potentially an existential risk for you.
No. I think you’re already I think that’s totally proven it. Proving out been proven out is certainly proving out right now. Okay.
That’s worthwhile. That’s interesting.
And and your recommended kind one of one of potentially, I suspect, many. We’re we can only do so much in an hour. But your recommended kind of mitigation here would be to be far more focused on the business, to be more t shaped, to understand the business drivers, to be more lean, to focus on things that work now, to insights that could be driven now and could be valuable now, and don’t get so hung up on building the next chat g p t? I’m I’m kinda paraphrasing.
Oh, I think they obviously, I mean, this you know, I mean, look. Data science is a nonlinear contributor to business performance. Right? It’s very time lagged, all that kind of stuff. So you’ve gotta start certain projects now if you’re gonna have any hope of ever delivering them. But, right, the price of that is you gotta be able to deliver some stuff now. Great example of this, right, is a friend of mine has a distillery.
The distillery is all about super high grade bourbon.
So he has to age the bourbon at least twelve years to even, you know, realize the kind of profit that he wants to realize.
In order to sustain the distillery in the intervening time and continue to make bourbon each year that then goes into the aging process, right, He makes vodka.
It’s his cash crop. Right? You mash it. You distill it. You bottle it, you sell it.
Later.
That’s it. Right?
And that is that’s that’s the approach. And that’s the approach here with data science, you know. I mean, this is gonna sound like super basic and you’re just gonna kinda go, really? Okay.
But sit down with your c suite and say, what are the big twenty questions that if we could give you statistically relevant answers to, and that is fifty percent or even forty percent confidence scores in in business. Okay? We can give you those answers that would be a real value to you right this minute that would help you make better decisions today than you did last week. Okay?
I guarantee you, they do not have any problem rattling those off.
You will have that one very short.
I don’t know a lot of business leaders. I mean, I’ve been in business over thirty years, and I don’t know a lot of people who are running a function. Name one. Manufacturing, procurement, HR, finance. Doesn’t matter. I’ve never met one that doesn’t want more data.
Never. Not not once.
And and so I I I kinda I I I kinda bristle at the idea of being data driven because, like, that the phrase in and of itself, it makes sense, and I get it. And I think we should all aspire to be more fact driven in our decision making.
But but I but I think getting back to your original assertion on, you know, the the cult of the perfect Mhmm. If you’re hung up on perfection or or some idea that I can’t do something today until I get this house in order, and I can never even actually even really define what that means.
Right? Because I don’t quantify the house. Right? I don’t I don’t I don’t know how I don’t even really know how much it costs. I certainly don’t know how much value it it odd it it adds, but I do need to clean it up.
And, again, getting hung up on that, is is is is, I think, just really, really, really, really problematic because, frankly, that’s what people were saying two years ago when the pandemic hit, to your point about the pandemic. And I was working at Gartner. They’d call me and say, hey. My business is asking us.
We need to take a hard left. Supply chains are disrupted. Everything’s gone upside down. And And to your point about what you were saying earlier, Mark, is the data and analytics leaders were being asked, okay.
Where do we go? What do we do? What do we how do we mitigate the supply chain disruption? How do we get more robustness in supply chain?
Or how do how do we change or adapt to changing customer behaviors? Right? And the data analytics folks were saying, I don’t know. Our house is a mess.
So make me a CDO. Give me the title. I’ll get the house in order, and then we’ll we won’t be flat footed in another two years if this happens again.
And I think two years have gone by, and three years have gone by, and now we’re at we’re talking about AI, and the same thing is being said, which is, I don’t know. Let me give me some time to get the house in order.
And and I and I think that’s why you’re seeing a a certain degree of of a frustration on both sides of the equation. And I I just I just love the advice that you just gave, which is pragmatic, which is which is spot on. Give me give me the it doesn’t even have to be forty or fifty questions. It could be five or ten.
Right? Like like, just what what are the questions you need answers to with with with a reasonable greater than fifty percent probability, but not ninety percent? I mean, I I mean, I love it. I mean, it just it just sounds so reasonable to me.
I I I think the other part to this is business leaders, and you see it in the in the in the BI side of life, BI is really starting to kind of I wouldn’t say crater, but it’s going down as a business.
Right? Why is that?
It’s because everybody has figured out in time of great volatility that data is only and always about the past.
Yeah.
Yeah. Right? And so past is not prologue.
Right?
And we can stare at beautifully rendered data vis all day long and, you know, and then we’re tempted to extrapolate and everybody that’s listening to this conversation knows how dangerous extrapolation is. Right? And so everybody has sort of figured out in the last, say, two to three years, okay, that there’s a time and a place for BI, but optimizing spend based on BI is not happening.
Right? You’ve gotta be dynamic and causal and all those kinds of things.
And so and they don’t see evidence that data science is moving fast enough. So, I mean, I hate to kinda end on this note. Right? But the reality here is when I talk to them, when I talk to CEOs and CFOs and people like that, and they’re, like, really being super honest, they are highly energized around this subject.
Like, you hear the f bomb dropped repeatedly in and around the words data science.
Yeah. Right?
And, like, when when a data science team comes in to brief them and they say, okay. This is kind of the situation. This is our answer on this. Right? But there’s, like, in the fine print, there’s, like, a hundred caveats to the answer.
They’re just like, what the hell am I supposed to do with that? Right?
So they see it. It’s been described to me by one CEO, okay, but a Fortune five hundred CEO.
He said my data science department operates a shell game.
I mean, think about that for a second. Is that fair? Probably not. Okay? Is it understandable why he would feel that way?
Yeah. Probably is. Right? So that means the truth is somewhere in the middle here. Right?
We gotta work that out.
The shell game.
Well, let’s hope that underneath some of these shells are in the in the very near future giant piles of money. Because I don’t know anybody that wants to continue to play the shell game endlessly. Under one of the three under one of the three is a giant pile of money, and let’s hope that when we lift that thing up or the next time somebody does, there is a giant pile of money there because we can deliver incredible value in the business. I have no doubt about that.
Data science teams can. Data and analytics teams can. I don’t doubt that for a second. I’ve built a career around it.
Mark, thank you so much for your time today. Really appreciate the insights.
Look forward to connecting with you in the future on LinkedIn. For anybody who’s listening now, please reach out to Mark on LinkedIn. He’s got some unbelievable content and some unbelievable insights that he’s sharing day in and day out. Thanks again, Mark.
Hey. It’s my pleasure. Thanks so much, Malcolm.
Awesome. Have a wonderful weekend, and thank you to everybody for tuning in and listening in. I’m Malcolm Hawker, the the host of the CDO Matters podcast, and I hope to see you at another one of these episodes sometime very soon. Thanks all.
Thank you.
ABOUT THE SHOW
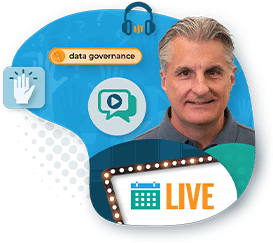