Episode Overview:
As we get carried away with modern data trends and new technical developments, it’s easy to forget the rich history behind computable data and analytics dating as far back as World War II in the 1940s. Luckily, we have a data historian to remind us!
Host Malcolm Hawker sits down with NY Times Chief Data Scientist (CDS) Chris Wiggins to chat about his new book and dive into what led us to this moment in the data space.
The two discuss:
- The ethics behind using data for social concepts
- Quantifying social data
- Early stages of AI and the creation of data computation
- The history of machine learning
- The internet boom of the 90s
- AI vs. copyright laws
…and so much more!
Episode Links & Resources:
Good morning, afternoon, or evening to everybody. This is Malcolm Hawker. I’m your host for the CDO Matters podcast. It is my distinct honor to be joined today by Chris Wiggins. Now this is only a 30-40 minute podcast, so I think just going through your bio, Chris, could consume a decent chunk a decent chunk of the time.
But but I I will I will stay high level here. Chris is, an associate professor at Columbia University, in applied mathematics.
Chris has a PhD from Princeton in mathematics.
Yes.
You are currently the chief data scientist at this publication known as The New York Times. Yes. And is the coauthor of two books that have published within the last month. So this is a very, very, busy gentleman.
One of those books is how data happened, the history from the the how data happened, a history from the age of reason to the age of algorithms. We’re gonna talk about that book in more depth today because I’m excited to go into details of some of the things that Chris and his coauthor shared in in that fantastic, in that fantastic book.
But but the other book is data science in context, foundations, challenges, and opportunities, also that he cowrote. So, that’s a lot to, to chew on from a intro perspective.
I was introduced to Chris by one of the smartest dudes that I know, mister Jeff Jonas, the CEO of, of Senzing, and now I know why.
Because of the connection. They’re probably from the mathematics perspective. There are other connections. But, I I was I was honored to meet your acquaintance. And, with that, Chris, welcome to the podcast.
Thanks for having me, Malcolm. Thanks for making time.
Wonderful. It’s it’s it’s my honor, and I know that our our guests will will find all this interesting. So let let’s let’s dive into to the book, of of how data happened. I found it a a a fascinating read, for a lot of different reasons.
But being kind of enmeshed, you know, day in and day out in the data world, I I think it’s easy to lose track of some how we kind of got to where we are, so I found that interesting. I’m a kind of a natural historian. I I found a lot of that interesting. But I think a lot of the data people see kind of data being a synonym with truth.
Right.
And you you can challenge that assertion if you’d like. But at the beginning of of your book, a lot of what you share is actually how data was being used going back a hundred, a hundred fifty years, in pretty nefarious ways to forward some pretty horrible social concepts.
So so so what can you share about how kind of data was maybe perverted away from the idea of truth, if to the degree that it ever was, towards some of these horrible things like eugenics and kind of social profiling and racially profiling and all these other things.
Yeah. So the the the book grew out of a class, and the class, I like to say, is a class about truth and power. Really, every every class should be about truth and power. So this class was about data, but it was about, like, how data relates to what we think is true, how we decide what’s true, and the role of data in power.
So, when it comes to truth, yeah, I mean, one of the stories of the book or the perennial themes in the book is the way that people feel like if you have numbers, then somehow a fact is more true. Like a qualitative fact somehow just doesn’t have the rhetorical impact of something that has a a statistic behind it or or has big data behind it one way or the other. So part of the goal of the book is to take anyone, whether you’re a technologist or not, and try to understand the limits of that, you know, the limits of the extent to which just because because you have data or even big data behind you, the extent to which that makes your argument true.
Now when it comes to power, a lot of what we do also is to try to situate historical examples that are gonna resonate for people today in which people who have a lot of data also have power. And And and sometimes that relates to truth because sometimes when you have a lot of data, you can say what’s true. But sometimes when you have a lot of data, you can say things like, why I have the data so I can make this policy decision, and I know that this is the way we should enact some policy decision.
So the way that ties into eugenics and all that, is really around the time that making sense of data became mathematical, and it happened to coincide with, like, the end well, I shouldn’t say the end of the British Empire, but basically, the the the waning of the the power of the Victorian Empire. And a lot of people were trying to think about how we can use statistics to try to improve the empire and and make sure that things seemed great. And the way they did it, you know, was to take advantage of the latest ideas of the day, which included statistics, but also, evolution and Darwinian conceptions of evolution.
So the person who really brings it all together is a guy named Sir Francis Galton who, you know, if you’re raised in certain ways, you’ll know his history. If you’re raised a statistician, you’ll just know that he gave us regression and correlation, which is true. He made up the two words, correlation and regression. He also made up the word eugenics. So we tell that story in the book in part, you know, just because we feel like telling not telling the story would sort of be like a lie by omission. But, I think the useful part about it is to say even when you think you’re doing something good for the species or for the society, you really need to think, you know, like, is what I’m doing, like, am I the baddie? Like, am am I doing something that’s really ethical?
And it’s and it’s hard to do that when you’re focused on the tech and how sweet the technology is, to take a step back and say, okay. Well, in addition to this being a sweet piece of technology, you know, how does this comport with respect for persons and, you know, informed consent, or how does this comport with our own notions of of justice or oppression or fairness and things like that. And I think within some people in the in the technical literature over the last couple of years, people looked at that in computer science and other fields, but, you know, the pace of data innovation is so fast. It can be very difficult if if you’re really optimizing for one thing, like moving fast, to also, either save space or better to incorporate into your process some way in which you’re also thinking about, you know, does this respect people’s, right to informed consent, and does this give them, does this comport with our norms around justice and fairness?
Well, one of the things that I was kinda struck by as as as a data person is applying math to some of those things. Right? So so you so you mentioned this this tension, for lack of a better word, that that that would exist between these forces. Right? Government forces and and and corporate forces and and and societal forces. And that’s a that’s a key kinda connecting tissue throughout throughout your book.
But one of the things that you mentioned was, you know, am I operating in in a socially responsible way or an ethical way?
And as a data person, I I found myself in the book often asking, how do I measure that?
And and and maybe this will become a bit of a fractal here is this you know, I’m holding a mirror in front of a mirror, but but is there a way to measure these things? Is is is this something that can actually be quantified, or is that a fool’s errand? And and and we know ethics when we see ethics.
I I think it’s good to think about quantifying it because it’s part of managing is to have some success metric that you you’re keeping track of.
But it it may not be as granular as we we like, you know, like click through rate. That is something that is easy to quantify and you can watch it. Or, like, let’s say revenue, right, or any Wall Street facing metric. You know, those metrics are are, by construction, easy to quantify.
Some of the things that a company might do in ethics might be diff more difficult to turn into a really, a really granularly quantification but still be quantified. For example, how many of your models are launched and what fraction of those models go through an ethical audit or some sort of ethical review? That’s an example of something you can turn into a success metric. It’s not as granular as, like, how many dollars do we bring in, but it is something that you can quantify as a success metric.
Or, you know, what fraction of people’s hours do they spend on ethical review, which depending on on how you wanna run your business could be large or small. How many people do we have who have passed unethical training. I mean, there’s there’s plenty of ways that you can come up with a number. It’s just that the numbers are not gonna be as obvious as the numbers, for example, with units of US dollars on it.
Like, again, if there’s a Wall Street facing metric, that’s usually something where it’s pretty real clear to everybody why that’s a number you wanna track. So ethical metrics are possible. They’re just usually not as granular and directly tied to, shareholder value as, as you’re used to.
So in your book, you you transition away from some of the early stages of kind of the application of of of data into more kind of more modern times, at least within the last hundred years. And and one of the things that you focus on was how government and military, have been responsible for the advancement in a lot of things that we know today as kind of modern data management or modern application of of statistics and and even even artificial intelligence.
I I’m glad you included the the the the touring story.
I I think it would be difficult not to, having watched I forget the name of the movie. It’s it was Imitation game. Yeah. Cumberbatch was was was touring. I mean, perf a perfect touring. Right?
But but some of the depth of the story there, I really appreciate appreciated and how important that that whole effort was was towards kind of modern artificial intelligence. So can you kinda connect some of the dots there from from your research and what you shared in your book and from how we got to some of the kind of the touring efforts to where we are in kind of the earlier stages of of AI?
Yeah. Exactly. So so what I was just talking about in, like, eugenics and the Victorian empire, that’s basically part of part one of the book. Yep.
And part two of the book opens up with Bletchley Park and Alan Turing. So, yeah. So it it’s not a story that was told for the first, like, I would say, fifty years after World War two, in in part because in the United Kingdom, state secrets were taken very seriously. And and a lot of the knowledge of what was done in, like, the very first data science problem.
Like, dealing computationally with messy streams of data was was really quite strongly classified until the seventies.
But, yeah, the story we tell in part two of the book is is really the creation of computation around data. So the, you know, arguably, the very first programmable computer was the one at Bletchley Park called the Colossus, which was completely secret for the for the next, like, forty years. And it was created for a data science problem dealing with messy streams of real world data in order to break break codes, and to perform active cryptanalysis or code breaking.
There’s there’s also immediately the creation of sort of an industrial scale of data. So immediately people realize, well, you could build special purpose hardware, but if you really wanna break codes all day every day, you’re gonna build rooms full of computers, and you’re gonna have to collaborate with industry. So, like, right away in the UK and the US, there was collaborations with industry around a data science problem, like, as people were building the first digital computers.
In the US, that mostly happens at Bell Labs, which goes on to really create data science. I mean, the mindset of dealing making sense of messy streams of data on a computer. Like, Bell Labs was was decades ahead of everybody else there, which, no surprise, is is why Claude Shannon had created the mathematical theory of cryptography, which the next year he declassified as the mathematical theory of crypto of information. So it’s basically the birth of information theory.
And a lot of what we know in in data science was born from that tradition. Similarly, IBM benefited from I mean, we would now call it the military industrial complex. Right. We weren’t calling it in the day, but, like, a lot of the first computers and and one of the, co organizers of the very first artificial intelligence workshop was was from IBM, and they were building these machines largely for either the NSA or the organizations that would later become the NSA.
And as they aged out and the NSA moved from the IBM seven zero one to the seven zero four, for example, IBM was like, well, what are we gonna do with these machines? And one of the things they did that was extremely attention getting was acts of machine learning. So the very first part I don’t know if it’s the first time the phrase machine learning was used. It’s kinda debated there. But if you look in dictionaries, they’ll say the first time machine learning was used in this is this one paper from an IBM researcher for playing checkers, and it was a hugely successful demo in, like, nineteen fifty six and then a paper in nineteen fifty nine. And so, there’s really an intimate role between data and computing, but also data computing and this sort of secret history of, military funding and and martial concerns that were really driving the problem, towards towards innovation.
One one of the things that that I found interesting was there there was somewhere and and I hope you can help connect the dots here. There was there was a there was a pivot away from what I would call kind of rules based AI and machine learning. More, you know, kind of, like, you you you could guess what the rules were. And if you and if you could anticipate all the rules and what rules would humans use to make a decision, well, then you could be create something that was artificially intelligent. But somewhere, that kind of broke where it went from kind of rules and heuristics driven to more pattern driven. What was what was that break away from okay. We can we can we can model a human brain by figuring out what rules they use to, let’s go to the data instead, and let’s figure out what the patterns are as a proxy for understanding human behavior.
So part of what I hope readers get from the book is, a reassurance that if terms like artificial intelligence, or even statistics seem confusing, it should be the way because it should be confusing. Because terms like artificial intelligence in nineteen eighty meant nothing, like Right. What artificial intelligence means in twenty twenty three. So part of that is to just look at root causes, like the origin of these terms.
So artificial intelligence as a term is born in nineteen fifty five, and it was created by a mathematician, because he wanted to get money for a study from, what eventually was funded by a Rockefeller Foundation. So we fund funded this workshop, and it was him and other mathematicians who really set the tone for the first couple of decades of artificial intelligence for how artificial intelligence should be should be achieved. Right? So the phrase artificial intelligence doesn’t tell you what method we’re gonna use.
It’s not like I tell you calculus. Right?
Right.
It just tells you what the aspiration is. And for the first couple of decades, the people who really shaped that field were people who thought that it we should get artificial intelligence the way that we think we think. And the way those people thought we think was in terms of schema and representations.
And what should we get artificial intelligence to do? Well, we we should get artificial intelligence to do the things that we think are the highest, aspirations of human intellect, which are proving theorems, right, and other things that are like organizing a business or something like that. So a lot of the emphasis was not on things that we, like, consider AI now, like telling if a picture has a cat face or a dog face in it or something like that, which which was a big, business for artificial intelligence the last, like, fifteen years or, like, to automatically tag an image or something.
That wasn’t what people were going at after in the AI community. By the eighties, it became clear that, like, that was a lot of work. Right? Even doing expert systems, which is a a style of artificial intelligence from the seventies and eighties, required you to go interview an expert and then try to figure out how precisely they are doing what they’re doing and then program into a computer every single brittle rule that an expert uses.
And, you know, a lot of cognitive science today is is countering that. It’s like, truth is we don’t exactly know why we do what we do. Sometimes people make a decision, and then then later, they’ll try to come up with some explanation of why they made that decision and there’s a lot of ways in which that breaks. So the paradigm from the late 90s and certainly from this century, this millennium, is instead to just take a bunch of data and then use the right mathematics to let the data speak.
And that and that’s what we now think of as the machine learning paradigm.
I wanna press on that a little bit more, but since we’re falling kind of a chronology here and I am I’m I’m I’m a Virgo, so I like to go in order. Love it.
You you touched on the nineties. Now now you’re barking right up my tree here with, with with with when I actually started to get involved, professionally. And one of the things that that I found very interesting, it was was your, you know, detail around some of the early years of the Internet and what you what you kind of rightfully call the development of of of persuasion architectures, and how the whole birth of the Internet, the commercialization of the Internet really kind of kind of turned some of I’ll just kind of say turned a lot of the developments here from the data and ML and AI perspective into guessing what people would want, what they would do, and how they would engage, which is particularly relevant in today’s world.
But but going back to nineteen ninety five and nineteen ninety six when I started to get involved here, there was there was it it was a wild west. There wasn’t a lot of rules to find. One of the things that I found most striking in in in your book, which was absolutely positively bang on, was what you said in essence, I’m paraphrasing you now, was that you said that, you know, the the the Internet was largely born out of twenty six words of the Communications Decency Act of nineteen ninety six that allowed Internet service providers to avoid being deemed a publisher of in of information, which is particularly relevant in a world of user generated content now where those publishers don’t have a authority over what people are putting on their platform.
But this happened in nineteen ninety six, and it was formulative.
So so so how do we how do we how does that actually influence what we know today as the Internet? That that those twenty six lines.
Yeah. So the so the other another aspect of the book or we hope a use of the book is to make the present strange, to to make people look back and say, well, how many different things had to come together sort of accidentally in order to create what we now think of as normal? Right? Because in nineteen ninety six, you know, the way we live with our relationship with information wasn’t set yet as norms.
And so a lot of things had to come together, norms, technology, markets, and, and law, right, and regulation.
So so ninety six. Right? So Communications Decency Act of nineteen ninety six was a was an attempt to make sure that the Internet was only sharing decent content, which you can imagine a lot of people wanted to support at the time who could be against decent content. But they had a a carve out that was inserted into the into the act, which was these twenty six words, now called section two thirty, which say that if you’re just the pipes and you’re just transmitting information from one place to another, you shouldn’t be responsible for what the content is.
And you’re right that it would have a complete chilling effect on our whole, well, if not the whole economy, certainly the tech economy. Right? We’ve got trillion dollar companies which, are benefiting from the fact that, like, they don’t have to do, any moderation of content other than a couple of protected types of content. Right?
So there’s, like, copyright violations, like porn and, like, other Slander.
Term liable.
Right. Exactly. Yeah. So in the or certainly in the case of newspapers also, which have extra regulations associated with them.
Right? So there’s a couple of things there. One, we all, like, we all like the first amendment, but that said, like, we all know that there actually are limits on the things you can transmit. For example, the, you know, protected classes we just, enumerated.
And as you just got at, if you’re a publisher, then there’s extra, things that you have to pay attention to. Right? And so that’s why it’s very important. If you are just the pipes that that are transmitting the information, you do not wanna be classified as a publisher because then you will be regulated according to those things.
So I guess another thing we hope people pick up out of the book is that, it didn’t have to be that way at all. And even though even the way we do regulation in the states is so sector by sector. Right? Unlike European regulations, which will just apply to technology horizontally across different sectors, we’ve done things over the last hundred years where, like, finance is regulated in a different way than publishing, which is regulated in a different way than something else.
But, yeah, by looking at these pivotal elements like the nineteen ninety six, CDA, right, we sort of see how things could have been different.
I so I was there at Grand Zero. I worked for this Internet startup called AOL from ninety five to ninety from ninety five to to two thousand and five. And the issue of, are we a publisher, or are we a common carrier? Right? Are we basically an AT and T? Right? This this was this was forefront, with a lot of a lot of what we were kind of trying to figure out at the time.
And those twenty six words really kind of galvanized. Uh-huh. We’re not gonna be held responsible for our users saying libelous things or slanderous things, because that’s their responsibility. If you do something dumb online, it’s gonna it falls to you.
It doesn’t fall to me as the Internet service provider. Right? And I think one could argue, that that alone was what kind of was the jet fuel, to allow the Internet to become what it was to what it is today because, otherwise, I I think it would have been take a lot longer to get to to where we are. So I I I was I was I was just so pleased that you touched on that in the book.
It was fantastic.
But there is kind of a tieback here to today’s world and, and AI, and particularly the use of data, which may or may not be in the public domain to train AI models. So what what are your perspectives on the issue of copyright as it relates today? Now we can we can it’s easy to pick on ChatGPT because a lot of people have have played with it, and a lot of kids are using it to do their homework.
Do you see there being any sort of regulatory focus there eventually?
And and I’ll I’ll I’ll tell this through a kind of an anecdote.
I’ve I’ve asked, of course, like many, I’ve asked chat GPT a lot of questions, and I asked it questions that I used to get as a Gartner analyst. And there was the answers that I got back, Chris, I could have swore I wrote them.
I could have swore that the content I was reading maybe and maybe you did.
Yeah. The content that written something you wrote.
Yeah. And the content that I was reading had come from something that I had assumed was was was copyright.
Do do you see there being, any sort of regulatory issues that will come up? I mean, I know that on image front, like, Getty is is suing, you know, for for that’s a layup. Right?
When you’ve got the the the watermark That’s exactly right.
That is a layup.
Yeah. That’s why. I mean, that that’s a layup. But, like, do you do you see any sort of kind of conflict erupting here between copyright and what’s in what’s happening in the AI world now?
Yeah. You know, it’s so hard to predict the future, but, the thing about the Getty one is is really good though because you literally can see it. Right? The the copyright violation is something you can see in your eyes.
The image and the watermark, and you can see it, like, in some of the images. So it’s harder to see it in the form of, content like an article, unless you ask it to quote something, in which which is also kind of fun, like asking it to quote asking it to quote some document or something like that. It’ll come up, particularly things like, first thing I did with Bard when I got access to Bard was I asked it, okay. What’s on the because unlike chat g b t, Bard is is is not it doesn’t say it in front.
Okay. What all the content is from two thousand one or two thousand twenty one or earlier. So I said to Bart, okay. Well, what is on the front page of The New York Times today?
And it gave me a list of the top stories in The New York Times today. And I said to Bart, Bard, doesn’t that violate, the licensing agreement you have with the New York Times? And it said, I’m sorry. I violated the licensing agreement with the New York Times, which was nice of it to say it.
Sorry. But Yeah. That’s an example where you can sort you can sort of just follow the follow the, logic and you can see.
But technically, I’m not human. So actually, no, I didn’t. Because copyright only only transfers to human beings. It doesn’t actually transfer to a machine.
Right. So so that’s gonna be an issue for lawyers to work out. And, I it’s a good example of the time scales. Right?
I said earlier that things are changed by, you know, markets and laws and technology and our own norms. And each one of those things has its own time scale. So technology clearly moves fast, and then our norms sort of catch up and we’re like, well, it’s a little uncool to send somebody a death notice that was written by Chet Chee Priti. That’s like a statement about how our norms catch up.
Law and, you know, marketplaces then catch up where people are like, okay. I’m gonna sell access to Chet GPT, and then eventually laws catch up. Right? So they so each one of those forces is real, but they all have a different time scale associated with them.
So it’s gonna take a while for the laws to to catch up. And I, you know, I I don’t know that I can make a prediction for how that’s gonna go down.
Getty, I think, is it like we said, it’s it’s it’s obvious. You can see it with your eyeballs, and and everybody’s gonna support, some sort of regulation that protects that. But for things that are like content, it’s it’s literally harder to see, right, the plagiarism. Even plagiarism detection is done statistically usually.
Those, I don’t know how they’re gonna be fought between, you know, it it it’s not like publishers have, like, a big lobby behind them the way tech does. So I’m not really sure how that fight is gonna go down.
Well, it reminds me a lot of the, early days of, kind of the recording industry versus the Internet. Right? And and, you know, peer to peer sharing, of, you know, copyrighted recorded music and how that kind of shook out. And that enabled the creation of digital rights management, which I would argue is, in many cases, a blatant violation of first use doctrine, but separate issue.
So before we before we transition away away from the book and and and AI and and data, one of the things that you were talking about also kind of reminded me recently. I I was watching Sam Altman on, Lex Fridman’s podcast. I I don’t know if you caught that or not.
A a student in my class said I should watch it, but I haven’t read it.
You should you should. It’s it’s a good conversation. For those who don’t know, so Sam Altman is kind of, like, one of the founders of of of OpenAI and arguably kind of one of the driving forces, obviously, not the technical driving forces. There’s many brilliant engineers that are behind it, but but he’s kind of the the spokesperson as it were for for OpenAI and ChatGPT.
And he was he was asked recently by by Lex Fridwin if if if he thought if sent out Altman thought that chat g p t four was was generalized artificial intelligence. So what does that mean to you, Chris, and what what is what’s what’s relevant there? I I I can give my perspective, but you’re you’re you’re the expert, not me.
Sure. Let me give a a a technical definition, and then I give sort of my take on it, or my the lesson that I’ve learned from the literature. So the the technical aspect there is in in early days, you you can look I I always like to look at primary documents. So in nineteen fifty five, when McCarthy was proposing to the Rockefeller Foundation Foundation that he have a workshop on artificial intelligence, he said, the workshop will be dedicated to the hypothesis that all aspects of intelligence can be so precisely described that it can be programmed.
So in in the original incarnation of the phrase artificial intelligence, that was a pretty lofty goal. Right? Every aspect of human intelligence could be so precisely described that you could program it. And the what was in scope there was every aspect of human intelligence, however you wanna define it.
Over the years, you get this sec separate community, which we don’t talk about very much anymore. At the time for in the fifties, it was called the pattern recognition community. It was a much smaller community in which Lou was looking at things like, I hand you a picture, and you tell me whether or not there’s a tank in that picture. I choose that example because this was also largely funded by military concerns.
So pattern recognition is the small community doing what we would now call discriminative learning or building a binary classifier from image data. And that community built the methods that eventually became rechristened as machine learning, and those methods work. Right? And so for a large part of this millennium, the applications that were driving a lot of innovation were things like multi class classification and high dimensional, problems.
So you take, like, an image where the features are every single pixel, and you classify it as being one of the ten digits, for example. That was a test case that really drove a lot of innovation. Or you classify it as being cat face or dog face, or you tag images within those within those images. Those are those are all things that worked, but they are not general artificial intelligence.
So people started using the words strong and weak AI, and then they started using the word general and narrow AI to refer to the ideas that some of the AI we’re doing is, like, hot dog versus non hot dog. It may work really well, but it’s kinda narrow small bore problem. It it it, it’s great on the way towards, but what we really want is general artificial intelligence, something that can ask that can, you know, emulate any aspect of human intelligence. That’s clearly a grand goal.
And to be clear, like, I don’t know that many people who would say that we’ve reached anything. That is actually general artificial intelligence. Although, I haven’t listened to that podcast yet, so I don’t know how Sam answered the question.
In any event, before you tell me, let me tell you what I’ve learned lesson I’ve learned from the literature. For around the same time, we mentioned Alan Turing earlier. So Alan Turing shows up in two subsequent chapters. He shows up in the chapter on Bletchley Park, of course, because he’s famous among other things for code breaking, but in the very next chapter about the birth of artificial intelligence.
And he writes this paper in nineteen fifty saying we would like to investigate if machines can think and right away he says that’s a bad question. I’m gonna replace it with a better question. Can I build the following imitation game? So right away, he operationalizes it.
And often when I hear people debate whether or not we have machines that are intelligent or they have cognition or they’re self aware, I just think, you know, like, we’re not gonna come to consensus. Let’s just agree to a couple of benchmark tasks and operationalize it, and then we have at least something concrete we could talk about. So you can ask questions like, can chat g p four pass the bar exam, which, by the way, it can.
It it does. Yep.
Yeah. So that’s an operationalization, and we can talk about that in a much more concrete term than whether or not it’s intelligent, which I find a little poetic. Because I knew there’s I only have a couple of, you know, years left on this planet. It’s just I don’t I don’t wanna spend hours time thinking about whether something is or is or not really intelligent. So so with that preamble so so what did Sam say?
Well, he said no. It’s it’s it’s it’s not, you know, general artificial intelligence, which which I think, at least for me, for for kind of, you know, not as smart people for me, means kind of logic and not logic. Reason, rationale, plus creativity, plus innovation, coming up with novel solutions that have not previously existed, that one couldn’t necessarily have patterned or predicted.
Mhmm. I I don’t know if that’s it’s useful, but that’s kinda how I think of it anyway.
When I think of kind of just pure pattern recognition, one of the things that I’m I’m slightly maybe worried about, and and I love your perspective on this, is that kind of and and, again, this is maybe a overly pedestrian way of looking at the world.
But if you’re always looking at previous data, to me, the the metaphor here is to, like, I don’t know if you’ve ever driven a boat. But if you’re looking backwards and you’re driving a boat, you can drive a boat off the wake. You can drive it in a straight line. But if you try to deviate from that if you wanna deviate from that straight line, you’re gonna you’re you’re gonna be in for some trouble.
Right? So if you’re only looking back, how do you how do you, based only on previous data, where does the world of kind of true innovation and creative thought live in an AI driven world? Do do you know what I’m asking? Where does true creativity come from if I’m only looking back?
Yeah. So this shows up in a lot of technical literatures.
For statisticians, sometimes they talk about the difference between, extrapolation and interpolation. So you can imagine it just in a curve. Right? And if I hand you a curve and the curve has some finite extent, it’s a lot easier to extrapolate, like, between the points that you have a training time than it is to extrapolate to some regime you’ve never seen before.
So that’s a statistical view. A different statistical view, which is related to natural sciences, but also, you know, economics and policy is the literature on causality. So causality is is is is is one whole literature that gets at what you’re getting at. So, like, in the book, for example, we talk about, when people started using statistics for policy.
I think this is chapter four. One of the first things that happened was somebody looked at how many poor people there were in every region of England and how many people were receiving assistance from welfare and how many people were receiving assistance in a poorhouse.
And there’s a positive correlation between how many people are receiving assistance and how many people there are getting welfare or or sorry. How many poor people there are. And the author of that experiment or that analysis concluded that because those are positively correlated, then giving people help must be causing poverty.
So that’s an example of of correlation and causation and how they can be conflated. It’s a good example because the title of the paper was On the Causes of Pauperism, and then in footnote foot number twenty five in that paper, it says, strictly speaking, you should read this as is associated with, not necessarily causes. So, that’s an example because you do not know what would happen in a world in which you doubled how many people got welfare or how many people, you know, just stopped giving welfare in that particular region. So you can predict things in this world from this one distribution, but it doesn’t necessarily give you a causal model of what would happen in a world in which you had done something that you had never done before.
And then this shows up all the time when people look at the limits of statistical modeling. Right? If you have statistical if you have data, right, and put the data drawn from a limited set of experiments, you really don’t know what would happen in a world in which you you you you introduce an innovation that’s never been done before. So, that’s a real challenge, and and it’s an example of the kind of thing that we should remember to have some humility about what we can do with statistical modeling. We can do a great job predicting other data that are similar to the data on which we’ve trained the model, But in often, we cannot make any predictions for what would happen in a world in which we break, how different observables are related to each other.
Strong argument for a little bit of chaos, in in our future. So that’s that’s a great way to segue away, from from purely talking about the book. But I would most certainly I found it a fascinating read. Would most certainly recommend How Data Happen by Chris Wiggins or any chief data officer out there, particularly if you want to, kind of extract your yourself away from some of the day to day and talk about and think about some bigger picture issues that that most certainly are relevant to what you do day in and day out. Let’s segue into the kind of the doing day in and day out. You are the chief data scientist at The New York Times.
It’s true.
I I recently had some conversations with a number of of CDOs and CIOs who all shared with me. There’s about thirty of them. We were having a kind of a workshop around recruitment and retrain and and training and retaining, data related talent. And a lot of them shared with me that they that they felt like the title of data scientist was kind of falling out of favor and that that may be in and of itself, overly lofty and that so many of them were kind of now aiming towards more kind of utility players and data more data engineer types. Are are you seeing the same? You’ve got a data scientist role. What do you think about that?
So what I’ve seen is things get, more granular. So a good so one of the things that and I shouldn’t leave the book, but one of the things we talk about in the book is, there’s this paragraph from Jeff Hammerbacher, which he wrote in two thousand and eight or two thousand and nine, saying what they did in the data science, team at Facebook. And he said data scientists could be building a multistage processing pipeline, building a data intensive product in Hadoop, doing a statistical analysis in R, and explaining the results to the rest of the organization in a clear and concise fashion. So many of those things have now gotten separate job titles. So, like, statistical testing at the New York Times largely is done by a group around experimentation and involves a lot of the data analysts.
And making sure that people mean the same thing when they start talk about, like, subscription, start and stops, that’s a group of data governance.
Building a multistage processing pipeline, we have a group now called data engineering. And the data scientists are focused on developing and deploying machine learning.
So I I think a lot of it is that the sort of unicorn like aspect of the job title in two thousand eight has now gotten more granular as people realize, oh, actually, each one of those is a separate skill set.
I should say we also try to hire for people that I I feel comfortable having them explain to the rest of the organization in a clear and concise fashion what we’re doing.
So, so there’s been some refinement as people realize that it’s not just sort of all an amorphous blob of data science, but there’s actually separate skills there. And and, like, building a really good data team involves having people who play different roles just like any other team. You don’t have, like, everybody playing shortstop. So, you know, everybody is is playing distinct roles, and and companies are realizing how those roles play well together.
Got it.
And I was interested.
I found the the the quote from from Facebook. The gentleman’s name, Jeff, and I will probably mispronounce his last name. Hammerbacher. Hammerbacher.
Yeah. Hammerbacher. Well, I I’m a fan of the All In podcast, and what and I don’t know if you’ve if you’ve ever watched that, but one of the people there is Chamath Palihapitiya, who was, who I worked with at AOL. Wouldn’t be able to pick me out of a crowd, but I worked with him at AOL.
And he went to Facebook, and I could’ve swore that that he said that he came up with a data scientist title.
He should he should definitely publicize that because most people attribute it to, DJ Patil and to Jeff Jeff Ammerbacher.
He was at Facebook, so maybe it was just he was just in the room. I don’t I don’t I don’t know. Our time is winding up here.
One of the thing one of the thing that I wanted I wanted to kinda to to tie off on was how data science is being leveraged at the at the New York Times. So so describe for me the the output of your work. How would that typically be leveraged within arguably the best known newspaper on the planet?
Sure. So I showed up in twenty thirteen.
And since then, we’ve built out a lot of functions in which data science can be helpful. So one is recommendation engines. So both recommending content in general and also personalizing content, those are done through machine learning algorithms.
Another is building a better paywall. So The New York Times, you know, has transitioned over the last twenty years from advertising based model to a subscription based model. And in particular, digital subscription model is really where the company sees its future, which means you need to have some sort of differentiated experience between free and pay.
So the sort of blunt instrument there is a is a paywall, which when it was launched in twenty eleven, I think the logic there was you get ten articles a month free, and that’s it. And then you have to to pay. So we’re now much more, granular about that and have a lot more flexibility.
And so that’s a machine learning, model that determines when is the appropriate moment for a person to to put up a paywall. And in fact, that’s something that you can optimize and tune between are you trying to optimize between engagement or short term subscriptions where engagement is sort of a proxy for long term subscriptions if you if you believe that people experiencing the breadth of the content will someday make them want to become a paid subscriber.
We have some, innovative ad products that we’ve, created in part to get away from surveillance capitalism. So to get away from the idea that you put a tracking pixel on every page that goes to a third party data broker, and then data broker says to you, I I think this person is a soccer dad or a NASCAR mom or something.
So we’ve built our own innovative ad products that are much more around contextual project contextual targeting.
For example, sentiment deep sentiment analysis that can say to marketers, this is an article that we think will make people feel excited or hopeful, and then marketers can decide if they wanna advertise next to that sorta sorta contextual advertising.
Those are all done by my team.
Bunch of other stuff. Some stuff we’ve discussed publicly, sometimes stuff not. But there’s a lot of things that we can do to improve efficiency and to drive up important KPIs to keep the company strong and independent.
Good stuff. I I’m struck by I’m going back to the book because I’m I’m struck by one of the one of the quotes, and I’m and I’m gonna quote it word for word here. One of the things you said, I don’t know if it was you or your coauthor, but but it it the quote is, what does it mean that our primary source of truth delivered to us in the palm of our hands is funded by and optimized for the surveillance ad model? That that’s something that really, really struck and and stuck with me, particularly when I look at some of the business models, that are out there today.
And and I guess our last question is is do you see a world where some of the business models, whether it’s traditional media, whether it’s the Internet, whether whatever, doesn’t matter, Do you see a world where we break away from some of these, what you call the kind of a surveillance ad model or just advertising driven writ large, Were we able to break away from that into other business models?
Sure. So, I mean, I’ve I’ve a bias here working at New York Times, but one obvious alternative is subscription models. Right. Right.
So you wanna keep a company in business, right, Spotify, Netflix, splitting companies that are doing well, with the dom with the subscription dominant rather than advertising ad model. By the way, I’ll let me come back to nineteen ninety six. Right? Yeah.
So the the so section two thirty made it possible for, tech companies not to worry about what the content was, and that made possible user generated content as the dominant source of content. Now the now that’s great for driving engagement, but you need to make it easy for anybody to use your site, which means you don’t want a subscription model. You want a you want a free model or advertising model. It also means that you’re gonna have so much content that you need some algorithm that’s gonna determine for this person which is the right user generated content from some other RANO on the Internet to show that person, which means you need machine learning.
So so looking back at the last thirty, forty years, how see you know, you can see in retrospect how all these things had to come together, technical decisions, economic decisions, our own norms. You know, the fact that people are like, yeah, advertising. I just expect that to be there. I don’t wanna have to pay for content.
But I think what you’re seeing a lot in the last decade is people saying, well, maybe some content actually is worth paying for rather than having, like, the information assault of a bazillion pieces of user generated content in order to find the one that’s actually useful to me.
And that was the that was the heart of my question because getting back to the previous questions about about AOL and the twenty six words that that founded the inner or that that helped, you know, fuel the Internet.
Yeah.
I think you’re absolutely right. There’s this underlying kind of assumption out there, writ large, whatever that means, that, well, it has to be advertising, and this is the way it is. It’s always been this way.
And when we get into conversations about kind of misinformation, what’s truth, what’s not truth, about persuasion, about people living in these these bubbles where they are only ever fed same the same, you know, ideas and the same concepts and and and all the rest.
That is largely a function of the business model, but there are other models out there. And I’m I’m optimistic that chief data officers, will will figure this out, whether they work in media companies or whether they work somewhere else because there are other worlds out there. I think there is a world for curated content. I think there is a world for authors and innovation and creation when it comes to to online content. We may not be seeing it today, but it didn’t always have to be this way.
Great way to tear off the conversation. Chris Wiggins, thank you so much. It’s my honor to talk to you. So so glad that Jeff connected us.
I could keep going for hours and hours. Again, totally recommend the book. It’s available on Amazon now. It wasn’t about a month ago.
When did you when did you release?
A couple weeks. Tuesday last week. So it’s only it’s only been eight days.
Alright. Fresh hot off the presses. Congratulations on the book. Thanks so much. It’s a fascinating read. Thank you so much for spending time with us today.
Thanks, Malcolm. Appreciate it. Bye bye.
ABOUT THE SHOW
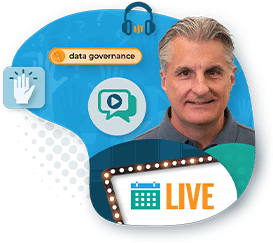
Malcolm Hawker
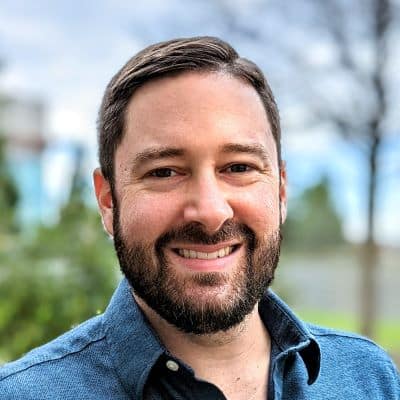
Benjamin Bourgeois
Ben Bourgeois is the Head of Product and Customer Marketing at Profisee, where he leads the strategy for market positioning, messaging and go-to-market execution. He oversees a team of senior product marketing leaders responsible for competitive intelligence, analyst relations, sales enablement and product launches. He has experience managing teams across the B2B SaaS, healthcare, global energy and manufacturing industries.